Developing spatially explicit and stochastic measures of ecological departure
Louis Provencher A * , Sarah Byer A , Kevin J. Badik A and Michael J. Clifford BA
B
Abstract
Ecological departure is a metric applied to mapped ecological systems measuring dissimilarity between the distributions of observed and expected proportions of non-stochastic reference vegetation classes within an area.
We created spatially explicit measures of ecological departure incorporating stochasticity for each ecological system and all ecological systems from a central Nevada, USA, landscape.
Spatially explicit ecological departures were estimated from a radius from each pixel governed by a distance-decay function within a moving window. Variability was introduced by simulating replicate climate time series for each spatial reference condition and calculating departure per replicate.
Single-system spatial ecological departure was high and extensive, except for one area of low-elevation groundwater-dependent systems. Variance of spatial ecological departure was extensively low, except in areas of lower ecological departure, despite vegetation differences among replicates. The multiple-system ecological departure exhibited lower values.
Spatial ecological departure is warranted for efficient land management as results were concordant between non-spatial and spatial metrics; however, rapid coding languages will be required.
Spatially explicit ecological departure of both single and multiple systems facilitate localised vegetation and wildlife habitat management and land protection decisions.
Keywords: central Nevada, USA, fire regime condition, historic range of variation, LANDFIRE, spatial ecological departure, state-and-transition simulation modelling, stochastic reference condition, ST-Sim, Syncrosim.
Introduction
The concept of Fire Regime Condition (FRC), and its categorical version called Fire Regime Condition Class (FRCC), were developed by the US National LANDFIRE project at the request of the US Wildland Fire Leadership Council in 2004 (https://landfire.gov/about.php, accessed 25 June 2022) to prioritise regional and massive funding for decades of fuels management (Rollins 2009; Blankenship et al. 2021). FRC measured the departure between the non-spatial distribution of observed (O) vegetation class proportions of each ecological system on a landscape and the distribution of expected (E) reference vegetation class proportions (Hann and Strom 2003; Shlisky and Hann 2003; Rollins 2009; Blankenship et al. 2021; Swaty et al. 2022). A vegetation class is a categorical representation of an ecological system’s species composition and structure representing pre-settlement succession classes or vegetation altered by European-caused processes (Provencher et al. 2016). For reporting at regional scale, multiple-system departure was summarised as a weighted average of single system values. Greater departure from single or multiple systems implied that more federal funding should be directed to US regions where fuels could be treated to reduce departure (Rollins 2009). The term Ecological Departure (ED; Provencher et al. 2013, 2021) was used here instead of FRC years before LANDFIRE replaced it with Vegetation Departure (Swaty et al. 2022). ED is a dissimilarity index where smaller values (proportions or percentages) indicated greater similarity to the reference condition:
where i identifies classes (i = 1, …, N classes) (Provencher et al. 2008; for an example, see Low et al. 2010). We termed the expected distribution of proportions the ‘reference condition’ representing either pre-European settlement vegetation or contemporary vegetation experiencing natural disturbance regimes under current climate (Provencher et al. 2008; Blankenship et al. 2021; Swaty et al. 2022). Eqn 1 has been non-spatial and non-stochastic since first proposed (Hann and Strom 2003; Blankenship et al. 2015, 2021; Swaty et al. 2022).
Departure between current and reference vegetation was described for the Australian Habitat Condition Assessment System (HCAS) where pairwise remote sensing and abiotic environmental dissimilarity was measured between single focal pixels and pixels sampled from special areas empirically assessed to be observed reference vegetation (Harwood et al. 2016). HCAS, however, is not calculated from a distribution of vegetation class proportions per system and the expected proportions of reference classes were modelled for Eqn 1 but sampled from current reference vegetation in HCAS. Whereas in the United States LANDFIRE mapped ED to inform nationwide resource allocation decisions, The Nature Conservancy partitioned Eqn 1 into its min(Oi, Ei) components to help public land management agencies identify the locations, extent and cost of specific restoration actions in large landscapes (Low et al. 2010; Provencher et al. 2013, 2021). Given the massive government funding influenced by ED, we were puzzled that it has remained non-spatial and non-stochastic since 2003 (Hann and Strom 2003). We propose here to first innovatively develop the spatially explicit component, which is then applied to the stochastic component.
