Examining the effect of moisture thresholds on post-fire water-repellent soil: a large-scale modelling approach applied to the Upper Arroyo Seco watershed, California, USA
Nawa Raj Pradhan
A
Abstract
Post-fire studies show that water repellency is limited by moisture conditions, but no existing study has examined this limiting effect at a watershed scale.
This study aimed to identify the soil moisture threshold value at which wildfire-induced hydrophobic condition transitions back to hydrophilic condition at a watershed scale.
The effect of moisture thresholds on post-fire water-repellent soil and hydrological variables including infiltration, runoff volume and peak flow are examined, using the post-wildfire hydrological model of the upper Arroyo Seco watershed, California, following the August 2009 Station Fire.
As the moisture threshold value increased from wilting point towards field capacity, the wildfire’s impact on runoff was greatest near the wilting point, and decreased sharply as the threshold increased. The percentage error in peak flow exponentially decreased as the moisture threshold increased and the corresponding Nash–Sutcliffe efficiency increased. Soil moisture threshold values >0.2 m3/m3 were significantly less sensitive to Nash–Sutcliffe efficiency, infiltration depth and percentage error in peak flow and runoff volume.
At the soil moisture threshold value of 0.25 m3/m3, transition from hydrophobic to hydrophilic conditions occurred. Identification of this watershed-scale soil moisture threshold value allows inclusion of the wildfire-induced hydrophobic transition back to hydrophilic condition in post-fire hydrological modelling of watersheds.
Keywords: burn severity condition, hydrological modelling, hydrophobic to hydrophilic condition, pre-fire and post-fire, SERVES soil moisture estimation, watershed scale, wildfire impacts, wildfire-induced soil hydraulic factors (WISH factors).
Introduction
Studies suggest that worldwide, wildfires are increasing in severity and frequency (Conard et al. 2002; Jolly et al. 2015; Bowman et al. 2017; Bondur et al. 2020; Brando et al. 2020; Nolan et al. 2020), which can be attributed to human-induced changes leading to climate extremes (Westerling et al. 2011; Adams 2013; Calkin et al. 2015; North et al. 2015; Abatzoglou and Williams 2016; Williams et al. 2019; Halofsky et al. 2020). Wildfires affect the hydro-physical properties of soil (Shakesby and Doerr 2006; Santi and Rengers 2020), including increased soil water repellency and decreased soil hydraulic conductivity, resulting in reduced infiltration and groundwater recharge (Imeson et al. 1992; Cerdà 1998; Certini 2005) and increased runoff (Bixby et al. 2015; Dahm et al. 2015). In vegetated soils, hydraulic conductivity is reduced by over 90%, compared with an unburned location. This can be attributed to an increase in combustion of organic matter and the sealing of soil matrix macro pores (Robichaud 2000; Blake et al. 2010; Neary 2011). Fire may also alter other physical properties of the soil, such as soil structure, texture, porosity and wilting point (Agbeshie et al. 2022). Furthermore, wildfires lead to a decrease in flood attenuation capacity through the removal of riparian vegetation (Benda et al. 2003), increased snow ablation (Harpold et al. 2012), and higher occurrence of landslides and debris flows (Foster et al. 1998; Huffman et al. 2001). This shows that wildfire impacts cascade through hydrological and geomorphological processes across different spatiotemporal scales (Robinne et al. 2018). Therefore, understanding the consequences of wildfires on hydrology necessitates understanding post-fire hydrological processes at relevant scales. Integrating the post-fire hydrological and geomorphological data obtained from remote sensing techniques into a process-based hydrological model enhances our understanding of wildfire effects and can aid post-wildfire mitigation (Miller et al. 2015).
Controlled laboratory and field measurements suggest that the effects of fire on soil water repellency and reduced infiltration decrease with increasing soil moisture content (MacDonald and Huffman 2004). Water repellency (hydrophobicity) in soils is primarily caused by hydrophobic, long-chained organic molecules that are released from decomposing or burning plant litter (Doerr et al. 2000). Soil water repellency is known to be most pronounced when the soil is dry and non-repellent (hydrophilic) when the soil is moist (Doerr and Thomas 2000). Several post-fire hydrological studies at a watershed scale consider soil water repellency and reduced infiltration (Rengers et al. 2016; McGuire et al. 2018; Rengers et al. 2019; Zema 2021). There are also studies that account for the detrimental effect of soil moisture on post-fire hydrological modelling at a watershed scale (Rengers et al. 2016; Rengers et al. 2019; Hoch et al. 2021). The initial soil moisture content affects the initial severity of the water repellent condition (DeBano 2000). In a post-fire scenario, the reduction of infiltration and water repellency is less pronounced when the initial soil moisture content is higher (Doerr and Thomas 2000; MacDonald and Huffman 2004; Rengers et al. 2016, 2019). It is important to note that soil moisture exhibits significant variation throughout a watershed, rather than being uniform (Western and Grayson 1998; Pradhan and Ogden 2010; Dorigo et al. 2021). Therefore, to relate post-fire soil water repellency and infiltration reduction to increasing soil moisture content in a distributed hydrological model at the watershed scale requires incorporating a distributed initial state of soil moisture. To study the transition from hydrophobic to hydrophilic condition, a distributed initial soil moisture condition has not been estimated or applied at a grid resolution in a distributed watershed-scale hydrological model. Moreover, in a distributed hydrological model, discrepancy in the grid scale between the hydrological model development and the input resolution of the initial soil moisture state results in inconsistent outputs from the hydrological model (Pradhan et al. 2020). The present study addresses these scale issues by using a distributed initial soil moisture content derived from satellite imagery to run the post-fire distributed hydrological model used for quantifying the soil moisture threshold value representing the transition from hydrophobic to hydrophilic conditions.
Although there are several studies that shed light on the transition from hydrophobic to hydrophilic conditions (Rengers et al. 2020; Liu et al. 2021; Thomas et al. 2021), only a few studies have attempted to specifically identify the value of this soil moisture threshold (Dekker and Ritsema 1995; Doerr and Thomas 2000; Huffman et al. 2001; MacDonald and Huffman 2004). These studies suggest a wide range at which the soil moisture threshold transitions from hydrophobic to hydrophilic conditions (Dekker and Ritsema 1996; De Jonge et al. 1999; Doerr and Thomas 2000). Scarcity of data, especially in areas burned by wildfire, limits the ability to suggest a soil moisture threshold for the elimination of soil water repellency and the effect on downstream flow conditions (MacDonald and Huffman 2004; Wang et al. 2020). Existing studies on soil moisture thresholds are limited to the observations made at a few sites. Studying hydrological responses to fire at the watershed scale is more complex compared with smaller scales, largely owing to the challenges associated with installing and maintaining data observation instruments that could capture the spatial heterogeneity of environmental factors.
This study identifies the soil moisture threshold value for the transition from hydrophobic to hydrophilic conditions at the watershed scale through the integration of distributed initial soil moisture conditions and a distributed post-fire hydrological process (Pradhan and Floyd 2021) with the physics-based Gridded Surface Sub-surface Hydrological Analysis (GSSHA) model (Downer and Ogden 2004; Pradhan et al. 2020). Pradhan and Floyd (2021) developed the post-fire hydrological model of the Arroyo Seco watershed covering 41.7 km2 in Los Angeles County, CA, USA. This model introduced reduction and burn severity factors as multipliers for soil hydraulic conductivity in the soil characteristic curve of the infiltration/runoff-generation process. The Arroyo Seco model deployed SERVES (Pradhan 2019), Soil-moisture Estimation of Root-zone through Vegetation-index based Evapotranspiration-fraction and Soil-properties, which is a method for estimating distributed initial soil moisture conditions based on soil properties and vegetation cover under burned and unburned conditions. Additionally, the model also adjusts the runoff routing parameterisation according to the burn severity condition (low, medium or high). The hydrological model of the Arroyo Seco watershed was deployed to study the critical soil moisture threshold for transitioning from a wildfire-induced condition of reduced soil infiltration caused by hydrophobicity back to a normal condition without this wildfire-induced hydrophobicity in the soil. The main purpose of this study is to identify the soil moisture threshold value at and above which post-fire effects on watershed-scale hydrology become insignificant. Identification of this watershed-scale soil moisture threshold value allows the inclusion of the wildfire-induced hydrophobic condition transition back to hydrophilic in post-fire hydrological modelling of a watershed.
