Augmenting rangeland condition monitoring with drones: potential for carbon farming projects to support widespread ecosystem assessment
Samuel Shumack A * , Gregor Sanders A , Michael Rooney A and Andrew O’Reilly-Nugent AA
Abstract
Large areas of the Australian rangelands are used for pastoral agriculture. Rangeland condition monitoring commonly operates at one of the following two scales: (1) detailed but spatially restricted on-ground surveys of farm and ecosystem condition, or (2) regional and national analyses using data from earth observation satellites such as Landsat or Sentinel-2. This study aimed to assess the potential of drone-based data collection from carbon farming projects to augment rangeland condition monitoring. The widespread adoption of carbon farming in Australian rangelands and the increasing use of drones to validate vegetation maps could provide an opportunity to bridge these two scales. Range condition was defined in terms of plant species composition, woody vegetation structure, and ground cover, focusing on indicators relevant to both carbon sequestration and broader ecosystem function. Existing maps of vegetation structure were combined with on-ground surveys and drone data collected at the scale of an individual rangeland property. Drone-mounted sensors produced ultra-high-resolution imagery (~2 cm GSD) and three-dimensional point clouds. This combination of imagery and three-dimensional data enabled measurement of condition indicators such as tree size distributions, canopy area and ground layer coverage. This case study of drone-based ecological profiling after land management change demonstrated that monitoring programmes of carbon farming projects can be leveraged for detailed and accurate investigation of ecosystem condition. Significant differences were found in canopy and ground layer elements between vegetation communities and land-use histories. These findings have highlighted the value of repurposing carbon farming project data for holistic landscape condition assessment. The value and importance of in situ vegetation structural measurements is further emphasised by the contrasting unsuitability of national-scale satellite remote-sensing products for precise property-level mapping. The potential for the growing in situ dataset to support both property-scale decision-making and understanding of the broader ecological effects of woody vegetation regrowth is discussed.
Keywords: Australian rangelands, drones, ecological profiling, land management change, mesoscale monitoring, UAVs, vegetation condition, canopy cover.
Introduction
Australia’s rangelands cover vast areas, comprising a complex mosaic of native vegetation profoundly altered by pastoral management since European settlement. Widespread management practices including clearing, fodder harvesting, and overgrazing by domestic, native and feral animals (Jones and Burrows 1994; Peeters and Butler 2014) have contributed to a decline in tree numbers and ecological condition across many landscapes (Witt et al. 2009; Fensham et al. 2011; Cohn et al. 2013). The land-use history and condition of the Australian rangelands has created opportunity for carbon farming. Carbon farming involves activities that reduce greenhouse gas emissions and/or sequesters carbon in vegetation and soils. In the rangelands, popular carbon farming activities aim to increase woody vegetation cover through managed grazing and reduced disturbance. By 2023, project areas registered under the human induced regeneration (HIR) method covered ~7% of the rangelands (Supplementary Fig. S1; Clean Energy Regulator 2024). These projects typically involve reducing and/or maintaining suitable stock numbers, removing feral goats, fencing repair and removal of watering points, implementing fire management plans and ceasing the clearing or chemical suppression of regenerating vegetation in eligible areas (Baumber et al. 2020). These activities are intended to target drivers that suppress the survival and growth of juvenile native trees (e.g. Harrington 1979; Brown 1985; Witt et al. 2011; Cohn et al. 2013; Travers et al. 2019). While increasing tree-based carbon storage is the primary goal, these activities may yield broader ecological benefits.
Reduced grazing intensity in arid and semi-arid rangeland environments has been linked to increased native grass recruitment (Anderson et al. 1996), higher herbaceous species counts (Friedel 1997; Landsberg et al. 2003; Witt et al. 2011), greater shrub density (Travers et al. 2019), and improved soil properties such as higher water infiltration rates and carbon:nitrogen ratios (Witt et al. 2011). Drivers such as grazing can have indirect effects by modifying landscape processes with complex feedbacks. For example, Ludwig et al. (2024) showed that sites further from water points (with lower grazing intensity) had larger tree groves with healthy upslope grass bands and fewer signs of erosion than did sites closer to water points, indicating improved capacity to capture and retain scarce water and nutrients in these semi-arid landscapes. Moreover, increasing native tree density provides structure and habitat for native fauna, potentially enhancing biodiversity (Peeters and Butler 2014). However, quantifying these co-benefits at landscape scales remains challenging because of the complexity of long-term ecological monitoring in heterogeneous rangeland systems. Current monitoring approaches still rely primarily on coarse-resolution satellite products (e.g. (Department of Environment and Science, 2021) or ground surveys (e.g. Novelly et al. 2008; Sparrow et al. 2020), which are constrained by resolution and scale respectively (Retallack et al. 2023). Although the HIR method was closed to new projects in September 2023, existing projects will continue to operate and collect data throughout their 25-year crediting periods. The thesis of this paper is that the regulatory requirements of carbon farming projects under the HIR method may offer a unique opportunity to address rangelands monitoring challenges.
The environmental data requirements of carbon farming
HIR projects must routinely collect a range of data for carbon accounting purposes. These include satellite-based remote sensing, aerial surveys and ground-based vegetation assessments. The HIR Mapping Guidelines require vegetation maps to identify areas of existing ‘forest’ (referring to regions of at least 0.2 ha with minimum 20% canopy coverage provided by trees reaching a minimum 2 m height) and regions with or without forest regeneration potential with >85% accuracy (Clean Energy Regulator 2019). Traditional approaches to map accuracy assessments (MAA) include site visits, photo points and forest inventory, or visual interpretation of remote-sensing imagery (Penman et al. 2016). However, the size of rangeland pastoral enterprises (ranging from ~10 to >100 kha) has meant that aerial surveys are often used to obtain sufficiently large samples to represent the broader landscape. Given the extent of HIR carbon farming projects (Supplementary Fig. S1), and these MAA requirements, there exists a large and growing dataset of airborne surveys that has potential to describe key ecosystem variables, beyond just forest regeneration potential. Repurposing this for ecological research can potentially overcome the logistical and financial barriers that have historically limited large-scale, long-term monitoring in rangelands.
Drones as tools for rangeland ecological profiling
Consumer-grade remotely piloted aircraft systems (RPAS; hereafter ‘drones’) provide a cost-effective means for conducting carbon project MAAs. Drones mounted with cameras and laser scanners (also known as light detection and ranging units; hereafter ‘lidar’) can collect centimetre-resolution imagery and three-dimensional data over 1–100 ha plots. These sensors detect fine-scale features of rangeland ecosystems that satellite-based remote sensing cannot, at scales much greater than that feasible with ground-based surveys. Drone-derived data have been used to map rangeland vegetation cover (Rango et al. 2006, 2009; Sankey et al. 2019; Amputu et al. 2023), estimate biomass (e.g. Cunliffe et al. 2016, 2022; Levick et al. 2021; Taugourdeau et al. 2022) and even identify dominant plant species (Sankey et al. 2021). Melville et al. (2019) demonstrated that multispectral drone imagery was able to distinguish between photosynthetic and non-photosynthetic vegetation, better than was equivalent Landsat satellite-based fractional cover estimates, whereas Rudge et al. (2021) showed that structural information from drone-based lidar was able to model individual tree size distributions. Despite the proven potential of drone-based methods to monitor key ecosystem variables, their integration into large-scale rangeland monitoring programmes remains limited.
Drone-assisted ecological assessment of carbon projects
Although drone-based applications in rangeland monitoring programmes show promise, none have yet achieved operational status (Retallack et al. 2023). However, carbon farming projects routinely employ drones for validating vegetation maps. The structural information collected by drones is well suited to mapping areas of forest and forest potential, but further analysis of ecosystem condition is not regularly undertaken. This study assessed the potential of drone-based data from carbon farming projects to enhance rangeland condition monitoring. The work consisted of a case study of drone-assisted ecological profiling in a recently registered HIR project in Queensland, Australia. Range condition was defined through plant species composition and woody vegetation structure, focusing on indicators relevant to both carbon sequestration and ecosystem function.
The aims were to:
Compare ecosystems and management histories using condition metrics derived from carbon farming project data, including drone and ground surveys.
Evaluate the integration of drone surveys with ground-based assessments for comprehensive vegetation condition monitoring in rangelands.
Highlight the importance of in situ data for vegetation condition monitoring by contrasting drone lidar-derived canopy coverage with outputs from a national-scale satellite-based model of woody vegetation cover.
Assess the long-term potential of this approach for understanding ecological response to land management changes, given the extent and duration of carbon farming projects in in the Australian rangelands.
The overarching objective was to demonstrate a novel pathway to leverage carbon farming data collection for broader ecological insights, potentially transforming the capacity for large-scale, long-term rangeland monitoring.
