Non-compliance and under-performance in Australian human-induced regeneration projects
Andrew Macintosh A * , Megan C. Evans

A
B
C
D
E
Abstract
The ‘boom-and-bust’ nature of rangelands makes them ill-suited to nature-based solutions (NbS) involving carbon sequestration in vegetation and soils. The variability in these ecosystems makes it difficult to determine whether carbon stock changes are attributable to project activities, creating additionality risks. Low and variable rainfall also means carbon stock increases will often be impermanent, being susceptible to reversals in droughts, a risk magnified by climate change. The small potential for gains per unit area over vast regions makes it difficult to accurately measure carbon stock changes at low cost. This creates pressure to trade accuracy for simplicity in measurement approaches, increasing the risk of errors. Despite these risks, rangelands have been advanced as suitable for offset projects because of low opportunity cost and a perception they are extensively degraded. The most prominent example globally is human-induced regeneration (HIR) projects under the Australian carbon credit unit (ACCU) scheme, which are purporting to regenerate permanent even-aged native forests (≥20% canopy cover from trees ≥2 metres high) across millions of hectares of largely uncleared rangelands, predominantly by reducing grazing pressure. Previous research found limited forest regeneration in the credited areas of these projects, and that most of the observed changes in tree cover were attributable to factors other than the project activities. Here we extend this research by evaluating compliance of a sample of 116 HIR projects with regulatory requirements and their performance in increasing sequestration in regeneration. The results suggest most HIR projects are non-compliant with key regulatory requirements that are essential to project integrity, and have had minimal impact on woody vegetation cover in credited areas. The findings point to major administrative and governance failings in Australia’s carbon credit scheme, and a significant missed opportunity to restore biodiversity-rich woodlands and forests in previously cleared lands via legitimate carbon offset projects.
Keywords: carbon markets, climate change, environmental governance, forest carbon, generalised additive mixed models, nature-based solutions, rangeland management, revegetation.
Introduction
Rangelands are dynamic environments supporting extensive management systems, where impacts from people on ecosystems are entwined with complex natural drivers (Walker and Janssen 2002). Over 50% of global land area is comprised of rangelands, incorporating diverse ecosystems (deserts, grasslands, shrub steppe, savannas, and open woodlands) primarily dominated by native grasses, forbs, shrubs and scattered trees (Briske and Coppock 2023; Briske et al. 2024). Rangeland ecosystems have been advanced as an attractive location for nature-based solution (NbS) carbon offset projects through reforestation, afforestation, and other forms of vegetation and land management (Veldman et al. 2015; Bond et al. 2019; Vetter 2020; Parr et al. 2024), including in Australia (Garnaut 2008, 2011; Commonwealth Scientific and Industrial Research Organisation (CSIRO) 2009; Cockfield et al. 2019; Baynes et al. 2022; Bowen et al. 2022; Fitch et al. 2022). The appeal of rangelands stems partly from a perception they are extensively degraded, with depleted carbon stocks in vegetation and soils. For advocates, there is an opportunity to replenish the stocks through carbon markets by incentivising changes in land use and land management (Garnaut 2008, 2011; Commonwealth Scientific and Industrial Research Organisation (CSIRO) 2009; Cockfield et al. 2019; Bowen et al. 2022; Fitch et al. 2022). Reflecting the constraints that low and variable rainfall place on carbon sequestration, the gains per unit area are generally small in rangeland ecosystems but the costs are low, due to the availability of cheap land and low opportunity costs (i.e. small forgone profit per unit land area from existing land management) (Garnaut 2008, 2011; Commonwealth Scientific and Industrial Research Organisation (CSIRO) 2009; Emissions Reduction Assurance Committee 2019; Cockfield et al. 2019; Bowen et al. 2022; Fitch et al. 2022). The concept is superficially enticing, seemingly offering a way of lowering the costs of mitigating greenhouse gas emissions, while restoring degraded ecosystems and improving the financial sustainability of pastoral enterprises and associated communities.
The difficulty for proponents of rangeland NbS carbon offset projects is that the defining features of rangelands – extensive areas with ‘boom-and-bust’ ecologies dictated by low and variable rainfall – creates integrity problems for carbon offsets (Gifford and McIvor 2009; Booker et al. 2013; Macintosh et al. 2024a). The integrity of carbon offset projects depends on whether the credits issued to them represent real, additional and permanent abatement (Breidenich et al. 1998; Schneider and La Hoz Theuer 2019; Emissions Reduction Assurance Committee 2021; Stubbs et al. 2021). For rangeland NbS carbon offset projects, meeting these standards requires well-designed and well-administered rules that ensure issued credits represent genuine increases in carbon stocks (realness) that would not have occurred without the incentive provided by the scheme (additionality), and for the credited increases in carbon stocks to persist (permanence) (Gifford and McIvor 2009; Booker et al. 2013; Macintosh et al. 2024a).
The characteristics of rangeland ecosystems make it hard to design and administer such rules. There are three challenges. First, the natural variability of rangelands makes it difficult to determine whether, and the extent to which, any observed changes in carbon stocks in vegetation and soils are attributable to the project activities (Gifford and McIvor 2009; Booker et al. 2013; Carmody et al. 2022; Macintosh et al. 2024a). That is, it is hard to determine whether increases in carbon stocks are additional. Second, the variability of rangeland systems means that, even where projects can generate additional increases in carbon stocks, the increases will often be impermanent, being susceptible to loss in droughts; a risk magnified in many areas by climate change (Gifford and McIvor 2009; Booker et al. 2013; Macintosh et al. 2024a). Third, the vast areas and small potential for gains make it difficult to accurately measure carbon stock changes at low cost, creating pressures to rely on simplified (and less accurate) measurement approaches (Victor 2009; Commonwealth of Australia 2014; Emissions Reduction Assurance Committee 2019). Reliance on these simplified approaches increases the risk of measurement errors. These challenges render rangeland NbS projects high risk because they make it near impossible to ensure the credits issued to the projects represent real, additional and permanent abatement and, where this occurs, the credits will generally facilitate increases in greenhouse gas emissions (Booker et al. 2013).
The most prominent example globally of rangeland NbS carbon offset projects are those registered under the Australian carbon credit unit (ACCU) scheme’s human-induced regeneration of even-aged native forests (HIR) method (Commonwealth of Australia 2013, 2015a, 2016, 2018). HIR projects are meant to involve the regeneration of even-aged native forests through changes in land management on land that previously contained forest cover. Although the method was originally intended for application to cleared lands (Parliament of Australia 2013; Clean Energy Regulator 2014; Department of the Environment 2014), most HIR projects are in rangeland areas that have never been comprehensively cleared of native vegetation (Macintosh et al. 2024a). At the time of writing, there were 465 of these projects, covering 42 million hectares, with most claiming they are regenerating forests by reducing grazing pressure from livestock and feral animals (Clean Energy Regulator 2024). To date, these projects have received almost 44 million ACCUs (Clean Energy Regulator 2024), making them the largest pure removal carbon offset type, and the 5th largest NbS offset type, in the world by carbon credit issuances (Macintosh et al. 2024a).
The HIR method and HIR projects have been highly controversial due to concerns the projects are unlikely to result in the regeneration of native forests in uncleared landscapes, and that any observed increases in woody cover are more likely to be attributable to seasonal variability than the project activities (Butler et al. 2022; Carmody et al. 2022; Long and McDonald 2022). However, a review published in early 2023, led by Australia’s former Chief Scientist, Professor Ian Chubb (Chubb Review), concluded that the ‘HIR method is sound – it meets the [offsets integrity standards] and is administered by a robust regulatory framework’ (Chubb et al. 2022, at 21). Then in December 2023, the Regulator published a commissioned report on its administration of the HIR method that reached a similar conclusion:
The independent audit reports and the [Regulator] reviews provide strong assurance that projects are being managed as per the legislative requirements and that appropriate methods have been used by the proponents or their agents in classifying the [carbon estimation areas] and identifying changes in regeneration canopy cover (Brack 2023, at 11).
Likewise, although not focused exclusively on HIR projects, a performance audit published by the Australian National Audit Office (ANAO) in April 2024 found the ACCU method development processes, and the administration of the issuance, compliance and contracting of ACCUs, were ‘largely effective’ (Australian National Audit Office 2024, at 7).
The findings from these government reviews contrast sharply with peer-reviewed research that analysed changes in woody cover within 182 HIR projects and found limited evidence of forest regeneration in credited areas (Macintosh et al. 2024a). The research also found that changes in woody vegetation cover in the credited areas of the HIR projects largely mirrored changes in adjacent comparison areas, outside the projects, suggesting the observable changes were predominantly attributable to factors other than the project activities. These results raise questions about the extent of compliance with the requirements of the HIR method and the veracity of the findings from the government reviews concerning the robustness of the regulatory framework.
Here, we aim to further investigate this issue by assessing the likely extent of compliance of HIR projects with key regulatory requirements. We evaluate compliance using four regulatory tests derived from the HIR method and regulatory guidelines published by the Regulator (Commonwealth of Australia 2013, 2015a, 2016, 2018; Clean Energy Regulator 2019). The assessment includes statistical analyses to test whether projects achieved additional increases in canopy cover from woody vegetation between project commencement and 2023. We also assess potential over-crediting of carbon sequestration by comparing changes in canopy cover to the number of carbon credits issued to HIR projects.
The remainder of the paper is set out as follows. The next section provides background context on the HIR method and its requirements. The third section details the method used to conduct the compliance analysis. The fourth section presents the results. The fifth section discusses the implications of the results and the sixth section provides a conclusion.
HIR method requirements and background context
In contrast to other NbS initiatives in rangelands involving afforestation and reforestation (Briske et al. 2024), HIR projects do not involve tree planting or direct seeding. The HIR method requires forest regeneration to be induced from in situ seed and seedlings, root stock or lignotubers through changes in land management, with the goal of regenerating permanent even-aged native forests across the entirety of the areas that are credited (‘credited areas’) (Commonwealth of Australia 2013, 2015a, 2016, 2018; Parliament of Australia 2013). (Under the ACCU scheme, credited areas are formally known as ‘carbon estimation areas’.) Eligible project activities include managing non-native plants and stopping clearing of native plant regrowth but, in practice, the dominant project activity is reducing grazing pressure from livestock and feral animals (Clean Energy Regulator 2024).