Materials and methods
This section is organised as follows: Study site, Data, Upper Arroyo Seco pre-fire hydrological model, and Upper Arroyo Seco post-fire hydrological model. The first two sections describe the study area and the data sources for the watershed modelling. The third section describes the hydrological model in the pre-fire condition and the final section describes the hydrological modelling in the post-fire condition. The sub-section in the latter further elaborates on the requirements for post-fire hydrological modelling, including: (i) post-fire soil moisture conditions, (ii) Wildfire Induced Soil Hydraulic factors (WISH factors), and (iii) identification of the hydrophobic to hydrophilic soil moisture threshold. Detailed descriptions are provided in the following sections.
Study site
The Upper Arroyo Seco watershed (Fig. 1) experienced extensive burning, with approximately 95% of the area impacted by the August 2009 fire event named Station Fire. This fire event resulted in a significant increase in water repellency and infiltration excess runoff during the post-fire rainfall events (Schmidt et al. 2011; Chen et al. 2013; Liu et al. 2021). This watershed is located in northeast Los Angeles County, between the San Gabriel Mountains and the Los Angeles River and is a sub-watershed of the Los Angeles National Forest. The climate of this region is semi-arid Mediterranean (Liu et al. 2021), characterised by long dry summers and wet winters, with 95% of the precipitation occurring between November and April. This climate, combined with factors like extreme precipitation events, watershed geology, geomorphology, vegetation and soil type make the region prone to wildfires, like other watersheds in southern CA (Lucas-Borja et al. 2020; Liu et al. 2021; Michaelis et al. 2022). The average annual precipitation in the watershed ranges from approximately 500 mm at the lower elevations to 760 mm at the higher elevations (North East Trees and Arroyo Seco Foundation 2002). The outlet of the watershed is located at 34°13′20″N and 118°10′36″W, resulting in a watershed drainage area of 41.7 km2 (Fig. 1).
Data
The GSSHA hydrological model of the study area (Fig. 1) was developed from a 30 m digital elevation model (obtained from the US Geological Survey (USGS) National Elevation Dataset) that was resampled to a grid resolution of 90 m. The data were downloaded through the National Map Viewer (http://nationalmap.gov/viewer.html).
The Google Earth Pro Imagery (Fisher et al. 2012) at 34°14′35″N and 118°08′50″W (https://www.google.com/earth/index.html, accessed 1 January 2021) showed that the channel width in Fig. 1 varied from 1 m at the starting point to 15 m towards the outlet, with an average effective width of 5 m. This effective width was used for the channels represented as trapezoidal cross-sections.
A GSSHA model’s parameter values associated with the hydrological processes are based on gridded land use and soil datasets. Changes to hydrodynamic and geophysical processes, along with associated parameter behaviour in a post-fire condition, result from the loss of vegetation and soil organic matter (Kinoshita and Hogue 2011). Therefore, analysing post-fire hydrology requires considering changes in land cover and the conditions of the burned soil. The burn severity map (Fig. 2) was generated using the Burned Area Reflectance Classification (BARC) method, which categorises areas into high, moderate or low burn severity or unburned based on the relationship between near- and mid-infrared reflectance values derived from satellite imagery (Parson et al. 2010). The burn severity map (https://www.mtbs.gov/viewer/index.html?region=all, accessed 1 January 2021) shows that 95% of this study area was affected by the Station Fire of August 2009; 18% experienced the low-burn severity condition, 42% the medium-burn severity condition and 35% the high-burn severity condition (Fig. 2). The land-use type data (Fig. 3a) was sourced from the National Land Cover Database (NLCD), and depict the land cover prior to the 2009 wildfire (http://www.mrlc.gov/, accessed 1 January 2021). Fig. 3b shows the final post-fire land-cover map obtained by overlaying Fig. 2 on Fig. 3a. Fig. 3a demonstrates that vegetation constitutes 95% of the land use type, of which more than 75% is shrub. Therefore, in deriving Fig. 3b, the burn severity condition is defined irrespective of the vegetation type.
The Natural Resources Conservation Service (NRCS) Soil Survey Geographic Database, SSURGO (http://websoilsurvey.sc.egov.usda.gov/App/WebSoilSurvey.aspx, accessed 1 January 2021) was used to identify sandy loam, a coarse-textured soil type (Liu et al. 2021), as the dominant soil type within the watershed (George and Lewis 1980). Values for the physical properties of the soil, including wilting point and field capacity, were obtained from this soil texture dataset using pedotransfer functions based on soil parameter values (Rawls et al. 1983). The final post-fire soil map (Fig. 4) was obtained by overlaying the burn severity map onto the uniform sandy loam soil map (Fig. 2). Each soil burn condition in this post-fire soil map (Fig. 4) is defined by a unique number, known as an index value. An index map is an ASCII file that contains index values corresponding to a particular location. This map links a soil type with associated soil parameter values in the GSSHA numerical simulation process.
The observed hourly discharge from USGS gauging station 11098000 near Pasadena (https://waterdata.usgs.gov/nwis/inventory/?site_no=11098000&agency_cd=USGS, accessed 1 January 2021) was used for calibration, parameter identification and verification of the post-fire watershed hydrological model. The hourly precipitation data employed in this study were obtained from the Los Angeles County Department of Public Works. The rain gauge station (Inspiration Pnt Precip’ gauging station) is located at 34°13′18″N and 118°06′34″W, at an elevation of 1366.7 m above sea level (https://www.ladpw.org/wrd/precip/index.cfm?Product=alertlist, accessed 1 January 2021). Both pre- and post-fire rainfall-runoff events were used in this study. The 10-h rainfall-runoff event of 4 January 2008, which started at 1:00 pm Pacific Standard Time (PST), was used as the pre-fire rainfall-runoff event. The 10-h rainfall-runoff event of 27 February 2010, which started at 12:00 am PST, was used as the post-fire rainfall-runoff event.
Upper Arroyo Seco pre-fire hydrological model
Pradhan and Floyd (2021) developed the Upper Arroyo Seco watershed GSSHA hydrological model, incorporating processes such as infiltration (Green and Ampt 1911), soil moisture accounting (Pradhan et al. 2020), two-dimensional hillslope/overland diffusive wave routing and one-dimensional diffusive wave channel routing. To numerically define these processes and identify the parameter values, the post-fire infiltration and routing process used the burn severity map overlaid on the land-use and soil maps shown in Figs 3b and 4, respectively. The pre-fire infiltration and routing processes used the land-use map in Fig. 3a with a uniform sandy loam soil. The infiltration process employed pedotransfer functions defined by Rawls et al. (1983) to estimate soil water property parameter values for the uniform sandy loam soil, as shown in Table 1. In both the pre-fire and post-fire scenarios, the Manning roughness parameter values for the two-dimensional hillslope/overland routing and one-dimensional channel routing were taken from the literature (Chow 1959; Engman 1986). The initial distributed soil moisture conditions for the pre-fire calibration were estimated for the January 2008 event (Fig. 5a) using SERVES (Pradhan 2019).