Methods
Background
This study drew on an existing drone-based carbon project MAA programme and the Accounting For Nature Framework – Integrated Vegetation Condition (IVC; Bush Heritage Australia & Climate Friendly 2022). The IVC protocol combines drones with on-ground botanical assessment to describe the ecosystem condition of distinct vegetation management units (Fig. 1). Although the addition of a floristic ground survey goes beyond the requirements of a MAA for carbon farming projects, it is necessary to overcome the limited ability of drone data to perform detailed species mapping (Gillan et al. 2020). Because ground surveys are complemented with drones, they can be conducted efficiently, without the need for coverage estimates (e.g. via point-intercept methods). Ecosystem metrics covered by the IVC protocol include (1) the condition and canopy structure of dominant tree species, (2) the composition of canopy and understorey species in terms of native or non-native species, and (3) the composition of the ground cover layer (such as litter and coarse woody debris).
Site description
This study took place on a 27,929 ha pastoral property in the semi-arid rangelands of Queensland, Australia. Although the exact location cannot be shared for privacy considerations, the property is approximately 90 km north of the town Bollon (28°01′51.2″S, 147°28′22.8″E; Fig. 2a). The property comprises Eucalyptus populnea (poplar box) woodlands on alluvium, sand plains, and foot slopes of hills and ranges, and Acacia anuera (mulga) woodlands on red earth plains or sand plains (Neldner et al. 2023; Fig. 2). Under the Queensland Vegetation Management Act (VMA, 1999), most of the property is designated as Category X (eligible for clearing), with the remainder being Category B (considered ‘remnant’ vegetation). Satellite-derived clearing maps from 1988 showed significant clearing, or re-clearing (Statewide Landcover and Trees Study Queensland Landsat Series 2023). The last major event occurred in 2005, with further minor clearing in 2013/14 and 2019. Wildfires affected the eastern half in 2011/12. From 2005 to 2016, the property was run as a mixed Dorper Sheep and Wagyu Cattle enterprise, transitioning to cattle in 2016. Feral goats were present throughout the property and were intermittently harvested. Mean rainfall (2002–2023) was 420 mm year−1, with half falling in the summer months (December–January). The site is intersected by a major creek, and several lower-order streams. Livestock water was sourced from a bore and surface capture. The property typified the region’s pastoral properties, where grazing and clearing had been used to balance grass cover with fodder trees (i.e. mulga) used to support livestock in dry periods. This land-use history provided an ideal context to examine vegetation structure and composition across varying disturbance and recovery gradients.
Site map. (a) Location of the 29 kha pastoral property in the semi-arid rangelands of Queensland, Australia. (b–d) Property stratification and sampling. The property is divided into four vegetation management units representing distinct classes of vegetation condition (IVC vegetation assets) based on the intersection of (b) Broad vegetation groups, and (c) Regulated vegetation management classes. (d) Shows the final stratification and sample sites. Drone surveys were conducted over 6 ha plots (n = 36). Canopy height metrics were derived from all drone plots, and high-resolution imagery from some plots was classified for cover metrics of non-tree ecosystem elements (n = 29). A botanical identification protocol was conducted by on-ground ecologists at ~0.5 ha plots (n = 14).
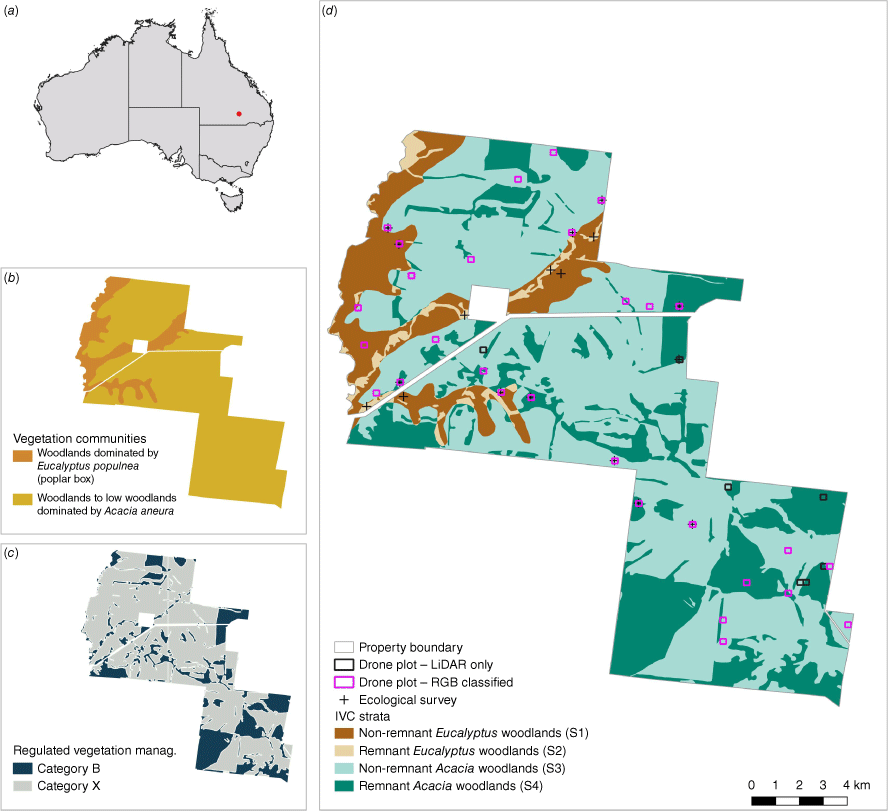
Property stratification and sampling strategy
The property was stratified into four vegetation management units by using a two-step process. Vegetation assets were defined on the basis of state-recognised broad vegetation groups (BVGs; Neldner et al. 2023): Eucalyptus populnea woodlands (BVG ID 17a) and Acacia aneura woodlands (23a; Fig. 2b). Vegetation assets were then subdivided into assessment units based on clearing history, because this significantly influences vegetation structure and composition. The basis for this subdivision was the VMA categories, namely, Category B (remnant vegetation) and Category X (non-remnant, eligible for clearing; Fig. 2c). This intersection of vegetation type and management history resulted in the following four distinct vegetation management units: S1 (non-remnant Eucalyptus woodlands), S2 (remnant Eucalyptus woodlands), S3 (non-remnant Acacia woodlands), and S4 (remnant Acacia woodlands) (Fig. 2d). This stratification captures both the ecological and management variation across the property.
Drone plots were placed consistent with the MAA requirements of the HIR Mapping Guidelines. Locations for ~6 ha drone plots (36 in total) were generated using stratified random sampling to cover non-regenerating, regenerating and forested areas. This involved overlaying a supervised, satellite remote sensing-based classification of existing tree cover on a clustering of maximum biomass potential, further refined by carbon project eligibility. Site accessibility also influenced plot placement. Drone surveys were conducted in September 2023 (Fig. 3). For ground surveys, 18 plots were manually placed within existing drone plots where possible. However, because of the focus on carbon project eligibility, only one drone plot was acquired in S2 (remnant Eucalyptus woodlands). To address this, additional ground survey plots were randomly generated in S2 to ensure that sufficient floristic data were collected in all vegetation management units. In total, six ground surveys do not coincide with drone surveys. Example photos of each stratum are shown from the ground surveys (Fig. 4).
A 15-month timeseries of rainfall at the study site, indicating the possible influence of water availability to drive differences in ground and drone-based surveys. The condition of ground cover elements is known to respond to recent rainfall. Rainfall and fractional cover data were sourced from VegMachine® (Beutel et al. 2019) (accessed from https://vegmachine.net/).
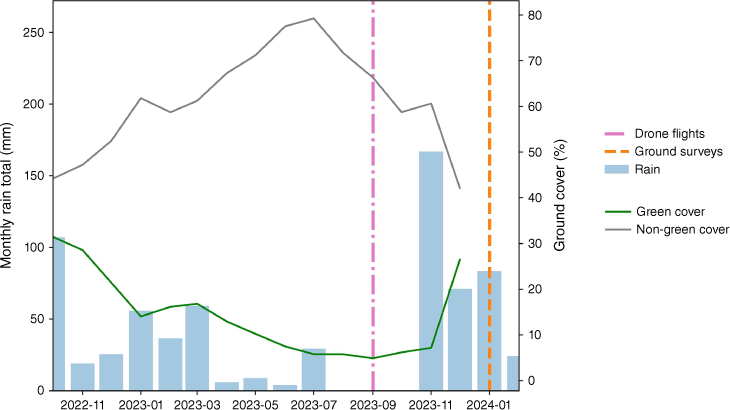
Drone data
The drone surveys used a DJI Matrice 300 equipped with a Zenmuse L1 sensor, integrating lidar and red green blue (RGB) camera modules. Flights were conducted at 80 m above ground level at 10 m s−1 (36 km h−1), with 30% side overlap for lidar and 45% side overlap with 60% forward overlap for RGB imagery. A RoboDot Global Navigation Satellite System (GNSS) base station and post-processed kinematic (PPK) correction were used to refine the recorded drone flight paths on the basis of errors observed in the static base station location. This configuration yielded point clouds with approximately 180 points per square metre (on average) and orthomosaic images with around 2 cm ground sampling distance (GSD). Point clouds and orthomosaics were processed separately by using the DJI Terra software (ver. 3.8.0; DJI 2023) package. All 36 drone plots had point clouds and most (33) had orthomosaic imagery (Fig. 5), which were used for canopy structure analysis and growth form classification respectively (Fig. 6b, c). An unspecified error in DJI Terra prevented orthomosaics from being produced for three plots. Woody vegetation was classified independently in both datasets, by using separate approaches because of sporadic spatial misalignment between tree features.