Spatially explicit estimation
The size of an ecological system within a landscape was completely ignored in the estimation of ED because it was based on proportions, not area (Provencher et al. 2008); therefore, distant areas (e.g. >30 km) of the same ecological system within a vast landscape are assumed to have the same ‘cause of departure’, expressed by the min(Oi, Ei) parts of Eqn 1, and a unique non-spatial ED value regardless of the distance among areas contained in a landscape whose boundaries were often administrative. We proposed to centre the estimation of ED on each map pixel (thus spatially explicit, and not only landscape wide) and defined a local radius of single- and multiple-system ED estimation that better matched land management goals or a more species-based perception (i.e. radius of ED estimation) of the local vegetation in which at-risk and hunted species forage.
Stochasticity
The percentages of vegetation classes in Eqn 1 observed from remote sensing have no variability. The real source of stochasticity in Eqn 1 can be assigned to the reference condition (i.e. E proportions), but users only employed temporal average proportions (as in Low et al. 2010). Using standard LANDFIRE methodology, the reference condition was obtained by running to equilibrium pre-Euro settlement state-and-transition simulation models of each ecological system in a landscape (i.e. 500–1000 years; Provencher et al. 2008; Blankenship et al. 2015). Temporal variance within replicates might be biased because observations from different years could be auto-correlated, thus not achieving true replication of the reference condition (Steele et al. 2006), and simulations might not have reached equilibrium in years prior to when a simulation was stopped by the user (also pointed out by Steele et al. 2006). Equilibrium proportions of vegetation classes were only affected by disturbance regimes with minimal random number generator variability built within the simulation software; however, averages were always used, and variance, which was trivial and software-dependent, was often ignored (Shlisky and Hann 2003; Keane et al. 2009; Blankenship et al. 2015, 2021). Ecological processes such as fire change over time and space; therefore, a reference condition dissociated from geology, soils, climate, topography and large mammal herbivory appeared unrealistic (Keane et al. 2009). The single average value for each of the expected percentages of a reference vegetation classes implies more precision than is possible and often sets unreasonable management goals (e.g. using LANDFIRE terminology, ‘we should have 37% of vegetation class A’ instead of ‘we are aiming for 35–45% of vegetation class A’).
Blankenship et al. (2015) used LANDFIRE reference models to introduce temporal variability in non-spatial replicated simulations of reference vegetation class proportions by annual sampling of mean fire return intervals from a Beta distribution. Although this approach allowed the estimation of among-replicate error by vegetation class proportions, ecological disturbances other than fire will vary independently from that of fire in the same year, although disturbances are usually tightly correlated by climate effects in natural systems (Provencher et al. 2016). For example, a year with large area burned of forest caused by severe drought will likely also have enhanced tree mortality due to drought or insect or disease outbreaks. To avoid this problem, one can use external and forecast time series of climate variability obtained from a stochastic weather generator based on historic climate time series and mathematically lock the variability of all ecological disturbances to the same set of external time series (Provencher et al. 2016, 2021).
Objectives
Two objectives were proposed:
Develop a spatially explicit and stochastic method to estimate ED for a real landscape where we expected areas of greater topographic heterogeneity to reduce spatially explicit ED compared with non-spatial ED:
Develop a spatially explicit and stochastic method to estimate multiple-system ED for the same landscape to demonstrate how single versus multiple systems can inform different land management goals.
Methods
Study area
In 2014, The Nature Conservancy mapped ecological systems and their vegetation classes in a 76,828-ha basin and range valley in central Nevada called the Northern Newark Valley in the Basin and Range geologic province (39°53′04.38″N, 115°40′39.19″W; Grayson 1993; Fig. 1). The 2014 map layers were repurposed for the present study. The remote sensing’s vegetation classification can be found in Supplementary File S1, the area per ecological system and vegetation class is presented in Supplementary File S2, and the 2014 mapping methodology is reported in Supplementary File S3, and was generally explained in Provencher et al. (2021) for a different landscape. The original map resolution of 1.5 m (SPOT 6 satellite imagery) was resampled to 60-m resolution (i.e. 213,787 pixels) to keep software computation time reasonable for this study. Vegetation was zonally stratified by precipitation and soils as often observed in the Great Basin ecoregion (Grayson 1993; Chambers et al. 2014). The dominant ecological systems are saline shrublands, sagebrush (Artemisia spp.) shrublands and sub-xeric woodlands. Wet meadows, mountain shrubs, aspen (Populus tremuloides) woodlands and subalpine conifers are scattered across these systems at different elevations.
Methodological overview
The methodology involved four steps: (a) estimating the distance-adjusted proportions of observed vegetation classes per ecological system within a radius of each raster pixel, (b) simulating replicates of the vegetation reference condition for 700 years and estimating the distance-adjusted proportion of expected vegetation classes per ecological system within the same radius of each raster pixel and per replicate at Year 700, (c) estimating the mean and variance of ED by applying Eqn 1 to each map pixel of the observed and expected rasters per replicate; and (d) recalculating the observed and expected distance-adjusted proportions among all ecological systems per map pixel and per replicate and application of Eqn 1.