Soil infiltration parameter value for sandy loam | Manning roughness value for land-use types | |||
---|---|---|---|---|
Soil physical property | Value | Land cover type and condition | Value (s/m1/3) | |
Saturated hydraulic conductivity (cm/h) | 0.81 | Woody wetland | 0.14 | |
Capillary head (cm) | 11.0 | Developed open space | 0.15 | |
Porosity (m3/m3) | 0.41 | Developed low intensity | 0.15 | |
Pore distribution index (cm/cm) | 0.37 | Barren land | 0.20 | |
Residual point (m3/m3) | 0.04 | Evergreen forest | 0.45 | |
Field capacity (m3/m3) | 0.2 | Mixed forest | 0.45 | |
Wilting point (m3/m) | 0.09 | Shrub | 0.44 | |
Grassland | 0.43 |
Upper Arroyo Seco post-fire hydrological model
Pradhan and Floyd (2021) developed a formulation for post-fire conditions by incorporating multiplying factors explicitly linked to the wildfire burn severity conditions. These multiplying factors reduce the hydraulic conductivity from the unburned soil condition and are applied in the infiltration process unsaturated soil characteristic curve. To limit water repellency during wetter post-fire conditions (Rengers et al. 2016, 2019; McGuire et al. 2018; Zema 2021), a soil moisture threshold was introduced into the formulation of the wildfire-induced soil hydraulic factor. A distributed initial soil moisture level, based on satellite imagery and soil properties formulation, was used. In the post-fire routing process, the hydraulic Manning roughness at a location in the watershed varies according to the burn severity condition (Pradhan and Floyd 2021). Further details regarding the initial soil moisture content, wildfire-induced soil hydraulic factors and soil moisture threshold formulation are discussed in the following sections.
Post-fire soil moisture condition
To estimate pre- and post-fire distributed soil moisture conditions, SERVES (Pradhan 2019; Pradhan 2023) was used. Fig. 5 illustrates SERVES estimates of soil moisture for both the pre-fire and post-fire conditions. The soil moisture was originally estimated at a resolution of 30 m and then resampled to a 90 m resolution. Fig. 5a, b represents the soil moisture levels for January 2008 and 2010, respectively. The soil moisture in the pre-fire condition, shown in Fig. 5a, is significantly higher than the estimated soil moisture in the post-fire condition illustrated in Fig. 5b. This demonstrates the ability of wildfires to reduce soil moisture (He et al. 2021).
SERVES estimates distributed soil moisture globally using a vegetation index-based evapotranspiration fraction and soil properties at a scale of 30 m grid resolution. The SERVES method is computationally straightforward and employs widely available web-based digital data (i.e. vegetation indexes from the joint NASA and USGS Landsat program (https://espa.cr.usgs.gov/index/, accessed 1 January 2021) and soil properties from the SSURGO database. The SERVES method/model successfully estimated the effective root zone soil moisture at several locations in the arid and semi-arid regions of United States, specifically in Idaho, Montana and Wyoming (Pradhan 2019). The climate of the Arroyo Seco watershed is also considered semi-arid, characterised by long, hot and dry summers.
In the SERVES method, the relationship between reference evapotranspiration fraction, ETrf, and soil moisture is defined as (Pradhan 2019):
where θ is soil moisture content, θfc is field capacity soil moisture content, θwp is wilting point soil moisture content, and i is any spatial location, grid or Triangular Irregular Networks (TIN) address, for a numerical model.
From the parametric uncertainty analysis of the linear deterministic relationships between ETrf and the normalized difference vegetation index (NDVI), the likelihood function of evapotranspiration fraction inversion through NDVI is defined as (Pradhan 2019):
Eqn 2 considers the change in the evapotranspiration fraction, ETrf, in a post-fire condition. ETrf is significantly reduced in areas with a burned-out canopy, indicating a dried-out environment under these burned conditions. The SERVES estimated soil moisture was used as an initial condition in the GSSHA runs. The GSSHA model was deployed to drive physics-based distributed watershed hydrological simulations of rainfall and runoff events.
Wildfire-induced soil hydraulic factors, WISH factors
To account for the relationship between burn severity and the corresponding reduction in soil hydraulic conductivity, Pradhan and Floyd (2021) developed a formulation for post-fire conditions that includes multiplying factors to reduce the hydraulic conductivity in the unburned condition. These multiplying factors are: (1) the reduction factor of hydraulic conductivity under extreme burn conditions, and (2) the burn severity factor. By multiplying these factors with the vadose zone unburned soil hydraulic conductivity soil characteristic curve, the resulting soil hydraulic conductivity for burned conditions can be obtained:
where Kburned is the hydraulic conductivity of the soil under burned conditions, Kunburned is the soil hydraulic conductivity under unburned conditions, Kburned ≤ Kunburned, BDF is the burn degree factor, and RFk is the reduction factor of hydraulic conductivity under high burn severity conditions.
The burn severity map (Fig. 2) is used to define BDF in Eqn 3. BDF serves as a calibration parameter, with values of 1, 2 and 3 assigned to high, medium and low burn severity cases in the study watershed, respectively (Pradhan and Floyd 2021). RFk refers to the maximum reduction of soil hydraulic conductivity under the high burn BARC classification, and through calibration, Pradhan and Floyd (2021) found this value to be 0.1. RFk = 0.1 indicates a maximum reduction of 90% in soil hydraulic conductivity under high burn severity conditions, which aligns with the findings of Blake et al. (2010). Further research on the reduction of soil hydraulic conductivity for different soil types under severely burned conditions (Blake et al. 2010) would aid in identifying the RFk as the maximum reduction factor of the hydraulic conductivity for a soil type. Just as actual soil moisture cannot exceed a soil’s porosity, the actual soil hydraulic conductivity reduction factor cannot exceed RFk. The actual dynamic soil hydraulic conductivity reduction factor is both space- and time-dependent as follows:
The space-dependent actual reduction factor of the soil hydraulic conductivity is determined by multiplying the maximum soil hydraulic conductivity reduction factor (RFk) and the burned degree factor (BDF), shown in Eqn 3, for a particular spatial location.
The time-dependent actual dynamic soil hydraulic conductivity reduction factor is obtained by multiplying the maximum soil hydraulic conductivity reduction factor (RFk) and the dynamic burned degree factor (BDF). The dynamic BDF can be obtained from the temporal changes in the burn severity condition, which occur during the watershed recovery stage. As this study focuses on rainfall event-based simulations rather than long-term simulations, the BDF remains constant at a particular location throughout this rainfall-runoff simulation.
These two points demonstrate the explicit link between the reduction factor formulation introduced by Pradhan and Floyd (2021) and the spatial and temporal aspects of post-fire physical properties, soil type and burn severity.
The unsaturated soil hydraulic conductivity (Kunsaturated) in Eqn 3 is defined as (Brooks and Corey 1964):
where θ is water content of the soil, θs is saturated water content of the soil, θr is residual water content of the soil, λ is soil distribution index.
The watershed soil and burn severity maps are combined to show the spatial location of burned soil. Eqn 3 is used per the combined soil and burn severity map.
Hydrophobic to hydrophilic soil moisture threshold identification
Initial soil moisture condition in this study is based on the SERVES estimated fine-resolution distributed soil moisture conditions. The model soil moisture condition is processed from this initial condition during the simulation runs. The soil moisture threshold values were selected in this study to limit the effects of wildfires on hydrology during the model run as:
where θt is the soil moisture threshold value.
To identify a consistent value of θt at watershed scales, model runs were performed with increasing θt values from wilting point. For each model simulation, the percentage error in peak flow and runoff volume (Wałęga 2016) and Nash–Sutcliffe efficiency were estimated.
Results and discussion
The simulated results from the watershed model and the analysis of these results are presented as follows: pre-fire hydrological calibrated model results; post-fire hydrological model results with soil moisture threshold; model sensitivity to varying soil moisture threshold values in the post-fire calibration event; and model sensitivity to varying soil moisture threshold values in the post-fire validation event. The post-fire hydrological model is built on the pre-fire hydrological model, incorporating the changes in the land-use type and soil map due to burned conditions. Therefore, it is crucial to have a well-calibrated pre-fire hydrological model. Simulated runoff from the pre-fire calibrated hydrological model is compared with the pre-fire calibrated hydrological model with added post-fire hydrological processes for a post-fire rainfall event. Finally, the effect of moisture thresholds on post-fire water-repellent soil is examined through a sensitivity analysis of the post-fire model results to varying soil moisture threshold values in the post-fire calibration and validation events. Through this analysis, a soil moisture threshold value for the post-fire watershed hydrological model was identified. Results and discussion are further extended in the sections: ‘Physical basis of the wildfire-induced soil hydraulic factors formulation and the soil moisture threshold in post-fire watershed hydrological modelling’, ‘Process dominance in the post-fire runoff simulation’ and ‘Vegetation interception’.
