Data-driven technologies and management practices for improving the sustainability of reproductive management
Julio O. Giordano A *A
Abstract
Dairy farms must constantly evolve to achieve sustainability goals, including profitability, minimal environmental impacts, and improving the well-being of cows and people. Data-driven management practices and automated technologies are a growing opportunity for improving the sustainability of reproductive management. Precision livestock farming technologies coupled with other herd management data, genomic information, and environmental monitoring tools are enabling the development of data-driven targeted reproductive management and automation of management tasks. An improved understanding of associations between putative data predictors of reproductive outcomes enables targeted reproductive management for cows that share similar expected performance or biological features. Tailored management interventions can be applied on subgroups of cows based on automated estrus alerts, genomic predictions, and ovarian status at the time of non-pregnancy diagnosis. Targeted interventions can lead to shorter interbreeding intervals, increased fertility, and fewer unnecessary interventions on cows. Major advances in engineering, advanced data analytics, and a better understanding of dairy cattle biology through data have also enabled progress with automation of management tasks such as detection of estrus, synchronization of ovulation, and pregnancy testing. Although concerted efforts in research and application are still needed to fully realize the full sustainability benefits of data-driven management and automation, collectively these innovations are reshaping reproductive management of dairy cattle.
Keywords: automation, dairy farm, dairy sustainability, data-driven, detection of estrus, fertility, predictors, reproductive management, synchronization of ovulation, targeted management.
Introduction
Dairy and other livestock farming enterprises are constantly evolving to remain sustainable. Farms must be profitable while minimizing environmental impacts and adapting to socioeconomic conditions that shape market trends and consumer demands. Structural changes in the dairy industry, including larger herd sizes, scarcity of qualified labor, increased labor costs, and interest in improving cow performance and farm personnel working conditions (von Keyserlingk et al. 2013), are major sustainability issues driving innovation in reproductive management. Other dairy farming sustainability challenges, including animal welfare concerns from consumers, are also intensifying as programs to achieve optimal reproductive performance evolve to include more numerous, inconveniently timed and invasive interventions in pursuit of increased efficiencies. These dynamic trends, which are constantly reshaping the dairy industry, are the driving forces of more sustainable reproductive management programs in dairy farms.
Even though the overall reproductive performance of dairy herds has improved dramatically in the last 15 to 20 years, dairy herd management challenges and limitations of dairy cow biology remain. There are also many opportunities to improve herd performance and management that have not yet been explored or fully optimized. Scientific discoveries and technological innovations are thus needed to fuel the evolution of reproductive management practices. Breakthroughs in the understanding of cow reproductive biology and advances in biotechnology and automation are the foundation for the development of novel methods to detect estrus, generate pregnancies, determine pregnancy status, and automate other reproductive management tasks. Data-driven technologies and automated or semi-automated tools for milking, performance monitoring, reproductive diagnostics, genetic selection, health management, feeding, and animal identification are either available or being rapidly developed. This has generated unprecedented opportunities to improve herd performance and management through novel data-driven reproductive programs and automation of reproductive monitoring and management tasks. For example, the growing availability and widespread adoption of precision livestock farming (PLF) technologies, genomics, herd management software, and environmental data collection systems are enabling the exploration and development of data-driven targeted reproductive management (TRM) (Giordano et al. 2022). This novel herd management concept, also known as ‘precision’ or ‘selective management’, entails tailoring reproductive interventions to the biological features and performance potential of subgroups of cows within a herd (Fig. 1). On the other hand, tools for automating or semi-automating human-executed tasks, including detection of estrus, hormonal treatments for synchronization of estrus and ovulation, pregnancy testing, and artificial insemination (AI), are also potential drivers of more sustainable dairy production. These tools can benefit farm management and cows as reducing labor needs and minimizing cow disruption become more relevant dairy sustainability issues. Therefore, this review discusses existing evidence and ongoing research aimed at addressing pressing sustainability issues faced by the dairy industry, and more specifically reproductive management programs. The role of data-driven technologies and management practices for mitigating sustainability challenges and taking advantage of new opportunities are discussed. This review focuses specifically on the potential value of TRM strategies and automation of management tasks for optimizing herd profitability, reducing reliance on reproductive hormones, and minimizing labor needs and cow manipulation.
Conceptual framework for targeted reproductive management (TRM) strategies. Major elements include predictors of reproductive outcomes for creating subgroups of cows of similar predicted reproductive potential (e.g. high, medium, and low probability of expressing estrus or becoming pregnant at first service). Example predictors are sensor data from automated monitoring systems, genetic traits, herd management data, early lactation health events, and environmental conditions. Once subgroups of cows within a herd are identified, different reproductive management strategies can be implemented to these subgroups (e.g. different methods of submission to AI, use of sexed semen). AI, artificial insemination; AIE, cows inseminated at detected estrus; TAI, timed AI; P/AI, pregnancies per AI. Adapted from Giordano et al. (2022).
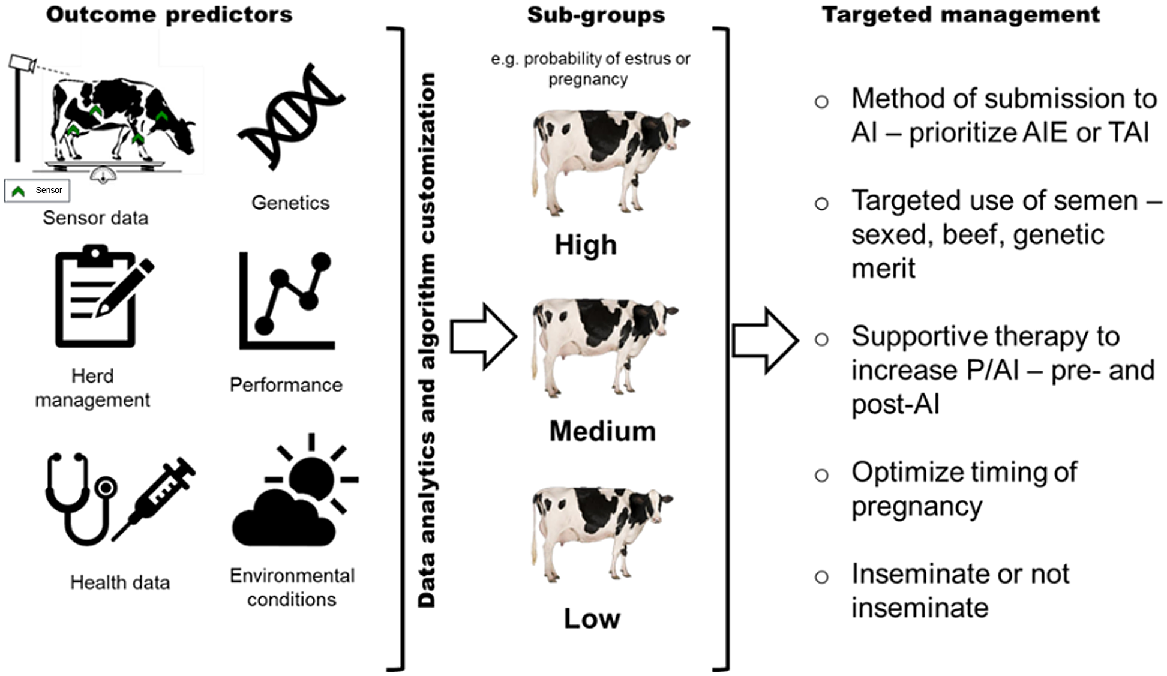
Reproductive programs and dairy sustainability
There are numerous connections between dairy cattle reproductive management and performance with dairy farm sustainability. Reproductive management plays a pivotal role in sustainable dairy production due to the role of pregnancy and lactation on the productive lifecycle of dairy cattle. Optimal days to pregnancy for both non-lactating heifers and cows increase the lifetime productivity, health, and well-being of dairy cattle which collectively increases farm profitability and reduces environmental impact (De Vries 2011; Liang and Cabrera 2015; Giordano 2019). Reproductive programs for heifers and cows that achieve optimal timing of pregnancy during rearing and lactation optimize milk production efficiency per unit of time and space at the dairy (Stangaferro et al. 2018a; Masello et al. 2021). Heifers that conceive early after becoming eligible for pregnancy generate more milk revenue and have reduced rearing costs per day of life than heifers with delayed pregnancy (Masello et al. 2021). Cows that conceive between the second and fourth month of lactation have a lactation length that maximizes income over feed costs during their productive life (De Vries 2006; Stangaferro et al. 2018b). Generating pregnancies efficiently also contributes to increasing the lifespan of dairy cattle. Timely pregnancy reduces the risk of herd exit (i.e. culling) directly because a pregnant cow can have a subsequent lactation and indirectly because of improved milk production efficiency and health. An effective reproductive program also provides the heifer replacement pipeline needed to achieve a financially optimal replacement dynamic. Farms that experience major improvements in reproductive performance are also able to benefit from a growing number of offspring marketing opportunities. Dairy farms with excellent reproductive performance and heifer rearing programs can increase revenue and reduce costs by diversifying the type of offspring born. These dairies can generate enough dairy offspring to meet the adult herd replacement needs and produce surplus dairy and crossbred or purebred beef calves through AI or embryo transfer (ET). Surplus dairy calves can be marketed as high valued dairy replacements whereas crossbred or purebred beef calves are sold at a premium in the beef market. Finally, minimizing the number of interventions needed for establishing and monitoring pregnancy until calving improves farm sustainability through a reduction in production costs. Thus, the ideal reproductive program for a dairy herd is the one that minimizes the number of interventions needed to achieve the maximum number of pregnancies at a time during the rearing period of heifers and lactation in cows that optimizes the lifetime profitability and well-being of cows. Moreover, an effective reproductive program also generates enough pregnancies to yield the replacements needed to maintain an optimal cow replacement dynamic while generating surplus calves for marketing dairy replacements and beef.
Predicting cow biology and future performance through data-driven technologies
Behavioral, physiological, and performance data from precision livestock farming technologies
Dairy farms are rapidly adopting multiple PLF technologies for automating reproductive monitoring and other herd management tasks. Both wearable and non-wearable sensor-based systems offer unprecedented opportunities to record automatically and in real time a vast array of behavioral, physiological, and performance parameters directly or indirectly associated with cow reproductive biology and performance. Sensor technologies enable data collection from more cows and their environment, in real time, at more frequent intervals, without cow manipulation, and at lower cost. Other sensor and non-sensor technologies used to monitor and record dairy herd management practices and environmental conditions are also readily available on commercial farms.