Estimating observed proportions
To achieve spatially explicit estimation, ED was estimated for each pixel based on the composition of surrounding pixels by first conducting a moving-window search with a defined radius of surrounding pixels from each pixel of the raster. The moving-window search was applied to all pixels of the current remotely sensed system by vegetation class to obtain the observed proportions of all vegetation classes. The same operation was performed on each replicate of the reference condition simulations (see under section Stochastic reference conditions). We scripted in R (R Core Team 2013) the estimation of ecological departure; however, we highly recommend future scripting in the Python language because it handles spatial data more rapidly and with less memory requirements (https://blog.enterprisedna.co/r-vs-python-the-real-differences/; accessed 20 August 2023). The search radius is defined by the user; we chose 900 m or fifteen 60-m pixels for this study. All pixels of the same ecological system within the search radius were counted, and weighted to give less weight to more distant pixels in the following illustrative negative exponential equation from Morrison (2007):
where distance is the distance in metres between the focal pixel at the centre of the moving window and the pixel at ≤1 radius. Users can use any distance weight equation to address their study objectives, including the special case of no distance decay with a weight of 1; we previously used this equation to model the contribution of human-caused fire ignitions from roads (Morrison 2007) to the non-random fire initiation probability raster of the landscape (Provencher et al. 2021). Therefore, Eqn 2 met our fire management goal where the spatial pattern of non-native annual fuels and ED coincided.
For non-spatial ED, the proportion of each vegetation class is the number of pixels of the same class divided by the total number of pixels from all classes in the ecological system. For spatially explicit ED, the value of the same vegetation class pixel as the focal pixel was 1 multiplied by the pixel weight (Eqn 2) as the distance effect from the focal pixel; therefore, the proportion of each vegetation class i of the focal pixel at location [k, p], where k and p were column and row positions, was obtained by summing the weights per same class type and dividing the sum of weights among all classes per ecological system:
where i and j code for the vegetation class i or j (i, j = 1, 2, …, N maximum number of classes); m and n code for column and row position; C and R, respectively, are the maximum number of columns and rows in the raster. For more efficient scripting, pixels at locations m and n stopped being considered if they were farther than the radius distance.
Stochastic reference conditions
The reference condition’s expected proportions were obtained by spatially simulating all ecological systems for 700 years (Blankenship et al. 2015) using the ST-Sim software supported by the Syncrosim platform (version 2.3.12; www.apexrms.com; Daniel et al. 2016). The description of the general modelling methodology is found in Provencher et al. (2021); however, here we removed all post-European settlement vegetation classes (e.g. classes with non-native plant species), disturbances (e.g. cattle grazing) and the effect of fire exclusion on the mean fire return intervals. The default fire return interval in each of the models’ class assumed pre-European settlement rates.
The model also required initial conditions of vegetation rasters be set for the reference condition. The remotely sensed map of current vegetation classes (Supplementary File S2) was used but all uncharacteristic (non-reference) vegetation classes were reclassified as the closest successional reference class from the same ecological systems based on the vegetation classification in Supplementary File S1. In lower and middle elevation upland systems, at least 70% of pixels were uncharacteristic owing to non-native annual grass species invasion of shrublands, conifer encroachment in shrublands caused by fire exclusion and lack of herbaceous understorey from historic livestock grazing. These classes were straightforward to reclassify to the closest reference vegetation class of the same successional phase. Any initial conditions were inconsequential because vegetation classes completely changed over 700 years of succession and disturbances. Roads were converted to No Data (i.e. empty space in the reference map) because it was often not easy to assign ecological systems and vegetation classes to vegetation long ago obliterated. Although roads converted to No Data can act as fuel breaks, wildfires can spread around them and be initiated on either side. For future projects, we recommend reclassifying roads and developed areas to the most likely ecological systems and to the vegetation class predicted to dominate the landscape.
Only disturbances found during pre-settlement were simulated. Fire has been a dominant process in western USA landscapes. Fire spread around natural fuel breaks pushed by prevailing winds greatly determined the spatial variation in the reference condition, which resulted in either early-successional vegetation classes if fire found a path around fuel breaks or late-successional vegetation classes if fire was stopped by fuel breaks. We captured this spatial variation using the moving window estimation previously discussed. Fire directionally spreads following prevailing winds (southwest to northeast is the most frequent direction; Provencher et al. 2021) and the speed of spread among pixels was influenced by slopes facing or opposing prevailing winds (fire spread more rapidly on steeper slopes facing the prevailing winds; Provencher et al. 2021).
