A targeted study to determine the conservation status of a Data Deficient montane lizard, the Eungella shadeskink (Saproscincus eungellensis)
Nicholas A. Scott A # , Jordan Mulder A # , Arman N. Pili A B , Paul M. Oliver

A
B
C
D
E
# These authors contributed equally to this paper.
§ Joint senior author.
Handling Editor: Dan Lunney
Abstract
Combatting biodiversity loss is often hamstrung by a lack of species-specific knowledge. Species considered Data Deficient (DD) on the IUCN Red List are poorly understood and often neglected in conservation investment, despite evidence they are often threatened. Reptiles have the highest percentage of DD species for any terrestrial vertebrate group.
We aimed to assess the conservation status of the DD Eungella shadeskink (Saproscincus eungellensis), which is endemic to Eungella National Park, Queensland, Australia.
A combination of a targeted field survey, ecological studies, and species distribution modelling were used.
Saproscincus eungellensis typically occurred within 25 m of streams, at elevations between 700 and 1000 m. The species is thigmothermic, with a low active body temperature (~23–26°C) and was predominantly observed on rocks and fallen palm fronds. The species has a highly restricted distribution with an estimated Area of Occupancy of 36 km2 and Extent of Occurrence of 81.7 km2, comprising one location (defined by the threat of climate change) with an estimated 16,352–52,892 mature individuals. The main threats are fire, invasive alien species and climate change, with the species forecast to lose all suitable habitat by 2080 under all climate change scenarios.
The species meets listing criteria for Critically Endangered under Criterion B of the International Union for Conservation of Nature.
Our results support recent studies indicating that some DD species are highly threatened. Our approach provides a template for conducting targeted studies to determine the conservation status of DD species, especially those with restricted ranges.
Keywords: climate change, Eungella National Park, extinction risk, fire, invasive species, IUCN Red List, Scincidae, SDM, species distributional modelling.
Introduction
The Data Deficient (DD) category of the International Union for Conservation of Nature (IUCN) Red List is considered the most controversial and misunderstood (Butchart and Bird 2010). The IUCN discourages the liberal use of this category, and recommends that Data Deficient species receive the same research attention as species listed as threatened (Morais et al. 2013; Bland 2014). However, in practice this is rarely followed, with conservation investment, planning and assessments generally neglecting Data Deficient species (Jarić et al. 2016; Bland et al. 2017). Alarmingly, studies have shown that numerous DD species may be more threatened than data sufficient species (Parsons 2016; González-del-Pliego et al. 2019; Gumbs et al. 2020; Borgelt et al. 2022). Many governments consider the DD category similar to the Least Concern category, effectively placing these species out of sight and out of mind (Parsons 2016; Braby 2018). However, if a key aim of conservation is to protect species from declines or extinction, we need to be proactive, rather than reactive, and take steps towards early identification of threats, declines in abundance, and contracting distributions (Lindenmayer et al. 2011; Martin et al. 2012). Given the large number of species listed as DD, it is increasingly important to prioritise research to remove them from this problematic category (Jarić et al. 2016).
Globally, high-elevation ecosystems, and especially montane forest biomes in tropical and subtropical areas, are of high conservation concern. In these areas, the rapid compression of climatic zones and habitats over elevational gradients have catalysed speciation, and ultimately, resulted in high species richness and endemism (Williams et al. 2003; Ashton et al. 2016). Species in these biomes often exhibit altitudinal stratification and very small ranges due to their thermal adaptations (Ashton et al. 2016; Hamilton et al. 2021). Such specialist species tend to have increased extinction risk, with a small population size and a restricted geographical range causing a synergistic double jeopardy (Colles et al. 2009; Williams et al. 2009). In the face of warming climates, mobile species specialising in high-elevation biomes may adjust their ranges through migration to neighbouring cooler latitudes. However, lowland warmer, and often drier, habitats separating mountains can severely restrict species immigration, especially for species that also have limited mobility (Laurance 2015; Ashton et al. 2016). Where mountains are isolated, low mobility species can typically only respond to warming by moving up in elevation (Peters and Darling 1985). For species restricted to the tops of mountains, this is expected to result in progressively smaller ranges over time, until the required climatic envelopes reach the elevational limits and extinction follows (Colwell et al. 2008; Román-Palacios and Wiens 2020). Encroachment of lower-elevational predators, competitors, and pathogens may also increase extinction risk (Walther et al. 2002; Seastedt and Oldfather 2021).