Carbon sequestration in regeneration is not directly measured under the HIR method. Proponents of HIR projects are required to estimate sequestration using the Australian Government’s Full Carbon Accounting Model (FullCAM) (or a simplified version of FullCAM called the Reforestation Modelling Tool (RMT)) (Richards and Evans 2004; Commonwealth of Australia 2013, 2015a, 2016, 2018). FullCAM is a relatively simple mechanistic model that was designed for landscape-scale use to estimate emissions and removals associated with reforestation and deforestation in Australia’s national greenhouse gas accounts (Richards and Evans 2004). The calibration used to model sequestration in HIR projects assumes credited areas start with little woody biomass, and that regeneration progresses towards the maximum woody biomass potential under native vegetation across the entirety of the credited areas, following a sigmoidal growth curve with a prescribed age of maximum growth (Paul et al. 2015; Paul and Roxburgh 2020).
The use of this simple modelled approach to estimate sequestration was intended to promote uptake by lowering measurement costs for proponents (Emissions Reduction Assurance Committee 2019). However, the trade-off was that it creates a material risk of over-crediting, where credited sequestration exceeds actual sequestration. To address this risk, seven requirements were included in the HIR method to ensure credited areas have the capacity to support forest regeneration and that the modelled outputs are likely to be conservative reflections of reality (Table 1).
No. | Regulatory requirement | |
---|---|---|
R1 | Baseline forest cover rule: Land included in the credited areas must not have had ‘forest cover’ (areas of at least 0.2 ha with woody vegetation ≥2 m in height and ≥20% crown cover) at any time during the projects baseline period, defined as the 10-year period prior to the project commencement date or the date of the application for project registration.A | |
R2 | Forest potential rule: Land included in the credited areas must have ‘forest potential’, defined as land ≥0.2 ha with trees that, having regard to the location and characteristics of the land, are reasonably likely to reach ≥2 m in height and provide crown cover of ≥20%.B | |
R3 | Suppression rule: Regeneration on land included in the credited areas must have been prevented from achieving forest cover over the preceding 10-years (baseline period) by relevant ‘suppressors’ (clearing, grazing by livestock or feral animals, or weeds). | |
R4 | Project activity rule: Credited areas must consist only of land on which the project activity is being undertaken, defined as inducing the establishment of a native forest from in situ seed, lignotubers or root stock (coppice) sources by undertaking one or more human-assisted regeneration activities. | |
R5 | Regeneration additionality rule: Either regeneration must be a direct result of the project activities or, at the end of the baseline period, it must have been reasonable to expect it would be necessary to undertake one or more of the project activities on the land for it to attain forest cover.C | |
R6 | Even-aged regeneration rule: Credited areas must consist only of land that first exhibited regeneration at or around the same time. | |
R7 | Consistent vegetation and management rule: The regeneration must consist of a similar mix of native vegetation and be managed in a consistent manner. |
These requirements, particularly the suppression (R3), project activity (R4), regeneration additionality (R5) and even-aged regeneration (R6) rules, were meant to ensure that only cleared lands that did not contain pre-existing mature trees were included in the credited areas of HIR projects, which reflect the assumptions underpinning the FullCAM HIR calibration (Paul and Roxburgh 2020). This is clear from the official legal guide to the interpretation of the original HIR method (known as the Explanatory Statement), which states:
The Determination applies to projects in which land has been cleared of native vegetation and where regrowth has been suppressed for at least 10 years (Parliament of Australia 2013, at 1).
To eliminate doubt, the Explanatory Statement says the same thing in two other places, including in the section that governs the eligibility of land:
The activity must occur on areas of cleared land on which regrowth has been regularly suppressed but has the potential to grow if suppression activities ceased, or on cleared areas that abut existing vegetation (Parliament of Australia 2013, at 7).
A review of the HIR method conducted by the Emissions Reduction Assurance Committee (ERAC) in 2017–2019 did not investigate the extent to which uncleared lands with pre-existing mature trees had been included in credited areas, primarily because the Committee assumed projects were located on cleared lands (Emissions Reduction Assurance Committee 2019, at 13). However, the ERAC raised concerns about the absence of observed regeneration in credited areas and the risk of over-crediting due to the reliance on FullCAM (Emissions Reduction Assurance Committee 2019). To address these concerns, in late 2018, the Government introduced a new rule requiring 90% of credited areas to achieve forest cover within 15 years, assessed at 0.2-ha scale (R8: forest cover attainment rule) (Commonwealth of Australia 2015b, 2015c).
The forest cover attainment rule was informed by an empirical relationship between tree and debris biomass in forest regeneration and crown cover in the forest systems where most HIR projects are located, which suggests forest cover should be achieved in stands of even-aged regeneration when biomass reaches 7.2–11 tonnes of dry matter per hectare (equivalent to 13.2–20.2 t CO2 ha−1) (Larmour et al. 2018). For most HIR projects, this level of sequestration is likely to be achieved after 10–14 years of unhindered modelled regeneration (Emissions Reduction Assurance Committee 2019). Based on this, 15-years was chosen as a ‘generous’ cut-off point, when forest cover should have been achieved in most projects. However, preferential treatment was given to projects that were registered before the rule was initiated (Commonwealth of Australia 2015b, 2015c). For projects registered before 15 August 2018 (‘existing projects’), the 15-year timeline runs from the date the projects were registered (or the date the proponent modelled the commencement of regeneration if it is after the date of registration). For projects registered after this date (‘new projects’), the timeline runs from the date they model the commencement of regeneration. This means existing projects often have more than 20 years to meet their compliance checks under the forest attainment rule (R8).
In response to the introduction of the forest cover attainment rule and concerns raised about the initial stratification of credited areas (Emissions Reduction Assurance Committee 2019), in May 2019 the Regulator introduced new guidance on stratification and its interpretation of the method requirements (Clean Energy Regulator 2019) (Table 2).A In this context, stratification refers to the mapping of credited areas in accordance with the eligibility requirements of the method. The Regulator’s guidance requires the credited areas mapped by proponents to be broken into assessment cells of a prescribed size and for canopy cover to be assessed in each cell to ensure compliance with the initial stratification requirements and to ensure regeneration is progressing towards forest cover (known as the ‘initial stratification and regeneration gateway check requirements’). Initial stratification gateway checks are conducted in Years 1–5 and focus on whether the credited areas have been mapped in accordance with the method’s land eligibility requirements. This is followed by two regeneration gateway checks: the first in Years 6–10 and the second in Years 11–15. The requirements get progressively more stringent, and the cells get progressively smaller, as projects approach the forest cover assessment date. The logic behind the progressive tightening is that credited areas will be re-stratified at each gateway as necessary to remove non-compliant cells; to ensure only areas that satisfy the forest cover attainment rule remain at the applicable 15-year cut-off point.
Initial stratification gateway | |
---|---|
Timing: Before submitting the first offset report due for submission after publication of the guidelines in 2019. | |
What: ‘Initial stratification must demonstrate, using the appropriate spatial scale that carbon estimation areas (CEA) [credited areas] are on eligible land that does not contain pre-existing forest cover and only contains land with forest potential’. | |
Guideline requirements: Credited areas must: | |
Size of assessment cells: |
First regeneration gateway | |
---|---|
Timing: Once between the 6th and 10th year of the project. | |
What: Credited areas must ‘be assessed and evidence must be demonstrated to show an increase in forest cover at the mapping scale appropriate to the relevant year. Remote sensing approaches must use the latest imagery sourced for the relevant year the report is submitted in or within the period being reported on’. | |
Guideline requirements: Credited areas covered in offsets reports submitted from the 6th to 10th project year must satisfy one of three tests: |
Second regeneration gateway | |
---|---|
Timing: Once every 5 years from the 10th year of the project. | |
What: Credited areas must ‘be assessed and evidence must be demonstrated to show an increase in forest cover at the mapping scale appropriate to the relevant year. Remote sensing approaches must use the latest imagery sourced for the relevant year the report is submitted in or within the period being reported on’. | |
Guideline requirements: Credited areas covered in offsets reports submitted from the 10th project year must satisfy one of two tests: |
Source: Clean Energy Regulator (2019).
Methods
Sample of HIR projects
As of 30 June 2024, there were 465 registered HIR projects (Clean Energy Regulator 2024). Credited area location data were available for 244 HIR projects, following changes to the law in April 2023 that allowed these data to be publicly available for the first time. The projects we analysed were confined to those registered between 2013 when the HIR method commenced and the end of 2016 (n = 130). Seven of these projects did not have published credited area data, and the credited area data of a further six was corrupted. One other project was completely encircled by other HIR projects, which impeded the analysis of compliance and enforcement, leaving a total sample of 116 projects (Fig. 1).
The extent of active HIR project areas (blue), with the subset of 116 projects analysed here (pink). Grey shading indicates the extent of rangelands defined by the Australian Collaborative Rangeland Information System. Source: Department of Climate Change, Energy, the Environment and Water (2021), Clean Energy Regulator (2024).
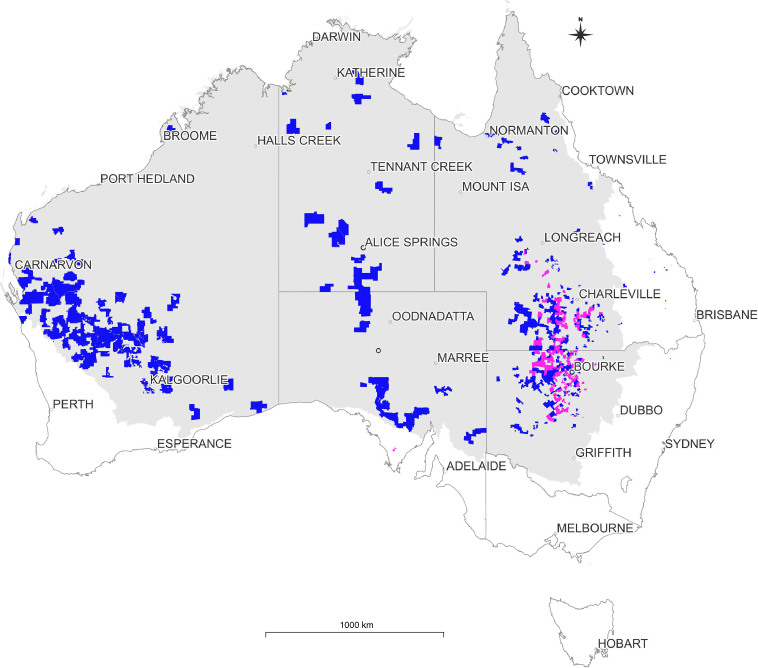
We confined the project sample to projects registered prior to 2017 for two reasons. First, given the age of these projects, it is reasonable to assume that, by 2023 (the latest year in the analysis), they had all been required to demonstrate compliance with both the initial stratification and first regeneration requirements. Second, most projects registered prior to April 2016 had backdated project commencement dates and regeneration modelling commencement dates (Clean Energy Regulator 2023a, 2024; Macintosh et al. 2023). Consequently, confining the sample to projects registered prior to 2017 ensured the sample was restricted to projects that, based on their credited sequestration, should have achieved significant forest regeneration (Larmour et al. 2018).