The growing availability of data from automated estrus monitoring systems that record physical activity and other behaviors, such as rumination, eating, and body temperature, enables the study of associations between expression of estrus in early lactation and subsequent reproductive performance. Several recent observational studies have extensively documented that cows with one or more estrus events recorded by automated estrus detection systems (AEDS) are more likely to express estrus again after the end of the voluntary waiting period (VWP), have greater pregnancies per AI (P/AI) to first and subsequent AI services, and are more likely to become pregnant throughout lactation. For example, in two recent studies from our group conducted on commercial farms in the USA and Mexico we reported similar results for cows fitted with either ear-attached or neck-attached sensors for automated detection of estrus (Rial et al. 2022; Laplacette et al. 2024). Cows with ≥1 automated estrus alerts (AEA) during a 50-day VWP (53% of cows) were more likely to be inseminated at detected estrus for first service when managed with programs that prioritized insemination in estrus (Table 1). Cows with AEA also had greater first service P/AI and more cows were pregnant by 150 days in milk (DIM) compared with cows with no AEA during the VWP (Table 1; Rial et al. 2022; Laplacette et al. 2024). Interestingly, the associations with P/AI and cows pregnant by mid-lactation were observed for cows managed with programs designed to maximize insemination in estrus or programs that used all-timed AI (TAI) for first service. In agreement with our findings, other research groups in North America and Europe have reported similar results (Borchardt et al. 2021; Bretzinger et al. 2023; Gonzalez et al. 2023). Moreover, others reported that features of automated estrus alerts during the VWP such as intensity of estrus, are associated with positive reproductive outcomes (Gonzalez et al. 2023). Collectively, a large body of evidence for a strong association between AEA during early lactation and subsequent reproductive performance suggested an opportunity to develop and implement TRM strategies on cows grouped based on having or not having a record of AEA during the VWP.
Item | Group | Diff. p.p | P | ||
---|---|---|---|---|---|
AEA-VWP | NoAEA-VWP | ||||
Cows AIE, % (n) | |||||
Study 1 | 85.7 (520) | 48.3 (457) | 37.4 | <0.01 | |
Study 2 | 83.3 (346) | 45.0 (233) | 38.3 | <0.01 | |
Average | 84.7 (866) | 47.2 (690) | 37.5 | ||
P/AI first AI, % (n) | |||||
Study 1 | 42.4 (785) | 36.8 (683) | 5.6 | 0.03 | |
Study 2 | 38.7 (648) | 23.6 (418) | 15.1 | <0.01 | |
Average | 40.7 (1433) | 31.8 (1101) | 8.9 | ||
Preg. 150 DIM, % (n) | |||||
Study 1 | 79.5 (785) | 70.8 (683) | 8.7 | <0.01 | |
Study 2 | 75.8 (691) | 58.5 (486) | 17.3 | <0.01 | |
Average | 77.8 (1476) | 65.7 (1169) | 12.1 |
Diff. p.p., difference in percentage points.
Data generated by PLF technologies could also be used to characterize behavioral, physiological, and performance drivers of variation in cow fertility that individually or collectively could be used to predict reproductive outcomes, such as the likelihood of pregnancy to an insemination. Thus, we recently focused on characterizing associations between cow, herd, and environmental data collected by PLF technologies with the outcome of individual inseminations. In one study conducted at a commercial farm where cows and facilities were fitted with several wearable and non-wearable sensors, we evaluated the pattern of several behavioral, physiological, and performance parameters recorded from late gestation to the time immediately before insemination in cows that became pregnant or not to the first service postpartum (Granados 2022). We also explored associations between the first service outcome with early lactation events, previous lactation performance, and environmental conditions. For primiparous cows, the most notable differences observed were greater milk yield, milk components yield, and fewer lying bouts per day for pregnant than non-pregnant cows. On the other hand, numerous and more diverse associations of different magnitude were observed for multiparous cows. In this group, cows that failed to become pregnant produced more milk and milk fat, had greater body temperature, more activity, more resting time, and had larger body weight changes after calving compared with pregnant cows. We also observed several associations of varying direction and magnitude between pregnancy outcome to first service with previous gestation, and previous and current lactation features, events, and performance for both primiparous and multiparous cows. Evidence of multiple associations between these several cow, herd management, and environmental parameters with the likelihood of pregnancy to first insemination suggested a potential path towards the development of pregnancy prediction models that could help group cows for TRM. Moreover, the large variation between parity groups for the direction and magnitude of the associations observed confirmed that cow parity is a major driver of variability and suggested that models for predicting pregnancy based on PLF data might have to be parity specific.
Although more research is needed to fully characterize the numerous and diverse potential associations between PLF data and reproductive outcomes, existing evidence provided the foundation for the exploration of some TRM strategies based on PLF technologies data.
Ovarian physiological status data from reproductive diagnostic tools
Another technology readily available for reproductive diagnostics in dairy farms is B-mode or Doppler ultrasonography. Coupled with recent advances in the understanding of ovarian function and pregnancy biology (Wijma et al. 2016; Ricci et al. 2017; Domingues et al. 2024), ultrasonography can be used to approximate the ovarian physiological status of cows (Wijma et al. 2017), enabling the development and implementation of targeted synchronization of ovulation protocols. As one of the most common uses of ultrasonography in dairy farms is non-pregnancy diagnosis (NPD), much of the efforts to develop TRM strategies including ultrasonography data have focused on previously inseminated cows. Recent studies that characterized in detail the follicular wave and corpus luteum (CL) growth and regression dynamics in cows with and without pregnancy loss helped identify subgroups of cows with contrasting ovarian status at the typical time when ultrasonography is used for NPD in many dairy herds (Wijma et al. 2016, 2017; Ricci et al. 2017). At 29 to 35 days after a previous AI, approximately 70–75% of non-pregnant cows had a functional CL responsive to one or two prostaglandin (PGF)2α treatments and at least one follicle in the growing or static phase of development (Wijma et al. 2017, 2018; Pérez et al. 2020). In most of these cows the follicle was also large enough (i.e. ≥10 mm) to growth to preovulatory size and ovulate after gonadotrophin-releasing hormone (GnRH) within 3–4 days of induction of luteolysis at NPD. On the other hand, the remaining 25–30% of cows either did not have a functional CL responsive to PGF or had a follicle that was too small to growth to preovulatory size or was at a stage of development at which it would be unresponsive to induction of ovulation within 3–4 days of induction of luteolysis at NPD. Thus, these two distinct groups of cows were considered good candidates to receive protocols for synchronization of estrus or ovulation tailored to their specific ovarian status at NPD. This improved understanding of the ovarian function of previously inseminated cows was the gateway for the design of programs that enable achieving key reproductive performance and management goals, including maximizing fertility, reducing the interbreeding interval, minimizing the number of unnecessary management interventions, and avoiding disruption of the normal pattern of return to estrus after a previous insemination.
Availability of novel methods for pregnancy testing and determination of the ovarian physiological status of cows such as Doppler ultrasonography, cow-side, in-line milk, or bench top on-farm assays for detection and measurement of markers of pregnancy and CL function can lead to further gains in the understanding of ovarian function status and potential before or at NPD. A greater ability to predict ovarian function can enable the development and refinement of novel programs to reduce the interbreeding interval and optimize the fertility of previously inseminated cows beyond what is possible with existing programs. For example, the ability to determine CL functionality on a single observation using Doppler ultrasonography could improve the classification of cows into subgroups with and without a functional CL at NPD. As cow-side or on-farm assay methods to determine progesterone levels become available or more widespread, these could also be used to identify cows in CL functionality groups. Another area of opportunity to improve the performance of re-insemination programs based on the ovarian status of cows at NPD, is improving the prediction of follicle function because follicle size is a poor predictor of the stage of follicle development. Although more difficult because of the less understood association between blood flow and follicular function, and the complexities of defining follicle blood flow on-farm, predicting the stage of follicle development based on Doppler US could help optimize tailored resynchronization of ovulation and estrus protocols.
Data for cow features, events, and performance records
A myriad of associations between cow features, events, and performance records of individual cows and herds with reproductive outcomes have been extensively documented. Therefore, any cow characteristic, application of a herd management practice, or the occurrence of events that are consistently associated with reproductive outcomes could be used as a predictor in TRM. For herds that genomically tests cows, fertility traits could be used to group cows because of the well-documented associations between traits such as genomic daughter pregnancy rate (gDPR) with several outcomes including the likelihood of insemination in estrus, P/AI, and pregnancy rate throughout lactation (Chebel and Veronese 2020; Lima et al. 2020; Sitko et al. 2023). Cow parity, which has been associated with the probability of estrus expression and fertility in multiple studies (Madureira et al. 2015; Pinedo et al. 2020), or records of health disorder events, which were associated with fewer P/AI, reduced pregnancy rates, and increased pregnancy losses (Carvalho et al. 2019) could also be used to classify cows in groups of different reproductive potential. Milk yield level within herds or for cows managed under the same conditions has also been linked to reproductive performance and could be used to group cows for TRM (Stangaferro et al. 2018b). Ultimately, each one of these factors and data associated with reproductive outcomes could be used as predictors for creating subgroups of cows for TRM. In contrast to PLF technologies used for reproductive management, data for several of these predictors are routinely collected or easily recorded in the herd management software at no extra cost as these are typically used for other herd management practices or decision-making.