Montane species in regions with relatively low topographic relief, such as Australia, have few options to adjust their ranges in response to climate change (Hughes 2011; Torkkola et al. 2022a, 2022b). Australia is the world’s flattest continent (Ebach and Michaux 2020) with the lowest mean elevational gradient and 99% of its land area under 1000 m altitude (Hughes 2011). Nonetheless, montane biomes across the continent have high levels of endemic and specialist species, all of which have been predicted to be at a particularly high extinction risk (Williams et al. 2003; Hughes 2011; Hagger et al. 2013; Torkkola et al. 2022a).
Reptiles are the most species-rich group of terrestrial vertebrates globally (Cox et al. 2022; Meiri et al. 2023). However, their conservation status is more poorly understood than that of other terrestrial vertebrate taxa, with reptiles holding both the highest percentage and highest raw numbers of DD classified species on the IUCN Red List (Cox et al. 2022; Meiri et al. 2023). Australia is a global hotspot of reptile diversity (holding ~10% of the world’s lizards and snakes [vs 5% of the world’s land area]; Tingley et al. 2019), including an exceptional diversity of skinks (~460 species) (Squamata: Scincidae; Wilson and Swan 2020; (IUCN 2024a). Many Australian skinks are listed as DD (17 of 444 assessed species) and have not been studied since their description (Chapple et al. 2019), highlighting significant knowledge gaps in skink ecology (e.g. population sizes, geographical ranges, trends, and threats; Chapple et al. 2019). With current trends in biodiversity loss Australia stands to lose significant proportions of skink diversity (Cox et al. 2022; Graham et al. 2023) and Chapple et al. (2021) found that 20% of skink species globally are threatened by extinction. Recent field-based studies have revealed three previously DD Australian skink species are threatened (DCCEEW 2023a, 2023b; Farquhar et al. 2023a; Graham et al. 2023; L. Bonifacio, J. E. Farquhar, A. Pili, J. Walsh, D. G. Chapple, unpubl. data). Thus, urgent targeted field research is required on other DD Australian skink species to reveal their true conservation status.
The Eungella shadeskink (Scincidae: Saproscincus eungellensis, Sadlier et al. 2005) (Fig. 1) is restricted to high elevations in the Clarke Range, with all but one observation from the Dalrymple Heights area within Eungella National Park (herein Eungella NP) of central-eastern Queensland (Fig. 2). It was described in 2005 (Sadlier et al. 2005) from 14 voucher specimens with limited ecological data, although an association with upland rainforest streams was apparent. The species has not received any targeted research effort since, although it was sporadically recorded during frog surveys reported by Meyer et al. (2020) (H. B. Hines, unpubl. data), including the only additional specimen collected since its description. Thus, it was assessed as DD by the IUCN Red List (Hoskin et al. 2018) and is not listed under the federal Environment Protection and Biodiversity Conservation Act 1999 (EPBC Act). It is listed as Vulnerable under the Queensland Nature Conservation Act (1992). It shows traits that are positively correlated with extinction risk (e.g. narrow-range, high altitude) and lives in an area impacted by multiple threats (Sadlier et al. 2005; Hines et al. 2020), raising the possibility that it is threatened, with Sadlier et al. (2005) and Wotherspoon et al. (2024) considering it to meet IUCN Red List criteria for Endangered.
The geographical range of Saproscincus eungellensis within the Eungella study area (using unvetted ALA (Atlas of Living Australia) 2023 records), prior to our study, relative to Queensland, Australia.