The reference condition scenario simulated for 700 years was replicated 20 times (ST-Sim/Syncrosim is a Monte Carlo simulator). Replicates were obtained from different time series of minimum and maximum temperatures and precipitation that were estimated with a stochastic weather generator (Verdin et al. 2015) applied to PRISM climate data (Daly et al. 2008) from 1950 to 2018 (see Provencher et al. 2021). The time series were used to estimate the Standard Precipitation and Evapotranspiration Index (SPEI; Hayes et al. 1999) specified for different months and lags that are relevant to different ecological processes. The SPEI values were used to introduce real variability around the fixed parameter value of each disturbance in the ST-Sim model pathways as explained in Provencher et al. (2016, 2021).
The proportion of each reference vegetation class per ecological system (i.e. the expected proportion) was estimated for each replicate. A replicate was defined by a distinct climate time series (i.e. distinct SPEI time series) from which we only retained the vegetation classes at Year 700 (we verified that values were at equilibrium). The pixel-by-pixel moving window was applied to each reference replicate raster on Year 700 using the same search radius and distance-decay weight function that was used to estimate observed pixels (Eqns 2 and 3). The last step of ED estimation was to apply Eqn 1 to each pixel location using the observed and reference condition proportions in the moving window. A raster map of variance was also estimated by pixel, although any statistical measure of variability would work.
Multiple system ecological departure
The concept of multiple-system ED is a more intuitive assessment of a landscape’s condition because humans and animals look for resources in all possible systems surrounding them. Eqns 1, 2 and 3 were used as before and the simulation of reference conditions was unchanged. What was different in multiple-system ED was how the proportions were organised to sum to 1.
Eqn 3 produced for each ecological system a proportion or percentage per class per ecological system, whereas in multiple-system estimation, we pro-rated that proportion from Eqn 3 by the proportion of the ecological system relative to others in the moving window:
where M is the multiple system proportion for the system by class combination, i, j and p have the same meaning as in Eqn 3, whereas r is the ecological system (r = 1 to Q, where Q is the maximum number of ecological systems in the landscape), which was 27 in Northern Newark Valley. The proportion of each ecological system among all was estimated in the moving window; therefore, small systems at the landscape level will always have more equal representation than large systems within a moving window. Eqn 4 was applied to observed and reference condition vegetation classes, and Eqn 1 was used to estimate ED.
Results
The non-spatial and non-stochastic ED were highly departed from reference condition for most systems, except wet meadow (low departure), greasewood (low departure; Sarcobatus vermiculatus), black sagebrush (intermediate departure; A. nova) and mixed salt desert (intermediate departure) (Fig. 2). No spatially explicit calculations were involved in Fig. 2 as ED values were entirely estimated from tabular data using Eqn 1.
Non-spatial non-stochastic traditional ED for ecological systems of Northern Newark Valley. Lower values indicate that ED of each ecological system in the entire landscape was closer to the reference condition.
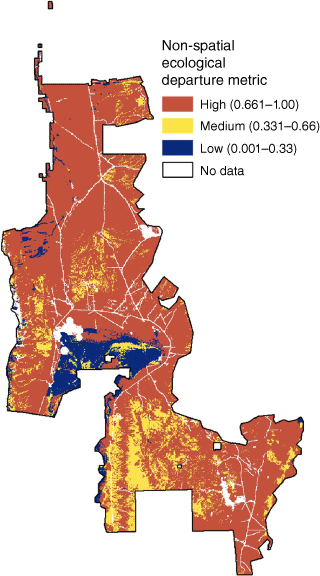
The spatially explicit and stochastic single-system ED was highly departed (closer to 1) over most of the landscape (Fig. 3). Overall, the non-spatial and spatially explicit EDs were similar in areas with less topographic variation (Figs 2 and 3); however, the spatially explicit ED showed several small zones of lower and higher ED, respectively, in systems with high and low departure, thus flipping ED. Only one large area in the south that was primarily dominated by wetland, wet meadow, saline meadow and greasewood shrubland communities was close to reference conditions (blue colour) as also observed for the non-spatial ED (Fig. 2). We observed few if any non-native plant species in those groundwater-dependent plant communities (see Supplementary File S2). Other areas with intermediate spatially explicit ED were generally small and at higher elevations. At lower and middle elevations, ED was higher than at higher elevations because the non-native annual grass Bromus tectorum was ubiquitous in upland communities or sagebrush communities often lacked a native herbaceous understorey (depleted sagebrush) or were encroached by conifers owing to fire exclusion.