The majority of the projects in the sample were registered in 2015 (n = 85) and 2016 (n = 27), with only a small number registered in 2013 and 2014 (n = 1 and n = 3, respectively). Most projects were in western New South Wales (n = 74), with the remainder in western Queensland (n = 41) and a single project in South Australia, which is also the only HIR project registered in 2013. The credited areas of the project in the sample cover 1.66 million ha, with 935,752 ha (56%) in New South Wales, 717,075 ha (43%) in Queensland and 3400 ha (0.2%) in South Australia (Clean Energy Regulator 2024).
Tests for regulatory compliance
We evaluated compliance with key requirements of the HIR method by dividing the credited areas of sampled projects into 100 ha (1000 m × 1000 m) cells and then applying four regulatory tests derived from the HIR method and the Regulator’s initial stratification and regeneration gateway check requirements (Table 3).
Test | Description | Applicable rule | |
---|---|---|---|
Cleared area test (T1) | Cells deemed compliant if ≥80% of credited area in cell assessed as having previously been comprehensively cleared. | Assesses compliance with the requirement to ensure credited areas consist only of cleared lands without pre-existing mature trees, which is derived from the suppression (R3), project activity (R4), regeneration additionality (R5) and even-aged regeneration (R6) rules. The 80% threshold was used to account for potential mapping inaccuracies. | |
Baseline forest test (T2) | Cells deemed compliant if, in the credited area of the cells, the 3-year average canopy cover from woody vegetation ≥2 m in height was <20% for all 3-year periods from 2001 to 2003 until the date of project registration. A | Assesses compliance with the baseline forest cover rule (R1), although with compliance assessed at the 100-ha rather than 0.2-ha scale. | |
First regeneration gateway test (T3) | Cells deemed compliant if, in the credited area of the cells, either: | Aligns with the first two limbs of the test used in the Regulator’s first regeneration gateway check, which is supposed to assess compliance with the forest potential rule (R2). B | |
T3-a: canopy cover from woody vegetation ≥2 m in height in 2023 was ≥7.5%. | |||
T3-b: canopy cover from woody vegetation ≥2 m in height in 2023 was ≥5% more than: (a) the lowest levels recorded between 2010 and the date of project registration for projects registered prior to 23 March 2016 (when backdating was allowed); or (b) the levels recorded in the year of project registration for projects registered on or after 23 March 2016 (T3-b). | |||
Additional cover test (T4) | Statistical analysis to assess whether, and the extent to which, the projects have resulted in increases in canopy cover from woody vegetation ≥2 m in height that would not have otherwise occurred. | Assesses compliance with the regeneration additionality (R5) rule, while also providing insights on compliance with the suppression (R3) and project activity (R4) rules. |
Cleared land data and analysis
We estimated the proportion of the credited area in the cells that had previously been comprehensively cleared (T1) using one of two datasets. For projects located in Queensland and South Australia, we used a national 100 m grid of major vegetation groups from the National Vegetation Information System (Version 6.0 released in 2020, Department of Climate Change, Energy, the Environment and Water 2023a). We deemed grid cells assigned Major Vegetation Group 25 (cleared) or 29 (regrowth) to be comprehensively cleared land. For projects in New South Wales, we used the NSW Statewide Vegetation Type data, generalised from a 5 m to a 100 m grid. We deemed cells not classified as a native Plant Community Type, or classified as one of several native Plant Community Types derived by clearing, to be previously comprehensively cleared (NSW Department of Climate Change, Energy, the Environment and Water 2023).
According to these classifications, areas that have substantially regrown from previous clearing are generally not identified as previously cleared. In practice, the period of time required for a comprehensively cleared area to recover sufficiently to be remapped as native vegetation, or ‘remnant vegetation’, is several decades (Neldner et al. 2023). As a result, although the mapping data used in this analysis were released in 2020, there is little risk that areas starting from a comprehensively cleared state would have been remapped as native vegetation as a result of HIR project establishment. However, areas that have experienced more subtle forms of clearing such as selective fodder harvesting or thinning (Witt et al. 2009; Witt 2013) are not generally classified as previously comprehensively cleared.
Woody vegetation cover dataset and analysis
We analysed canopy cover from woody vegetation in the credited areas using the Woody Cover Fraction (WCF) dataset (Liao et al. 2020). WCF is a Landsat-derived product that represents the fraction of canopy from woody vegetation ≥2 m height, incorporating both woody and leaf canopy components. Cover and height estimates derived from airborne LiDAR and inventory-based biomass estimates were used to train a Random Forest algorithm to derive WCF, resulting in a high accuracy for cover (estimation error of 0.07). Data resolution of WCF is 25 m across the Australian continent from 1988 onwards.
WCF canopy cover estimates equate to foliage projective cover (FPC), which is the proportion of ground area covered by the vertical projection of the foliage of tree crowns. To convert these estimates to crown projective cover (CPC) (the proportion of ground area covered by the vertical projection of tree crowns), we used the parameterised equation from Fisher et al. (2018):
We chose WCF for the analysis for three reasons. First, WCF has a low estimation error and was calibrated and validated using a large dataset that includes data from sites in the regions containing HIR projects. Second, WCF was used in the 2023 verification report of the Regulator’s administration of the HIR method for the purposes of assessing compliance with the initial stratification and gateway checks, demonstrating its acceptance by the Regulator and other relevant stakeholders as an appropriate remote sensing product for these purposes (Brack 2023). Third, using the WCF for these purposes provides a way of cross-checking the results from Macintosh et al. (2024a), as that study relied on the National Forest & Sparse Woody Vegetation Database (Department of Climate Change, Energy, the Environment and Water 2023b).
Estimated credited sequestration
Under the ACCU scheme, projects are not required to publish data on the conduct and outcomes of projects, including the offset reports that trigger credit issuances, audit reports and data submitted as part of gateway checks. This hinders the assessment of potential mismatches between credited and actual sequestration. To address this, we calculated credited sequestration from credit issuances and then used the known relationship between biomass in forest regeneration and crown cover in the forest systems where HIR projects are located to assess potential over-crediting (Larmour et al. 2018). This relationship suggests forest cover should be achieved in stands of even-aged regeneration when biomass reaches the equivalent of 13.2–20.2 t CO2 ha−1 (Larmour et al. 2018). Accordingly, where credited sequestration exceeds these levels and forest cover has not been achieved, it suggests the project is likely to have been over-credited.
Following Macintosh et al. (2024a), we used a conservative approach to estimate per hectare credited sequestration in our sample of HIR projects using data from the Emission Reduction Fund Project Register (Clean Energy Regulator 2024). We adjusted total credit issuances to each project to 30 June 2023 to account for relevant discounts (5% risk of reversal buffer and a 20% permanence period discount for projects with 25-year permanence periods). We made a uniform and conservative 0.5% deduction to account for fossil fuel use, based on an Australian Government analysis (Emissions Reduction Assurance Committee 2019). The resulting estimates were converted from CO2 to C using the atomic mass ratio, 44/12.
Additional cover test (T4) analysis
We undertook the T4 analysis by fitting generalised additive mixed models (GAMMs) (Wood 2017) implemented in the ‘mgcv’ package (Wood 2011) in the statistical program R (R Core Team 2024). Using GAMMs allowed us to account for non-linear changes in canopy cover over time across all projects in response to varying climatic conditions, whilst testing how cover changed differentially between project and non-project areas. First, we matched project cells with comparison cells from 3 km wide buffer areas around the boundaries of each project, excluding areas that intersected with other HIR projects. We randomly selected two hundred 100 ha potential comparison cells for each project from these buffer areas. We then matched each project cell to a comparison cell by computing the Euclidean distances of canopy cover estimates between each project cell and potential comparison cells, only considering the years prior to project commencement, starting from 1990. Data on project commencement were available for 41 of the 116 projects (Clean Energy Regulator 2024). For the remaining projects, project commencement was assumed to be 3 years prior to the date of project registration (Clean Energy Regulator 2024). We allowed comparison cells to be matched to multiple project cells. With a transformation of CPC as our response variable, we constructed GAMMs, testing the linear and non-linear effects of time, the linear effects of project cells compared to comparison cells, and the interaction effects of the change over time and cell type (project vs comparison). We used this interaction term as the test of whether there had been a difference in canopy cover in project cells compared to comparison cells over time. Our model formula was:
Cwhere log(1 + Cover)t,c is the log-transformed canopy cover at year t in cell c. Before transformation, we added a constant to CPC to avoid log transforming values of zero. We log transformed this proportional response to fit the assumption of a Gaussian error distribution in the model better. β0 is the intercept and f1(Yt) is the smooth (nonlinear) function of year (Yt). β0 to β3 are the coefficients of the linear effects of year (Yt), cell type (CC), and their interaction, respectively. f2(latc, lonc) is a smooth interaction function for latitude and longitude, using a Gaussian Process. We included f2(latc, lonc) to account for spatial autocorrelation between all cells. We also included random intercept effects for each cell ID (b1,CellID[c]), to account for correlation between repeated measures at each cell over time. We scaled all continuous predictor variables to have a mean of zero and standard deviation of one (Gelman 2008).
Given the computational challenges of fitting the model to the entire dataset, we adopted a repeated sampling approach. For each of 1000 repeats, we fitted a model using a random sample of 12 cells within each project (six project cells, six comparison cells). This resulted in samples of between approximately 15,000 and 15,600 per repeat. We used the mean values across these iterations as our estimated coefficients and calculated the standard errors based on the variance of the sampled estimates. This repeated sampling method allowed us to account for spatial and temporal dependencies between cells effectively while reducing computational demands.
We also conducted tests of T4 at the individual project level for all projects. We used the same model structure as above. We fitted a model for each project separately, this time using all cell values within the project. To account for the increased probability of false positives when performing these multiple comparisons, we applied the Benjamini–Hochberg correction to control the false discovery rate (Benjamini and Hochberg 1995).
Results
Cleared area test (T1)
Under the cleared area test (T1), cells were deemed compliant if ≥80% of the credited area in the cell is assessed as having previously been comprehensively cleared. Of the 35,362 cells assessed across the 116 projects, only 1692 (5%) satisfied the conditions of T1 (Supplementary Tables S1 and S2). There were different rates of compliance depending on the date of project registration and the jurisdictions in which the projects are located (Fig. 2). Projects registered in New South Wales had the highest rates of non-compliance (99% of cells were non-compliant), followed by those in Queensland (91%) and then the single project in South Australia (78%). Almost 100% of cells in projects registered in 2014 were non-compliant, while 95% of cells in projects registered in 2015 and 2016 were non-compliant (Table S2).