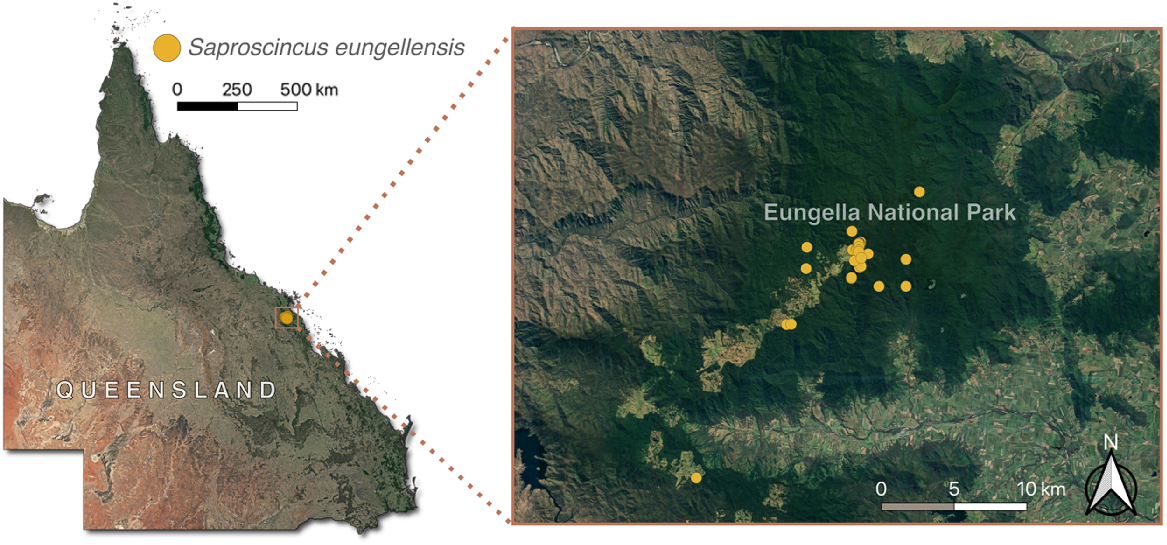
Climate change and the associated increased risk of severe drought, heatwaves and fire (Climate Change in Australia 2020) are likely to pose significant conservation risk to S. eungellensis. The species has limited dispersal capabilities, and the nearest climatically similar habitats are hundreds of kilometres away. They occur close to the maximum elevation of Eungella NP (1250 m a.s.l.) raising the possibility that changing climate may leave the species with no available habitat (Sadlier et al. 2005; Ashton et al. 2016). Further, closely related Saproscincus species display low critical thermal maxima (CTMax) compared to other Australian skink species, suggesting a high vulnerability to increasing temperatures or loss of cool rainforest microhabitats (Table 1; Greer 1980, 1989; Sadlier et al. 2005; Muñoz et al. 2016). Severe drought leading to extreme fire has also already extensively impacted fire-sensitive rainforests at Eungella NP (Hines et al. 2020).
Species | CTMax (°C) | Source | |
---|---|---|---|
Saproscincus hannahae | 35.5 | Greer (1980) | |
Saproscincus mustelinus | 38.6 | Greer (1980) | |
Saproscincus tetradactylus | 35.52 | Muñoz et al. (2016) | |
Saproscincus czechurai | 37.75 | Muñoz et al. (2016) | |
Saproscincus basiliscus | 38.04 | Muñoz et al. (2016) | |
Carlia rubigularis | 43.31 | Muñoz et al. (2016) | |
Gnypetoscicnus queenslandiae | 36.85 | Muñoz et al. (2016) | |
Lampropholis similis | 42.57 | Muñoz et al. (2016) | |
Lampropholis bellendenkerensis | 43.05 | Muñoz et al. (2016) |
Here we conduct a targeted field study and niche modelling (e.g. Le Breton et al. 2019) for S. eungellensis in order to better understand its distribution, thermal requirements and conservation status. Specifically, we aim to (1) determine the current geographical distribution (i.e. Extent of Occurrence [EOO], Area of Occupancy [AOO]), and elevational limits of S. eungellensis; (2) estimate population size, (3) better understand the species’ ecology and thermal biology; (4) model potential future distribution under various climate scenarios; (5) evaluate known and potential threats; and (6) assess conservation status using the IUCN Red List Guidelines.
Materials and methods
Field surveys to determine the habitat preferences of S. eungellensis
To assess the influence of time of day, temperature, and solar radiation on the activity times of S. eungellensis, we conducted systematic visual surveys (n = 56) from 18 November to 6 December 2023. Four 150 m long transects were established along two high-elevation tributaries of Cattle Creek (900–1000 m a.s.l.) at Eungella NP known to support the species (Supplementary material Fig. S1). We conducted 60 min visual surveys along these transects during four time windows (09:00–10:00, 11:00–12:00, 13:00–14:00, 15:00–16:00 hours AEST). Each site was surveyed on three separate occasions for every time window (i.e. a total of 12 surveys per site). The order of surveys was randomised with respect to site and time window. Active searches involved two researchers slowly traversing the transects in both directions and visually scanning habitat for lizards. Once detected, the time and location of each lizard observation was recorded on EcoGIS (iPhone app, UgMedia). For each S. eungellensis observation, microhabitat temperature was recorded using a digital thermometer (Omega HH508 digital thermometer, Omega Engineering Inc.) with a K-type thermocouple probe placed approximately 5 cm above the substrate the lizard was observed on. The temperature of the substrate at occupied sites (Tsurf) and the maximum temperature (TsurfMax) of substrate found within a 1 m radius of the lizard was recorded using an infrared temperature gun (Fluke 566 IR Thermometer). To assess the species’ relationship to open water, distance from water was recorded (in m) for each individual using a tape measure. Microhabitat preference was recorded as the perch substrate the lizard was first observed on (wood = fallen coarse woody debris, rock, litter, palm = fallen palm frond, flood debris).