Examples of data collected from drone surveys. Left: georeferenced optical imagery processed into an orthomosaic. Middle: a colourised point cloud of lidar returns. Right: a rasterised canopy height model. (a) Images in this row show a single 6 ha plot, and (b, c) images in these rows show two distinct insets. Canopy height models are normalised so that 0 m corresponds to the ground.
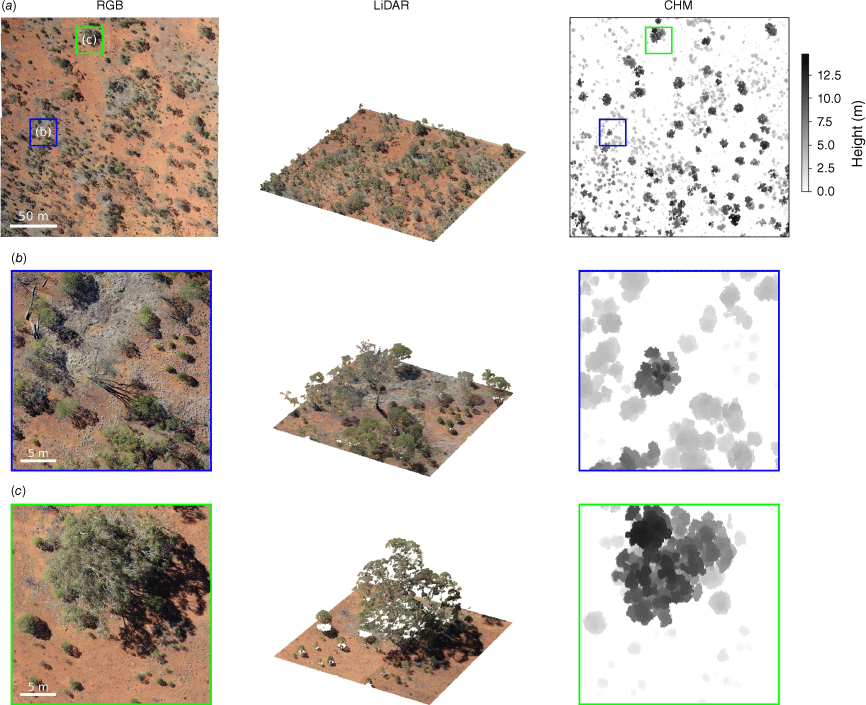
(a) An RGB orthomosaic is classified into (b) cover metrics for dry herbaceous material, woody debris, woody canopy, bare ground and shadow. (c) A canopy height model is thresholded into canopies and tree locations are identified by canopy peaks. (d) The size structure of the canopy height model and tree population shows that woody canopy occupies less than half of the overall area. The distributions of canopy pixels and tree tops, shown using probability density functions (PDF) and empirical cumulative density functions (ECDF), display two distinct cohorts. No photosynthetic ground layer was detected in this survey.
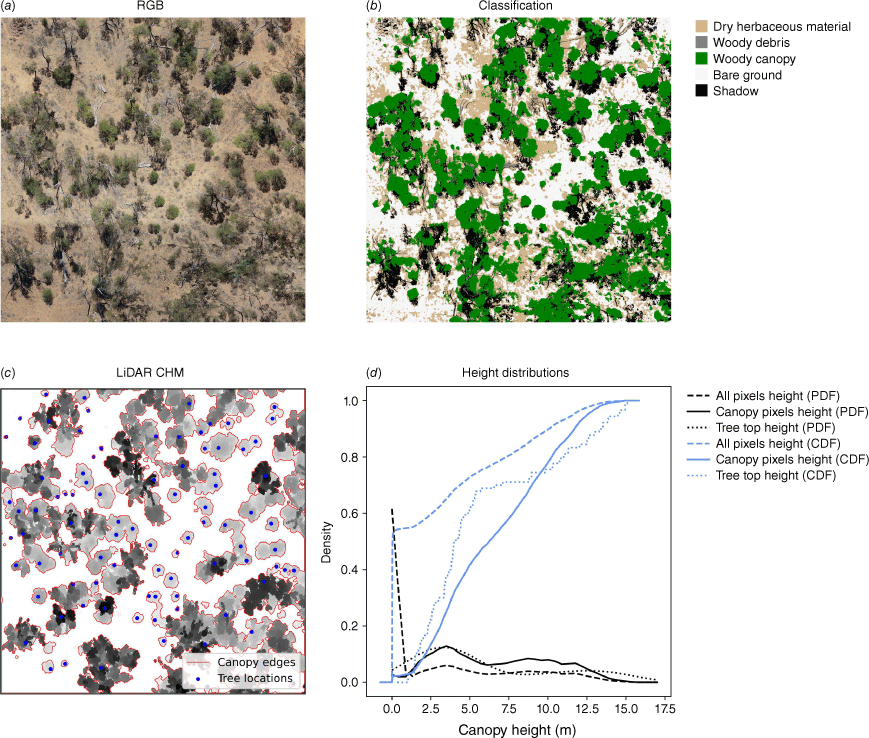
Drone imagery was classified into bare ground, ground cover, woody vegetation canopy, woody debris, or shadow (Fig. 6b) by using a two-stage process. Individually calibrated random forest classifiers (Breiman 2001) were first created for an initial set of 18 orthomosaics. Each orthomosaic required a unique classifer because of variations in vegetation type and appearance, and in overall colour distributions. The classifiers were trained by manually annotating on the basis of image interpretation and model outputs were post-processed to reduce noise in the woody canopy class by segmenting images and relabelling pixels within each segment to the majority class if either the majority was woody canopy or the initial pixel prediction was woody canopy. In the second stage, the random forest outputs were used to train a U-Net convolutional neural network (CNN; Ronneberger et al. 2015), enabling classification of all plots with a single model. The U-Net architecture is commonly employed for image classification in remote-sensing applications and can achieve high accuracy in fine-grained segmentation of vegetation (Kattenborn et al. 2019). The fastai (Howard and Gugger 2020) and TorchGeo (Stewart et al. 2022) libraries were used for U-Net training and orthomosaic classifications respectively. Ground-layer materials were dry and senescent at the time of the drone survey and were not able to be separated into photosynthetic and non-photosynthetic components. Shadow was classified where low illumination conditions meant it was not possible to reliably determine the true label. When summarising cover fractions for each plot, the shade fraction was distributed proportionally between the four remaining classes. This proportional allocation of shade was found to improve the agreement between image-based woody vegetation cover and lidar-based cover (see Supplementary Fig. S3).
Classification accuracy was estimated using a confusion matrix from 620 manually labelled points, randomly sampled across all images and classes. Overall (OA), producer’s (PA) and user’s (UA) accuracies were estimated. OA estimates the proportion of all pixels that were correctly classified. The estimate of OA was bootstrapped using 1000 samples to estimate the mean and 95% confidence intervals (Lyons et al. 2018). UA estimates the probability that a pixel with a given map label is correct. Conversely, PA estimates the probability of a pixel with a given reference class being correctly labelled by the model. OA and PA were estimated with and without points predicted as shadow. It is unclear whether false predictions of shadow should be weighted the same as other classification errors. Incorrectly classifying a pixel as shadow merely reduces the sample area because shadow pixels are redistributed. However, this reduction may be biased because shadows are more likely to occur within and round tree canopies. In contrast, where a shadow was observed and was classified as any other class, this was treated as an error. It was assumed that if a pixel’s class was indeterminable due to shading, the model was unlikely to reliably determine the true underlying class.
Point clouds were classified into ground and non-ground points by using a cloth-simulation filter (Zhang et al. 2016). Ground points produced a digital terrain model used to normalise the z-values of the point cloud to height above ground. Canopy height models with 15 cm resolution were created, from which woody vegetation canopies were classified using hysteresis thresholding (lower bound of 0.5 m and upper bounds of 1 m or 2 m to distinguish different vertical strata). Hysteresis thresholding classifies pixels above the upper bound as woody canopy and allows pixels above the lower bound to be included if they are topologically connected to other canopy pixels. Three methods were used to summarise woody canopy structure. First, a variable window filter was applied to identify individual treetops by finding the highest point within an allometrically informed search window, the size of which is determined by tree height (Popescu et al. 2002; Supplementary Fig. S2). Second, the height values were summarised as a proportion of canopy pixels, expressed as probability density or cumulative distribution functions (PDFs or CDFs), to compare tree heights by their relative contribution to the total canopy area (Fig. 6d). PDFs and CDFs were also created for canopy cover values over 30 m grids within each plot. Last, the height values were summarised in terms of total area of all pixels (0.01 m2 per pixel) within height bins, including non-vegetated areas, to describe the total volume under the canopy.
Ground-based ecological surveys
Ground surveys were conducted in January 2024, following substantial summer rainfall (>150 mm in November 2023; Fig. 3). This meant that ground layer vegetation was notably greener than that during the September 2023 drone survey, which occurred after nearly 12 months of low rainfall and an especially dry winter. The IVC protocol was followed, by using a nested circular plot design to record four metrics, namely, species richness, canopy health, weediness, and juvenile tree cover.