Baseline forest test compliance (T2)
Under the baseline forest test (T2), cells were deemed compliant if, in the credited area of the cells, the 3-year average canopy cover from woody vegetation ≥2 m in height was <20% for all 3-year periods from 2001 to 2003 until the date of project registration. Of the 35,362 cells we assessed, 25,051 (71%) satisfied the conditions of T2 (Tables S1, S2). Compliance was lower in projects in New South Wales relative to those in Queensland, while the one project in South Australia was 100% compliant (Fig. 3). We found that rates of compliance increased progressively over project registration years 2014 (64%), 2015 (68%) and 2016 (77%).
Percentage of cells within each project that were compliant with the baseline forest test (T2), by registration year and jurisdiction. Length of whiskers indicates 1.5 × interquartile range.
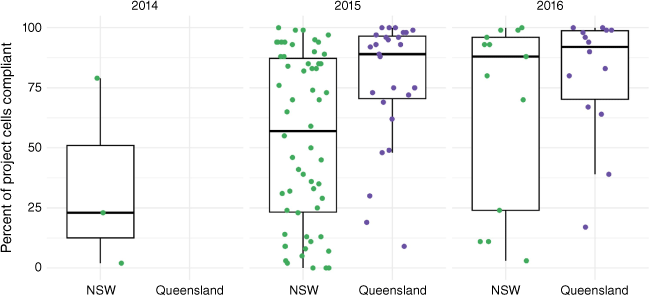
A substantial proportion of projects had high to very high rates of non-compliance with T2. For example, ≥50% of the cells in 41 projects did not satisfy the requirements of T2 (35% of the sample) (Supplementary Fig. S1). Thirteen projects (11%) had T2 non-compliance rates ≥90%.
First regeneration gateway test compliance (T3-a and T3-b)
We deemed cells to be compliant with the first regeneration gateway test (T3) if they satisfied either T3-a (canopy cover ≥7.5% in 2023) or T3-b (≥5% increase in canopy cover). Cells were deemed compliant with T3-a if, in the credited area of the cells, canopy cover from woody vegetation ≥2 m in height in 2023 was ≥7.5%. Cells were deemed compliant with T3-b if, in the credited area of the cells, canopy cover from woody vegetation ≥2 m in height in 2023 was ≥5% more than: (i) the lowest levels recorded between 2010 and the date of project registration for projects registered prior to 23 March 2016 (when backdating was allowed); or (ii) the levels recorded in the year of project registration for projects registered on or after 23 March 2016.
Of the 35,362 assessed cells, 18,987 (54%) satisfied the conditions of T3-a, with the remaining 16,375 (46%) being non-compliant (Tables S3, S4). The rates of non-compliance with T3-a were highest in South Australia (71%), then Queensland (55%), then New South Wales (39%). The rates of non-compliance with T3-a were generally higher in projects that were registered more recently: the proportion of non-compliant cells was 12% for 2014 projects, 46% for 2015 projects, and 51% for 2016 projects, the inverse of the trends with T2. 71% of the cells in the single 2013 project were non-compliant with T3-a.
The results for T3-b were almost the inverse of those for T3-a: 16,053 (45%) of the assessed cells satisfied the conditions of T3-b, with the remaining 19,309 (55%) cells being non-compliant (Tables S3, S4). The rates of non-compliance were highest in South Australia (76%), then Queensland (59%), then New South Wales (51%). The rates of non-compliance with T3-b were the highest in the single 2013 project (76%) and projects registered in 2016 (66%). Twenty-one per cent (21%) of the cells from the three projects registered in 2014 were non-compliant. Fifty-one per cent (51%) of the cells from projects in 2015 did not comply with the cover change requirements of T3-b.
Consistent with the standalone results for T3-a and T3-b, we found high rates of non-compliance with the combined requirements of the first regeneration gateway check: 45% of assessed cells did not satisfy either T3-a or T3-b (Table S2, Fig. 4). The rate of non-compliance was highest in the single project in South Australia (69% of cells), followed by the Queensland projects (53% of cells). Thirty-eight per cent (38%) of the cells in projects registered in New South Wales did not comply with the requirements of either T3-a or T3-b. In terms of the year of registration, non-compliance was highest in the single 2013 project (69%), then the projects registered in 2016 (51%) and 2015 (44%). The three projects registered in 2014 had the highest compliance rate, with 88% of cells compliant.
Additional cover test compliance (T4)
The data show canopy cover in the credited areas mostly fluctuating between 0 and 30%, with the peaks corresponding to wet periods (particularly the La Niña events in 1998–2001, 2010–2012, and 2020–2023) and the troughs corresponding to dry periods (particularly the El Niño events 1991–1992, 2002–2003, 2006–2007, 2015–2016, and 2017–2019) (Fig. 5). Individual project trends are provided in the Supplementary Fig. S2. Given the extent of tree cover across the credited areas when the projects started, it is likely that most of the observed changes in canopy cover are attributable to changes in the size and density of the canopy in pre-existing trees in response to dry and wet conditions rather than repeated cycles of widespread tree death and regeneration (Crowley and Murphy 2023). If the projects were compliant with the requirements of the method and performing in accordance to how they have been credited, canopy cover in each 100-ha cell within credited areas should have started near zero and increased to 20% or more over the period 2010–2023. The effects of the project activities would also be evident in a substantial departure of canopy cover in the project cells from the trends in the corresponding matched comparison cells.
Average canopy cover in credited areas of projects, 1990 to 2023, by jurisdiction (grey shows standard error of weighted mean across the project cells). Dashed black line shows expected CPC if regeneration was occurring as credited on previously cleared land (as required by the HIR method), based on modelled regeneration starting in 2010, using credited area average maximum biomass under native vegetation (M) for projects in each jurisdiction, converted to CPC using parameterised equation from Larmour et al. (2018).
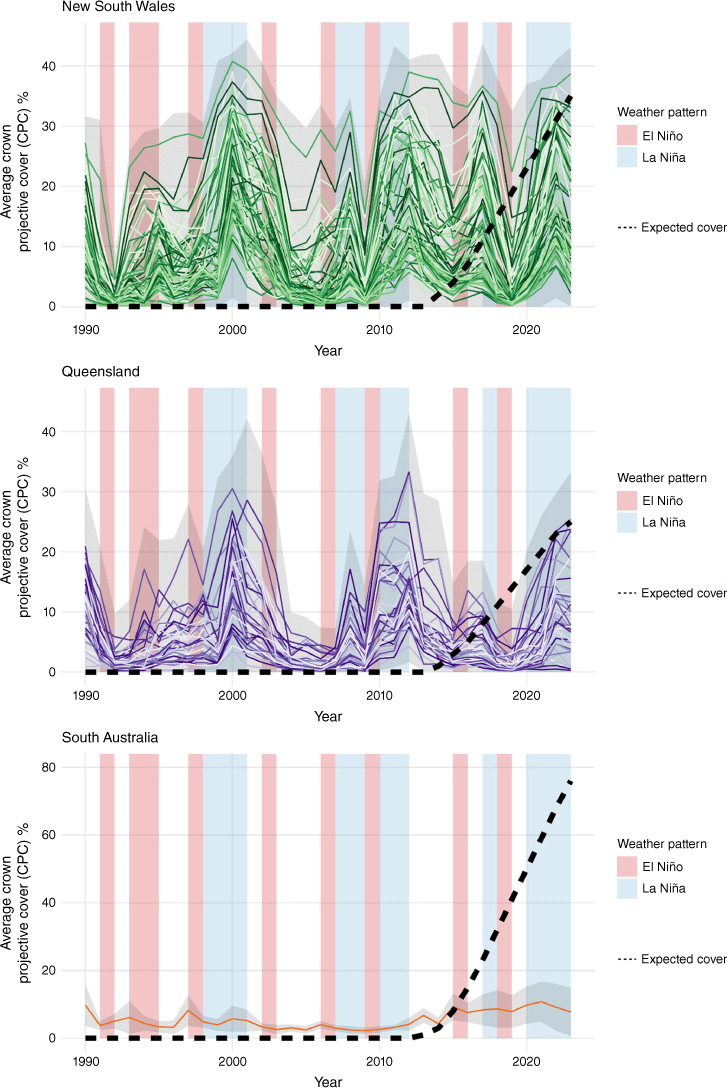
The GAMMs we fitted for the overall model on all projects revealed a small but significant average linear increase in additional canopy cover in project cells relative to comparison cells over the period of 2012–2023 (Coefficient = 0.021, s.e. = 0.006, 95% CI = 0.008, 0.034) (Fig. 6, Table 4). For the project-level GAMMs, 49 projects exhibited significant positive linear differences in CPC compared to comparison areas from project commencement to 2023, 36 projects exhibited significant negative linear differences in CPC compared to comparison areas, and 31 projects exhibited no significant linear difference (Fig. 7, see Tables S5, S6 for all model coefficients. Supplementary Fig. S3 provides model predictions for CPC for six projects within the study to illustrate the range and magnitude of modelled differences).
Model prediction plot representing the mean and standard errors for Crown Projective Cover in comparison and project cells over time from 2012 to 2023. Plot based aggregated estimates and standard errors from 1000 models fitted on random samples.
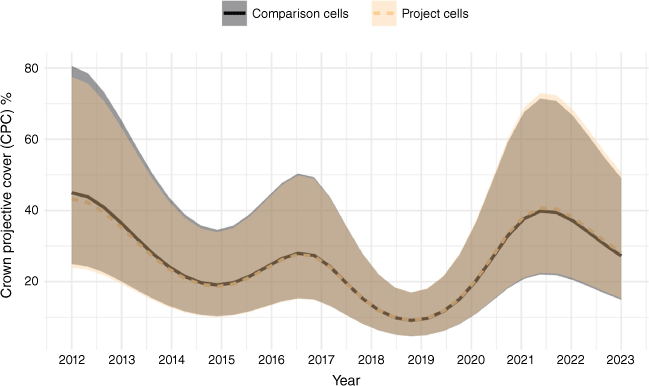
Parametric component | |||||
---|---|---|---|---|---|
Term | Estimate | s.e. | Lower 95% CI | Upper 95% CI | |
Intercept | 2.158 | 0.027 | 2.116 | 2.251 | |
Year (Y) | 0.534 | 0.071 | 0.373 | 0.687 | |
Project cells (P) | −0.003 | 0.014 | −0.035 | 0.027 | |
Year × Cell type (Y:P) | 0.021 | 0.006 | 0.008 | 0.034 |
Smooth component | |||
---|---|---|---|
Term | EDF | Ref EDF | |
Year (Y) | 7.981 | 8.000 | |
Latitude (lat), Longitude (lon) | 24.089 | 24.159 | |
CellID | 1238.940 | 1312.759 |
Cell type is the effect of project cells compared to comparison cells. The term (Y:C) represents the statistical test for changes in the differences between project and buffer cells over time. s.e., standard error; EDF, estimated degrees of freedom; Ref EDF, reference estimated degrees of freedom.