Combination of multiple predictors
The drivers and factors of biological variation and cow performance that can be used for prediction can act independently and synergistically. Moreover, individual cows can present one or more of these features or be affected by one or more of the factors that influence performance. Therefore, a potential strategy to create subgroups of cows with the largest possible differences in predicted reproductive potential is to combine data from multiple predictors. This was recently explored in a study from our group in which we documented the effect on reproductive outcomes of grouping cows based on the combination cow features, performance, and early lactation events data with AEA during the VWP (Rial and Giordano 2024). Cows were first grouped based on the presence or absence of factors that were associated with poor reproductive outcomes. These included a record of health disorders during the early postpartum period, ranking in the low tertile for gDPR, and ranking in top tertile of milk yield within the herd. Cows were also grouped based on the presence or absence of a record of AEA during the VWP. Finally, cows were grouped based on the combination of risk factors for poor reproductive performance and AEA during the VWP. In support of our hypothesis, grouping cows based on the combination of the risk factors and records of AEA resulted in the creation of groups of cows with larger and more consistent differences in reproductive outcomes than when a single predictor was used to group cows. For example, cows that had at least one AEA during the VWP and did not present any of the risk factors for poor reproductive performance had the greatest likelihood of expressing estrus, had the most P/AI at first service, and the most cows pregnant by 150 DIM compared with the rest of the cows in the herd. On the other hand, cows with no AEA and at least one of the risk factors for poor reproductive performance had the worst reproductive outcomes. These encouraging results provided foundational evidence to explore the combination of multiple predictors for creating groups of cows with stark differences in reproductive performance potential for TRM.
In summary, a large body of evidence currently supports several consistent associations between predictors of reproductive potential and reproductive and management outcomes of interest. The differences observed for cows grouped based on these predictors were of sufficient magnitude to justify exploration and implementation of TRM strategies. Combining multiple predictors might be beneficial for creating subgroups of cows for TRM because no single factor can explain all the variation within and across herds and the multiple predictors available are likely to interact with each other.
Data-driven reproductive strategies for optimizing herd management and performance
Conceptually, TRM consists of identifying groups of cows within a herd that share similar characteristics or predicted performance and therefore could benefit from tailored management strategies or interventions (Giordano et al. 2022). In principle, TRM should optimize reproductive performance, management, profitability, or all to a greater extent than if the whole herd is managed with a single reproductive program. Cows can be grouped based on one or more predictors of performance such as for example genetic merit for fertility (Sitko et al. 2023), estrus expression during the voluntary waiting period (Rial et al. 2022; Gonzalez et al. 2023; Laplacette et al. 2024), milk production level (Arbel et al. 2001), or early lactation health. Ultimately, the goal of implementing TRM is contributing to the sustainability of reproductive programs and dairy farms by increasing herd profitability, reducing reliance on reproductive hormones, and minimizing labor needs and cow manipulation.
Automated detection of estrus during the VWP
Based on the known association between occurrence of AEA during the VWP and reproductive performance (Borchardt et al. 2021; Rial et al. 2022; Gonzalez et al. 2023), first service management programs aimed at optimizing use of insemination in estrus and TAI have been designed for cows with or without AEA during the VWP.
In a recent experiment, Rial et al. (2022) compared the reproductive performance of cows managed with a TRM program designed to prioritize insemination in estrus (TP-AIE) and optimize timing of first service by grouping cows based on AEA recorded during the VWP (Fig. 2). The TP-AIE program aimed to maximize AI to estrus for cows with AEA during the VWP by providing these cows 31 days for detection of estrus. If not inseminated in estrus, cows were enrolled in the P4-Ovsynch protocol (GnRH plus controlled internal drug release (CIDR) insertion-7 days later PGF2α and CIDR removal-1 day later PGF2α-32 h later GnRH-16–18 h later AI) for TAI. Conversely, cows with no AEA during the VWP were given only 17 days for detection of estrus and thereafter were enrolled in the P4-Ovsynch protocol. The idea behind this strategy was to minimize use of TAI and maximize use of AI in estrus in cows most likely to express estrus and have good fertility if inseminated to estrus. On the other hand, the more aggressive use of TAI for cows with no AEA during the VWP was expected to help these cows which are less likely to express estrus and have poor fertility if inseminated in estrus. The performance and herd management benefits and drawbacks of the TP-AIE program were compared with a program that used TAI after a Double-Ovsynch protocol and had a longer VWP (ALL-TAI), and a non-TRM program that also prioritized AI in estrus (P-AIE) but did not use AEA during the VWP to group cows. The latter enabled evaluating the effects of managing cows based on AEA during the VWP. The ALL-TAI treatment served as positive control because the program used is known to maximize first service fertility and contribute to an excellent pregnancy rate.
Lactating Holstein cows were assigned to a program that used TAI after a Double-Ovsynch protocol with two PGF2α and an extended VWP for all cows (ALL-TAI), a program that prioritized AIE and used TAI for cows not detected in estrus (P-AIE), or a TRM program that prioritized AIE and provided different periods for detection of estrus to cows that had or did not have AEA during the VWP (TP-AIE). AEA, automated estrus alerts; AIE, AI at detected estrus based on AEA; P4-Ovsynch, Ovsynch protocol with two PGF2α and progesterone supplementation via a CIDR device. Adapted from Rial et al. (2022).
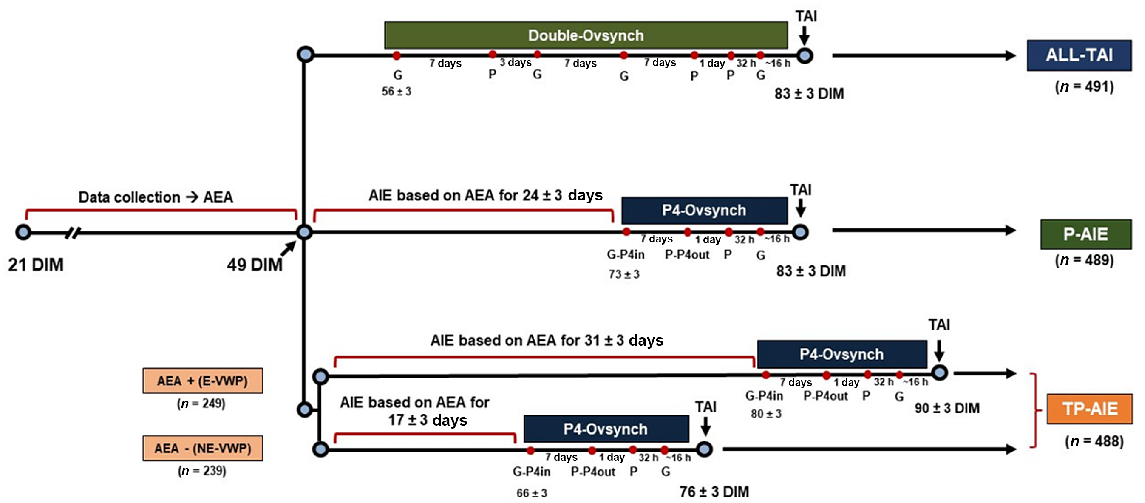
Interestingly, the TP-AIE program resulted in a greater pregnancy rate after calving (HR = 1.2; 95% CI 1.1–1.4) but the same proportion of cows pregnant by 150 DIM than the ALL-TAI program (Fig. 3). Moreover, TP-AIE generated pregnancies faster after calving despite having reduced P/AI to first service (~8 percentage points (p.p.)). As a result, ~50% of the cows were pregnant by the time that cows in the ALL-TAI treatment received first service. This was due to a combination of earlier DIM at first service and early re-insemination of non-pregnant cows. In addition, overall P/AI to first and second services was relatively high (~40%) which contributed to generate many early pregnancies. Although the TP-AIE treatment was effective at increasing the pregnancy rate early in lactation, this gain was diluted as the lactation progressed because the ALL-TAI treatment compensated for the delayed timing of first service through greater P/AI. Thus, from a practical perspective, the TP-AIE program can help maximize insemination of cows in estrus while targeting TAI to cows that otherwise would have a delayed first service. This approach reduces reliance on reproductive hormones and provides a better justification for the use of synchronization of ovulation for TAI. For example, in the experiment of Rial et al. (2022), only 15 and 53% of the cows (~30% overall) with and without AEA during the VWP received TAI as compared with 100% of the cows in the ALL-TAI treatment. In contrast, other than providing an optimized period for AI in estrus and a data-driven justification for the use of TAI (i.e. only cows that need synchronized are synchronized), no major benefits on performance were observed for the TP-AIE than the P-AIE program. Overall, the proportion of cows AI in estrus (P-AIE = 72.3%, TP-AIE = 68.2%), P/AI (P-AIE = 40.2% and TP-AIE = 39.5%), and the pregnancy rate (HR = 1.2; 95% CI 1.1–1.4) did not differ for these two programs. Although the experiment reported by Rial et al. (2022) was conducted at a single herd in the USA, the results were replicated almost exactly in another more recent experiment from our group in which used a similar experimental design but with lactating dairy cows at a dairy in Mexico and used a neck-attached instead of an ear-attached sensor based automated estrus detection system (Laplacette et al. 2024). Similar experiments from other groups which have implemented TRM management based on AEA during the VWP to optimize AI in estrus, and TAI have also reported similar results and benefits to herd management than our experiments (Fricke et al. 2014; Gonzalez et al. 2023).
Survival curves for time to pregnancy up to 150 days in milk (DIM). The hazard ratio (HR) and 95% confidence interval (CI) for time to pregnancy was greater (P = 0.008) for the targeted predominant AI in estrus program (TP-AIE; HR = 1.2; 95% CI 1.1–1.4) and predominant AI in estrus program (P-AIE; HR = 1.2; 95% CI 1.1–1.4) than for the all-timed AI (ALL-TAI) treatment. The was no difference between the P-AIE and TP-AIE treatments (HR = 1.0; 95% CI 0.9–1.2). Median time to pregnancy was 102, 89, and 89 days for the ALL-TAI, P-AIE, and TP-AIE treatments, respectively. Adapted from Rial et al. (2022).