Pre-fire calibrated hydrological model results
Table 1 presents all model parameter values for the pre-fire rainfall event. The parameter values, with the exception of saturated hydraulic conductivity, are identified as discussed in the section ‘Upper Arroyo Seco pre-fire hydrological model’. The value for saturated hydraulic conductivity resulted from calibration. The Nash–Sutcliffe efficiency of this pre-fire hydrologic model, as shown in Fig. 6a, is 88%.
Post-fire hydrological model results with soil moisture threshold
The pre-fire calibrated hydrological model was used to build the post-fire hydrological model, incorporating the changes in land-use type and soil resulting from burned conditions. In the post-fire hydrological model development, the roughness values in the routing process were changed according to the burned conditions shown in Table 2. Eqn 5 was used to incorporate the reduction in infiltration caused by wildfires into the post-fire hydrological model.
Burned condition | Infiltration process | Routing process | ||
---|---|---|---|---|
Hydraulic conductivity reduction factor (RFk) | Burned degree factor (BDF) | Manning roughness value (s/m1/3) | ||
High | 0.1 | 1 | 0.15 | |
Medium | 0.1 | 2 | 0.18 | |
Low | 0.1 | 3 | 0.2 | |
No burn | – | – | As per Table 1 |
The multiplying factors in Eqn 5 were applied to the pre-fire soil hydraulic conductivity, as shown in Table 1. A soil moisture threshold of 0.25 m3/m3 was used as a constraint in Eqn 5. The Nash–Sutcliffe efficiency for this post-fire hydrologic model, shown in Fig. 6b, is 97%. Applying the pre-fire calibrated Arroyo Seco model directly to this post-fire rainfall event produced no simulated runoff. This pre-fire calibrated model is meant for hydrophilic conditions where wildfire-induced water repellency is absent. Several studies following the Station Fire in the San Gabriel Mountains suggest that the dominant runoff in the region is infiltration excess overland flow. Therefore, the transition from hydrophobic to hydrophilic conditions in the current study aims to eliminate soil water repellency due to increased soil moisture level. This transition does not imply a shift in the dominant runoff generation mechanism of the watershed from infiltration excess (Schmidt et al. 2011; Chen et al. 2013; Liu et al. 2021) to saturation excess during post-fire rainfall events.
Bayad et al. (2020) show a decreased persistence of soil water repellency (SWR) from the point scale test of water drop penetration time (WDPT) as a function of water content (θ). Their observations indicate that increasing soil moisture results in a decrease in WDPT, with WDPT reaching a very low value for the majority of tested soil types above a soil moisture content of 0.2 m3/m3. Based on these findings by Bayad et al. (2020), the present study considered a soil moisture threshold of 0.25 m3/m3 as the transition point from hydrophobic to hydrophilic conditions.
Table 2 shows the burned degree factor values for Eqn 5, as identified by Pradhan and Floyd (2021). In their study, these identified BDF values are considered constant for the watershed. A possible future research area could include testing and verifying whether the BDF values identified in Table 2 apply to other burned watersheds.
By deploying Eqn 5 with a soil moisture threshold constraint of 0.25 m3/m3 and a pre-fire calibrated soil saturated hydraulic conductivity of 0.18 cm h−1 (Table 1), RFk was used as a calibration factor. Table 2 displays the calibrated RFk value of 0.1, which aligns with the value identified by Pradhan and Floyd (2021) but with a higher Nash–Sutcliffe efficiency of 97% (Fig. 6b). Therefore, the application of the soil moisture threshold constraint in Eqn 5 produced an optimised simulation result with higher efficiency. The value of RFk = 0.1 signifies that the maximum reduction in soil hydraulic conductivity is 90% compared with the pre-fire soil hydraulic conductivity, which is in agreement with the findings of Blake et al. (2010).
Model sensitivity to varying soil moisture threshold values in the post-fire calibration event
The post-fire calibrated model run was executed by individually setting the soil moisture threshold in Eqn 5 to values of 0.11–0.41 m3/m3 (porosity) at an interval of 0.02 m3/m3. This analysis, referred to as a soil moisture sensitivity analysis for identifying the transition from hydrophobic to hydrophilic conditions, examined the impact of changing the soil moisture threshold value. The results were analysed based on: (a) simulated infiltration, and (b) simulated hydrograph.
The total catchment infiltration at the end of the rainfall event simulation was examined for different soil moisture threshold values used in Eqn 5 (Fig. 7). The catchment’s total infiltration is highest for the soil moisture threshold value near the wilting point. This high sensitivity of infiltration to a low soil moisture threshold value is reduced at higher values. Above the soil moisture threshold value of 0.21 m3/m3, the sensitivity of infiltration to changes in the soil moisture threshold becomes almost negligible (Fig. 7). We also examined the grid-based distributed cumulative infiltration at the end of the simulation for different soil moisture threshold values. The watershed hydrological model consists of 5124 90 m grids within the watershed. The cumulative infiltration in each grid for different soil moisture threshold values is presented in Fig. 8. At a soil moisture threshold value of 0.11 m3/m3, all of the grids in the watershed had cumulative infiltration values greater than 0.0314 m. This results from not considering the post-fire reduction in hydraulic conductivity above the soil moisture threshold value of 0.11 m3/m3. As the soil moisture threshold value gradually increased, the filtered-out lower cumulative infiltration values began to appear. Over the threshold value of 0.2 m3/m3, the distribution of cumulative infiltration values was consistent. Observations by Bayad et al. (2020) also showed that an increase in soil moisture decreased the WDPT (decreased water repellency and increased infiltration). For the majority tested soil types, WDPT decreased to a very low value, just above the soil moisture content of 0.2 m3/m3.
When the model was adjusted with different soil moisture thresholds, the resulting simulated hydrographs with values above the threshold of 0.2 m3/m3 were closer to the observed hydrograph and to one another compared with the simulated hydrographs with threshold values lower than 0.2 m3/m3 (Fig. 9a). The percentage error in runoff volume decreased from 99 to 3% when the soil moisture threshold was increased from 0.11 to 0.2 m3/m3 and remained consistent for soil moisture thresholds above 0.2 m3/m3. The Nash–Sutcliffe efficiency steeply increased to the highest level when the soil moisture threshold was increased from 0.11 to 0.2 m3/m3 and remained consistent for soil moisture thresholds above 0.2 m3/m3 (Fig. 10a). The percentage error in peak flow decreased exponentially when the soil moisture threshold value, θt, was increased from 0.11 to 0.25 m3/m3 (Fig. 11a). Eqn 6 represents the relationship between the percentage error in peak flow and the soil moisture threshold value (0.11–0.25 m3/m3). The peak flow error estimated by Eqn 6 has a coefficient of determination (R2) of 0.9873.
where PFE is peak flow error in percentage.
The simulated hydrographs, (a) rainfall event of 27 Feb. 2010, and (b) rainfall event of 18 Jan 2010, at different soil moisture threshold values (m3/m3).
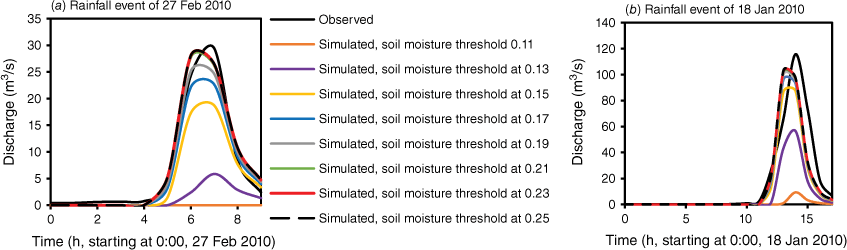
The Nash–Sutcliffe Efficiency of the simulated hydrographs, (a) rainfall event of 27 Feb. 2010, and (b) rainfall event of 18 Jan 2010, for different soil moisture threshold values.