Project-level model estimates for the mean effects of project cells compared to comparison cells (Project cells) and the interaction of this and time (Year:Project cells). Estimates to the left of the dashed lines indicate negative effects and estimates to the right of the dashed lines indicate positive effects. Larger points indicate that the corrected P values for the estimate were significant (<0.05). Error bars represent 95% confidence intervals. Pink indicates positive effects, black indicates negative effects. For example, effects to the right of the dashed line in the Year: Project cells column indicate that project cells have increased in CPC over time compared to comparison cells. See Supplementary Fig. S3 for CPC predictions over time for a selection of these models.
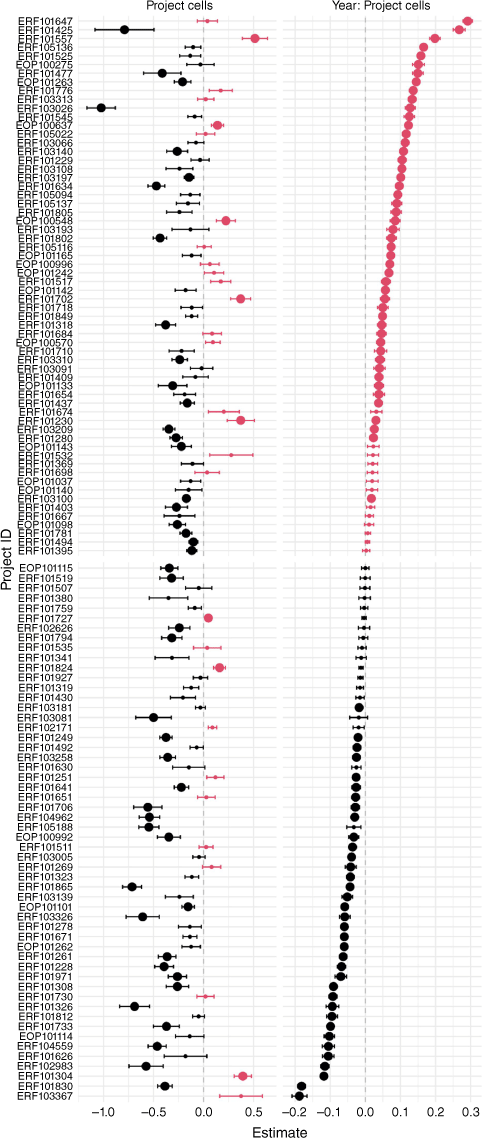
The results from this additional cover analysis relate only to the difference in change in cover over time between project cells and comparison cells. A positive significant effect size means that there is a statistically significant higher slope for project cells relative to comparison cells. However, statistical significance does not always correspond to a meaningful difference commensurate with crediting: a significant positive difference may be small on a practical level and does not mean a project is performing as expected or in accordance with how it has been credited. Projects are credited for absolute increases in cover, not additional cover relative to a comparison area. A positive model effect size could even correspond to a decrease in cover over time for a project. If both project and comparison cells had decreased cover over time, but project cells decreased less than comparison cells, that would correspond to a positive effect size in the additional cover model.
To assess the materiality and potential causes of the detected differences, we subjected the 49 projects with positive linear differences to further evaluation. There was no relationship between the detected difference in change between project cells and comparison cells and the observed change in canopy cover between project commencement and 2023 (Fig. S4). The average canopy cover in the credited areas of these projects in 2023 was only slightly above the average from the whole sample (mean 14.2% (s.d. 8.7%) vs 13.3% (9.3%)), as was the average canopy cover change between project commencement and 2023 (7.8% (s.d. 5.4%) vs 6.6% (s.d. 5.0%)).
Thirty-seven (37) of the 49 projects (76%) had credited sequestration ≥13.2 t CO2 ha−1, indicating that canopy cover from the credited regeneration (excluding cover from pre-existing mature vegetation) should be near or above 20%. Yet only 4 of the 37 projects (11%), and 5 of the 49 projects (10%), experienced a ≥15% increase in canopy cover between project commencement and 2023 (Fig. S5).
Most of the credited area of these 49 projects has not previously been comprehensively cleared, with 92% of the cells from these projects failing the cleared area test. Canopy cover at commencement in the 49 projects (mean 6.8%, s.d. 5.2%) was effectively the same as in the whole sample (mean 7.1%, s.d. 6.1%). The data provided no obvious explanations for the positive detected difference in these projects, indicating a need for further investigation.
Credited sequestration and canopy cover
The average credited sequestration of the full sample at 30 June 2023 was 22.1 t CO2 ha−1 (median 17.3 t CO2 ha−1, s.d. 17.1 t CO2 ha−1). Eighty-seven of the projects (75%) had credited sequestration ≥13.2 t CO2 ha−1 (mean 26.2 t CO2 ha−1, median 21.8 t CO2 ha−1, s.d. 17.9 t CO2 ha−1) (Clean Energy Regulator 2024). This credited sequestration suggests these 87 projects should have had near 100% forest cover across their credited areas at the time of the analysis solely from the regeneration induced by the projects (Larmour et al. 2018). When the cover from pre-existing trees and shrubs is added, the canopy cover in the credited areas of these projects should generally be well above the 20% threshold for forest.
Seven of the 87 projects (8%) with credited sequestration ≥13.2 t CO2 ha−1 experienced a ≥15% increase in canopy cover between project commencement and 2023, with only one project (1%) experiencing a ≥20% increase in canopy cover over this period. The average change in canopy cover from project commencement to 2023 in the credited areas of these projects was 7.4% (median 7.1%, s.d. 4.6%). The average change in canopy cover from project commencement to 2023 across all 116 projects was 6.6% (median 5.9%, s.d. 5.0%) (Fig. S6). Fifty-three projects (46%) experienced a <5% increase in average canopy cover over this period, with nine projects (8%) experiencing a decline in average cover.
Reflecting the relatively small detected change in cover, only 27 of the 87 projects (31%) had an average canopy cover in 2023 ≥20%, and only 28% of the cells of these 87 projects (7069 of 25,403) had an average canopy cover in 2023 ≥20%. Average canopy cover of the 87 projects over the period 2020–2023 was 14.8% (median 11.7%, s.d. 9.3%). Given 2023 coincided with the last year of a rare 3-year, triple-dip wet La Niña event, we would expect detected canopy cover to be high in that year as a consequence of the seasonal conditions.
Discussion
Inappropriate targeting of rangeland ecosystems for forest-based nature-based solutions (NbS) is of global concern (Veldman et al. 2015; Kumar et al. 2020; Briske et al. 2024; Parr et al. 2024). Afforestation of naturally open ecosystems containing little or sparse woody vegetation can have detrimental consequences for biodiversity and for local communities who rely on the continued provision of ecosystem services (Fleischman et al. 2020; Vetter 2020). Unlike the large-scale afforestation programs occurring in the rangelands of Africa, Asia and South America (Vetter 2020; Briske et al. 2024), HIR projects do not involve any direct tree planting. However, HIR projects still rely on the misconception that rangelands are degraded forests, with critical implications for the climate and biodiversity.
The key risk for the climate is that the carbon credits issued to projects do not represent real, additional, or permanent abatement, which will typically result in increased carbon emissions (Booker et al. 2013). This risk is a product of the fact carbon credits are a form of emissions allowance that are used by polluters to enable increases in emissions above regulatory limits. Where the credits do not represent real, additional, or permanent abatement, they facilitate increases in emissions from heavy emitting facilities, but there is no offsetting emission reduction to compensate for the increase (Macintosh et al. 2024a).
For biodiversity, the primary damage done by low integrity HIR projects has been to crowd out high-integrity NbS projects, particularly those involving the restoration of cleared native woodlands and forests (Evans et al. 2015; Evans 2016). To date, native reforestation projects have only accounted for 2% of ACCU issuances (Clean Energy Regulator 2024). The absence of these projects is primarily due to their inability to compete with low integrity projects, which put downward pressure on credit prices.
Consistent with the findings from Macintosh et al. (2024a), our new analysis reported here suggests HIR projects have had little influence on observed levels of woody vegetation cover. More than half (55%) of the assessed cells failed the second limb of the first generation gateway test (T3-b), meaning average canopy cover across the credited area component of these cells was relatively unchanged (<5% increase) between project commencement and 2023. Remarkably, despite 2021–2023 being a particularly wet period, the average change in canopy cover from project commencement to 2023 was 6.6% (median 5.9%, s.d. 5.0%) and only 45% of assessed cells experienced a ≥5% increase in canopy cover over this period.
The additional cover test analysis (T4) found that, according to the model fit on all projects, project cells experienced a small positive additional linear increase in canopy cover on average relative to comparison cells (effect size 95% CI: 0.008–0.034). Although 42% of projects exhibited statistically significant positive linear differences over time relative to comparison cell change, many projects exhibited either no significant difference (27%) or statistically significant negative differences (31%). Further, the change in canopy cover in the projects that exhibited significant positive differences was relatively small (mean 7.8%, s.d. 5.4%), and the average canopy cover in these projects in 2023 was still relatively low (mean 14.2%, s.d. 8.7%). Based on the levels of credited sequestration, canopy cover from new regeneration alone should have been near or above 20% by 2023 in 76% (37) of these projects. Yet only four of these projects (11%) experienced a ≥15% increase in canopy cover between project commencement and 2023, and only one experienced a ≥20% increase in canopy cover.
The biophysical explanation for the small observable project effect and relative absence of regeneration is simple. Because the HIR projects are almost exclusively located in uncleared arid and semi-arid rangeland areas, their capacity to increase sequestration in these areas through natural regeneration of woody vegetation is likely to be limited (Richards and Brack 2004; Gifford and McIvor 2009; Sankaran et al. 2013). The areas are likely to be at or near their carrying capacity for woody vegetation, meaning any changes in tree cover are most likely to be attributable to seasonal variability in rainfall (Gifford and McIvor 2009; Eldridge and Sala 2023).