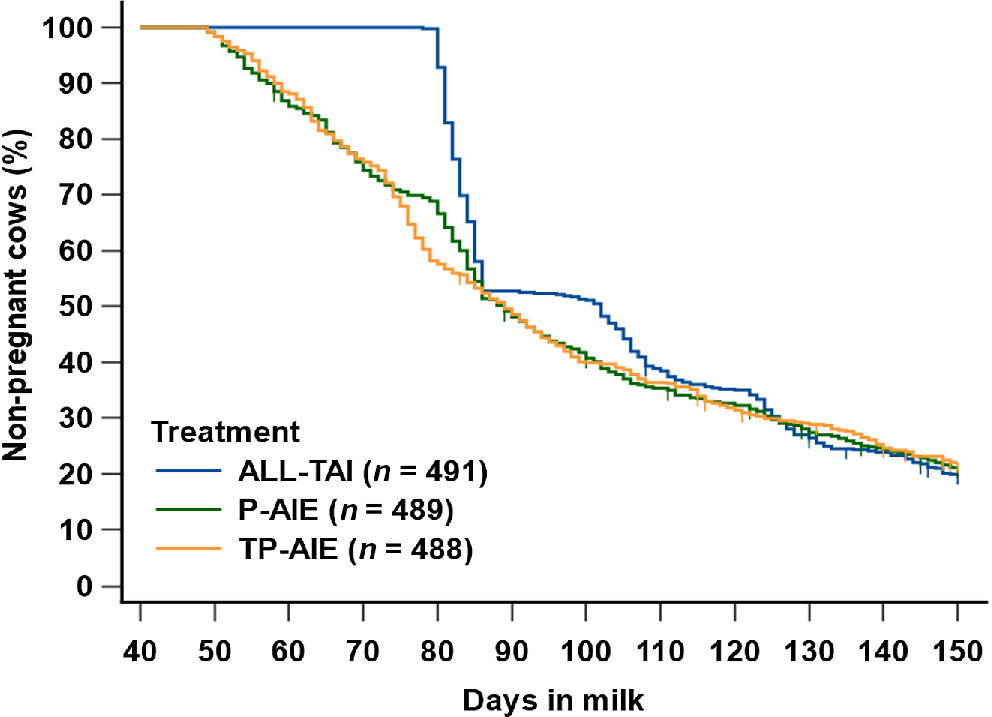
Taken together, current evidence suggests that TRM strategies that use AEA during the VWP to prioritize insemination of cows in estrus and optimize timing of insemination might be a reasonable strategy to use when the pregnancy rate after calving and the percentage of cows pregnant by mid-lactation are the most relevant outcomes of interest. Conversely, TRM programs that prioritize insemination in estrus might not be ideal for herds that prefer to or need to maximize first service P/AI. Critical to the success of this TRM based on AEA during the VWP is the implementation of efficient and accurate estrus detection programs combined with systematic and timely use of synchronization of ovulation. This can be easily accomplished in farms that use AEDS during the VWP because estrus alerts can be easily transferred to the dairy herd management software to assign cows to tailored management programs.
Ovarian status at non-pregnancy diagnosis
Recently, TRM strategies designed to tailor hormonal therapy for cows with different ovarian physiological status at NPD were developed and evaluated. These strategies aim to increase AI in estrus and fertility to TAI while reducing the interbreeding interval without disrupting spontaneous expression of estrus. From a management perspective, reinseminating more cows in estrus is appealing to some farms because fewer interventions and cow manipulation are needed for application of hormonal treatments and implementation of pregnancy tests.
A program specifically developed for optimizing reproductive performance and management through more AI in estrus, a short interbreeding interval, and targeted use of TAI for cows that truly need synchronization and hormonal supplementation is Short-Resynch plus P4-Ovsynch. Based on evidence that most cows with a CL ≥ 15 mm and a follicle >10 mm (herein CL cows) at NPD 32 ± 3 days after AI can be synchronized and have acceptable P/AI without the first GnRH of Ovsynch (Wijma et al. 2017), the Short-Resynch (PGF2α-24 h later-PGF2α-32 h later-GnRH-16–18 h later TAI; Fig. 4) protocol is applied on these cows. The TAI interbreeding interval for CL cows, which represent 70–75% of the non-pregnant cows, is 35 ± 3 days. This is a full week shorter than if these cows were enrolled in the Ovsynch protocol at NPD. On the other hand, for the 25–30% of cows that have no luteal tissue, a CL < 15 mm, or a follicle >10 mm at NPD (herein NoCL cows), the P4-Ovsynch protocol (Fig. 4) is used. These cows benefit from the targeted use of P4 supplementation and two PGF2α treatments. The extra P4 optimizes the endocrine environment during follicle growth. The additional PGF treatment ensures complete luteal regression in a group of cows that due to the high ovulatory response to GnRH (Giordano et al. 2016) is likely to have a single 7-day-old CL somewhat refractory to a single PGF treatment at induction of luteolysis (Carvalho et al. 2018). Ultimately, the overall goal of the Short-Resynch plus P4-Ovsynch program is to achieve a combination of benefits. These include maximizing AI in estrus before NPD, reducing the interbreeding interval for CL cows, and optimizing the fertility of NoCL cows. Experiments at commercial farms confirmed the expected positive effects of these programs on herd management outcomes and demonstrated improved herd performance compared with the commonly used D32-Resynch protocol (GnRH-7 days later-PGF2α-56 h later GnRH-16–18 h later TAI). The Short-Resynch plus P4-Ovsynch program increased the hazard of pregnancy by ~20%, reduced days to pregnancy by 11 days, and reduced the proportion of non-pregnant cows at the end of lactation by ~7 p.p. (Wijma et al. 2018). Notably, these positive effects were attained without disrupting insemination in estrus before NPD, which not only contributes to achieving excellent pregnancy rates but also reduces the number of hormonal treatments (i.e. only cows that need TAI receive hormonal treatments) and pregnancy tests needed.
Schematic representation of the Short-Resynch (PGF2α-24 h later-PGF2α-32 h later-GnRH-16–18 h later TAI) plus P4-Ovsynch (GnRH plus CIDR insertion-7 days later PGF2α and CIDR removal-1 day later PGF2α-32 h later GnRH-16–18 h later AI) program. This management strategy is aimed at maximizing the proportion of inseminations at detected estrus before non-pregnancy diagnosis, reduce the interbreeding interval for most cows (i.e. cows with CL), and increase the fertility of cows without a CL at non-pregnancy diagnosis. AIE, insemination at detected estrus; NPD, non-pregnancy diagnosis; TAI, timed AI.
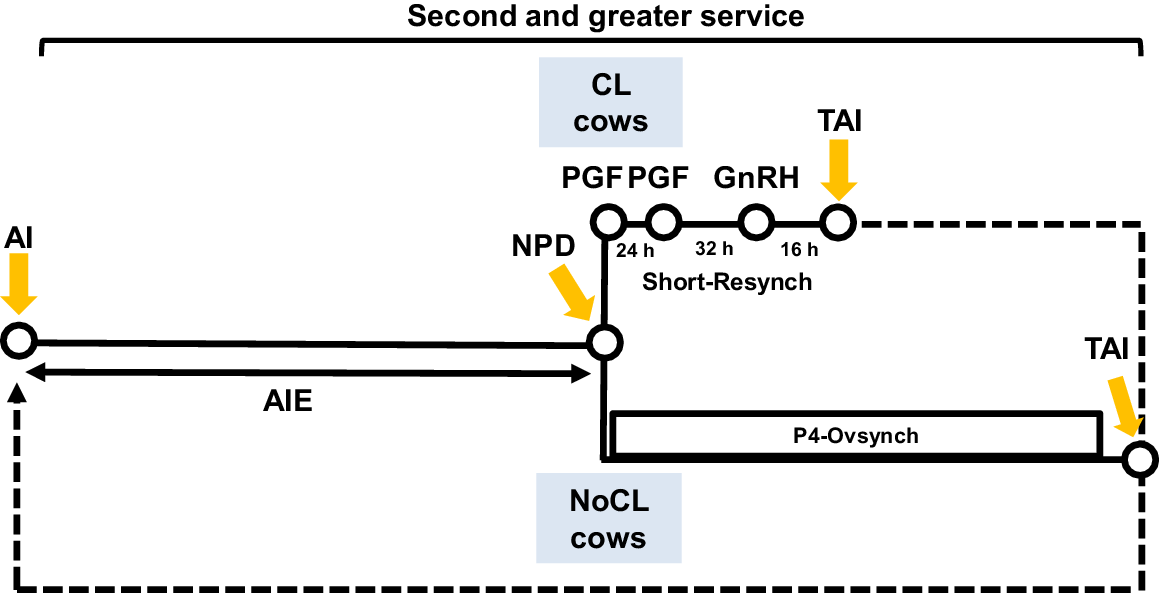
Some of the herd management benefits of the Short-Resynch plus P4-Ovsynch protocol are also obvious when compared with the alternative D25-Resynch (25 ± 3 days after AI GnRH-7 days later NPD and PGF2α-24 h later PGF2α-32 h later GnRH-16–18 h later TAI) for CL cows and P4-Ovsynch program for NoCL cows. In this program, all previously inseminated cows receive a GnRH treatment to initiate a new follicular wave 7 days before NPD. As expected, including the GnRH treatment increases the percentage of CL cows at NPD (~10 p.p.) and their P/AI (~6–9 p.p.). Nevertheless, fewer cows are re-inseminated in estrus before NPD (10 p.p.), more cows require NPD and synchronization, and the interbreeding interval for CL cows is longer than with Short-Resynch because these cows are forced to receive TAI. Notably, the more P/AI for CL cows that receive GnRH did not reduce days to pregnancy or increase the percentage of cows pregnant by the end of lactation because inseminating more cows in estrus and the overall shorter interbreeding interval obtained with the Short-Resynch plus P4-Ovsych program fully compensated for the difference in P/AI (Pérez et al. 2020). From a management perspective, a major caveat of including the GnRH treatment before NPD is that all previously inseminated cows must receive the injection. This causes unnecessary application of one extra dose of GnRH to all pregnant cows, adding costs and disrupting cows and farm management routines. Altogether, evidence from multiple experiments (Wijma et al. 2017, 2018; Pérez et al. 2020) and anecdotal evidence from commercial farms, suggests that the Short-Resynch plus P4-Ovsynch program might be a valuable strategy not only for achieving optimal reproductive performance but also for meeting herd management goals including AI of more cows in estrus, reducing hormonal interventions and reliance on TAI, reducing pregnancy testing needs, and reducing cow manipulation and disruption.