Species richness was assessed within an 80 m diameter circle (~0.5 ha) for large tree species and a 12 m diameter circle (~0.05 ha) for all vascular plants. Canopy health was estimated for each large tree (>2 m tall) within a 36 m diameter circle (~0.1 ha), on the basis of percentage canopy loss, with or without epicormic growth. Canopy health of 100% represented a tree with no loss, whereas 0% represented a completely dead tree. Health scores were binned into six increments (0, 1–25, 26–50, 51–75, 76–90 and 91–100%) and used the median increment and the ordinal variance to represent the plot. Weediness was calculated as the proportion of non-native to native species richness. The projective cover of juvenile trees, less than 1 m tall, was estimated in three 12 m radius circles (~0.05 ha), averaged to represent the plot.
Statistical analysis
Descriptive statistics were used to summarise the results within each vegetation management unit, including means and standard deviations. Non-parametric Wilcoxon rank-sum tests, which are suitable for small sample sizes, non-normally distributed data, and applicable to count data, proportions, and binned data, were used for comparisons between units. Pairwise comparisons were conducted between non-remnant and remnant units within each vegetation type (S1 vs S2, S3 vs S4) for variables including species richness, canopy health, weediness, and canopy and ground cover elements. All statistical tests were conducted using a significance level of α = 0.05. Statistical analyses were performed using R statistical software (ver. 4.1.0; R Core Team 2025).
Comparison of drone outputs with satellite-derived woody vegetation cover
Drone-derived canopy cover was contrasted with a national-scale satellite-based cover to demonstrate the importance of in situ data for rangelands vegetation monitoring. The woody cover fraction product (WCF; Liao et al. 2020) is a model of vegetation cover based on Landsat satellite imagery. WCF is interpreted as the complement of the canopy gap fraction whereas the lidar-based canopy cover measurements represented crown projective cover (CPC; Fisher et al. 2020). An empirical relationship was applied to the WCF product to approximate CPC (Fisher et al. 2018; Supplementary file S2). Satellite-derived canopy cover approximations were evaluated against lidar-derived cover by using RMSE, MAE, bias (absolute and relative), and R2.
Results
Strata summaries
Within the 18 ground plots, 143 vascular plant species were identified. Dominant canopy species included poplar box, mulga, Acacia excelsa (ironwood), and Acacia catenulate (bendee). Occasional Eucalyptus camaldulensis (river redgum) and Corymbia terminalis (bloodwood) were observed, along with other small trees and large shrubs (e.g. Eremophila spp.). The floristic surveys largely confirmed the adopted stratification approach. Plots followed the broad vegetation groups, which can include multiple canopy species, and were typically dominated by either poplar box or mulga. The primary canopy species in some Acacia woodlands plots was either poplar box or bendee, with some mulga being present, illustrating the complex mosaic of species within broad vegetation classifications. However, one plot was re-assigned from S4 (remnant Acacia woodlands) to S2 (remnant Eucalyptus woodlands) on the basis of the recorded species composition and proximity to the border of the two mapped vegetation types. Total species richness was similar in Acacia-dominated woodlands (90 species) and Eucalyptus woodlands (91 species; 59 species in common).
Non-remnant Eucalyptus woodland (S1) plots had the greatest richness on average (29 ± 6.8 species per plot; Table 1); however, there were no significant differences in richness between vegetation management units (S1 vs S2: P = 0.54; S3 vs S4: P = 1). Median canopy health was high (more than half of trees had >75% live canopy) in all plots that had trees. There were no significant differences in plot-level median health between vegetation management units (S1 vs S2: P = 0.24; S3 vs S4: P = 0.85). Dispersion around the median was higher in remnant vegetation management units (S2: 0.6 median variance within plots; S4: 2.4) than in non-remnant units (S1: 0.0; S2: 0.2). Weediness was low in all plots (≤5% of richness was non-native) and no significant differences were identified between vegetation management units (S1 vs S2: P = 0.71; S3 vs S4: P = 0.71). Low woody vegetation (<1 m) was dominated by native shrub species in most plots; however, juvenile trees were present in all strata. No significant differences in juvenile tree cover were identified between vegetation management units (S1 vs S2: P = 0.10; S3 vs S4: P = 0.62).
Vegetation unit | Area surveyed (ha) | Number of plots | Median species richness (total richness) | Canopy health (%) | Weediness (%, ±s.d.) | Juvenile tree canopy in woody vegetation <1 m (%, ±s.d.) | |
---|---|---|---|---|---|---|---|
S1 (non-remnant Eucalyptus woodlands) | 2 | 4 | 29 (60) | 90–100 | 5 (±4) | 19 (±25) | |
S2 (remnant Eucalyptus woodlands) | 2.5 | 5 | 24 (67) | 90–100 | 7 (±2) | 11 (±24) | |
S3 (non-remnant Acacia woodlands) | 2.5 | 5 | 24 (69) | 90–100 | 6 (±3) | 11 (±15) | |
S4 (remnant Acacia woodlands) | 2 | 4 | 25 (57) | 50–75 | 4 (±5) | 39 (±46) |
Most sites are nested within drone plots (see Fig. 2).
Classification accuracy
An initial assessment of the classification accuracy, including all processed plots, yielded an OA of 76% (95% CI: 72–79). However, on closer inspection, it was determined that four plots were classified poorly, exhibiting accuracies between 50% and 60%. In two of these plots, a possible cause was uncharacteristically dark imagery, whereas the other two cases lacked an obvious cause. These poor classifications were determined to be insufficiently reliable for our intended condition assessment and were removed, excluded from the subsequent analysis. This decision is justified as a form of quality control because our primary objective was to accurately assess ecological condition. Analyses and conclusions are explicitly limited to the metrics derived from these 29 orthomosaic plots and their aggregation within strata. Therefore, the accuracy estimates reported below reflect the reliability of these 29 classifications only and should not be interpreted as a general performance metric for the U-Net model across the wider landscape or for future applications. Since misclassifications in the four excluded plots were seemingly unrelated to any biophysical properties of the plot areas, their removal should not have biased the condition assessment of affected strata.
The estimated OA for the final set of 29 plots was 79% (95% CI: 75–82; Table 2), and OAA increased to 81% (95% CI: 77–85) when areas classified as shadow were disregarded. Among the target classes, bare ground and woody canopy classes were estimated to be the most complete classifications (lowest omission error and highest PA) (PAA = 98% and 88% respectively), whereas woody cover and ground cover were classified most precisely (lowest commission error and highest UA) (UA = 90 and 83%). Conversely, ground cover was estimated to be less complete (PAA = 58%), and observed ground cover points were frequently misclassified as bare ground. Woody debris exhibited the lowest accuracy among all classes (PAA = 58%; UA = 69%), frequently being confused with ground cover. Woody debris was uncommon within the samples collected for accuracy assessment.
Cover and structural summaries
Drone-derived data showed substantial differences in cover composition among vegetation management units. Lidar showed that woody cover was highest in the remnant Acacia woodlands (S4: 44.8%; Table 3) compared with non-remnant Acacia woodlands (S3: 23.7%) and non-remnant Eucalyptus woodlands (S1: 14.6%). The sole drone plot recategorised as remnant Eucalyptus woodlands had 26.4% canopy cover. The coefficient of variation (CV) for canopy cover ranged from 30.4% (S4) to 58.1% (S3), indicating considerable heterogeneity among these woodland systems. Classified orthomosaics were observed to underpredict lidar-derived woody cover (Supplementary Fig. S2), but the differences between vegetation management units were consistent. Higher canopy cover in remnant Acacia woodlands (S4) coincided with insignificant differences in visible woody debris (S3 vs S4: P = 0.4) and ground cover (S3 vs S4: P= 0.47). The total planimetric cover of canopies and ground layer elements (i.e. the complement to bare ground) was similar between vegetation management units.
Vegetation unit | Hectares (% area) | Hectares surveyed (classified) | Number of plots (classified) | Bare ground (%, ±s.d.) | Ground cover (%, ±s.d.) | Coarse woody debris (%, ±s.d.) | Woody vegetation (%, ±s.d.) | Canopy of any height (%, ±s.d.) | Canopy below 2 m tall (%, ±s.d.) | Canopy above 2 m tall (%, ±s.d.) | |
---|---|---|---|---|---|---|---|---|---|---|---|
S1 (non-remnant Eucalyptus woodlands) | 3179 (11) | 18.8 (18.8) | 3 (3) | 66.1 (±10.8) | 20.9 (±10.3) | 1.4 (±0.4) | 11.5 (±4.5) | 14.6 (±6.7) | 0.8 (±0.2) | 13.8 (±6.5) | |
S2 (remnant Eucalyptus woodlands) | 670 (2) | 6.3 (6.3) | 1 (1) | 66.3 | 15 | 1.9 | 16.8 | 26.4 | 0.5 | 25.9 | |
S3 (non-remnant Acacia woodlands) | 16,546 (60) | 137.5 (118.8) | 22 (19) | 51.7 (±13.6) | 25.9 (±13) | 2.7 (±2.4) | 19.7 (±8.8) | 23.7 (±13.8) | 1.2 (±0.9) | 22.5 (±14) | |
S4 (remnant Acacia woodlands) | 7295 (26) | 62.5 (37.5) | 10 (6) | 44.9 (±22.9) | 22.9 (±8.5) | 3.9 (±3.6) | 28.2 (±11.5) | 44.8 (±13.6) | 0.4 (±0.3) | 44.4 (±13.7) |
One site was incidentally surveyed in S2, having been originally designated S4 but reallocated (see results description). Canopy metrics are derived from lidar data (grey; n = 36) and other elements are derived from classified orthomosaics (n = 29) with shadows removed.