The only way HIR projects could regenerate forests in these areas is if grazing had previously significantly reduced tree cover. Grazing in Australia’s uncleared rangelands has generally not had this effect. Vegetation structure in uncleared parts of Australia’s eastern rangelands is broadly similar to that described by early explorers (Fensham 2008; Silcock et al. 2013). If anything, tree cover has tended to increase in grazed rangelands, at least since the mid twentieth century (Burrows 1973; Cunningham and Walker 1973; Moore 1973; Harrington 1979a; Booth and Barker 1981; Hodgkinson and Harrington 1985; McKeon et al. 2004; Witt et al. 2009; Witt 2013). The literature suggests grazing can have local, short-term negative effects on the recruitment and growth of trees and shrubs in rangelands (Harrington 1979b; Brown 1985). However, over larger scales of space and time, it generally does not cause significant reductions in tree cover and its influence on woody plants is minimal compared to the effect of variable rainfall (Lett and Knapp 2005; Eldridge et al. 2011; Fensham et al. 2011; Anadón et al. 2014; Archer and Predick 2014). Due to this, the presumption of significant suppression of forest cover in grazed rangelands prior to HIR project establishment, so that project activities are necessary for forest cover to develop, is extremely weak. It is therefore unlikely that projects would induce significant additional regeneration commensurate with how they are credited. The data suggest they are not. While some projects appear to have had a positive additional effect on canopy cover, even for these projects, the effects are small relative to the levels of credited sequestration and there is limited evidence of material regeneration. At the aggregate level, across all projects in the sample, the data suggest the projects have had only a small effect on canopy cover, with most of the change being attributable to seasonal variability in rainfall.
Inaccuracy in the WCF dataset is a possible explanation for these results. However, several factors suggest this is unlikely. First, the WCF dataset has relatively high accuracy, with an estimation error of 0.07 for cover and no material bias (Liao et al. 2020). Second, the Random Forest algorithm used to generate the WCF dataset was trained using a large number of biomass inventory sites in the rangelands in New South Wales and Queensland in regions where the HIR projects in the sample are located (Liao et al. 2020, at 5). Third, given the absence of material bias, error in the dataset cannot explain the small linear difference in canopy cover in project cells relative to comparison cells. Fourth, although satellite-based estimates of woody cover can be biased toward overestimating cover when actual tree cover is low (<10–20% FPC) (Armston et al. 2009; Liao et al. 2020), this would tend to make projects appear to have more cover, rather than less. In this case, if the projects were performing in accordance with how they have been credited, average canopy cover across the credited area of the projects in 2023 should have been ~20–60% (Fig. 8), where bias tends to be lowest. Based on the levels of credited sequestration, and the relationship between live biomass in regeneration and canopy cover from Larmour et al. (2018), the average minimum expected level of canopy cover across the projects in 2023 was 30.4% (s.d. 11.5%). Yet average canopy cover in the credited area of the projects in 2023 was 13.3% (s.d. 9.3%), substantially below the expected minimum. The tendency for WCF to over-estimate cover at low levels may have acted to reduce the detected canopy cover change from project commencement to 2023. However, based on crediting, canopy cover increases from new regeneration alone across the projects should have been at least 23.4% (s.d. 8.3%). The gap between observed and credited canopy cover change appears to be too large to be explained by known issues with satellite-based canopy cover estimates.
Average canopy cover in credited areas of projects at commencement and 2023, compared to minimum expected canopy cover in 2023. Minimum expected canopy cover calculated as sum of canopy cover at commencement and canopy cover associated with credited regeneration. Cover from credited regeneration calculated using relationship from Larmour et al. (2018), up to 10 t C per ha of live above- and below-ground biomass. Live biomass proportion estimated using representative project plots based on average maximum biomass under native vegetation from credited area of each project.
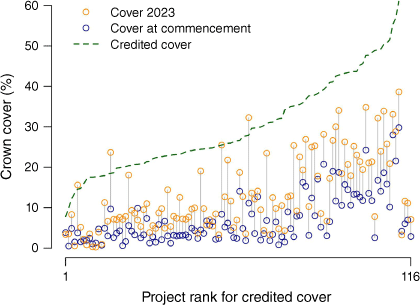
Apart from the lack of regeneration, the results also suggest there are very high levels of non-compliance in HIR projects with key regulatory requirements (Commonwealth of Australia 2013, 2015a, 2016, 2018; Clean Energy Regulator 2019). Most (95%) of the 35,362 assessed 100 ha cells did not satisfy the requirements of the cleared area test (T1), indicating widespread non-compliance with the requirement to ensure credited areas consist only of cleared lands without pre-existing mature trees. The Regulator and the relevant Australian Government Department have recently claimed the HIR method does not require credited areas to be located on cleared lands (Clean Energy Regulator & Department of Climate Change, Energy, the Environment and Water 2023). This claim is inconsistent with the provisions of the method and is directly contradicted by the Explanatory Statement, which, by law, is intended to assist in the interpretation of the method (Legislation Act 2003 (Cth), s 13; Acts Interpretation Act 1901 (Cth), s 15AB). The claim the HIR method does not require lands included in credited areas to have been cleared also runs counter to materials published by the Regulator and Department in 2013 and 2014 (Clean Energy Regulator 2014; Department of the Environment 2014).
A significant proportion (29%) of assessed cells did not satisfy the requirements of the baseline forest test (T2), meaning pre-existing forest cover has not been excluded from these cells, contrary to the requirements of the HIR method (baseline forest cover rule, R1) (Commonwealth of Australia 2013, 2015a, 2016, 2018; Clean Energy Regulator 2019). High to very high rates of non-compliance with T2 (≥50% of cells) were found in more than 1/3rd of the sampled projects. The effect of this non-compliance is that projects are being credited for regenerating forests in areas that contained forest cover when the projects started.
Almost half of the assessed cells (45%) did not satisfy the requirements of the first regeneration gateway test (T3), meaning these cells had less than 7.5% canopy cover in 2023 (T3-a) or experienced a less than 5% increase in canopy cover between commencement and 2023 (T3-b). This result is made more significant by how low the bar is for compliant regeneration under the Regulator’s guidelines (Clean Energy Regulator 2019). The first limb of the gateway check (T3-a) does not require an increase in cover, with cells able to meet the test based solely on the canopy of pre-existing woody vegetation. Cells can meet the regeneration test even if canopy cover declines; a bizarre situation that has arisen because of the Regulator’s decision to allow credited areas to be located on uncleared land containing a significant number of mature trees at project commencement (Clean Energy Regulator 2019). The 5% or more increase in canopy cover required under the second limb of the check (T3-b) is also lenient, particularly given the age of the modelled regeneration in the projects in the sample. The way the T3-b test was implemented for the analysis made it even more generous because, for projects registered prior to 23 March 2016, it used the lowest levels of cover recorded between 2010 and the date of project registration.
Notably, 72% of assessed cells across the sample did not satisfy either the baseline forest test (T2) or the first regeneration gateway test (T3), both of which form part of the Regulator guidelines (Clean Energy Regulator 2019). Seemingly, a significant proportion of the assessed cells were able to meet T3 only because they started with relatively high levels of canopy cover.
Overall, our results suggest the compliance regime for HIR projects has failed to uphold the integrity rules that are supposed to underpin the projects. As of 30 June 2023, the projects in the sample had received 24.6 million ACCUs. The credited sequestration in most of the projects (75%) has exceeded the point where the credited areas should have achieved forest cover from the regeneration alone, excluding the cover provided by pre-existing mature trees and shrubs. Yet only 31% of these projects had an average canopy cover in 2023 ≥20%. Given that 2023 was the third year of a rare triple-dip wet La Niña, if the projects were performing as expected, observed levels of canopy cover across the projects would be significantly higher.
The results suggest the impacts of the projects on regeneration has been small. Canopy cover in the assessed cells has mostly fluctuated between 0 and 30% depending on seasonal conditions, with little evidence of even-aged forest regeneration, as is supposed to be occurring. We found the projects had a small positive effect on canopy cover relative to comparison cells, but that fluctuations in cover in the credited areas have largely been in line with those in comparison cells, outside of the projects. The results are hard to reconcile with claims made by the Clean Energy Regulator and the Emissions Reduction Assurance Committee that there is no ‘persuasive evidence of a lack of integrity with the HIR method or any material problems with compliance’ (Emissions Reduction Assurance Committee 2022, at 2; Clean Energy Regulator 2022).
Our findings here and in Macintosh et al. (2024a) also contrast sharply with the conclusions of three recent Australian government commissioned reports, which all assert that there is a ‘robust regulatory framework’ governing the administration of HIR projects (Chubb et al. 2022; Brack 2023; Australian National Audit Office 2024). In relation to the Chubb Review and ANAO audit, the different conclusions are partly explained by the fact that neither of these reviews systematically analysed relevant data on the delineation of credited areas, regeneration within credited areas, and compliance with regulatory requirements.
Brack (2023) did quantitatively assess relevant data on regulatory compliance in HIR projects in an analysis that aimed to verify the robustness of the Regulator’s processes for administering the initial stratification and regeneration gateway checks. To do this, Brack (2023) quantitatively assessed whether a sample of 25 projects met the initial stratification and regeneration gateway checks, using ~19 250 m × 250 m (6.25 ha) cells within the credited areas of each the sampled projects. The report relied on the Australia’s Environment Explorer (AEX) platform for these purposes, which uses WCF, only with the data presented at 250 m × 250 m scale (i.e. average canopy cover in the 250 m × 250 m cells derived from the underlying 25 m × 25 m WCF data).
This present study and Brack (2023) relied on the same underlying dataset and both studies evaluated compliance with the initial stratification (tested here using T2) and regeneration gateway check (tested here using T3a and T3b) requirements. Our results and conclusions and that of Brack (2023) should be similar, at least in relation to T2, T3-a and T3-b, but they are not.
On the baseline forest cover rule, Brack (2023, at 7) found that ‘of the total sample points observed, 85% (82–88% at P = 0.05) had proponent classifications that agreed with the classification resulting from AEX modelling’. Here, only 71% of assessed cells across the 116 projects were found to be compliant with T2. The difference between the results is most likely explained by the fact we converted the WCF estimates into CPC to better reflect the regulatory requirements, whereas Brack (2023) did not. It is also not clear how Brack (2023) selected sample points or which specific projects were analysed.
Brack (2023) suggests most of its sampled HIR projects satisfied the regeneration gateway check requirements, with ‘the mean canopy area for [19 of the 26 sampled projects being] significantly greater than 7.5%’ (Brack 2023, at 9). According to Brack (2023, p. 9), ‘[o]verall, the [credited areas] appear to be regenerating well in the project areas, especially since 2020 and on average are significantly (P = 0.05) above the 7.5% canopy cover threshold’. The difficulty with the Brack (2023) findings on the regeneration gateway checks is that its method does not reflect the applicable regulatory requirements. Neither the Regulator’s gateway requirements nor the HIR method allow compliance to be assessed on the basis of averages drawn from the entire credited area (Commonwealth of Australia 2013, 2015a, 2016, 2018; Clean Energy Regulator 2019). Compliance must be assessed on a cell-by-cell basis at the prescribed scale. At the first regeneration gateway check, each 100-ha cell must be assessed against the applicable requirements. For projects relying on remote sensing data, these requirements are reflected in T3-a and T3-b. Brack (2023) did not assess compliance on cell-by-cell basis; but rather assessed compliance at the project level based on whether average canopy cover across its ~19 6.25 ha assessment cells was ≥7.5%.B Despite these inconsistencies, the Regulator has claimed the Brack (2023) findings ‘provide further confidence in the ACCU scheme and the integrity of the HIR project portfolio’ (Clean Energy Regulator 2023b).