Another type of program that aims to maximize re-insemination of cows at detected estrus for reducing interbreeding intervals and reliance on hormonal interventions for TAI consists of treatment with PGF2α on CL cows and P4-Ovsynch for NoCL cows at NPD 32 ± 3 days after AI (Fig. 5) (Giordano et al. 2015; Masello et al. 2020a). The PGF2α treatment promotes expression of estrus which allows for immediate re-insemination after NPD of a substantial proportion of cows. This program is especially appealing to farms that have high estrus detection efficiency with traditional estrus detection methods or the growing number of farms that utilize AEDS. Treatment of cows based on ovarian structures increases efficiency and fertility of subgroups of cows because PGF2α is only applied to cows likely to express estrus in response to the treatment, whereas the P4-Ovsynch protocol is applied to cows not expected to respond to PGF2α and known to have increased fertility with this protocol (Wijma et al. 2018). Experiments that evaluated the re-insemination dynamic of lactating dairy cows managed with this type of program (Giordano et al. 2015; Masello et al. 2020a) demonstrated similar performance when compared with enrollment of all cows in Ovsynch-like protocols when at least ≥40% of the open cows with a CL receive AI in estrus after NPD. Because neither overall P/AI nor time to pregnancy improve with these programs compared with alternative resynchronization protocols, their greatest value is for increasing re-insemination of cows at detected estrus rather than by TAI. Ultimately, farms can expect a reduction in the number of hormonal interventions for TAI (Giordano et al. 2015; Masello et al. 2020a).
Schematic representation of a reproductive management strategy aimed at maximizing the proportion of inseminations at detected estrus through synchronization of estrus for cows with a corpus luteum and synchronization of ovulation for cows without a corpus luteum at the time of non-pregnancy diagnosis. AIE, insemination at detected estrus; NPD, non-pregnancy diagnosis; TAI, timed AI; P4-Ovsynch, GnRH plus CIDR insertion-7 days later PGF2α and CIDR removal-1 day later PGF2α-32 h later GnRH-16–18 h later AI.
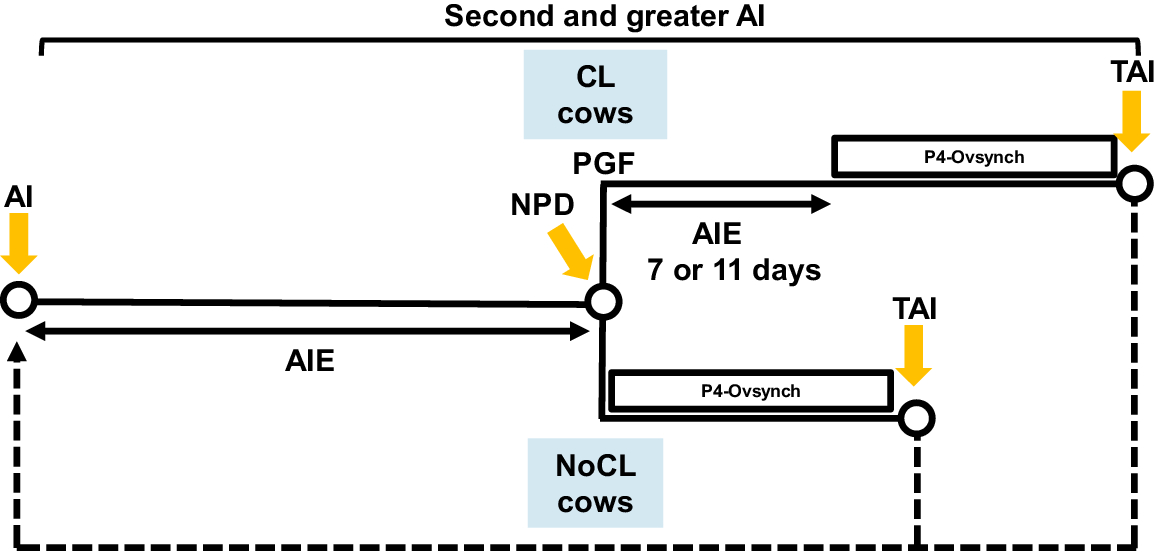
As novel data-driven tools become more readily available to more accurately classify cows in the CL and NoCL group, determine the ability of follicles to grow to preovulatory size after induction of luteolysis, predict the likelihood of expression of estrus after AI, and enable earlier NPD, programs that rely on determination of the ovarian status of cows at NPD for tailoring treatments will become more effective and will help achieve more stringent herd management goals relevant to dairy sustainability.
Use of genomic predictions for targeted reproductive management
Based on the known association between genetic merit for fertility and reproductive performance (Chebel and Veronese 2020; Lima et al. 2020; Sitko et al. 2023), genomic predictions have been used to create subgroups of cows for TRM. In an experiment using primiparous lactating Holstein cows from six commercial farms in NY (Sitko et al. 2023), we tested the hypothesis that cows of different genetic merit for fertility would have different responses to a reproductive management program that prioritized AI in estrus (P-AIE) versus one that prioritized TAI (P-TAI) for all AI services (Fig. 6). More specifically, we hypothesized that cows of superior genetic merit for fertility would have similar reproductive performance when managed with the P-AIE or P-TAI program, whereas cows with inferior fertility potential would have improved reproductive performance when managed with the P-TAI program. The idea behind this hypothesis was that high-fertility cows would have good performance regardless of type of management received, whereas the low-fertility cows would benefit from aggressive use of TAI. To test this hypothesis, cows classified in a high (Hi-Fert), medium (Med-Fert), and low (Lo-Fert) fertility group based on a Reproduction Index (RI) value calculated from several gPTA values provided by the Clarifide test (Zoetis Inc.) were assigned to the P-AIE or P-TAI treatment.
Cows in the predominant timed AI (P-TAI) treatment received timed AI (TAI) at 84 ± 3 DIM after Double-Ovsynch. Thereafter, cows received AI at detected estrus (AIE) any time or TAI if not AIE. Non-pregnant cows with a corpus luteum ≥15 mm and an ovarian follicle ≥10 mm received a D25-Resynch protocol with two PGF2α treatments. Cows that did not meet these criteria received a modified Ovsynch-56 protocol with two PGF2α treatments and progesterone supplementation (P4-Ovsynch; GnRH plus CIDR insertion-7 days later PGF2α and CIDR removal-1 day later PGF2α-32 h later GnRH-16–18 h later AI). Cows in the P-AIE treatment were eligible for AIE after a PGF2α treatment at 53 ± 3 days in milk (DIM). Cows not AIE by 74 ± 3 DIM received timed AI after P4-Ovsynch. Thereafter, cows were AIE at any time. Non-pregnant cows at the pregnancy diagnosis at 32 ± 3 days after AI received P4-Ovsynch for TAI at 42 ± 3 days after AI. AIE*, cows AIE during synchronization of ovulation. Adapted from Sitko et al. (2023).
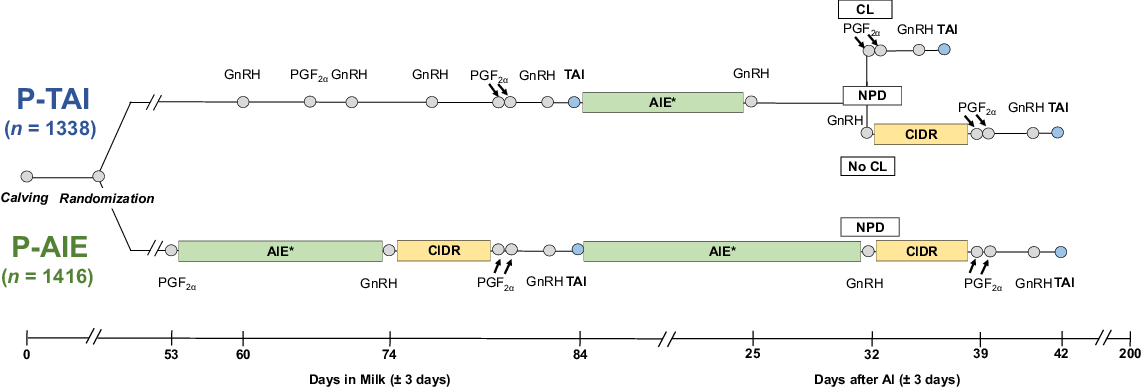
Data partially supported our hypothesis because cows in different fertility groups had a different response to treatments but only for some of the outcomes evaluated. Moreover, some responses were not in line with our expectations. For the first service, the P-TAI treatment increased P/AI for cows in all fertility groups (Table 2). This agreed with the idea that the Lo-Fert group would benefit by the combination of a longer VWP and use of a fertility protocol (Double-Ovsynch). Thus, these two strategies combined might be used to increase the fertility of the Lo-Fert group when the main goal is to maximize first service P/AI. Alternatively, data also showed that the P-AIE program, which might be simpler, cheaper to implement, and minimizes use of TAI, would most likely reduce P/AI for low-fertility cows (Table 2). Interestingly, cows in the Hi-Fert and Med-Fert group in the P-TAI treatment had increased P/AI, and the increase was of a similar magnitude than for cows in the Lo-Fert group. Thus, our hypothesis that cows of superior genetic merit for fertility would have a similar response to the P-AIE and P-TAI programs was not supported. Data suggested that either the mechanisms by which genetics improves fertility are different but synergistic with those enhanced by the fertility protocol and longer VWP, or the latter enhance the physiological mechanisms under genetic control that cause differences in fertility.
Item A | Fertility group (FG) based on Reproduction Index (RI) | P-value | ||||||||
---|---|---|---|---|---|---|---|---|---|---|
High | Medium | Low | ||||||||
P-AIE | P-TAI | P-AIE | P-TAI | P-AIE | P-TAI | Trt | FG | Trt × FG | ||
P/AI first test (n) | 55.0Aa (387) | 64.3Ab (379) | 47.8Ba (524) | 59.4Bb (466) | 43.4Ca (423) | 52.0Cb (386) | <0.01 | <0.01 | 0.81 | |
P/AI confirm (n) | 53.8Aa (387) | 61.5Ab (379) | 45.4Ba (524) | 55.8Bb (466) | 41.1Ca (423) | 48.4Cb (386) | <0.01 | <0.01 | 0.79 | |
Preg. loss (n) | 2.0 (207) | 4.3 (239) | 5.0 (247) | 6.0 (273) | 5.5 (185) | 6.8 (201) | 0.14 | 0.10 | 0.68 |
Different uppercase letter superscripts (A, B, C) within a row indicate (P ≤ 0.05) between fertility groups.
Different lowercase letter superscripts (a, b, c) within a row indicate (P ≤ 0.05) between treatment groups.
Preg. loss, pregnancy loss between the two pregnancy tests; Trt, treatment; FG, fertility group; Trt × FG, treatment by fertility group interaction.