Analysis of vegetation structure showed bimodal height distributions in both Acacia-dominated strata (S3 and S4), with distinct vertical strata at 1–4 and 10–12 m tall (Fig. 7). The lower strata comprised a mixture of regenerating canopy species (e.g. mulga and poplar box) and smaller midstorey trees and shrubs, interpreted from survey data and photos. Overall, vegetation in non-remnant Acacia woodlands (S3) was shorter, with ~85% of treetops less than 5 m tall compared with only ~22% of treetops in remnant Acacia woodlands (S4). Canopy pixel heights showed a greater proportion concentrated above 5 m, reflecting the larger contribution of taller (and wider) trees to the total canopy area. Remnant Acacia woodlands (S4) had a right-shifted canopy height distribution and a concentration of tall trees, indicating mature woodland structure. In non-remnant Acacia woodlands (S3), canopy pixel height was more concentrated around the 2.5 m mode than the 10 m mode. Substantial variation in canopy height distributions was observed, particularly in non-remnant Acacia woodlands (S3). Two plots in S4 were distinct outliers, with up to 80% of canopy pixels below 5 m. Some variation was the result of drone plots crossing boundaries between strata, but the variation largely reflected the heterogeneous condition of woodland ecosystems (e.g. regenerating, open areas within remnant forest). Canopy cover was unevenly distributed within plots, with PDFs generally lacking strong peaks, indicating heterogeneous canopy structure at the plot scale (Fig. 7). Where peaks in cover probability density were observed, they tended to occur at higher coverage values in remnant woodlands (S4) than in non-remnant woodlands (S3), suggesting greater canopy cover heterogeneity in areas with a history of clearing.
(Left) Tree height, (middle) canopy height, and (right) canopy cover distributions for all drone plots for remnant Acacia woodlands (Stratum 3) and non-remnant Acacia woodlands (Stratum 4). Distributions are shown using probability density functions (PDF) and empirical cumulative density functions (ECDF). Individual plots are shown in light blue and grey (n = 32), whereas the solid lines represent the distributions for all plots pooled per stratum.
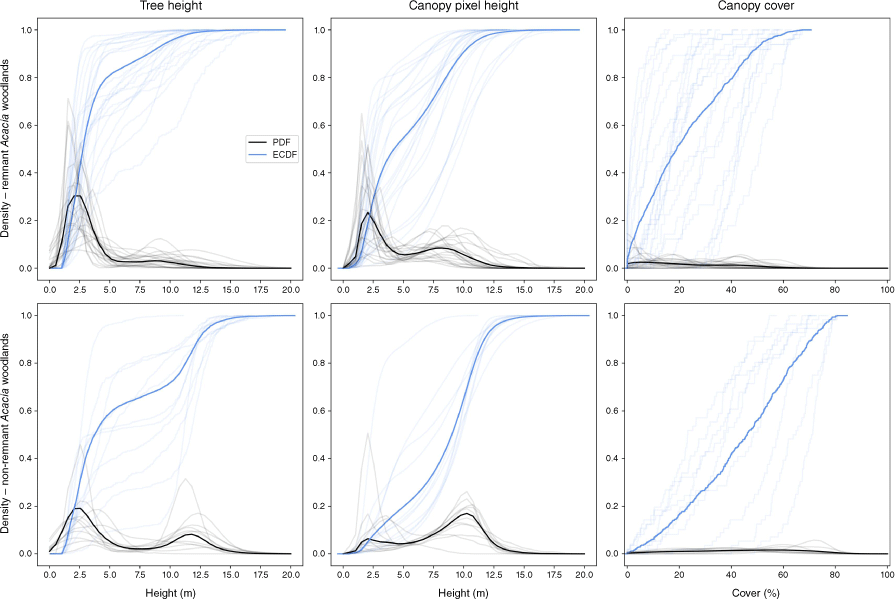
To demonstrate how structural analysis can be applied, four plots from remnant and non-remnant Acacia woodlands (S3 and S4) were selected (non-randomly) to present an idealised progression of recovering vegetation states (Fig. 8). Frequency distributions of canopy height are depicted in an ascending order of tree height and cover, from recent regeneration (new recruits, 1–3 m tall) to mature ecosystems (a mixture of large trees and new recruits, 1–14 m tall). Mixtures of size cohorts may be present in intermediate states (e.g. Fig. 8c) owing to recovery from non-uniform disturbance, multiple recruitment events and/or a diversity of species/growth forms. A notable trend is that canopy height scales with canopy extent, representing total woody biomass of the plot.
Comparison with satellite-derived canopy cover
A key application of drone-based surveys is to provide in situ reference data for verification of satellite-derived maps. The WCF model, derived from Landsat imagery, exhibited a weak correlation with drone-measured woody cover (R2 = 0.29; Fig. 9), high error (RMSE = 15.9 percentage points), and a consistent tendency to underestimate canopy cover. Absolute bias was greatest where observed canopy is high (>60% canopy cover); however, relative bias was greatest for sparsely vegetated areas, where the WCF tended to estimate less than 5% cover (often near 0%) in areas with lidar-based estimates up to 20% (the threshold cover for ‘forest’).
Comparison of drone- and satellite-derived canopy cover estimates. Drone canopy cover here represents canopy areas with at least some pixels reaching 2 m in height. (a) Scatter plot with summary error statistics. Grey diagonal line indicates 1:1 equivalence. (b) Absolute and relative bias in Landsat-based cover.
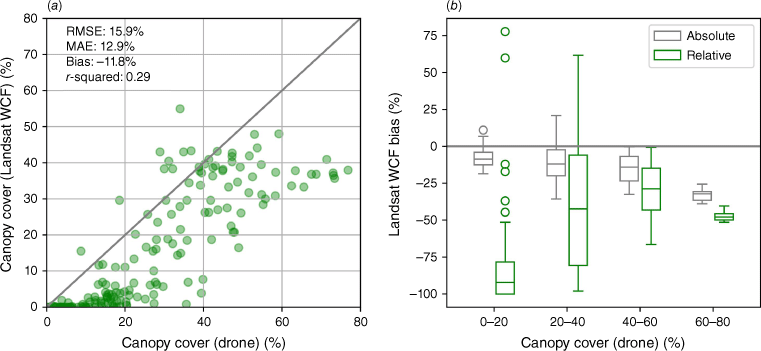
Discussion
Carbon farming through an ecological lens
Carbon farming projects in Australian rangelands offer an unexpected opportunity to enhance the understanding of these vast and complex ecosystems. This study has demonstrated the potential of repurposing carbon farming project data for ecological monitoring in rangelands at scales that have been challenging to capture with traditional methods. In particular, the use of drones bridges the gap between broad-scale satellite observations and localised ground surveys, providing a more comprehensive view of key ecosystem attributes such as ground cover, woody debris, canopy structure and heterogeneity. Although the primary purpose of these drone surveys was to determine area eligibility for carbon farming activities under the Carbon Credits (Carbon Farming Initiative) Act (2011), the data are well suited for landscape condition assessment.
These findings have showed structurally diverse vegetation that is not observed in remotely sensed tree cover metrics. In Acacia-dominated strata, a bimodal distribution of tree heights was observed; a higher proportion of treetops in non-remnant Acacia woodlands (S3) was under 5 m tall than in remnant Acacia woodlands (S4). This difference reflects varied land-use histories and stages of recovery. Variation of tree heights and canopy cover within non-remnant vegetation units is likely to reflect complex disturbance histories and a progression of structural states that vary across the landscape (Fig. 8). Dividing broad vegetation groups by their regulated clearing eligibility does not pre-suppose that vegetation is even aged. Instead, this coarse stratification reflects the aggregate condition of vegetation management units that includes regenerating and mature vegetation. These findings support the idea that long-term recovery from land management practices that promote clearing and disturbance is not a uniform process. Drone-borne lidar provides the means to quantify structural differences at a landscape scale, offering a new perspective on the recovery potential and resilience of rangeland vegetation.
Combining drone-derived structural data with ground-based floristic surveys can show patterns that might otherwise be missed when using remote sensing alone. The observation of somewhat consistent species richness across different vegetation management units suggests a more complex relationship between disturbance and biodiversity than is often assumed. This finding, coupled with the consistently low weediness (4–7%) across all strata, indicates a degree of ecological resilience in rangelands ecosystems. It suggests that native species can maintain their presence even in areas with a history of clearing and grazing, which has important implications for restoration and conservation strategies. Further, the addition of ground surveys for canopy health and the proportion of juvenile trees across provides insights the long-term trajectory of these ecosystems. Although significant differences between strata were not observed, the presence of juvenile trees indicates that disturbed ecosystems have regenerative capacity and the potential to return to states resembling remnant vegetation. Information collected on remnant vegetation, or at intact reference sites, can be used to develop benchmarks for condition assessments in heavily modified landscapes.