Conclusions
US legal scholar Dan Farber once wrote that, ‘[i]n all areas of law, there are gaps between the ‘law on the books’ and the ‘law in action’, but in environmental law the gap is sometimes a chasm’ (Farber 1999, at 297). The results of the analysis suggest HIR projects are such a case, where there is a substantial gulf between the legal requirements of the method (the ‘law on the books’) and how projects are being regulated in practice (the ‘law in action’).
Carbon offsets are a high-risk policy instrument, both because of the difficulty in ensuring credits represent real, additional and permanent abatement and the fact that, when they do not, offsets tend to increase greenhouse gas emissions. The high-risk nature of offsets means they should be used with caution. Robust scheme rules are needed to ensure offset projects are only allowed to be undertaken when integrity risks can be adequately managed. Scheme rules should also mandate conservative estimates of abatement and put in place appropriate measures to mitigate permanence risks. However, well-designed scheme rules are of little use without robust compliance and enforcement.
In the context of debates about the integrity of HIR projects and the robustness of the regulatory framework governing them, in this study, we quantitatively assessed the likely extent of compliance with key regulatory requirements under the HIR method and their performance in increasing sequestration in regeneration. We evaluated compliance using four regulatory tests derived from the HIR method and regulatory guidelines published by the Regulator. We divided the credited areas of 116 HIR projects into 35,362 100-ha cells and assessed canopy cover in each cell using the WCF dataset, which was relied on in a verification report published by the Regulator in 2023 (Brack 2023). We used the levels of canopy cover, and the change in canopy cover, both in credited areas and in 100 ha comparison cells from 3 km buffer areas around the projects, to assess compliance with the regulatory tests and project performance. We undertook a statistical analysis to assess the extent to which the projects have resulted in increases in canopy cover that would not have otherwise occurred.
These findings are of global significance, raising questions about the role offsets can play in global decarbonisation efforts. Offsets theoretically provide a means of lowering the cost of mitigating greenhouse gas emissions while enabling hard-to-abate sectors to contribute decarbonisation while technical solutions to abate onsite emissions are progressed. The prominence of offsets is reflected in international legal and policy structures, including the Paris Agreement’s Article 6.4 Mechanism and the Carbon Offsetting and Reduction Scheme for International Aviation. There is already a large and growing body of evidence suggesting offset schemes are frequently plagued by integrity issues (Schneider and Kollmuss 2015; Cames et al. 2016; West et al. 2020, 2023, 2024; Badgley et al. 2022; Stapp et al. 2023). The focus on compliance failures in this study adds a new dimension to the literature, showing how integrity problems can arise from the maladministration of methods and associated breakdowns in governance processes.
Data availability
The data that support this study are available in Open Science Framework (OSF) at https://osf.io/48nq6. A preprint version of this article is available at https://doi.org/10.32942/X2162R.
Conflicts of interest
Andrew Macintosh is a non-executive director of Paraway Pastoral Company Ltd. Paraway Pastoral Company Ltd has offset projects under Australia’s offset scheme. Paraway Pastoral Company Ltd does not have any human-induced regeneration projects. Andrew Macintosh, Don Butler, Dean Ansell and Marie Waschka advise public and private entities on environmental markets and Australia’s carbon offset scheme, including on the design of carbon offset methods.
Author contributions
A. M., M. C. E., D. B., P. L., K. H. & M. J. E. initiated and designed the research, with input from D. A., M. W., and D. L. A. M. & M. C. E. led the drafting, with input from all authors. P. L. & C. E. performed the canopy cover data analysis. A. M. compiled and analysed data on projects and crediting. M. J. E. & K. H. undertook the statistical analysis and generated the figures.
References
Anadón JD, Sala OE, Turner BL, Bennett EM (2014) Effect of woody-plant encroachment on livestock production in North and South America. Proceedings of the National Academy of Sciences 111(35), 12948-12953.
| Crossref | Google Scholar | PubMed |
Archer S, Predick K (2014) An ecosystem services perspective on brush management: research priorities for competing land-use objectives. Journal of Ecology 102, 1394-1407.
| Crossref | Google Scholar |
Armston JD, Denham RJ, Danaher TJ, Scarth PF, Moffiet TN (2009) Prediction and validation of foliage projective cover from Landsat-5 TM and Landsat-7 ETM+ imagery. Journal of Applied Remote Sensing 3, 033540.
| Crossref | Google Scholar |
Badgley G, Freeman J, Hamman J, et al. (2022) Systematic over-crediting in California’s forest carbon offsets program. Global Change Biology 28, 1433-1445.
| Crossref | Google Scholar | PubMed |
Benjamini Y, Hochberg Y (1995) Controlling the false discovery rate: a practical and powerful approach to multiple testing. Journal of the Royal Statistical Society: Series B (Statistical Methodology) 57, 289-300.
| Crossref | Google Scholar |
Bond WJ, Stevens N, Midgley GF, Lehmann C (2019) The trouble with trees: afforestation plans for Africa. Trends in Ecology & Evolution 34, 963-965.
| Crossref | Google Scholar | PubMed |
Booker K, Huntsinger L, Bartolome JW, Sayre NF, Stewart W (2013) What can ecological science tell us about opportunities for carbon sequestration on arid rangelands in the United States? Global Environmental Change 23, 240-251.
| Crossref | Google Scholar |
Booth CA, Barker PJ (1981) Shrub invasion on sandplain country west of Wanaaring, New South Wales. Journal of the Soil Conservation Service of New South Wales 37, 65-70.
| Google Scholar |
Bowen M, Chudleigh F, Sallur N, Sommerfield C (2022) Opportunities to build resilience of beef cattle properties in the mulga lands of south-western Queensland, Australia. The Rangeland Journal 44(2), 115-128.
| Crossref | Google Scholar |
Breidenich C, Magraw D, Rowley A, Rubin JW (1998) The Kyoto Protocol to the United Nations framework convention on climate change. American Journal of International Law 92, 315-331.
| Google Scholar |
Briske DD, Coppock DL (2023) Rangeland stewardship envisioned through a planetary lens. Trends in Ecology & Evolution 38, 109-112.
| Crossref | Google Scholar | PubMed |
Briske D, Vetter S, Coetsee C, Turner M (2024) Rangeland afforestation is not a natural climate solution. Frontiers in Ecology and the Environment 22, e2727.
| Crossref | Google Scholar |
Brown RF (1985) The growth and survival of young mulga (Acacia aneura f. Muell) trees under different levels of grazing. Australian Rangeland Journal 7(2), 143-148.
| Crossref | Google Scholar |
Burrows WH (1973) Studies in the dynamics and control of woody weeds in semi-arid Queensland. 1. Eremophila gilesii. Queensland Journal of Agricultural and Animal Sciences 30, 57-64.
| Google Scholar |
Clean Energy Regulator (2014) ‘Sequestration methodology: Human induced regeneration of a permanent even-aged forest.’ (Commonwealth of Australia: Canberra, ACT) Available at https://webarchive.nla.gov.au/awa/20140211231154/http://www.cleanenergyregulator.gov.au/Carbon-Farming-Initiative/methodology-determinations/Pages/Sequestration-methodology-Human-induced-regeneration-of-a-permanent-even-aged-native-forest.aspx [accessed 11 June 2024]
Clean Energy Regulator (2022) Emissions reduction fund: method claims not substantiated. Available at https://cer.gov.au/emissions-reduction-fund-method-claims-not-substantiated [accessed 26 June 2024]
Clean Energy Regulator (2023b) Independent review provides confidence in the integrity of human-induced regeneration projects. Available at https://cer.gov.au/independent-review-provides-confidence-integrity-human-induced-regeneration-projects [accessed 14 May 2024]
Clean Energy Regulator (2024) ‘Emissions Reduction Fund Register.’ (Commonwealth of Australia: Canberra, ACT) Available at https://cer.gov.au/markets/reports-and-data/accu-project-and-contract-register?view=Projects [accessed 16 September 2024]
Clean Energy Regulator & Department of Climate Change, Energy, the Environment and Water (2023) ‘Joint CER/DCCEEW response to ANU papers on Human Induced Regeneration.’ (Commonwealth of Australia: Canberra) Available at https://cer.gov.au/joint-cerdcceew-response-anu-papers-human-induced-regeneration [accessed 2 June 2024].
Cockfield G, Shrestha U, Waters C (2019) Evaluating the potential financial contributions of carbon farming to grazing enterprises in Western NSW. The Rangeland Journal 41, 211-223.
| Google Scholar |
Crowley G, Murphy S (2023) Carbon-dioxide-driven increase in foliage projective cover is not the same as increased woody plant density: lessons from an Australian tropical savanna. The Rangeland Journal 45(2), 81-95.
| Crossref | Google Scholar |
Cunningham GM, Walker PJ (1973) Growth and survival of mulga (Acacia aneura F. Muell, ex Benth.) in western New South Wales. Tropical Grasslands 7(1), 69-77.
| Google Scholar |
Department of Climate Change, Energy, the Environment and Water (2023a) ‘Major Vegetation Groups (Version 6.0).’ (Commonwealth of Australia: Canberra, ACT) Available at https://www.dcceew.gov.au/environment/land/native-vegetation/national-vegetation-information-system/data-products#mvg60 [accessed 11 June 2024]
Eldridge DJ, Sala O (2023) Australia’s carbon plan disregards evidence. Science 382, 894.
| Crossref | Google Scholar | PubMed |
Eldridge DJ, Bowker MA, Maestre FT, Roger E, Reynolds JF, Whitford WG (2011) Impacts of shrub encroachment on ecosystem structure and functioning: towards a global synthesis. Ecology Letters 14, 709-722.
| Crossref | Google Scholar | PubMed |
Evans MC (2016) Deforestation in Australia: drivers, trends and policy responses. Pacific Conservation Biology 22, 130-150.
| Crossref | Google Scholar |
Evans MC, Carwardine J, Fensham RJ, Butler DW, Wilson KA, Possingham HP, Martin TG (2015) Carbon farming via assisted natural regeneration as a cost-effective mechanism for restoring biodiversity in agricultural landscapes. Environmental Science & Policy 50, 114-129.