Regardless of the reasons for these observations, our data suggested that certain herd management strategies are possible for cows of superior and intermediate fertility potential. If the goal is to maximize fertility to first service and using a complex protocol for synchronization plus an extended VWP is not a problem, this strategy could be used to maximize P/AI of Hi- and Med-Fert cows. These cows might be good candidates for TRM such as AI with sexed-semen, costly semen from high genetic merit bulls, longer VWP, or other strategies that require the highest possible fertility to first service. Alternatively, if the main goal is to simplify management or reduce reliance on synchronization of ovulation, cows of superior genetic merit for fertility are expected to have good fertility if submitted to AI with a program that prioritizes AI in estrus. Thus, for farms in which management constraints are more important than maximizing first service fertility, prioritizing AI in estrus might be a good alternative for cows of superior fertility potential. On the other hand, for cows of inferior fertility potential, a program that prioritizes TAI with a fertility protocol and an extended VWP could improve performance.
Like for first service outcomes, data for the pregnancy rate by 200 DIM partially supported the hypothesis that cows of different genetic merit for fertility would respond differently to the P-AIE and P-TAI programs (Fig. 7a, b). We observed differences for some but not all outcomes, and for the most part the differences were the result of the different VWP and the insemination pattern caused by the synchronization protocols. Cows in the Hi-Fert group in the P-AIE treatment (Fig. 7a) had a greater pregnancy rate after calving which reduced time to pregnancy and had more cows pregnant by 100 DIM (66.8 vs 61.2%; P < 0.05). Earlier re-insemination because of the shorter VWP and more cows AI in estrus before NPD compensated for the lower first service P/AI than for the P-TAI group. Despite these early lactation benefits for the P-AIE treatment, the proportion of cows pregnant at 200 DIM was the same for both treatments (91.2 vs 91.3%; P > 0.05). On the other hand, for the Lo-Fert group, there was no difference in pregnancy rate (Fig. 7b) or cows pregnant for the P-AIE and P-TAI treatments by 100 (53.7 vs 47.8%; P > 0.05) or 200 DIM (85.6 vs 85.9%; P > 0.05). This was likely the result of a smaller percentage of cows AI in estrus (during the warm season only) and P/AI to first AIE services for the P-AIE program. Reduced fertility for AI in estrus meant that more cows needed re-insemination which offset the benefits of a shorter VWP. Collectively, our results indicated that the Hi- and Med-Fert groups had larger differences in pregnancy rate after calving than the Lo-Fert group when managed with the P-AIE or P-TAI treatment. Nevertheless, effects were only obvious in early lactation with either marginal or no differences after 100 DIM. Thus, if the choice of reproductive management program for primiparous lactating Holstein cows is based purely on reducing time to pregnancy after calving, cows of superior genetic merit for fertility (based on RI or alike) might benefit from programs like the P-AIE in the experiment of Sitko et al. (2023). Cows of inferior genetic merit for fertility could be managed with programs that prioritize either AI in estrus or TAI as no major differences in performance should be expected.
(a) Effect of treatments on time to pregnancy for the high-fertility group. The hazard of pregnancy was greater (P < 0.01) for the predominant AI in estrus (P-AIE) than the predominant timed AI (P-TAI) treatment (HR = 1.4, 95% CI 1.2–1.6). Mean and median days to pregnancy were 94 and 81 for the P-AIE treatment and 107 and 86 for the P-TAI treatment, respectively. (b) Effect of treatments on time to pregnancy for the low-fertility group. No difference was observed for the hazard ratio (HR) for time to pregnancy between the P-AIE and P-TAI treatment (HR = 1.1, 95% CI 1.0–1.3). Mean and median days to pregnancy were 106 and 92 for the P-AIE treatment and 114 and 100 for the P-TAI treatment, respectively. Adapted from Sitko et al. (2023).
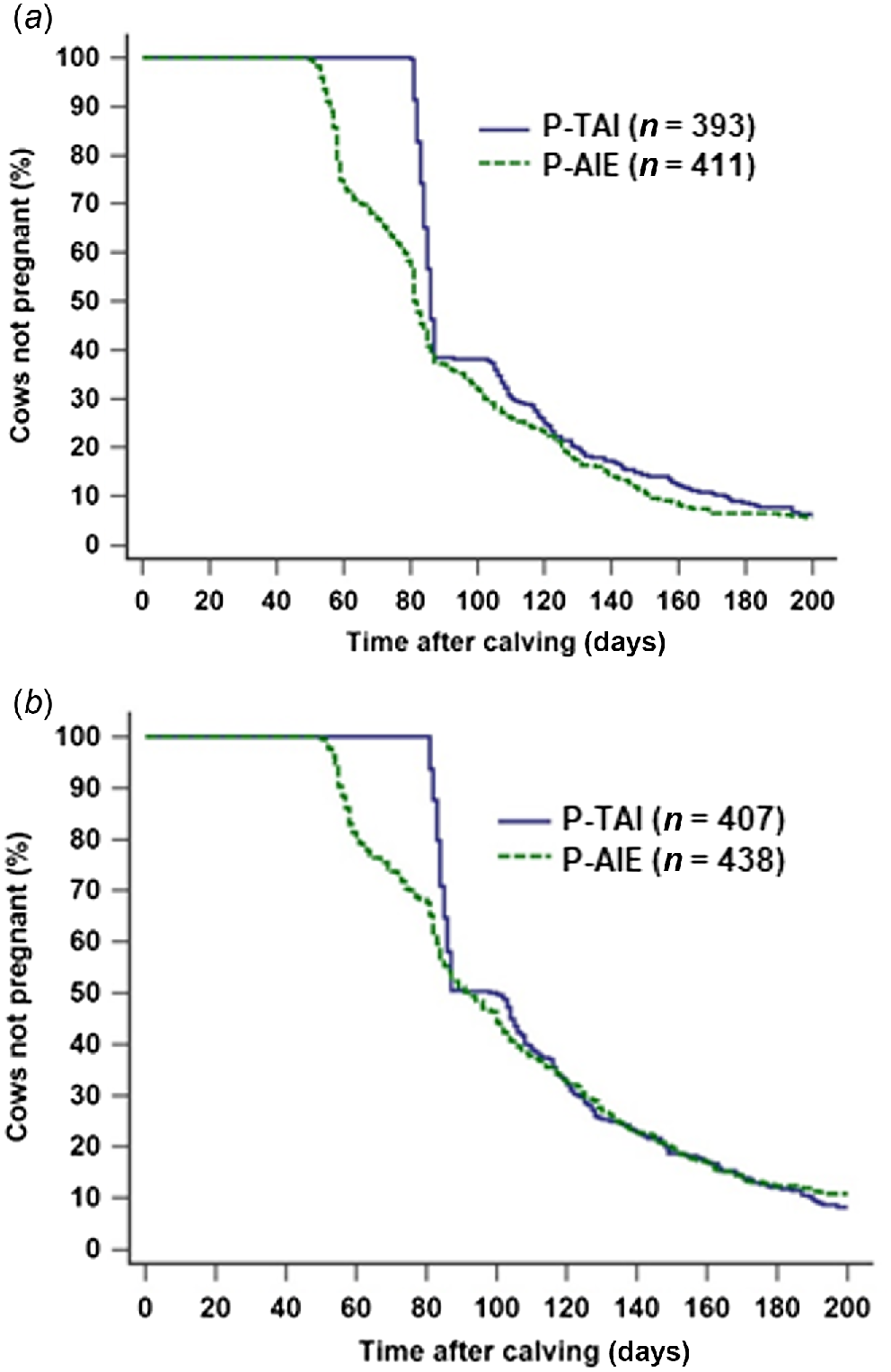
Thus, current evidence suggests that the effect of TRM based on genetic merit for fertility depends on the individual or sets of reproductive outcomes under consideration. The potential advantages of tailoring management based on genetic merit for fertility depend mostly on the value of inseminating more cows in estrus versus TAI, maximizing fertility, reducing, or increasing days to pregnancy, or a combination of all. Likewise, the value of TRM based on genetic merit for fertility for some farms might be more obvious for improving herd management practices rather than performance. For example, farms could simplify reproductive program implementation or reduce reliance on hormonal interventions while not compromising reproductive performance if cows of different genetic merit are targeted with programs that prioritize AIE or TAI. Ultimately, the choice of outcome or set of outcomes to optimize is farm dependent.