While this study has provided valuable insights from a single timepoint, greater potential lies in the ongoing monitoring inherent to carbon farming projects. The monitoring programmes of HIR project involve regular data collection over 25-year crediting periods, offering an important opportunity for long-term ecological research in rangelands. These monitoring programmes could track shifts in vegetation structure as woody plants mature, document the recovery trajectories of different species assemblages following disturbance, or detect the impacts of climate change on rangeland ecosystems. Ground cover and ground layer richness may be more susceptible to short-term changes, likely responding to herbivory and recent climate conditions (Friedel 1997; Landsberg et al. 2003; Witt et al. 2011). Although the adopted stratification is probably best suited to comparing woody vegetation structure, the ecological responses of non-woody vegetation to broad stocking rates and climatic variation should be detectable within vegetation management units (Sinclair and Facelli 2018). Moreover, because carbon farming projects are distributed across diverse rangeland types, they create a network of long-term monitoring sites that can capture regional variations in ecosystem responses to both management interventions and environmental changes. The value of distributed monitoring networks increases exponentially over time, with potentially transformative potential to inform adaptive management strategies that can balance carbon sequestration goals with broader ecological outcomes.
Drones bridge a gap in rangeland vegetation monitoring
Drone-based data collection offers a unique complement to existing rangeland monitoring approaches by addressing a fundamental challenge, namely, bridging the gap between broad-scale satellite observations and localised ground measurements. There is a common trade-off in the design of monitoring programmes among coverage, frequency, and detail.
Satellite-based approaches offer extensive coverage but cannot resolve fine-scale features. Publicly available products calibrated and produced at national scales must be used with caution. These limitations are exemplified by the strong underestimates of canopy cover provided by the WCF model (Fig. 9). Satellite-based monitoring often requires regional or local calibration to achieve accuracy suitable for property-scale assessments scale (Shumack et al. 2021), particularly in rangelands where soil and vegetation spectral signatures differ significantly from those in higher-rainfall areas. Further, scientific-grade optical satellite datasets such as Sentinel-2 and Landsat, although being widely used, are limited to mapping simplified descriptors such as green, non-photosynthetic, and woody vegetation cover (e.g. Guerschman et al. 2015; Gill et al. 2017; Liao et al. 2020; Shumack et al. 2021). These datasets often struggle to disentangle changes in vegetation composition, further highlighting the need for finer-scale data sources (cf. Allred et al. 2021).
At the other end of the spectrum, ground-based surveys can obtain detailed floristic data, such as species composition for both the ground layer and canopy species. However, they are limited in the area they can cover at any given site or plot, usually less than 1 ha. Rangeland ecosystems are typically patchy, where vegetation structure and condition can change over tens or hundreds of metres. This spatial heterogeneity means that many ground surveys are required to obtain a representative sample (Rudge et al. 2022). As such, ground surveys are often impractical for monitoring vast rangelands landscapes because of economic constraints and bias (Booth and Tueller 2003). Drones address the coverage limitations of ground-based surveys and can be integrated with ground surveys to overcome challenges associated with detailed species identification. Drones, with their higher resolution and ability to capture three-dimensional structure, offer a valuable tool for bridging the gap between broad-scale satellite observations and localised ground measurements.
Given the complementary strengths of ground-, drone- and/or satellite-based approaches, several programmes have attempted to integrate multiple methods to achieve more comprehensive vegetation assessments. The Queensland Government’s Spatial BioCondition protocol combines ground-based surveys with satellite imagery for vegetation condition mapping (Spatial BioCondition: Vegetation Condition Map for Queensland 2021), but is ultimately subject to the resolution constraints of satellite datasets. Similarly, the Statewide Landcover and Trees Study (SLATS) programme for monitoring the extent and change in woody vegetation (Statewide Landcover and Trees Study Queensland Landsat Series 2023) relies on a combination of ground surveys and satellite imagery. Although there are existing research protocols for combining drone data with ground surveys, these remain limited in scope (Retallack et al. 2023). Australia’s Terrestrial Ecosystem Research Network (TERN) has begun hosting drone data (TERN Landscapes et al. 2022; see also Cunliffe et al. 2022) and their network of SuperSites has been imaged with airborne sensors and assessed with a ground protocol (Karan et al. 2016), including two sites from Australia’s rangelands. Although the recently released guidelines by TERN for drone-data acquisition and processing anticipate an increasing adoption of these technologies (Sivanandam and Turner 2023), we believe that carbon farming projects currently deliver the only broadscale application of drones in the rangelands. Extending data collections beyond the regulatory requirements of vegetation mapping requires a vegetation condition monitoring framework that can be applied at the scale of large rangeland properties. This case study has indicated that the IVC protocol can realistically fulfil this requirement.
Methodological considerations and technical challenges
The the stratification approach of this study, based on broad vegetation groups and clearing history, effectively captured major differences in vegetation structure and composition. However, the observed heterogeneity within strata, particularly in non-remnant Acacia woodlands (S3), highlights the complexity of rangeland ecosystems. This complexity presents a challenge for delineating management units and selecting benchmark sites or statistics for condition assessments. Heterogeneity is to be expected within the broad vegetation groups used in this study. For instance, structural variation within a stratum, such as a mix of poplar box and mulga, could be mistaken for differences in condition or recovery stage. Therefore, when selecting benchmarks, careful consideration of what constitutes an ‘ideal’ ecological state for a given vegetation type must be given. Furthermore, the variation in canopy cover, height distributions and dominant canopy species underscores the importance of robust sampling designs that account for within-strata variability and the need for careful interpretation of results in heterogeneous landscapes.
Drone-based data collection offers advantages over ground surveys. Drone platforms can cover larger areas and access sites that are difficult or dangerous for ground crews to reach. However, current regulations often restrict drone operation to within 1 km of roads and tracks, limiting their full potential. The trade-off between flying time and the impact of shadows similarly curtails their applicability. Additionally, drone-derived data are less effective for ground-layer characterisation in dense canopies, requiring supplementary ground surveys. Ground-based surveys remain the most reliable method for detailed species identification. Combining drone and ground survey data is substantially more straightforward than building species detection systems with drone data alone. Coordinating drone and ground surveys can be challenging. This can lead to mismatches between the timing and spatial distribution of data. This study experienced such a mismatch, with drone surveys being conducted in September 2023 and ground surveys in January 2024. This resulted in ground layer vegetation appearing notably greener in the ecological surveys compared to the drone imagery. Although such phenological differences can complicate data interpretation, their impact may be less significant in a long-term monitoring programme.
This study highlighted three technical limitations of drone surveys that should be considered in future applications. First, the orthorectification process, which is used to create orthomosaics from multiple overlapping images, can sometimes produce blurred artefacts. This blurring may have contributed to the discrepancy between the canopy cover estimated from the image classification and the lidar-based estimate (Supplementary Fig. S2). Blurring was especially noticeable in the canopies of taller trees with sparse canopies, where even small amounts of wind can create movement between image captures and make feature-matching difficult. Dense, low-lying vegetation was usually better resolved. Second, pre-processing lidar and camera data separately sometimes resulted in spatial misalignment between the two datasets. Improved co-registration of these datasets would enhance the efficiency of the classification process by allowing height information to contribute to distinguishing ground and canopy layers. Adoption of multispectral or hyperspectral sensors could also increase the spectral separability of target cover components (e.g. Melville et al. 2019; Sankey et al. 2021). Finally, while the U-Net model was shown to be generally effective for estimating cover metrics in this study, the exclusion of four poorly classified plots (with accuracies between 50% and 60%) underscores the need for extensive training data to ensure reliable generalisation across a broader region. Model accuracy may be further enhanced with the collection of on-ground datasets required for verification of drone-based estimates of cover elements, such as debris. Addressing these methodological considerations will be crucial for improving the reliability and interpretability of data collected through this integrated approach.
Future directions and policy implications
The integration of carbon farming project data into ecological monitoring frameworks represents a novel approach to leverage existing investments for broader environmental benefits. The potential to scale up rangeland monitoring by using this method is particularly large in the semi-arid areas of New South Wales, Queensland, and Western Australia where existing HIR projects are concentrated, with more recent projects expanding into central and northern Australia (Supplementary Fig. S1). While the HIR method is now closed to new project registrations, this has little impact on the data collected by existing projects, which will continue monitoring throughout their 25-year crediting periods. This long-term perspective offers a unique chance to study the long-term effects of management practice change in ecosystems with complex and gradual recovery processes (Westoby 1979).
Future carbon farming and nature repair methods will likely require greater ecological understanding than the broad stroke of ‘forest attainment’. Key policy innovations are moving towards increased measurement and management of variables beyond carbon sequestration, such as biodiversity, ecosystem condition, and habitat quality. The protocols presented here can be leveraged in new methods, allowing for greater nuance in policies incentivising ecological change. For example, structural information on ecological condition, such as gradients in canopy size or volume distributions (e.g. Fig. 8), is likely to play an increasing role in carbon farming and nature repair methods that integrate ecosystem restoration science (e.g. Standards Reference Group SERA 2021). The approach shown here addresses current gaps in rangeland monitoring by providing high-resolution, property-scale data that could capture both structural and compositional changes over time.