Average spatially explicit and stochastic ED for ecological systems of Northern Newark Valley. Lower values indicate that ED of each pixel per ecological system in the moving window was closer to the reference condition. Pixel resolution is 60 m.
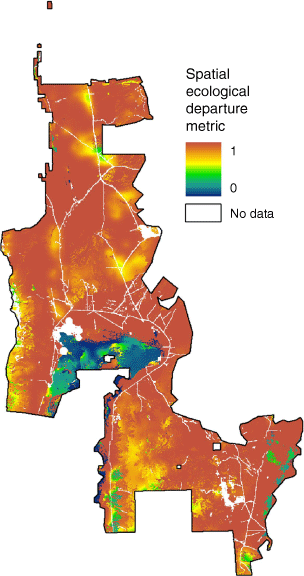
The spatially explicit variance of ED was low (close to 0) over most of the landscape (Fig. 4), which was surprising because we observed large vegetation classes differences among replicates (four shown for illustrative purposes in Fig. 5). Only a few small areas whose pixels’ variance reached up to 0.27 were observed. Several of the higher-variance areas overlapped with low ED values, especially on the western boundary, which suggested an edge effect as prevailing winds were from southwest to northeast (as in Provencher et al. 2021). However, the larger area of ED in the southern part of the landscape showed low variance. This area was one with longer or no natural fire return intervals in wet plant communities.
Spatially explicit variance of ED for ecological systems of Northern Newark Valley. Pixel resolution is 60 m.
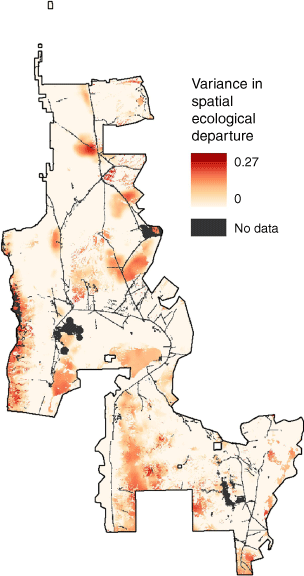
Four replicates (a–d) of zoomed‐in spatial‐explicit reference condition vegetation classes for big sagebrush‐upland with trees of Northern Newark Valley. Different colors represent different vegetation classes. Pixel resolution is 60 m.
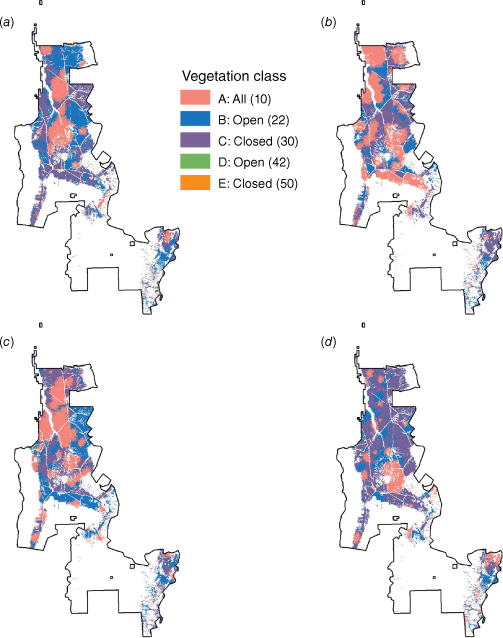
The lowest values of the multiple-system ED (i.e. closer to the reference condition; Fig. 6) at least matched the lower values of the single-system spatially explicit ED (Fig. 3). The multiple-system ED, however, exhibited lower values throughout the landscape (Fig. 3) than the single-system ED (Fig. 6). The highest values of the multiple-system ED, albeit at intermediate ED, were in the valley floors. The pixels with the highest variance in the multiple-system ED also matched the highest values of the single-system spatial ED in the lower elevations; however, variances were slightly lower for multiple-system ED (Figs 4 and 7).
Discussion
The ED metric (Eqn 1) has not changed since it was originally proposed (Hann and Strom 2003) in part because it easily lends itself to the partitioning of vegetation class contributions to ED. ED’s simplicity, however, created impractical management decisions in large landscapes with expansive ecological systems because it operationally assumed a single reference condition per system to aim for and that the full extent of the system was relevant for management actions. The modifications to ED developed here mitigate these limitations by primarily defining a local radius of assessment applied to each pixel governed by a distance-decay function perhaps more akin to animal and human perception of vegetation. Although search algorithms and pixel-based analyses are not novel in ecology and mapping, they have not been applied to Eqn 1 to our knowledge.