Automation of management tasks and decision-making
Due to the multiple tasks and decisions involved in reproductive management of dairy cattle, the opportunities for improving existing and expanding the suite of available technologies for monitoring and management and decision-making are extensive. To this end, automated or semiautomated tools for completing human-executed tasks and decision-making might facilitate adoption of reproductive management strategies that improve farm sustainability. These tools can help farms that lack the facilities, personnel, or frequent access to cows implement complex reproductive management programs that are currently out of their reach. For example, automating processes for reproductive monitoring and decision-making before cows become eligible for pregnancy could enable novel TRM strategies for first service. Integration of data from multiple PLF technologies that monitor behavior, physiology, and performance along with other herd management and environmental data into the dairy herd management software can be used to automatically generate groups of cows for TRM and track these cows through their respective programs for submission to AI or ET. Some early evidence of the value of combining multiple predictors for creating subgroups of cows with stark differences in performance, or predict the likelihood of reproductive outcomes at the cow level, are already available (Granados 2022; Rial and Giordano 2024). Moreover, the herd management software tools (e.g. DairyComp305, ValleyAg software; Bovisync; PCDart, DRMS) to assign cows to treatments has been available and used in the dairy industry for a long time. Once cows are eligible for pregnancy, automated detection of estrus and synchronization of ovulation can simplify submission of cows to AI or ET, reducing labor needs and cow manipulation while enhancing reproductive performance. For example, a reproductive monitoring management task that has been successfully automated is detection of estrus (Mayo et al. 2019; Schilkowsky et al. 2021). Today, evidence of the value of AEDS for herd management abounds (Rial et al. 2022; Gonzalez et al. 2023; Laplacette et al. 2024) and a myriad of AEDS are available and used by dairy farms. On the other hand, automated synchronization of ovulation is not yet possible, but several technologies might enable automation of this tedious management task. For example, the e-Synch system has been developed for integrating hormone delivery and sensing through an implantable intravaginal electronic device integrated with an IoT platform for programming and remote monitoring (Masello et al. 2020b; Ren et al. 2023a, 2023b). This device allows the user to select pre-established synchronization of ovulation protocols or customize a protocol by setting the type, dose, and time of hormone release. Once programmed to schedule hormone release, activated, and inserted in the vagina, hormones are released according to a selected protocol, or a customized sequence and hormone quantity selected by the user. Concomitantly, sensor data is broadcasted towards a gateway that uploads data to a cloud server for device monitoring and data visualization. In vivo experiments with dairy cows demonstrated that the current e-Synch system could be successfully programmed to deliver hormone solutions in a timely manner and cause luteal regression in response to PGF2α (Masello et al. 2020b) and a surge of LH in response to GnRH (Ren et al. 2023a). However, the device needs refinement and the ability of the system to fully synchronize ovulation must be demonstrated. Another technology under development that could automate or at least semi-automate synchronization of ovulation is a robotic arm for automating injections (Pharm Robotics, San Jacinto, CA, USA). Although this tool would not eliminate the need to sort cows for administering injections, labor needs and cow manipulation for synchronization of ovulation could be drastically reduced. Automated or semi-automated in-line milk (Bruinjé et al. 2017; Bruinjé et al. 2019) or cow-side tests (Mayo et al. 2016; Akköse 2023) for hormone determination and profiling are other technologies that could have substantial herd management and performance benefits. For example, a tool for fully automated hormone profiling based on in-line milk P4 monitoring already exists and is available for on-farm use in some parts of the world (Herd Navigator, DeLaval, Tumba, Sweden). This tool has been proven effective for determination of pregnancy status of lactating cows and for P4 profiling after calving (Bruinjé et al. 2019; Antanaitis et al. 2020). On the other hand, enzyme-linked immunosorbent assays, visual lateral flow assays, and lateral flow assays coupled with electronic readers have also become available and are effective for detecting and measuring reproductive hormones for pregnancy testing and ovarian status determination. Assays and tools are available for detecting and measuring pregnancy-associated glycoproteins (Mayo et al. 2016; Akköse 2023; Hussain et al. 2024; Rial et al. 2024) and P4 (Masello et al. 2020c). The potential value of tools for serial or single time hormone profiling is in helping implement TRM strategies for first service, hasten re-insemination, and detecting and monitoring pregnancy. These tools could also facilitate implementation of targeted hormonal and non-hormonal therapy after AI such as P4 supplementation during early embryo development (Monteiro et al. 2015).
Except for AEDS which have been validated in research and widely adopted by commercial farms, most of the other technologies for automating or semi-automating reproductive monitoring and management tasks are either in their infancy or have not yet been fully tested on commercial farms. Others are not yet widely adopted due to a series of technical limitations, cost, or other constraints. Therefore, extensive research and development must be conducted before the value of some of these technologies is fully realized. Special emphasis must be placed on developing tools that are effective and reliable, cost-effective, cow and user friendly, integrated with other on-farm technologies, and acceptable to consumers.
Conclusions
Data-driven technologies and management practices represent a growing opportunity for improving the sustainability of reproductive management programs for dairy cattle. As dairy farms strive to remain profitable, minimize environmental impacts, and adapt to growing societal pressures, data-driven and automated management can help improve herd performance while optimizing reproductive management tasks. A growing number of PLF technologies coupled with other herd management data, genomic information, and environmental monitoring tools are facilitating the development and implementation of data-driven TRM. A first step in the development of this novel concept to herd management is identifying data predictors to create subgroups of cows that can receive tailored management interventions. Therefore, significant research efforts have focused on understanding associations between putative predictors and reproductive outcomes. For example, based on the positive association between estrus during the VWP and reproductive potential, TRM programs based on AEA recorded during the VWP were developed. This type of strategy was shown to be as effective as traditional non-TRM programs that rely heavily on TAI. A better understanding of ovarian function after a previous insemination supported the development of TRM programs based on the ovarian status of cows at NPD. These programs were proven to reduce the interbreeding interval, increase fertility of subgroups of cows, and minimize unnecessary interventions for synchronization of ovulation and NPD. A better characterization of the association between genomic predictions for fertility and reproductive phenotypes enabled the development of TRM based on genomic predictions. In the future, unraveling some of the complex associations between data predictors and reproductive function will facilitate the development and implementation of TRM strategies that create subgroups of cows with larger differences in reproductive potential by combining data from several rather than single predictors.
Tools for fully automating or semi-automating management tasks and decision-making can also play a major role in helping dairy farms address sustainability issues. Much progress has been made with automating certain management tasks, such as detection of estrus and pregnancy testing, but progress for automating other tasks or adoption by commercial farms has been slower and more tedious. Major advances in engineering technology and Internet of Things (IoT) along with a better understanding of dairy cattle biology are needed for enabling the development of digital tools that improve automated dairy herd reproductive monitoring and management. Major efforts and coordination between researchers, dairy farms, and data-driven technology providers remain to further develop the technologies needed to realize the benefits of data-driven management strategies and automation of reproductive management programs.
Declaration of funding
This review did not receive any specific funding. The author’s work was supported by the USDA National Institute of Food and Agriculture (Washington, D.C.), Farm of the Future program Project # 2023-77038-38865, Animal Health Program Project # 2017-67015-26772, Animal Reproduction program Project # 2020-08769, Hatch project NYC-2020-21-255, and Multistate project 1021189. Research presented in this review was also partially supported by Zoetis Inc, Merck Animal Health, and SCR Engineers. Any opinions, findings, conclusions, or recommendations expressed in this publication are those of the author and do not necessarily reflect the view of the National Institute of Food and Agriculture (NIFA) or the United States Department of Agriculture (USDA).
References
Akköse M (2023) Comparative evaluation of two commercial pregnancy-associated glycoproteins tests for early detection of pregnancy in dairy cattle. Theriogenology 200, 11-17.
| Crossref | Google Scholar | PubMed |
Antanaitis R, Malašauskienė D, Televičius M, Juozaitienė V, Žilinskas H, Baumgartner W (2020) Dynamic changes in progesterone concentration in cows’ milk determined by the at-line milk analysis system herd navigator™. Sensors 20(18), 5020.
| Crossref | Google Scholar | PubMed |
Arbel R, Bigun Y, Ezra E, Sturman H, Hojman D (2001) The effect of extended calving intervals in high lactating cows on milk production and profitability. Journal of Dairy Science 84(3), 600-608.
| Crossref | Google Scholar | PubMed |
Borchardt S, Tippenhauer CM, Plenio J-L, Bartel A, Madureira AML, Cerri RLA, Heuwieser W (2021) Association of estrous expression detected by an automated activity monitoring system within 40 days in milk and reproductive performance of lactating Holstein cows. Journal of Dairy Science 104(8), 9195-9204.
| Crossref | Google Scholar | PubMed |
Bretzinger LF, Tippenhauer CM, Plenio J-L, Heuwieser W, Borchardt S (2023) Effect of transition cow health and estrous expression detected by an automated activity monitoring system within 60 days in milk on reproductive performance of lactating Holstein cows. Journal of Dairy Science 106(6), 4429-4442.
| Crossref | Google Scholar | PubMed |
Bruinjé TC, Colazo MG, Gobikrushanth M, Ambrose DJ (2017) Relationships among early postpartum luteal activity, parity, and insemination outcomes based on in-line milk progesterone profiles in Canadian Holstein cows. Theriogenology 100, 32-41.
| Crossref | Google Scholar | PubMed |
Bruinjé TC, Colazo MG, Ribeiro ES, Gobikrushanth M, Ambrose DJ (2019) Using in-line milk progesterone data to characterize parameters of luteal activity and their association with fertility in Holstein cows. Journal of Dairy Science 102(1), 780-798.
| Crossref | Google Scholar | PubMed |
Carvalho PD, Santos VG, Giordano JO, Wiltbank MC, Fricke PM (2018) Development of fertility programs to achieve high 21-day pregnancy rates in high-producing dairy cows. Theriogenology 114, 165-172.
| Crossref | Google Scholar | PubMed |
Carvalho MR, Peñagaricano F, Santos JEP, DeVries TJ, McBride BW, Ribeiro ES (2019) Long-term effects of postpartum clinical disease on milk production, reproduction, and culling of dairy cows. Journal of Dairy Science 102(12), 11701-11717.
| Crossref | Google Scholar | PubMed |
Chebel RC, Veronese A (2020) Associations between genomic merit for daughter pregnancy rate of Holstein cows and metabolites postpartum and estrus characteristics. Journal of Dairy Science 103(11), 10754-10768.
| Crossref | Google Scholar | PubMed |
De Vries A (2006) Economic value of pregnancy in dairy cattle. Journal of Dairy Science 89(10), 3876-3885.
| Crossref | Google Scholar |
Domingues RR, Andrade JPN, Cunha TO, Madureira G, Hoppman AS, Teixeira NN, Monteiro PLJ, Gomez-Leon VH, Martins JPN, Wiltbank MC (2024) Profiles of interferon-stimulated genes in multiple tissues and circulating pregnancy-associated glycoproteins and their association with pregnancy loss in dairy cowsdagger. Biology of Reproduction 110(3), 558-568.
| Crossref | Google Scholar | PubMed |
Fricke PM, Giordano JO, Valenza A, Lopes G, Jr., Amundson MC, Carvalho PD (2014) Reproductive performance of lactating dairy cows managed for first service using timed artificial insemination with or without detection of estrus using an activity-monitoring system. Journal of Dairy Science 97(5), 2771-2781.
| Crossref | Google Scholar | PubMed |
Giordano J (2019) Economic impact of reproductive performance in dairy herds and approaches for program selection. Clinical Theriogenology 11(3), 329-335.
| Google Scholar |
Giordano JO, Stangaferro ML, Wijma R, Chandler WC, Watters RD (2015) Reproductive performance of dairy cows managed with a program aimed at increasing insemination of cows in estrus based on increased physical activity and fertility of timed artificial inseminations. Journal of Dairy Science 98(4), 2488-2501.
| Crossref | Google Scholar | PubMed |
Giordano JO, Thomas MJ, Catucuamba G, Curler MD, Masello M, Stangaferro ML, Wijma R (2016) Reproductive management strategies to improve the fertility of cows with a suboptimal response to resynchronization of ovulation. Journal of Dairy Science 99(4), 2967-2978.