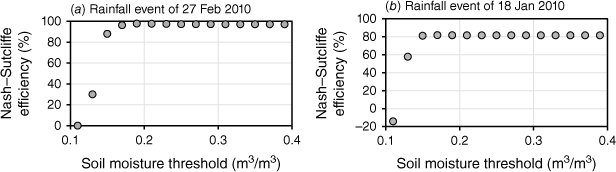
The peak flow error of the simulated hydrographs, (a) rainfall event of 27 Feb. 2010, and (b) rainfall event of 18 Jan 2010, for different soil moisture threshold values.
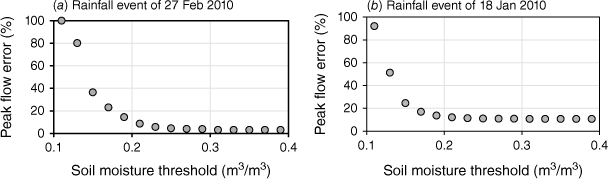
When the soil moisture threshold value is set near the wilting point, the total infiltration in the catchment is highest, resulting in the lowest peak flow. This high sensitivity to infiltration diminishes when the soil moisture threshold values are increased (Figs 7 and 8), causing the peak flow error to diminish exponentially (Eqn 6). Moody et al. (2009) also showed the exponential decay behaviour of soil hydraulic properties, like sorptivity, after a fire. They observed this exponential decay as a function of the initial soil moisture content.
Model sensitivity to varying soil moisture threshold values in the post-fire validation event
The soil moisture threshold analysis conducted above for the post-fire rainfall event of 27 February 2010 was also replicated for the post-fire rainfall event of 18 January 2010 in the watershed. The main purpose of repeating this analysis for another post-fire event was to validate the consistency of the soil moisture threshold analysis across multiple post-fire events within the same watershed. The same multiplying factors specified in Eqn 5 (values shown in Table 2) were used in this event, along with the same post-fire hydrological parameter values.
An analysis of the simulated hydrograph with different soil moisture thresholds is shown in Fig. 9b. The simulated hydrographs above the soil moisture threshold of 0.2 m3/m3 are close to the observed hydrograph and to one another. However, this is not the case for simulated hydrographs at soil moisture threshold values lower than 0.2 m3/m3 (Fig. 9b). This analysis aligns with the simulated results and analysis presented in Fig. 9a. When the soil moisture threshold was increased from 0.11 to 0.2 m3/m3, the Nash–Sutcliffe efficiency also increased from −14 to 82% and remained consistent for soil moisture thresholds above 0.2 m3/m3 (Fig. 10b), which is consistent with the conclusions drawn from Fig. 10a. Under the same conditions, the percentage error in runoff volume (Fig. 12) decreased from 95 to 14% and remained consistent for soil moisture thresholds above 0.2 m3/m3.
The total runoff volume error of the simulated hydrographs of 18 Jan 2010 rainfall event for different soil moisture threshold values.
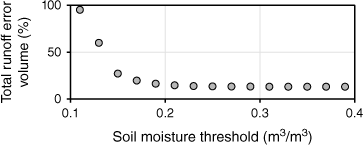
The peak flow error (Fig. 11b) for the corresponding simulated hydrograph of the 18 January 2010 rainfall event at different soil moisture threshold values shows a similar trend of decrease as shown in Fig. 11a for the post-fire rainfall event of 27 February 2010.
Physical basis of the wildfire-induced soil hydraulic factors formulation and the soil moisture threshold in post-fire watershed hydrological modelling
The exponential decay in the peak flow error (Eqn 6 and Fig. 11a) and the steep increase in the quality of the simulated hydrograph (Fig. 10a) show that a wildfire’s effect on post-fire runoff generation is most pronounced at or below the wilting point. This effect diminishes as the soil moisture content increases and finally stabilises above a soil moisture content (soil moisture content threshold value in Eqn 5) of 0.2 m3/m3 (Figs 10a and 11a). Figs 10a and 11a, obtained from the post-fire rainfall event of 27 February 2010, show consistent results with the post-fire rainfall event of 18 January 2010 (Figs 10b and 11b). Figs 7, 8, 9a, 10a and 11a (rainfall event of 27 February 2010) and Figs 9b, 10b, 11b and 12 (rainfall event of 18 January 2010) demonstrate that the change in the hydrological output from 0.2 m3/m3 soil moisture threshold to 0.25 m3/m3 is significantly low. Therefore, a soil moisture content of 0.25 m3/m3 is considered the threshold above which the effect of a wildfire on soil infiltration becomes negligible. The justification for initially using and recommending the soil moisture threshold value of 0.25 m3/m3 is as follows:
Bayad et al. (2020) observed that an increase in soil moisture led to a decrease in the WDPT. For the majority of their tested soil types, WDPT decreased to a very low value above a soil moisture content of 0.2 m3/m3.
Similar soil moisture threshold values for severely burned locations have been reported by Huffman et al. (2001) and MacDonald and Huffman (2004).
The results of the present study (Figs 7–12) show that the change in hydrological output from a soil moisture threshold of 0.2–0.25 m3/m3 is significantly small. Therefore, this study at a watershed scale agrees with the observations made by Bayad et al. (2020).
By employing Eqn 5 with a soil moisture threshold constraint of 0.25 m3/m3 and a pre-fire calibrated saturated soil hydraulic conductivity of 0.18 cm h−1 (Table 1), RFk was used as a calibration factor in setting up the post-fire hydrological model. At 97% Nash–Sutcliffe efficiency, the calibrated RFk was found to be 0.1. An RFk value of 0.1 indicates a maximum soil hydraulic conductivity reduction of 90% from pre-fire soil hydraulic conductivity, which agrees with the findings of Blake et al. (2010).
This study incorporated the soil moisture threshold in the post-fire hydrological model and conducted a detailed analysis of the threshold value based on the model results. This analysis included references to onsite observations made at point scales (Bayad et al. 2020) to justify and verify the application of the threshold value at a watershed scale.
In the same watershed study region, a recent post-fire watershed hydrological model developed by Wang et al. (2020) recommends a reduced saturation hydraulic conductivity value in post-fire hydrological conditions. Likewise, separate post-fire watershed hydrological modelling conducted by Liu et al. (2021) in the Upper Arroyo Seco also recommends a reduced saturated hydraulic conductivity parameter value in a post-fire condition. Unlike these studies (Wang et al. 2020; Liu et al. 2021), the formulation in the present study enhances the rate of decrease of soil hydraulic conductivity (rather than the saturated soil hydraulic conductivity) in the soil characteristics curve under burned conditions and below the soil moisture threshold value. The rate of change in soil hydraulic conductivity in the post-fire condition is dependent on RFk and BDF. Like saturated hydraulic conductivity, RFk is a physical soil characteristic based on soil type (Blake et al. 2010). BDF is the parameter that adjusts RFk according to the burn severity map. Over an extended period, a watershed impacted by wildfire undergoes a process of recovery that also changes the burn severity at a location and the burn severity map of the watershed. Therefore, if a long-term simulation is set up for a post-wildfire hydrological model, it is crucial to consider the dynamic nature of the BDF. This demonstrates that the post-fire formulation presented in Eqn 5 has a physical basis that is spatially and temporally distributed as per the grid-based distributed linkage of RFk, BDF and θt to the soil type, burn severity conditions (Fig. 2) and soil moisture conditions (Fig. 5) respectively. The section ‘Wildfire-induced soil hydraulic factors, WISH factors’ also describes the explicit link between the wildfire-induced soil hydraulic factors and the spatial and temporal aspects of post-fire physical properties, soil type and burn severity. The soil moisture condition changes via wetting and drying hydrodynamics in the vadose zone. Likewise, the burn severity condition changes as burn recovery progresses with time. The explicit linkage of gridded RFk, BDF and θt with this spatially distributed dynamic soil moisture and burn severity conditions makes the intensity of the hydrophobicity, which is based on the reduction of the hydraulic conductivity in Eqn 5, dynamic as well. In this study, there is a two-way coupling of the soil hydraulic conductivity reduction state simulation capability of the post-fire hydrological process, Eqn 5, and the soil moisture physical state accounting of the infiltration/runoff generation mathematical formulation in the GSSHA hydrology model. A continuous exchange of this soil hydraulic conductivity reduction state and the soil moisture state information is set at the infiltration time-step in the post-fire hydrological model runs.