An important aspect of spatially explicit and stochastic modelling was that the reference condition changed at each pixel and among replicate simulations depending on random disturbance events shaped by raster-defined prevailing wind directions, topography, fire initiation probabilities, natural fuel beaks, and spread of native insect and disease outbreaks and conifers. Forest scientists working on the spatial LANDSUM model (Steele et al. 2006; Keane et al. 2009) were the first to discuss the spatial variability of reference conditions, but they primarily examined temporal variability within simulations, not alternative future spatial independent replicates of whole landscapes. Moreover, LANDSUM did not generate pixel-based reference conditions because even as a spatial simulation platform, it was not producing pixel-based results. Blankenship et al. (2015) created alternative non-spatial future reference conditions for uncorrelated disturbances, thus achieving true among-simulation replication, but could not extend analysis to pixels and did not estimate ecological departure. The creation of among-simulation replicates of the spatial reference condition appears to not have been done before for large landscapes, perhaps because computational power was not available and pixel-based user-friendly downloadable freeware with documentation, such as the ST-Sim package in the Syncrosim platform we used, did not exist until 2013 (Daniel et al. 2016).
Non-spatial ED was comparable with the single-system spatially explicit and stochastic ED in areas of lower topographic variation but differed with elevation. Localised differences in ED primarily reflected variation in cover of uncharacteristic vegetation classes. At lower elevations, these uncharacteristic classes were widespread in extensive ecological systems; therefore, ED was generally higher and more homogeneous. The uncharacteristic classes were in minority compared with current vegetation dominated by reference vegetation classes at higher elevations in the same systems. This greater heterogeneity at higher elevations meant that ED was lower for the same systems, a feature that would have been impossible to capture with non-spatial ED. The differences in ED with elevation allowed different choices of restoration actions more precisely tailored to areas based on their local departure situated in proximity of ecological values, such as mule deer (Odocoileus hemionus) mountainous migration routes in Newark Valley. The more surprising result was that multiple-system ED generally departed less from the reference condition than single-system ED, although spatial distribution of ED values was similar and spatial variance equally low. We think this reflects the contributions from other systems with less observed uncharacteristic classes that resulted in a lower ED.
Using a moving window weighted by a distance-decay function to evaluate both observed and reference vegetation class proportions has implications for land management. On public lands in the United States, the regulatory justification for proposed actions can be specifically tailored to an agency’s management objective, such as habitat restoration for a wildlife species (NEPA 1970; Provencher et al. 2013, 2021). Moreover, different moving windows can each be easily deployed for different objectives. On private lands, a moving window approach can be used by conservation organisations to evaluate the ecological value of an entire property at different locations based on all ecological systems (i.e. multiple-system ED) within its boundary as protection of land would not be limited to just one ecological system while ignoring others. Moreover, knowing from moving-window analysis that some parts of the property are in worse condition that others could prevent land acquisition because different sources of degradation might forecast the need for very expensive management. Multiple-system ED can be also used to evaluate the vegetation for a species with a broad diet and diverse habitat use, such as mule deer. Mule deer are well known to require a large variety of browse and thermal cover from different systems within the same day. Multiple-system ED might be used to imitate the mule deer’s or other species’ daily perception of resources. A challenge for users will be to determine the moving window’s radius in an extensive landscape with many objectives of widely different radii when the desired approach is to have one or few. Although that question was not addressed in this study, suggestions to investigate might be to: (i) choose the largest home range or propagule dispersal distance among all at-risk species, and (ii) use the classic species-area-curve (Preston 1962) to determine the area needed to include a certain number of species for which data regionally exist.
The moving-window algorithm weighted by a distance-decay function might have minimised the long-known theoretical problem of assessing a landscape of a sufficient area to allow enough disturbances that shape the expression of most successional classes (Hann and Strom 2003; Steele et al. 2006). The reference condition and the observed vegetation were always defined within a moving window in the present study; therefore, the area of the landscape was largely immaterial because the pixels outside the window centred on the focal pixel were not included, whereas the non-spatial ED would be biased in too small a landscape because non-spatially explicit reference conditions always assume the inclusion of all the system’s vegetation classes shaped by dominant disturbances. The size of the landscape, however, still matters but more so in landscapes where the spread of fire is random (e.g. non-spatial simulation), whereas we hypothesised that simulated prevailing winds, topography, natural fuel breaks and non-random fire initiations rendered the role of distant disturbances shaping the reference condition elsewhere in the landscape less important.