Saproscincus eungellensis individuals were hand captured where possible, and field-selected body temperatures (Tb) were recorded within 10 s of capture using a digital thermometer with a K-type thermocouple probe inserted approximately 5 mm into the cloaca. Sex was determined through eversion of hemipenes in males and inspection of females for obvious signs of gravidness. Once caught, the following linear morphological variables were measured using digital callipers (Mituyo 0–200 mm) and a ruler: snout–vent length (SVL), head length, head width, head depth and tail length. Upon each unique capture, lizards were marked on each flank using non-toxic white paint (Born Inc. acrylic paint) to allow re-captured animals to be recorded through the study period. Life stage (juvenile, adult), and lizard colour (light, dark) was also recorded for all observations.
In addition to these four creek-line survey sites we conducted surveys at four transects ~30 m upslope (Fig. S1) from each of them (for a total of eight transects) to assess the species’ use of denser rainforest habitat at short distances from its assumed preferred creek habitat. These surveys followed similar survey procedures as the creek line sites (150 m transect at each site, surveyed by two researchers for 60 min) except that these rainforest sites were surveyed on only two occasions each, in the two time windows that lizards were found to be most active (11:00–12:00, 13:00–14:00 hours AEST).
To quantify solar radiation experienced during surveys a solar radiation pyranometer (SR05-A1 solar meter, Hukseflux Inc.) and data logger (LI19 handheld data logger, Hukseflux Inc.) were deployed in a standard position in every transect during each survey and set to record incident solar energy (W/m2) for every minute of each survey period (Fig. S2).
Distributional surveys to determine elevational extent and habitat associations
To clarify the distributional and elevational extent of S. eungellensis in Eungella NP we conducted timed visual surveys (n = 6) from the 7 December to 15 December 2023. We undertook 4–5 h visual surveys between 09:00 and 16:00 hours AEST along each of the following creek lines: Cattle Creek high elevation (800–920 m a.s.l), Cattle Creek low elevation (100–200 m a.s.l), Cattle Creek mid elevation (620–750 m and 620–590 m a.s.l) and Broken River (690–740 m a.s.l) (a catchment with a single previous non-specimen backed record of the species – Sadlier et al. 2005). Active searches involved two researchers traversing the creek lines scanning habitat for lizards. If a S. eungellensis was observed, thermal and morphological data were taken as described above. We also included any additional records of S. eungellensis detected incidentally when traversing to/from sites.
Microhabitat use and thermal biology
At each of the survey sites, vegetation density was measured vertically using the touch pole method (e.g. Lees et al. 2022) with a 1.8 m touch pole separated into three height brackets (0–50, 51–100, 101–180 cm). Every 10 m along each transect the touch pole was stuck into the ground and all vegetation touches for each height bracket were recorded. No attempt was made to distinguish between live and dead plant matter (Godínez-Alvarez et al. 2009). The substrate type (litter, rock, water, bare soil, wood, palm, or flood debris) beneath the pole at each position was recorded (Godínez-Alvarez et al. 2009). At each 10 m intercept we also sampled at three points either side of, and at right angles to, the transect line at 1 m intervals, making a total of seven touches/intercept and 105 touches/site. To quantify canopy openness every 20 m along the transect a photo was taken of the canopy from a height of approximately 140 cm and analysed using Percentage Cover (iPhone app, Mark Mignanelli). The canopy cover percentages were then averaged across the transect.