Process dominance in the post-fire runoff simulation
In this study watershed, the post-fire process defined by Eqn 5 played a dominant role in simulating the post-fire hydrograph. For the post-fire rainfall runoff event of 18 January 2010, the pre-fire calibrated model simulated only 2% of the observed peak flow. By incorporating the post-fire parameter change in the routing process, as shown in the far-right column of Table 2 (without the infiltration process shown in Table 2), the model simulated only 5% of the observed peak flow. With the addition of post-fire parameter change for the infiltration process as well, as shown in Table 2, the model simulated 90% of the observed peak flow with a Nash–Sutcliffe efficiency of 82%. The recent post-fire modelling studies conducted by Liu et al.(2021) and Wang et al.(2020) do not mention or show such comparative process dominance in post-fire hydrological modelling of runoff.
Vegetation interception
Although no pre-fire and post-fire results and analysis conducted above incorporated vegetation interception of rainfall, the interception process is significant for both pre-fire and post-fire watershed hydrological modelling (Gary 1970; Wang et al. 2020; Weltz et al. 2021). Therefore, the significance of incorporating the vegetation interception process in the post-fire condition was compared with the pre-fire condition. A simple two-parameter vegetation interception process (Gary 1970) was applied in the pre-fire hydrological model of the 4 January 2008 rainfall event and the post-fire hydrological model of 18 January 2010 rainfall event.
The interception rate at time t, i(t), is expressed as:
where p denotes rainfall intensity at time t, S is the storage capacity (mm), C is the interception coefficient (unitless, between 0.0 and 1.0), and I is the cumulative interception depth.
S for the pre-fire hydrological model of the 4 January 2008 rainfall event was 0.18 mm for forest and 0.1 mm for shrub and grassland, and I was 11% for forest and 6% for shrub and grassland. These parameter values of S and I were applied as per Gary (1970), Weltz et al. (2021) and Zhong et al. (2022). For the post-fire hydrological model of the 18 January 2010 rainfall event, 60% lower values of I and S (as mentioned by Wang et al. 2020) for all burned locations were applied. The simulation results showed that applying the vegetation interception process in the pre-fire hydrological model reduced the peak flow by 40% compared with that without it, whereas for the post-fire hydrological model, the simulated peak flow decreased by 16% with the application of the vegetation interception process. This shows that vegetation interception on peak flow reduction is 61% less in the post-fire event than in the pre-fire event.
Conclusion
This study introduces a soil moisture threshold into the formulation of wildfire-induced soil hydraulic factors to limit the impacts of post-fire water repellency on runoff generation. The formulation, including the soil moisture threshold, was deployed in the Arroyo Seco GSSHA hydrological model, with distributed initial post-fire soil moisture content values estimated using the SERVES method. The model specifically focuses on post-fire conditions following the Station Fire of August 2009, during which 95% of the watershed was burned, with 80% of the area experiencing medium to severe burn severity. To identify the soil moisture threshold value at the watershed scale, this study examined the impact of limiting the post-wildfire effect on the infiltration process for several soil moisture states, increasing the value from near wilting point to field capacity. The major observations and findings of this study are as follows:
Applying a soil moisture threshold value of 0.11 m3/m3, near the wilting point, resulted in a substantial underestimation of simulated runoff, with a peak flow error of 95% and a corresponding Nash–Sutcliffe efficiency of 0.007%. Therefore, the absence of soil water repellency and a decreased soil hydraulic conductivity in the numerical infiltration process, especially at a lower soil moisture state following a wildfire, results in a significant underestimation of the simulated runoff.
When the soil moisture threshold value was increased from 0.11 to 0.23 m3/m3, the peak flow error dramatically decreased from over 95 to below 14%. The corresponding Nash–Sutcliffe efficiency increased from below 0.007% to above 81%. These results illustrate that although limiting the wildfire hydrological process with a lower soil moisture threshold value has a significantly large impact on runoff, this impact decreases as the soil moisture threshold value increases.
The Nash–Sutcliffe efficiency, infiltration depth, runoff volume and peak flow error displayed significantly less sensitivity to soil moisture threshold values above 0.2 m3/m3. Results from the post-fire hydrological model of the Arroyo Seco watershed showed a complete transition from hydrophobic to hydrophilic conditions at a soil moisture threshold value of 0.25 m3/m3. This shows that in a burned watershed, there exists an effective soil moisture threshold value above which soil water repellency and decreased soil hydraulic conductivity cease.
The use of physically based distributed hydrological models that can simulate the effect of wildfire on runoff production based on soil moisture content is indicated for modelling post-wildfire hydrology. Bridging observations, theory and numerical simulation is also indicated for a physics-based post-fire hydrological model at the watershed scale. The results obtained from the Arroyo Seco watershed model are based on rainfall events from the wet months immediately following the Station wildfire in southern California, which burned 95% of the watershed. The application and analysis of this method across watersheds in diverse climatic regions, with varying land cover, soil types and burn conditions, and at various stages of burn recovery are a subject for future research and development.
Data availability
The data supporting this study will be shared upon reasonable request to the corresponding author.
Declaration of funding
This research was supported by the US Army Corps of Engineers Post-Wildfire Flood Risk Management Research and Development Program.
Acknowledgements
Constructive comments and valuable advice from Editor Susan G. Conard, an anonymous Associate Editor and three anonymous reviewers are greatly appreciated.
Author contributions
Methodology and software development: N. R. P.; conceptualisation, analysis, writing, reviewing, and editing: N. R. P. and I. F. Both authors have read and agreed to the submitted version of the manuscript.
References
Abatzoglou JT, Williams AP (2016) Impact of anthropogenic climate change on wildfire across western US forests. Proceedings of the National Academy of Sciences 113(42), 11770-11775.
| Crossref | Google Scholar | PubMed |
Adams MA (2013) Mega-fires, tipping points and ecosystem services: managing forests and woodlands in an uncertain future. Forest Ecology and Management 294, 250-261.
| Crossref | Google Scholar |
Agbeshie AA, Abugre S, Atta-Darkwa T, Awuah R (2022) A review of the effects of forest fire on soil properties. Journal of Forestry Research 33, 1419-1441.
| Crossref | Google Scholar |
Bayad M, Chau H W, Trolove S, Moir J, Condron L, Bouray M (2020) The relationship between soil moisture and soil water repellency persistence in hydrophobic soils. Water 12, 2322.
| Crossref | Google Scholar |
Benda L, Miller D, Bigelow P, Andras K (2003) Effects of post-wildfire erosion on channel environments, Boise River, Idaho. Forest Ecology and Management 178, 105-119.
| Crossref | Google Scholar |
Bixby RJ, Cooper SD, Gresswell RE, Brown LE, Dahm CN, Dwire KA (2015) Fire effects on aquatic ecosystems: an assessment of the current state of the science. Freshwater Science 34, 1340-1350.
| Crossref | Google Scholar |
Blake WH, Theocharopoulos SP, Skoulikidis N, Clark P, Tountas P, Hartley R, Amaxidis Y (2010) Wildfire impacts on hillslope sediment and phosphorus yields. Journal of Soils and Sediments 10, 671-682.
| Crossref | Google Scholar |
Bondur VG, Mokhov II, Voronova OS, Sitnov SA (2020) Satellite monitoring of Siberian wildfires and their effects: features of 2019 anomalies and trends of 20-year changes. Doklady Earth Sciences 492, 370-375.