Broader applications
The original impetus for the development of a spatially explicit ED was the application to larger geographies (e.g. >809,370 ha) for the management of wide-ranging species at risk, such as greater sage-grouse (Chambers et al. 2014). The assessment of large geographies for the purpose of planning proposed actions creates difficulties because the variation of reference conditions with soil productivity (Keane et al. 2009), the number of different ecological systems to model and the cost of remote sensing mapping ecological systems and their vegetation classes will increase with extent. Successful natural resource management will require a counter effort to lower model complexity and cost of remote sensing.
Model complexity can be lowered by grouping functionally similar ecological systems into one ecologically consistent cluster represented by one state-and-transition simulation model. For example, black and low sagebrush communities might be grouped into ‘Dwarf sagebrush’. Clustering traditional ecological systems makes remote sensing easier because systems only distinguished by different dominant species of the same type (e.g. sagebrush) that look similar from satellite imagery or a helicopter do not need to be separated; only the current vegetation classes need to be interpreted. Additionally, a smaller number of clustered systems might increase stakeholder understanding and acceptance of models. We estimated that clustering systems could reduce the cost of high-resolution remote sensing analysis by 20–30% in Intermountain West vegetation. The consideration of cost of remote sensing to obtain an accurate and high-resolution map was critical because it can easily represent 50% of the total mapping and simulation cost for studies, as described in Provencher et al. (2013, 2021). In the present study, we repurposed existing map layers whose cost was incurred on another project; however, this will generally not be a luxury afforded to other practitioners.
A key challenge with clustered systems is that the reference condition can substantially change along abiotic gradients among systems within a cluster. For example, the fire return interval can vary from 50 years at 2500 m of elevation to 120 years at 1675 m of elevation in a big sagebrush (Artemisia tridentata) cluster in central Nevada because fire-free intervals change with soil productivity (Keane et al. 2009). When the mean fire return interval varies, the proportions of early to late-successional classes will vary too. If the reference condition changes within a cluster, then a non-spatial Eqn 1 for ED is not applicable because Eqn 1 explicitly assumes a single expected value per vegetation class. To eliminate this problem while retaining Eqn 1, the reference condition should be spatially created by multiplying the rate of each disturbance in vegetation models with a multiplier assigned to each pixel of a raster representing an abiotic gradient uploaded into the simulation software (e.g. fire rate controlled by a soil productivity gradient), which is straightforward to achieve in the ST-Sim software (e.g. Provencher et al. 2021). With underlying spatial rasters, the spatially explicit reference condition can be simulated with replication and reference proportions calculated within the same radius as the observed proportions (see Eqns 2 and 3); therefore, a spatially explicit ED will be applicable. Another necessary innovation applied to Eqn 1 for clusters would be to multiply the radius-defined pixel weight of Eqn 2 by an ecological similarity between the focal pixel and the distant pixel to discount ones in dissimilar abiotic environments (e.g. higher elevation) that should not contribute measurable weight to the tally of observed vegetation class proportions. Harwood et al. (2016) also acknowledged this necessary adjustment for the Australian HCAS by multiplying a pixel’s spectral similarity to each reference vegetation pixel with the abiotic factor similarity between the same two pixels. Ecological similarity between pixels ranging from zero to one, perhaps obtained from statistical multivariate ordination (as in Harwood et al. 2016), can be computed from the gradient rasters. Ecological similarity was not part of this study because this similarity only applies to clustered systems.
Data availability
The data and R scripts supporting the findings of this study are available for download from dryad.com @ https://doi.org/doi:10.5061/dryad.3n5tb2rrm. R scripts were also uploaded to GitHub: https://github.com/sbyer-tnc/IJWF-Spatial-ED-Publication.
Declaration of funding
We are grateful to The Nature Conservancy in Nevada and A. L. Kelso for funding. The original mapping was funded by Barrick Gold Corporation as part of the 2015 Barrick–Nevada Greater Sage-grouse Mitigation Bank Enabling Agreement.
Acknowledgements
We thank Kori Blankenship and Jim Smith for reviewing the manuscript before submission. Original mapping was conducted by Spatial Solutions Inc. Anna Richards (CSIRO) suggested the use of the species-area-curve to determine the extent of a moving window. We thank two external reviewers and the Associate Editor whose contributions improved the manuscript.