Quantifying thermal regimes of habitat
We deployed 32 iButton temperature data loggers (Thermocrom DS1921G, Maxim, San Jose, USA) set to record hourly ambient air and refuge temperatures (Ta ± 0.5°C) from 19 November to 15 December 2023. At each transect (n = 8), four iButtons were deployed, each in a zip-lock bag, which was then taped to the end of a thin wooden stake. For air temperature the stake with the iButton approximately 40 cm above the ground was inserted in the creek line near where lizards had been observed (see Fig. S3). Within 0.5–5 m, a second iButton was inserted into a potential refuge (in a rock crevice, beneath a boulder, or under a log). This paired deployment was repeated at a second location on the transect approximately 100 m away. As Saproscincus species are primarily diurnally active, only daytime temperatures (07:00–17:00 hours AEST) were considered for analysis.
To quantify spatial variation in thermal environments within the Cattle Creek catchment of Eungella NP we similarly deployed eight iButtons across two lower elevation tributaries (elevation 270 m and 735 m) from 25 November to 12 December 2023. At each site, two paired air-refuge iButton stations were established approximately 200 m apart along the creek line. Sites were chosen based on having similar characteristics to the higher elevation tributaries of Cattle Creek (i.e. rainforest, rocky creek).
Statistical analyses
All analyses were conducted in the R statistical environment (2023.12.1 + 402; R Core Team 2023) unless stated otherwise. A principal component analysis (PCA) was performed using the packages FactorMineR (v2.9; Lê et al. 2008) and factoextra (v1.0.7; Kassambara and Mundt 2020) to visualise patterns of habitat change between the sites (creek and rainforest). Transect touch counts of palm and flood debris were excluded from the model due to infrequent occurrence (<30 observations out of a total of 2381). PC loadings were inspected and the Kaiser-Guttman criterion (eigenvalue >1) was used to determine PCs for further analysis (Jackson 1993). We used permutational multivariate analysis of variance (PERMANOVA; Anderson 2017) with the package vegan (R Core Team 2023) on the scores of PC1 to assess differences in habitat characteristics between the sites. Euclidean distance in the PERMANOVA analysis was used, as many habitat characteristics were correlated with each other (Grady et al. 2013).
To assess which variables affect Tb, we applied a linear mixed effect model where Tb was the response variable, survey number was used as the random factor, and SVL, mass, Tsurf, TsurfMax, air temperature, average refuge temperature and solar energy were considered as fixed effects (Sannolo et al. 2014; Ortega and Pérez-Mellado 2016). A saturated model with all potential explanatory variables was considered first. Akaike Information Criterion (AIC; Burnham and Anderson 2004) was then used to remove non-significant variables and interactions (P > 0.05) in a stepwise procedure (Ortega and Pérez-Mellado 2016). The simplest significant model was then chosen to explain the observed active body temperatures of S. eungellensis. We tested whether S. eungellensis showed between-sex differences in morphological variables by conducting a series of one-way ANOVAs using each morphological feature as dependent variables and sex as the factor with the car (Companion to Applied Regression) package. Prior to analysis, parametric assumptions were tested and, where morphological variables deviated too far due to small sample sizes, a Wilcoxon rank sum test was used instead.
To determine if ambient temperatures in the air and in refuges differed across or among sites or elevations, t-tests were conducted using the car (Fox and Weisberg 2019) package.
The AOO and EOO of S. eungellensis were estimated using the Geospatial Conservation Assessment Tool (GeoCAT; Bachman et al. 2011). Existing records were downloaded from the Atlas of Living Australia (ALA (Atlas of Living Australia) 2023), and vetted for locational accuracy and combined with unpublished records from the frog survey dataset of Meyer et al. (2020) and our 2023 field surveys (Fig. S4). GeoCAT estimates the EOO by calculating a convex hull defined as the smallest polygon in which no internal angle exceeds 180° and contains all sites of occurrence, and the AOO by calculating the sum of 2 km2 grid cells containing the sites of occurrence (Bachman and Moat 2012).
Population size was estimated using QGIS (QGIS Development Team 2022). As our study confirmed that S. eungellensis is highly dependent upon upland rainforests (>700 m a.s.l), we created a shapefile of drainage lines above 700 m asl, buffered by 10 m. KML files containing wetland information were downloaded from the Queensland Government website (Department of Environment, Science and Innovation, Queensland 2024a, 2024b) and converted into a shapefile. Drainages were clipped to the NP boundary, as this excludes cleared and heavily disturbed areas outside the park where the species is not known and unlikely to occur. As our quantitative assessment showed a marked increase in relative abundance of S. eungellensis above 950 m we stratified buffered streams in two groups, those above 950 m and those between 700 and 950 m. The area of the buffered creek lines for both shape files was then calculated. The number of mature individuals was calculated using the following IUCN endorsed formula: d × A × p, where d is an index of population density, A is an estimate of area and p represents the proportion of individuals that are mature (Table 2; IUCN 2024b). Using the highest and lowest number of S. eungellensis observed at the creek line survey sites (>950 m a.s.l.), we calculated a high and low range population estimate. For the 700–950 m a.s.l range, we calculated only one estimate because of the low number of individuals observed in this elevational range. We then used the number of S. eungellensis found during our surveys to determine the density for this area using a simple equation: we calculated the estimate based on the assumptions that (1) S. eungellensis occur in all creek lines above 700 m in Eungella NP, (2) species density differs between the two elevation bands, and (3) the species’ core habitat is restricted to creek lines.