This case study, although limited to a single site and time point, has illustrated the potential of this approach. Further applications of the integrated vegetation condition (IVC) method, such as those being undertaken as part of the Queensland Landscape Restoration Fund, will evaluate its suitability across a range of ecosystems and management contexts. The 25-year span of carbon farming projects opens opportunities for repeated assessments and replication across environmental gradients and vegetation communities. Moreover, the detailed management histories collected as part of carbon farming projects provide crucial context for interpreting ecological changes, addressing a common limitation in large-scale observational studies. However, research drawing on carbon farming data will need to address challenges such as data standardisation and integration across a range of diverse ecosystems, land management contexts and agricultural organisations. Leveraging data collections for carbon farming for research and monitoring approach enhances our ability to monitor and understand rangeland ecosystems, and can inform more nuanced and effective environmental policies, ultimately contributing to more sustainable management of Australia’s rangelands.
Conclusions
As we face increasing environmental challenges, including climate change and biodiversity loss, the need for comprehensive, efficient monitoring of vast rangelands becomes ever more critical. It has here been demonstrated that drone surveys used for monitoring carbon farming projects are also well suited for monitoring vegetation condition in the Australian rangelands. Condition indicators derived from drone data, such as tree size distributions, canopy area and ground layer coverage, are valuable for property-scale decision making and understanding of the broader ecological effects of woody vegetation regrowth. The approach presented here extends carbon farming monitoring for broader ecological insights, offering a promising path forward.
Conflicts of interest
The authors work for Climate Friendly and receive financial renumeration from the adoption and performance of carbon farming projects.
Acknowledgements
This paper could not have happened without support from Rencon Air & Land, John & Vanessa Hunter, and other Climate Friendly staff, with special thanks to Molly Blackburn, Rumi Salazar, Madhav Padmakumar, and Jay Hender. The authors thank Wyuna Ag. for allowing us to use their property as a case study and we thank our collaborators at Bush Heritage Australia for their contributions to the IVC protocol.
References
Allred BW, Bestelmeyer BT, Boyd CS, Brown C, Davies KW, Duniway MC, Ellsworth LM, Erickson TA, Fuhlendorf SD, Griffiths TV, Jansen V, Jones MO, Karl J, Knight A, Maestas JD, Maynard JJ, McCord SE, Naugle NE, Starns HD, Twidwell D, Uden DR (2021) Improving Landsat predictions of rangeland fractional cover with multitask learning and uncertainty. Methods in Ecology and Evolution 12, 841-849.
| Crossref | Google Scholar |
Amputu V, Knox N, Braun A, Heshmati S, Retzlaff R, Röder A, Tielbörger K (2023) Unmanned aerial systems accurately map rangeland condition indicators in a dryland savannah. Ecological Informatics 75, 102007.
| Crossref | Google Scholar |
Anderson V, Hodgkinson K, Grice A (1996) The influence of recent grazing pressure and landscape position on grass recruitment in a semi-arid woodland of eastern Australia. The Rangeland Journal 18(1), 3-9.
| Crossref | Google Scholar |
Baumber A, Waters C, Cross R, Metternicht G, Simpson M (2020) Carbon farming for resilient rangelands: people, paddocks and policy. The Rangeland Journal 42(5), 293-307.
| Crossref | Google Scholar |
Beutel TS, Trevithick R, Scarth P, Tindall D (2019) VegMachine.net. online land cover analysis for the Australian rangelands. The Rangeland Journal 41(4), 355.
| Crossref | Google Scholar |
Booth DT, Tueller PT (2003) Rangeland monitoring using remote sensing. Arid Land Research and Management 17(4), 455-67.
| Crossref | Google Scholar |
Breiman L (2001) Random forests. Machine Learning 45(1), 5-32.
| Crossref | Google Scholar |
Brown R (1985) The growth and survival of young mulga (Acacia aneura F.Muell) trees under different levels of grazing. The Rangeland Journal 7(2), 143-148.
| Crossref | Google Scholar |
Bush Heritage Australia & Climate Friendly (2022) AfN-METHOD-NV-10: Integrated Vegetation Condition Method. In ‘Method catalogue’. (Accounting for Nature). Available at https://www.accountingfornature.org/method-catalogue
Clean Energy Regulator (2024) ACCU Project and Contract Register. Available at https://cer.gov.au/markets/reports-and-data/accu-project-and-contract-register [retrieved 3 March 2024]
Clean Energy Regulator (2019) Guidelines on stratification, evidence and records or projects under the Human-Induced Regeneration of a Permanent Even-Aged Native Forest and Native Forest from Managed Regrowth methods. https://cer.gov.au/document/guidelines-stratification-evidence-and-records-hir-and-nfmr
Cohn JS, Lunt ID, Bradstock RA, Hua Q, McDonald S (2013) Demographic patterns of a widespread long‐lived tree are associated with rainfall and disturbances along rainfall gradients in SE Australia. Ecology and Evolution 3(7), 2169-2182.
| Crossref | Google Scholar | PubMed |
Cunliffe AM, Brazier RE, Anderson K (2016) Ultra-fine grain landscape-scale quantification of dryland vegetation structure with drone-acquired structure-from-motion photogrammetry. Remote Sensing of Environment 183, 129-143.
| Crossref | Google Scholar |
Cunliffe AM, Anderson K, Boschetti F, Brazier RE, Graham HA, Myers-Smith IH, Astor T, Boer MM, Calvo LG, Clark PE, Cramer MD, Encinas-Lara MS, Escarzaga SM, Fernández-Guisuraga JM, Fisher AG, Gdulová K, Gillespie BM, Griebel A, Hanan NP, et al. (2022) Global application of an unoccupied aerial vehicle photogrammetry protocol for predicting aboveground biomass in non‐forest ecosystems. Remote Sensing in Ecology and Conservation 8(1), 57-71.
| Crossref | Google Scholar | PubMed |
Department of Environment and Science (2021) Spatial BioCondition: Vegetation condition map for Queensland. Queensland Government, Brisbane. https://www.qld.gov.au/__data/assets/pdf_file/0015/230019/spatial-biocondition-vegetation-condition-map-for-queensland.pdf
DJI (2023) DJI Terra. Shenzhen: DJI. Available at https://www.dji.com/au/dji-terra/info
Fensham RJ, Powell O, Horne J (2011) Rail survey plans to remote sensing: vegetation change in the Mulga Lands of eastern Australia and its implications for land use. The Rangeland Journal 33(3), 229-238.
| Crossref | Google Scholar |
Fisher A, Scarth P, Armston J, Danaher T (2018) Relating foliage and crown projective cover in Australian tree stands. Agricultural and Forest Meteorology 259, 39-47.
| Crossref | Google Scholar |
Fisher A, Armston J, Goodwin N, Scarth P (2020) Modelling canopy gap probability, foliage projective cover and crown projective cover from airborne lidar metrics in Australian forests and woodlands. Remote Sensing of Environment 237, 111520.
| Crossref | Google Scholar |
Friedel MH (1997) Discontinuous change in arid woodland and grassland vegetation along gradients of cattle grazing in central Australia. Journal of Arid Environments 37(1), 145-164.
| Crossref | Google Scholar |
Gill T, Johansen K, Phinn S, Trevithick R, Scarth P, Armston J (2017) A method for mapping Australian woody vegetation cover by linking continental-scale field data and long-term Landsat time series. International Journal of Remote Sensing 38(3), 679-705.
| Crossref | Google Scholar |
Gillan JK, Karl JW, van Leeuwen WJD (2020) Integrating drone imagery with existing rangeland monitoring programs. Environmental Monitoring and Assessment 192(5), 269.
| Crossref | Google Scholar | PubMed |
Guerschman JP, Scarth PF, McVicar TR, Renzullo LJ, Malthus TJ, Stewart JB, Rickards JE, Trevithick R (2015) Assessing the effects of site heterogeneity and soil properties when unmixing photosynthetic vegetation, non-photosynthetic vegetation and bare soil fractions from Landsat and MODIS data. Remote Sensing of Environment 161, 12-26.
| Crossref | Google Scholar |
Harrington G (1979) The effects of feral goats and sheep on the shrub populations in a semi-arid woodland. The Rangeland Journal 1(4), 334-345.
| Crossref | Google Scholar |
Howard J, Gugger S (2020) Fastai: a layered API for deep learning. Information 11(2), 108.
| Crossref | Google Scholar |
Jones P, Burrows WH (1994) State and transition models for rangelands. 13. A state and transition model for the mulga zone of south-west Queensland. Tropical Grasslands 28, 279-283.
| Google Scholar |
Karan M, Liddell M, Prober SM, Arndt S, Beringer J, Boer M, Cleverly J, Eamus D, Grace P, Van Gorsel E, Hero J-M, Hutley L, Macfarlane C, Metcalfe D, Meyer W, Pendall E, Sebastian A, Wardlaw T (2016) The Australian SuperSite Network: a continental, long-term terrestrial ecosystem observatory. Science of The Total Environment 568, 1263-1274.