| Crossref | Google Scholar |
Bowman DMJS, Williamson GJ, Abatzoglou JT, Kolden CA, Cochrane MA, Smith AMS (2017) Human exposure and sensitivity to globally extreme wildfire events. Nature Ecology & Evolution 1, 58.
| Crossref | Google Scholar | PubMed |
Brando PM, Soares-Filho B, Rodrigues L, Assunção A, Morton D, Tuchschneider D, Fernandes E, Macedo MN, Oliveira U, Coe MT (2020) The gathering firestorm in southern Amazonia. Science Advances 6, eaay1632.
| Crossref | Google Scholar | PubMed |
Calkin DE, Thompson MP, Finney MA (2015) Negative consequences of positive feedbacks in US wildfire management. Forest Ecosystems 2, 9.
| Crossref | Google Scholar |
Cerdà A (1998) The influence of aspect and vegetation on seasonal changes in erosion under rainfall simulation on a clay soil in Spain. Canadian Journal of Soil Science 78, 321-330.
| Crossref | Google Scholar |
Certini G (2005) Effects of fire on properties of forest soils: a review. Oecologia 143, 1-10.
| Crossref | Google Scholar | PubMed |
Chen L, Berli M, Chief K (2013) Examining modeling approaches for the rainfall-runoff process in wildfire-affected watersheds: using San Dimas experimental forest. Journal of the American Water Resources Association 49(4), 851-866.
| Crossref | Google Scholar |
Conard SG, Sukhinin AI, Stocks BJ, Cahoon DR, Davidenko EP, Ivanova GA (2002) Determining effects of area burned and fire severity on carbon cycling and emissions in Siberia. Climatic Change 55, 197-211.
| Crossref | Google Scholar |
Dahm CN, Candelaria‐Ley RI, Reale CS, Reale JK, Van Horn D J (2015) Extreme water quality degradation following a catastrophic forest fire. Freshwater Biology 60, 2584-2599.
| Crossref | Google Scholar |
DeBano LF (2000) The role of fire and soil heating on water repellency in wildland environments: a review. Journal of Hydrology 231–232, 195-206.
| Crossref | Google Scholar |
De Jonge LW, Jacobsen OH, Moldrup P (1999) Soil water repellency: effects of water content, temperature, and particle size. Soil Science Society of America Journal 63, 437-442.
| Crossref | Google Scholar |
Dekker LW, Ritsema CJ (1995) Fingerlike wetting patterns in two water-repellent loam soils. Journal of Environmental Quality 24, 324-333.
| Crossref | Google Scholar |
Dekker LW, Ritsema CJ (1996) Variation in water content and wetting patterns in Dutch water repellent peaty clay and clayey peat soils. Catena 28, 89-105.
| Crossref | Google Scholar |
Doerr SH, Thomas AD (2000) The role of soil moisture in controlling water repellency: new evidence from forest soils in Portugal. Journal of Hydrology 231–232, 134-147.
| Crossref | Google Scholar |
Doerr SH, Shakesby RA, Walsh RPD (2000) Soil water repellency: its causes, characteristics and hydro-geomorphological significance. Earth-Science Reviews 51, 33-65.
| Crossref | Google Scholar |
Dorigo W, Himmelbauer I, Aberer D, Schremmer L, Petrakovic I, Zappa L, Preimesberger W, Xaver A, Annor F, Ardö J, Baldocchi D, Bitelli M, Blöschl G, Bogena H, Brocca L, Calvet JC, Camarero JJ, Capello G, Choi M, Cosh MC, van de Giesen N, Hajdu I, Ikonen J, Jensen KH, Kanniah KD, de Kat I, Kirchengast G, Kumar Rai P, Kyrouac J, Larson K, Liu S, Loew A, Moghaddam M, Martínez Fernández J, Mattar Bader C, Morbidelli R, Musial JP, Osenga E, Palecki MA, Pellarin T, Petropoulos GP, Pfeil I, Powers J, Robock A, Rüdiger C, Rummel U, Strobel M, Su Z, Sullivan R, Tagesson T, Varlagin A, Vreugdenhil M, Walker J, Wen J, Wenger F, Wigneron JP, Woods M, Yang K, Zeng Y, Zhang X, Zreda M, Dietrich S, Gruber A, van Oevelen P, Wagner W, Scipal K, Drusch M, Sabia R (2021) The International Soil Moisture Network: serving Earth system science for over a decade. Hydrology and Earth System Sciences 25, 5749-5804.
| Crossref | Google Scholar |
Downer CW, Ogden FL (2004) Appropriate vertical discretization of Richard’s equation for two-dimensional watershed-scale modelling. Hydrological Processes 18, 1-22.
| Crossref | Google Scholar |
Engman ET (1986) Roughness coefficients for routing surface runoff. Journal of Irrigation and Drainage Engineering 112, 39-53.
| Crossref | Google Scholar |
Fisher GB, Amos CB, Bookhagen B, Burbank DW, Godard V (2012) Channel widths, landslides, faults, and beyond: The new world order of high-spatial resolution Google Earth imagery in the study of earth surface processes. In ‘Google Earth and Virtual Visualizations in Geoscience Education and Research’. (Eds SJ Whitmeyer, JE Bailey, DG De Paor, T Ornduff) pp. 1–22. (Geological Society of America, USA) 10.1130/2012.2492(01)
Foster DR, Knight DH, Franklin JF (1998) Landscape patterns and legacies resulting from large, infrequent forest disturbances. Ecosystems 1, 497-510.
| Crossref | Google Scholar |
Halofsky JE, Peterson DL, Harvey BJ (2020) Changing wildfire, changing forests: the effects of climate change on fire regimes and vegetation in the Pacific Northwest, USA. Fire Ecology 16, 4.
| Crossref | Google Scholar |
Harpold A, Brooks P, Rajagopal S, Heidbuchel I, Jardine A, Stielstra C (2012) Changes in snowpack accumulation and ablation in the Intermountain West. Water Resources Research 48, W11501.
| Crossref | Google Scholar |
He J, Chen D, Jenkins L, Loboda TV (2021) Impacts of wildfire and landscape factors on organic soil properties in Arctic tussock tundra. Environmental Research Letters 16, 085004.
| Crossref | Google Scholar |
Green WH, Ampt GA (1911) Studies on soil phyics. The Journal of Agricultural Science 4, 1-24.
| Crossref | Google Scholar |
Hoch OJ, McGuire LA, Youberg AM, Rengers FK (2021) Hydrogeomorphic recovery and temporal changes in rainfall thresholds for debris flows following wildfire. Journal of Geophysical Research: Earth Surface 126(12), e2021JF006374.
| Crossref | Google Scholar |
Huffman EL, MacDonald LH, Stednick JD (2001) Strength and persistence of fire-induced soil hydrophobicity under ponderosa and lodgepole pine, Colorado Front Range. Hydrological Processes 15, 2877-2892.
| Crossref | Google Scholar |
Imeson AC, Verstraten JM, van Mulligen EJ, Sevink J (1992) The effects of fire and water repellency on infiltration and runoff under Mediterranean type forest. Catena 19, 345-361.
| Crossref | Google Scholar |
Jolly WM, Cochrane MA, Freeborn PH, Holden ZA, Brown TJ, Williamson GJ, Bowman DM (2015) Climate-induced variations in global wildfire danger from 1979 to 2013. Nature Communications 6, 7537.
| Crossref | Google Scholar | PubMed |
Kinoshita AM, Hogue TS (2011) Spatial and temporal controls on post-fire hydrologic recovery in Southern California watersheds. Catena 87, 240-252.
| Crossref | Google Scholar |
Liu T, McGuire LA, Wei H, Rengers FK, Gupta H, Ji L, Goodrich D C (2021) The timing and magnitude of changes to Hortonian overland flow at the watershed scale during the post‐fire recovery process. Hydrological Processes 35(5), e14208.
| Crossref | Google Scholar |
Lucas-Borja ME, Bombino G, Carrà BG, D’Agostino D, Denisi P, Labate A, Plaza-Alvarez PA, Zema DA (2020) Modeling the soil response to rainstorms after wildfire and prescribed fire in Mediterranean forests. Climate 8, 150.