References
Blankenship K, Frid L, Smith JL (2015) A state-and-transition simulation modeling approach for estimating the historical range of variability. AIMS Environmental Science 2, 253-268.
| Crossref | Google Scholar |
Blankenship K, Swaty R, Hall KR, Hagen S, Pohl K, Shlisky Hunt A, Patton J, Frid L, Smith J (2021) Vegetation dynamics models: a comprehensive set for natural resource assessment and planning in the United States. Ecosphere 12(4), e03484.
| Crossref | Google Scholar |
Chambers JC, Pyke DA, Maestas JD, Pellan, M, Boyd CS, Campbell SB, Espinosa S, Havlina DW, Mayer KE, Wuenschel A (2014) Using resistance and resilience concepts to reduce impacts of invasive annual grasses and altered fire regimes on the sagebrush ecosystem and greater sage-grouse: a strategic multi-scale approach. General Technical Report RMRS-GTR-326. 73 p. (USDA Forest Service, Rocky Mountain Research Station: Fort Collins, CO)
Daly C, Halbleib M, Smith JI, Gibson WP, Doggett MK, Taylor GH, Curtis J, Pasteris PP (2008) Physiographically sensitive mapping of climatological temperature and precipitation across the conterminous United States. International Journal of Climatology 28, 2031-2064.
| Crossref | Google Scholar |
Daniel CJ, Frid L, Sleeter BM, Fortin M-J (2016) State‐and‐transition simulation models: a framework for forecasting landscape change. Methods in Ecology and Evolution 7, 1413-1423.
| Crossref | Google Scholar |
Harwood TD, Donohue RJ, Williams KJ, Ferrier S, McVicar TR, Newell G, White M (2016) Habitat Condition Assessment System: a new way to assess the condition of natural habitats for terrestrial biodiversity across whole regions using remote sensing data. Methods in Ecology and Evolution 7, 1050-1059.
| Crossref | Google Scholar |
Hayes MJ, Svoboda MD, Wilhite DA, Vanyarkho OV (1999) Monitoring the 1996 drought using the standardized precipitation index. Bulletin of the American Meteorological Society 80, 429-438.
| Crossref | Google Scholar |
Keane RE, Hessburg PF, Landres PB, Swanson FJ (2009) The use of historical range and variability (HRV) in landscape management. Forest Ecology and Management 258, 1025-1037.
| Crossref | Google Scholar |
Low G, Provencher L, Abele S (2010) Enhanced conservation action planning: assessing landscape condition and predicting benefits of conservation strategies. Journal of Conservation Planning 6, 36-60.
| Google Scholar |
Preston FW (1962) The canonical distribution of commonness and rarity: Part I. Ecology 43, 185-215.
| Crossref | Google Scholar |
Provencher L, Campbell J, Nachlinger J (2008) Implementation of mid-scale fire regime condition class mapping. International Journal of Wildland Fire 17, 390-406.
| Crossref | Google Scholar |
Provencher L, Anderson T, Low G, Hamilton B, Williams T, Roberts B (2013) Landscape Conservation Forecasting™ for Great Basin National Park. Park Science 30, 56-67.
| Google Scholar |
Provencher L, Frid L, Czembor C, Morisette JT (2016) State-and-transition models: conceptual vs. simulation perspectives, usefulness and breadth of use, and land management applications. In ‘Exotic brome grasses in arid and semi-arid ecosystems of the western US: causes, consequences and management implications’. Springer Environmental Series. (Eds MJ Germino, JC Chambers, CS Brown) pp. 371–407. (Springer: Zug, Switzerland)
Provencher L, Badik K, Anderson T, Tuhy J, Fletcher D, York E, Byer S (2021) Landscape conservation forecasting for data-poor at-risk species on western public lands, United States. Climate 9, 79.
| Crossref | Google Scholar |
R Core Team (2013) ‘R: A language and environment for statistical computing.’ (R Foundation for Statistical Computing: Vienna, Austria) Available at http://www.R-project.org/
Rollins MG (2009) LANDFIRE: a nationally consistent vegetation, wildland fire, and fuel assessment. International Journal of Wildland Fire 18, 235-249.
| Crossref | Google Scholar |
Steele BM, Reddy SK, Keane RE (2006) A methodology for assessing departure of current plant communities from historical conditions over large landscapes. Ecological Modelling 199, 53-63.
| Crossref | Google Scholar |
Swaty R, Blankenship K, Hall KR, Smith J, Dettenmaier M, Hagen S (2022) Assessing ecosystem condition: use and customization of the vegetation departure metric. Land 11, 28.
| Crossref | Google Scholar |
Verdin A, Rajagopalan B, Kleiber W, Katz RW (2015) Coupled stochastic weather generation using spatial and generalized linear models. Stochastic Environmental Research and Risk Assessment 29, 347-356.
| Crossref | Google Scholar |