| Crossref | Google Scholar | PubMed |
Kattenborn T, Eichel J, Fassnacht FE (2019) Convolutional Neural Networks enable efficient, accurate and fine-grained segmentation of plant species and communities from high-resolution UAV imagery. Scientific Reports 9, 17656.
| Crossref | Google Scholar | PubMed |
Landsberg J, James CD, Morton SR, Müller WJ, Stol J (2003) Abundance and composition of plant species along grazing gradients in Australian rangelands. Journal of Applied Ecology 40(6), 1008-1024.
| Crossref | Google Scholar |
Levick SR, Whiteside T, Loewensteiner DA, Rudge M, Bartolo R (2021) Leveraging TLS as a calibration and validation tool for MLS and ULS mapping of savanna structure and biomass at landscape-scales. Remote Sensing 13(2), 257.
| Crossref | Google Scholar |
Liao Z, Van Dijk AIJM, He B, Larraondo PR, Scarth PF (2020) Woody vegetation cover, height and biomass at 25-m resolution across Australia derived from multiple site, airborne and satellite observations. International Journal of Applied Earth Observation and Geoinformation 93, 102209.
| Crossref | Google Scholar |
Ludwig JA, Tongway DJ, Hindley N (2024) Can simple, on-ground vegetation and soil measures reliably indicate the health of rangelands? An application in Australia’s semi-arid woodlands. The Rangeland Journal 45(6), 235-245.
| Crossref | Google Scholar |
Lyons MB, Keith DA, Phinn SR, Mason TJ, Elith J (2018) A comparison of resampling methods for remote sensing classification and accuracy assessment. Remote Sensing of Environment 208, 145-153.
| Crossref | Google Scholar |
Melville B, Fisher A, Lucieer A (2019) Ultra-high spatial resolution fractional vegetation cover from unmanned aerial multispectral imagery. International Journal of Applied Earth Observation and Geoinformation 78, 14-24.
| Crossref | Google Scholar |
Novelly PE, Watson IW, Thomas PWE, Duckett NJ (2008) The Western Australian Rangeland Monitoring System (WARMS) - operating a regional scale monitoring system. The Rangeland Journal 30(3), 271-281.
| Crossref | Google Scholar |
Peeters PJ, Butler DW (2014) ‘Mulga – Regrowth benefits management guideline.’ (Department of Science, Information Technology, Innovation and the Arts: Brisbane, Qld, Australia) Available at https://www.qld.gov.au/__data/assets/pdf_file/0025/68191/mulga-management-guideline.pdf
Penman J, Green C, Olofsson P, Foody GM (2016) ‘Integration of remote-sensing and ground-based observations for estimation of emissions and removals of greenhouse gases in forests.’ (Global Forest Observations Initiative) Available at https://www.reddcompass.org/mgd/resources/GFOI-MGD-3.1-en.pdf
Popescu SC, Wynne RH, Nelson RF (2002) Estimating plot-level tree heights with lidar: local filtering with a canopy-height based variable window size. Computers and Electronics in Agriculture 37(1–3), 71-95.
| Crossref | Google Scholar |
R Core Team (2025) R: A Language and Environment for Statistical Computing. R Foundation for Statistical Computing, Vienna, Austria. https://www.R-project.org/
Rango A, Laliberte A, Steele C, Herrick JE, Bestelmeyer B, Schmugge T, Roanhorse A, Jenkins V (2006) Using unmanned aerial vehicles for rangelands: current applications and future potentials. Environmental Practice 8(3), 159-168.
| Crossref | Google Scholar |
Rango A, Laliberte A, Herrick JE, Winters C, Havstad K, Steele C, Browning D (2009) Unmanned aerial vehicle-based remote sensing for rangeland assessment, monitoring, and management. Journal of Applied Remote Sensing 3(1), 033542.
| Crossref | Google Scholar |
Retallack A, Finlayson G, Ostendorf B, Clarke K, Lewis M (2023) Remote sensing for monitoring rangeland condition: current status and development of methods. Environmental and Sustainability Indicators 19, 100285.
| Crossref | Google Scholar |
Ronneberger O, Fischer P, Brox T (2015) U-Net: Convolutional networks for biomedical image segmentation. In ‘Medical Image Computing and Computer-Assisted Intervention – MICCAI 2015’. Lecture Notes in Computer Science. (Ed. N Nassir) pp. 234–241. (Springer International Publishing: Cham, Switzerland) 10.1007/978-3-319-24574-4_28
Rudge MLM, Levick SR, Bartolo RE, Erskine PD (2021) Modelling the diameter distribution of savanna trees with drone-based LiDAR. Remote Sensing 13, 1266.
| Crossref | Google Scholar |
Rudge MLM, Levick SR, Bartolo RE, Erskine PD (2022) Developing landscape-scale forest restoration targets that embrace spatial pattern. Landscape Ecology 37(7), 1747-1760.
| Crossref | Google Scholar |
Sankey TT, Leonard JM, Moore MM (2019) Unmanned aerial vehicle-based rangeland monitoring: examining a century of vegetation changes. Rangeland Ecology & Management 72(5), 858-863.
| Crossref | Google Scholar |
Sankey JB, Sankey TT, Li J, Ravi S, Wang G, Caster J, Kasprak A (2021) Quantifying plant-soil-nutrient dynamics in rangelands: fusion of UAV hyperspectral-LiDAR, UAV multispectral-photogrammetry, and ground-based LiDAR-digital photography in a shrub-encroached desert grassland. Remote Sensing of Environment 253, 112223.
| Crossref | Google Scholar |
Shumack S, Fisher A, Hesse P (2021) Refining medium resolution fractional cover for arid Australia to detect vegetation dynamics and wind erosion susceptibility on longitudinal dunes. Remote Sensing of Environment 265, 112647.
| Crossref | Google Scholar |
Sinclair R, Facelli JM (2018) Ninety years of change on the TGB Osborn Vegetation Reserve, Koonamore: a unique research opportunity. The Rangeland Journal 41(3), 185-187.
| Crossref | Google Scholar |
Sivanandam P, Turner D (2023) ‘Drone Data Collection Protocol using DJI Matrice 300 RTK: Imagery and Lidar.’ (The Terrestrial Ecosystem Research Network) Available at https://www.tern.org.au/wp-content/uploads/20230829_drone_data_collection.pdf
Sparrow BD, Foulkes JN, Wardle GM, Leitch EJ, Caddy-Retalic S, van Leeuwen SJ, Tokmakoff A, Thurgate NY, Guerin GR, Lowe AJ (2020) A vegetation and soil survey method for surveillance monitoring of rangeland environments. Frontiers in Ecology and Evolution 8, 157.
| Crossref | Google Scholar |
Standards Reference Group SERA (2021) ‘National Standards for the Practice of Ecological Restoration in Australia. Edition 2.2.’ (Society for Ecological Restoration Australasia) Available at https://www.seraustralasia.com/standards/NationalStandards2_2.pdf
Statewide Landcover and Trees Study Queensland Landsat Series (2023) ‘Open Data Portal.’ (Department of Environment, Science and Innovation) Available at https://www.data.qld.gov.au/dataset/statewide-landcover-and-trees-study-queensland-landsat-series
Stewart AJ, Robinson C, Corley IA, Ortiz A, Ferres JML, Banerjee A (2022) TorchGeo. In ‘Proceedings of the 30th International Conference on Advances in Geographic Information Systems’. pp. 1–12. (ACM: New York, NY, USA) 10.1145/3557915.3560953
Taugourdeau S, Diedhiou A, Fassinou C, Bossoukpe M, Diatta O, N’Goran A, Auderbert A, Ndiaye O, Diouf AA, Tagesson T, Fensholt R, Faye E (2022) Estimating herbaceous aboveground biomass in Sahelian rangelands using Structure from Motion data collected on the ground and by UAV. Ecology and Evolution 12(5), 8867.
| Crossref | Google Scholar | PubMed |
Travers SK, Eldridge DJ, Val J, Oliver I (2019) Rabbits and livestock grazing alter the structure and composition of mid-storey plants in a wooded dryland. Agriculture, Ecosystems & Environment 277, 53-60.
| Crossref | Google Scholar |
Westoby M (1979) Elements of a theory of vegetation dynamics in arid rangelands. Israel Journal of Botany 28(3–4), 169-194.
| Crossref | Google Scholar |
Witt GB, Harrington RA, Page MJ (2009) Is ‘vegetation thickening’ occurring in Queensland’s mulga lands? A 50-year aerial photographic analysis. Australian Journal of Botany 57(7), 572-582.
| Crossref | Google Scholar |
Witt GB, Noël MV, Bird MI, Beeton RJS, Menzies NW (2011) Carbon sequestration and biodiversity restoration potential of semi-arid mulga lands of Australia interpreted from long-term grazing exclosures. Agriculture, Ecosystems & Environment 141(1–2), 108-118.
| Crossref | Google Scholar |
Zhang W, Qi J, Wan P, Wang H, Xie D, Wang X, Yan G (2016) An easy-to-use airborne lidar data filtering method based on cloth simulation. Remote Sensing 8(6), 501.
| Crossref | Google Scholar |