| Crossref | Google Scholar | PubMed |
Giordano JO, Sitko EM, Rial C, Pérez MM, Granados GE (2022) Symposium review: Use of multiple biological, management, and performance data for the design of targeted reproductive management strategies for dairy cows. Journal of Dairy Science 105(5), 4669-4678.
| Crossref | Google Scholar | PubMed |
Gonzalez TD, Factor L, Mirzaei A, Montevecchio AB, Casaro S, Merenda VR, Prim JG, Galvão KN, Bisinotto RS, Chebel RC (2023) Targeted reproductive management for lactating Holstein cows: reducing the reliance on exogenous reproductive hormones. Journal of Dairy Science 106(8), 5788-5804.
| Crossref | Google Scholar | PubMed |
Granados G (2022) Characterization of sensor and non-sensor cow, herd management, and environmental data and use of machine learning algorithms for prediction of pregnancy in dairy cattle. MS Thesis, Cornell University, Ithaca, New York, USA. Available at https://ecommons.cornell.edu/items/013fc983-8455-4edb-9c8a-e863d2dd1b88
Hussain I, Rial C, Boza J, Tompkins S, Branen J, Giordano J, Erickson D (2024) Design of a handheld and portable fluorescence imaging system for quantitative detection of pregnancy-specific biomarkers in cattle. Analytical and Bioanalytical Chemistry 416, 4101-4109.
| Crossref | Google Scholar | PubMed |
Laplacette AL, Rial C, Magaña Baños GS, García Escalera JA, Torres S, Kerwin A, Giordano JO (2024) Effect of a targeted reproductive management program based on automated detection of estrus during the voluntary waiting period on reproductive performance of lactating dairy cows. Theriogenology 225, 130-141.
| Crossref | Google Scholar | PubMed |
Liang D, Cabrera VE (2015) Optimizing productivity, herd structure, environmental performance, and profitability of dairy cattle herds. Journal of Dairy Science 98(4), 2812-2823.
| Crossref | Google Scholar | PubMed |
Lima FS, Silvestre FT, Peñagaricano F, Thatcher WW (2020) Early genomic prediction of daughter pregnancy rate is associated with improved reproductive performance in Holstein dairy cows. Journal of Dairy Science 103(4), 3312-3324.
| Crossref | Google Scholar | PubMed |
Madureira AML, Silper BF, Burnett TA, Polsky L, Cruppe LH, Veira DM, Vasconcelos JLM, Cerri RLA (2015) Factors affecting expression of estrus measured by activity monitors and conception risk of lactating dairy cows. Journal of Dairy Science 98(10), 7003-7014.
| Crossref | Google Scholar | PubMed |
Masello M, Ceglowski B, Thomas MJ, Giordano JO (2020a) A reproductive management program aimed at increasing reinsemination of nonpregnant dairy cows at detected estrus resulted in similar reproductive performance to a program that favored timed artificial insemination. Journal of Dairy Science 103(4), 3719-3729.
| Crossref | Google Scholar | PubMed |
Masello M, Ren Y, Erickson D, Giordano JO (2020b) An automated controlled-release device for intravaginal hormone delivery. JDS Communications 1(1), 15-20.
| Crossref | Google Scholar | PubMed |
Masello M, Lu Z, Erickson D, Gavalchin J, Giordano JO (2020c) A lateral flow-based portable platform for determination of reproductive status of cattle. Journal of Dairy Science 103(5), 4743-4753.
| Crossref | Google Scholar | PubMed |
Masello M, Perez MM, Granados GE, Stangaferro ML, Ceglowski B, Thomas MJ, Giordano JO (2021) Effect of reproductive management programs for first service on replacement dairy heifer economics. Journal of Dairy Science 104(1), 471-485.
| Crossref | Google Scholar | PubMed |
Mayo LM, Moore SG, Poock SE, Silvia WJ, Lucy MC (2016) Technical note: Validation of a chemical pregnancy test in dairy cows that uses whole blood, shortened incubation times, and visual readout. Journal of Dairy Science 99(9), 7634-7641.
| Crossref | Google Scholar | PubMed |
Mayo LM, Silvia WJ, Ray DL, Jones BW, Stone AE, Tsai IC, Clark JD, Bewley JM, Heersche G, Jr. (2019) Automated estrous detection using multiple commercial precision dairy monitoring technologies in synchronized dairy cows. Journal of Dairy Science 102(3), 2645-2656.
| Crossref | Google Scholar | PubMed |
Monteiro PLJ, Jr., Nascimento AB, Pontes GCS, Fernandes GO, Melo LF, Wiltbank MC, Sartori R (2015) Progesterone supplementation after ovulation: effects on corpus luteum function and on fertility of dairy cows subjected to AI or ET. Theriogenology 84(7), 1215-1224.
| Crossref | Google Scholar | PubMed |
Pérez MM, Wijma R, Scarbolo M, Cabrera E, Sosa F, Sitko EM, Giordano JO (2020) Lactating dairy cows managed for second and greater artificial insemination services with the short-resynch or day 25 resynch program had similar reproductive performance. Journal of Dairy Science 103(11), 10769-10783.
| Crossref | Google Scholar | PubMed |
Pinedo P, Santos JEP, Chebel RC, Galvão KN, Schuenemann GM, Bicalho RC, Gilbert RO, Rodriguez-Zas SL, Seabury CM, Rosa G, Thatcher W (2020) Associations of reproductive indices with fertility outcomes, milk yield, and survival in Holstein cows. Journal of Dairy Science 103(7), 6647-6660.
| Crossref | Google Scholar | PubMed |
Ren Y, Duhatschek D, Bartolomeu CC, Laplacette AL, Perez MM, Rial C, Erickson D, Giordano JO (2023a) An automated system for cattle reproductive management under the IoT framework. Part II: induction of luteinizing hormone release after gonadotropin releasing hormone analogue delivery with e-Synch. Frontiers in Animal Science 4, 1093857.
| Crossref | Google Scholar |
Ren Y, Duhatschek D, Bartolomeu CC, Erickson D, Giordano JO (2023b) An automated system for cattle reproductive management under the IoT framework. Part I: the e-Synch system and cow responses. Frontiers in Animal Science 4, 1093851.
| Crossref | Google Scholar |
Rial C, Giordano JO (2024) Combining reproductive outcomes predictors and automated estrus alerts recorded during the voluntary waiting period identified subgroups of cows with different reproductive performance potential. Journal of Dairy Science 107, 7299-7316.
| Crossref | Google Scholar | PubMed |
Rial C, Laplacette A, Giordano JO (2022) Effect of a targeted reproductive management program designed to prioritize insemination at detected estrus and optimize time to insemination on the reproductive performance of lactating dairy cows. Journal of Dairy Science 105(10), 8411-8425.
| Crossref | Google Scholar | PubMed |
Rial C, Hussain I, Hoff R, Tompkins S, Erickson D, Branen J, Giordano JO (2024) Development and evaluation of a lateral flow-based portable optical system for determination of the pregnancy status of dairy cows. Journal of Dairy Science 107(10), 8642-8653.
| Crossref | Google Scholar |
Ricci A, Carvalho PD, Amundson MC, Fricke PM (2017) Characterization of luteal dynamics in lactating Holstein cows for 32 days after synchronization of ovulation and timed artificial insemination. Journal of Dairy Science 100(12), 9851-9860.
| Crossref | Google Scholar | PubMed |
Schilkowsky EM, Granados GE, Sitko EM, Masello M, Perez MM, Giordano JO (2021) Evaluation and characterization of estrus alerts and behavioral parameters generated by an ear-attached accelerometer-based system for automated detection of estrus. Journal of Dairy Science 104(5), 6222-6237.
| Crossref | Google Scholar | PubMed |
Sitko EM, Di Croce FA, McNeel AK, Weigel DJ, Giordano JO (2023) Effect of reproductive management programs that prioritized artificial insemination at detected estrus or timed artificial insemination on the economic performance of primiparous Holstein cows of different genetic merit for fertility. Journal of Dairy Science 106(9), 6495-6514.
| Crossref | Google Scholar | PubMed |
Stangaferro ML, Wijma R, Masello M, Thomas MJ, Giordano JO (2018a) Economic performance of lactating dairy cows submitted for first service timed artificial insemination after a voluntary waiting period of 60 or 88 days. Journal of Dairy Science 101(8), 7500-7516.
| Crossref | Google Scholar | PubMed |
Stangaferro ML, Wijma R, Masello M, Thomas MJ, Giordano JO (2018b) Extending the duration of the voluntary waiting period from 60 to 88 days in cows that received timed artificial insemination after the double-ovsynch protocol affected the reproductive performance, herd exit dynamics, and lactation performance of dairy cows. Journal of Dairy Science 101(1), 717-735.
| Crossref | Google Scholar | PubMed |
von Keyserlingk MAG, Martin NP, Kebreab E, Knowlton KF, Grant RJ, Stephenson M, Sniffen CJ, Harner JP, III, Wright AD, Smith SI (2013) Invited review: Sustainability of the US dairy industry. Journal of Dairy Science 96(9), 5405-5425.
| Crossref | Google Scholar | PubMed |
Wijma R, Stangaferro ML, Kamat MM, Vasudevan S, Ott TL, Giordano JO (2016) Embryo mortality around the period of maintenance of the corpus luteum causes alterations to the ovarian function of lactating dairy cows. Biology of Reproduction 95(5), 112.
| Crossref | Google Scholar | PubMed |
Wijma R, Stangaferro ML, Masello M, Granados GE, Giordano JO (2017) Resynchronization of ovulation protocols for dairy cows including or not including gonadotropin-releasing hormone to induce a new follicular wave: effects on re-insemination pattern, ovarian responses, and pregnancy outcomes. Journal of Dairy Science 100(9), 7613-7625.
| Crossref | Google Scholar | PubMed |
Wijma R, Pérez MM, Masello M, Stangaferro ML, Giordano JO (2018) A resynchronization of ovulation program based on ovarian structures present at nonpregnancy diagnosis reduced time to pregnancy in lactating dairy cows. Journal of Dairy Science 101(2), 1697-1707.
| Crossref | Google Scholar | PubMed |