| Crossref | Google Scholar |
MacDonald LH, Huffman EL (2004) Post-fire soil water repellency: persistence and soil moisture thresholds. Soil Science Society of America Journal 68, 1729-1734.
| Crossref | Google Scholar |
McGuire LA, Rengers FK, Kean JW, Staley DM, Mirus BB (2018) Incorporating spatially heterogeneous infiltration capacity into hydrologic models with applications for simulating post‐wildfire debris flow initiation. Hydrological Processes 32, 1173-1187.
| Crossref | Google Scholar |
Michaelis AC, Gershunov A, Weyant A, Fish MA, Shulgina T, Ralph FM (2022) Atmospheric river precipitation enhanced by climate change: a case study of the storm that contributed to California’s Oroville Dam crisis. Earth’s Future 10, e2021EF002537.
| Crossref | Google Scholar |
Miller ME, Billmire M, Elliot WJ, Endsley KA, Robichaud PR (2015) Rapid response tools and datasets for post-fire modeling: linking Earth observations and process-based hydrological models to support post-fire remediation. International Archives of the Photogrammetry, Remote Sensing & Spatial Information Sciences XL-7/W3, 469-476.
| Crossref | Google Scholar |
Moody JA, Kinner DA, Úbeda X (2009) Linking hydraulic properties of fire-affected soils to infiltration and water repellency. Journal of Hydrology 379, 291-303.
| Crossref | Google Scholar |
Nolan RH, Boer MM, Collins L, Resco de Dios V, Clarke H, Jenkins M, Kenny B, Bradstock RA (2020) Causes and consequences of eastern Australia’s 2019–20 season of mega-fires. Global Change Biology 26, 1039-1041.
| Crossref | Google Scholar | PubMed |
North MP, Stephens SL, Collins BM, Agee JK, Aplet G, Franklin JF, Fulé PZ (2015) Reform forest fire management. Science 349, 1280-1281.
| Crossref | Google Scholar | PubMed |
Pradhan NR (2019) Estimating growing-season root zone soil moisture from vegetation index-based evapotranspiration fraction and soil properties in the Northwest Mountain region, USA. Hydrological Sciences Journal 64, 771-788.
| Crossref | Google Scholar |
Pradhan NR (2023) ‘Soil-Moisture Estimation of Root-Zone through Vegetation-Index-Based Evapotranspiration-Fraction and Soil-Properties (SERVES) User’s Manual Version 1. ERDC/CHL CHETN-XII-3.’ (US Army Engineer Research and Development Center: Vicksburg, MS) 10.21079/11681/47399
Pradhan NR, Floyd I (2021) Event based post-fire hydrological modeling of the upper Arroyo Seco watershed in southern California. Water 13(16), 2303.
| Crossref | Google Scholar |
Pradhan NR, Ogden FL (2010) Development of a one-parameter variable source area runoff model for ungauged basins. Advances in Water Resources 33, 572-584.
| Crossref | Google Scholar |
Pradhan NR, Floyd I, Brown S (2020) Satellite imagery-based SERVES soil moisture for the analysis of soil moisture initialization input scale effects on physics-based distributed watershed hydrologic modelling. Remote Sensing 12, 2108.
| Crossref | Google Scholar |
Rawls WJ, Brakensiek DL, Miller N (1983) Green-Ampt infiltration parameters from soils data. Journal of Hydraulic Engineering 109, 62-70.
| Crossref | Google Scholar |
Rengers FK, McGuire LA, Kean JW, Staley DM, Hobley DEJ (2016) Model simulations of flood and debris flow timing in steep catchments after wildfire. Water Resources Research 52(8), 6041-6061.
| Crossref | Google Scholar |
Rengers FK, McGuire LA, Kean JW, Staley DM, Youberg AM (2019) Progress in simplifying hydrologic model parameterization for broad applications to post‐wildfire flooding and debris‐flow hazards. Earth Surface Processes and Landforms 44(15), 3078-3092.
| Crossref | Google Scholar |
Rengers FK, McGuire LA, Oakley NS, Kean JW, Staley DM, Tang H (2020) Landslides after wildfire: initiation, magnitude, and mobility. Landslides 17(11), 2631-2641.
| Crossref | Google Scholar |
Robichaud PR (2000) Fire effects on infiltration rates after prescribed fire in Northern Rocky Mountain forests, USA. Journal of Hydrology 231–232, 220-229.
| Crossref | Google Scholar |
Robinne FN, Bladon KD, Miller C, Parisien MA, Mathieu J, Flannigan MD (2018) A spatial evaluation of global wildfire–water risks to human and natural systems. Science of the Total Environment 610–611, 1193-1206.
| Crossref | Google Scholar | PubMed |
Santi PM, Rengers FK (2020) Wildfire and Landscape Change. In ‘Reference module in earth systems and environmental sciences’. (Ed. JF Shroder) pp. 765–797. (Academic Press) 10.1016/B978-0-12-818234-5.00017-1
Schmidt KM, Hanshaw MN, Howle JF, Kean JW, Staley DM, Stock JD, Bawden GW (2011) Hydrologic conditions and terrestrial laser scanning of post-fire debris flows in the San Gabriel Mountains, CA, U.S.A. Italian Journal of Engineering Geology and Environment 583-593.
| Crossref | Google Scholar |
Shakesby RA, Doerr SH (2006) Wildfire as a hydrological and geomorphological agent. Earth-Science Reviews 74, 269-307.
| Crossref | Google Scholar |
Thomas MA, Rengers FK, Kean JW, McGuire LA, Staley DM, Barnhart KR, Ebel BA (2021) Post-wildfire soil-hydraulic recovery and the persistence of debris flow hazards. Journal of Geophysical Research: Earth Surface 126(6), e2021JF006091.
| Crossref | Google Scholar |
Wałęga A (2016) The importance of calibration parameters on the accuracy of the floods description in the Snyder’s model. Journal of Water and Land Development 28, 19-25.
| Crossref | Google Scholar |
Wang J, Stern MA, King VM, Alpers CN, Quinn NWT, Flint AL, Flint LE (2020) PFHydro: a new watershed-scale model for post-fire runoff simulation. Environmental Modelling & Software 123, 104555.
| Crossref | Google Scholar |
Weltz MA, Hernandez M, Nearing MA, Spaeth KE, Pierson FB, Williams CJ, AlHamdan OZ, Nouwakpo SK, Armendariz G, Haiyan W, Goodrich DC, Guertin P, Unkrich C, Polyakov V, McGwire K, Nesbit J, Frazier G, Jolley L, Stone J (2021) ‘Rangeland Hydrology and Soil Erosion Processes: A guide for Conservation Planning with the Rangeland Hydrology and Erosion Model (RHEM)’. Handbook No. 647. 80 p. (USDA Natural Resources Conservation Service)
Westerling AL, Bryant BP, Preisler HK, Holmes TP, Hidalgo HG, Das T, Shrestha SR (2011) Climate change and growth scenarios for California wildfire. Climatic Change 109, 445-463.
| Crossref | Google Scholar |
Western AW, Grayson RB (1998) The Tarrawarra data set: soil moisture patterns, soil characteristics, and hydrological flux measurements. Water Resources Research 34, 2765-2768.
| Crossref | Google Scholar |
Williams AP, Abatzoglou JT, Gershunov A, Guzman‐Morales J, Bishop DA, Balch JK, Lettenmaier DP (2019) Observed impacts of anthropogenic climate change on wildfire in California. Earth’s Future 7, 892-910.
| Crossref | Google Scholar |
Zema DA (2021) Post-fire management impacts on soil hydrology. Current Opinion in Environmental Science & Health 21, 100252.
| Crossref | Google Scholar |
Zhong F, Jiang S, van Dijk AIJM, Ren L, Schellekens J, Miralles DG (2022) Revisiting large-scale interception patterns constrained by a synthesis of global experimental data. Hydrology and Earth System Sciences 26, 5647-5667.
| Crossref | Google Scholar |