| Crossref | Google Scholar |
Farber D (1999) Taking slippage seriously: non-compliance and creative compliance in environmental law. Harvard Environmental Law Review 23, 297-325.
| Google Scholar |
Fensham R (2008) Leichhardt’s maps: 100 years of change in vegetation structure in inland Queensland. Journal of Biogeography 35, 141-156.
| Crossref | Google Scholar |
Fensham R, Powell O, Horne J (2011) Rail survey plans to remote sensing: vegetation change in the Mulga Lands of eastern Australia and its implications for land-use. Rangeland Journal 33, 229-238.
| Crossref | Google Scholar |
Fisher A, Scarth P, Armston J, Danaher T (2018) Relating foliage and crown projective cover in Australian tree stands. Agricultural and Forest Meteorology 259, 39-47.
| Crossref | Google Scholar |
Fleischman F, Basant S, Chhatre A, Coleman EA, Fischer HW, Gupta D, Güneralp B, Kashwan P, Khatri D, Muscarella R, Powers JS, Ramprasad V, Rana P, Solorzano CR, Veldman JW (2020) Pitfalls of tree planting show why we need people-centered natural climate solutions. BioScience 70, 947-950.
| Crossref | Google Scholar |
Gelman A (2008) Scaling regression inputs by dividing by two standard deviations. Statistics in Medicine 27(15), 2865-2873.
| Crossref | Google Scholar | PubMed |
Harrington G (1979a) Estimation of above-ground biomass of trees and shrubs in a Eucalyptus populnea F. Muell. Woodland by regression of mass on trunk diameter and plant height. Australian Journal of Botany 27, 135-143.
| Crossref | Google Scholar |
Harrington G (1979b) The effects of feral goats and sheep on the shrub populations in a semi-arid woodland. The Australian Rangeland Journal 1(4), 334-345.
| Crossref | Google Scholar |
Hodgkinson KC, Harrington G (1985) The case for prescribed burning to control shrubs in eastern semi-arid woodlands. The Australian Rangeland Journal 7(2), 64-74.
| Crossref | Google Scholar |
Kumar D, Pfeiffer M, Gaillard C, Langan L, Martens C, Scheiter S (2020) Misinterpretation of Asian savannas as degraded forest can mislead management and conservation policy under climate change. Biological Conservation 241, 108293.
| Crossref | Google Scholar |
Lett M, Knapp A (2005) Woody plant encroachment and removal in Mesic grassland: production and composition responses of herbaceous vegetation. The American Midland Naturalist 153(2), 217-231.
| Crossref | Google Scholar |
Liao Z, Van Dijk A, He B, Larraondo P, Scarth P (2020) Woody vegetation cover, height and biomass at 25-m resolution across Australia derived from multiple site, airborne and satellite observations. International Journal of Applied Earth Observation and Geoinformation 93, 102209.
| Crossref | Google Scholar |
Long S, McDonald A (2022) Insider blows whistle on Australia’s greenhouse gas reduction schemes. Australian Broadcasting Corporation (ABC), 24 March 2022. Available at https://www.abc.net.au/news/2022-03-24/insider-blows-whistle-on-greenhouse-gas-reduction-schemes/100933186 [accessed 11 June 2024]
Macintosh A, Butler D, Larraondo P, Waschka M, Evans M, Ansell D (2023) ‘The under-performance of human-induced regeneration (HIR) projects: Analysis of misinformation disseminated by the Clean Energy Regulator.’ (The Australian National University: Canberra, ACT) Available at https://law.anu.edu.au/files/2024-01/Response%20to%20CER%20HIR%20graphs%20190623.pdf [accessed 11 June 2024]
Macintosh A, Butler D, Larraondo P, Evans MC, Ansell D, Waschka M, Fensham R, Eldridge D, Lindenmayer D, Gibbons P, Summerfield P (2024a) Australian human-induced native forest regeneration carbon offset projects have limited impact on changes in woody vegetation cover and carbon removals. Communications Earth & Environment 5, 149.
| Crossref | Google Scholar |
Macintosh A, Evans MC, Larraondo P, Butler D, Eldridge D, Ansell D (2024b) Analysis of Brack report on Human Induced Regeneration Gateway Regeneration Checks. The Australian National University, Canberra, ACT. Available at https://law.anu.edu.au/analysis-brack-report-human-induced-regeneration-gateway-regeneration-checks [accessed 26 June 2024]
Moore CWE (1973) Some observations on the ecology and control of woody weeds on mulga lands in north western New South Wales. Tropical Grasslands 7, 79-88.
| Google Scholar |
Neldner VJ, Wilson BA, Dillewaard HA, Ryan TS, Butler DW, McDonald WJF, Richter D, Addicott EP, Appelman CN (2023) ‘Methodology for survey and mapping of regional ecosystems and vegetation communities in Queensland. Version 7.0. Updated December 2023.’ (Queensland Herbarium, Queensland Department of Environment, Science and Innovation: Brisbane, Qld)
NSW Department of Climate Change, Energy, the Environment and Water (2023) ‘NSW State Vegetation Type Map (C2.0M2.0, December 2023).’ (NSW Government: Sydney) Available at https://datasets.seed.nsw.gov.au/dataset/nsw-state-vegetation-type-map [11 June 2024]
Parr CL, te Beest M, Stevens N (2024) Conflation of reforestation with restoration is widespread. Science 383, 698-701.
| Crossref | Google Scholar | PubMed |
Paul K, Roxburgh S (2020) Predicting carbon sequestration of woody biomass following land restoration. Forest Ecology and Management 460, 117838.
| Crossref | Google Scholar |
Paul K, Roxburgh S, England J, et al. (2015) Improved models for estimating temporal changes in carbon sequestration in above-ground biomass of mixed-species environmental plantings. Forest Ecology and Management 338, 208-218.
| Crossref | Google Scholar |
R Core Team (2024) ‘R: A language and environment for statistical computing. Version 4.4.1.’ (R Foundation for Statistical Computing: Vienna, Austria) Available at https://www.R-project.org/
Richards G, Brack C (2004) A continental biomass stock and stock change estimation approach for Australia. Australian Forestry 67, 284-288.
| Crossref | Google Scholar |
Richards G, Evans D (2004) Development of a carbon accounting model (FullCAM Vers. 1.0) for the Australian continent. Australian Forestry 67, 277-283.
| Crossref | Google Scholar |
Sankaran M, Augustine D, Ratnam J (2013) Native ungulates of diverse body sizes collectively regulate long-term woody plant demography and structure of a semi-arid savanna. Journal of Ecology 101, 1389-1399.
| Crossref | Google Scholar |
Schneider L, Kollmuss A (2015) Perverse effects of carbon markets on HFC-23 and SF6 abatement projects in Russia. Nature Climate Change 5, 1061-1064.
| Crossref | Google Scholar |
Schneider L, La Hoz Theuer S (2019) Environmental integrity of international carbon market mechanisms under the Paris Agreement. Climate Policy 19, 386-400.
| Crossref | Google Scholar |
Silcock J, Piddock T, Fensham R (2013) Illuminating the dawn of pastoralism: evaluating the record of European explorers to inform landscape change. Biological Conservation 159, 321-331.
| Crossref | Google Scholar |
Stapp J, Nolte C, Potts M, et al. (2023) Little evidence of management change in California’s forest offset program. Communications Earth & Environment 4, 331.
| Crossref | Google Scholar |
Veldman JW, Overbeck GE, Negreiros D, Mahy G, Le Stradic S, Fernandes GW, Durigan G, Buisson E, Putz FE, Bond WJ (2015) Where tree planting and forest expansion are bad for biodiversity and ecosystem services. BioScience 65, 1011-1018.
| Crossref | Google Scholar |
Vetter S (2020) With Power Comes Responsibility – a rangelands perspective on forest landscape restoration. Frontiers in Sustainable Food Systems 4, 549483.
| Crossref | Google Scholar |
Walker BH, Janssen MA (2002) Rangelands, pastoralists and governments: interlinked systems of people and nature. Philosophical transactions of the Royal Society of London. Series B, Biological sciences 357, 719-725.
| Crossref | Google Scholar | PubMed |
West T, Bomfim B, Haya B (2024) Methodological issues with deforestation baselines compromise the integrity of carbon offsets from REDD+. Global Environmental Change 87, 102863.
| Crossref | Google Scholar |
West T, Börner J, Sills EO, Kontoleon A (2020) Overstated carbon emission reductions from voluntary REDD+ projects in the Brazilian Amazon. Proceedings of the National Academy of Sciences 117(39), 24188-24194.
| Crossref | Google Scholar | PubMed |
West T, Wunder S, Sills EO, et al. (2023) Action needed to make carbon offsets from forest conservation work for climate change mitigation. Science 381(6660), 873-877.
| Crossref | Google Scholar | PubMed |
Westoby M (1984) The self-thinning rule. In ‘Advances in ecological research’. (Eds A MacFadyen, ED Ford) pp. 167–225. (Academic Press) 10.1016/S0065-2504(08)60171-3
Witt GB (2013) Vegetation changes through the eyes of the locals: the ‘artificial wilderness’ in the mulga country of south-west Queensland. The Rangeland Journal 35, 299-314.
| Crossref | Google Scholar |
Witt GB, Harrington R, Page M (2009) Is ‘vegetation thickening’ occurring in Queensland’s mulga lands – a 50-year aerial photographic analysis. Australian Journal of Botany 57, 572-582.
| Crossref | Google Scholar |
Wood SN (2011) Fast stable restricted maximum likelihood and marginal likelihood estimation of semiparametric generalized linear models. Journal of the Royal Statistical Society Series B: Statistical Methodology 73(1), 3-36.
| Crossref | Google Scholar |
Wood SN (2017) ‘Generalized additive models: an introduction with R.’ 2nd edn. (Chapman and Hall/CRC) 10.1201/9781315370279
Footnotes
AIn Australia’s legal system, any guidance issued by government regulators on the interpretation of the law is not determinative and does not shape or constrain how courts interpret the law (Gageler 2015). Regulatory guidance documents merely provided direction on how the relevant regulator intends to interpret and apply the law.
BThere are other peculiar aspects of the method used in Brack (2023), including the choice of 6.25 ha cells (rather than 100 ha) and the fact it assessed compliance based on maximum canopy cover levels over the period 2020–2022 rather than canopy cover in the most recent applicable dataset immediately prior to the submission of the relevant offset report, as required under the rules (Macintosh et al. 2024b). It also does not quantitatively assess compliance in terms of cover increase, as per T3-b (Macintosh et al. 2024b).