Species distribution modelling
We predicted the potential distribution of S. eungellensis using the Maximum Entropy species distribution modelling algorithm (MaxEnt, Phillips et al. 2006, adapted from Farquhar et al. (2023a); refer to Figs S5 and S6 for the modelling background). We nominated a suite of 27 prospective predictor variables (Supplementary Table S1). Because this species is an ectotherm, and is likely highly affected by temperature and precipitation regimes (Powney et al. 2010; Farquhar et al. 2023a), we therefore primarily selected bioclimatic variables. The environmental predictor variables were resampled from a spatial resolution of ~1 km2 to match the resolution of the environmental layers using the mask function in the raster package (Hijmans et al. 2005).
To minimise multicollinearity between predictor variables, we inspected the relationship of the predictor variables through principal components (PC; Fig. S7) and pair-wise Pearson correlation coefficients. We retained variables with the likely greatest ecological relevance for S. eungellensis with a Pearson correlation coefficient <0.7: Bio1 = Annual mean temperature (°C); Bio6 = Minimum temperature of coldest month (°C); Bio7 = Annual temperature range (°C); Bio12 = Annual precipitation (mm); Euclidean distance to watercourses (Fig. S7).
To compensate for uneven sampling effort (both current surveys and historical records) across the modelling region and spatial non-independence of species occurrence points, we reduced spatial clustering by thinning S. eungellensis records so there were no records less than 1 km distant from each other using the thin function of the R package spThin (Aiello-Lammens et al. 2015), resulting in 12 occurrence points. Due to the small extent of the Eungella study area, we then sampled 1150 background points weighted against the likelihood density values of the occurrence points. Thus, background data points were selected with approximately the same sampling bias as the occurrence points (Kramer-Schadt et al. 2013; Farquhar et al. 2023a).
MaxEnt model calibration was run using ENMevaluate function of the ENMeval package (v2.0.3; Muscarella et al. 2014; Kass et al. 2021) which runs MaxEnt models across multiple combinations of feature classes and values of the regularisation multiplier to allow comparisons of model performance. We selected the MaxEnt combination that balanced model fit and predictive ability. Models were built with regularisation multiplier options set to 1, 2, and 3 and with three varying feature class combinations (1. Linear; 2. Quadratic; 3. Linear + Quadratic); resulting in nine parameter combinations.
The MaxEnt models were evaluated using metrics AICc, AUCTEST, AUCDIFF, ORMTP, OM10 and Boyce Index (see Table S2 for definitions and explanations of metrics). We visually inspected the predictions of each model and evaluated the validity of each model with our knowledge of the ecology of S. eungellensis and of the Eungella region. We chose the parameter combination that returned an optimal MaxEnt model – that is, the parameter produced a model that performed well against multiple metrics and generated ecologically plausible predictions for S. eungellensis (see Farquhar et al. 2023a for full modelling approach). The model output was used to produce a map of potentially suitable pre-clearing habitat.
Projecting future suitable habitats
To assess the potential effects of future climate change on the distribution of S. eungellensis, we forecast pre-clearing habitat suitability, under different climate change scenarios using the Coupled 106 Model Intercomparison Project Phase 6 (CMIP6) multi-model (Eyring et al. 2016; Meinshausen et al. 2020; Masson-Delmotte et al. (2021)) and Euro-Mediterranean Centre on Climate Change coupled climate model CMCC-CM2, (Cherchi et al. 2019; Ortega-Andrade et al. 2021) because it falls within the mean of the suite of climate models (Grose et al. 2023). The CMCC-CM2 models can also reproduce the main patterns of future temperature and precipitation regimes at a very high spatial resolution (Scoccimarro et al. 2021).
CMIP6 uses future emission scenarios, known as shared socioeconomic pathways (SSPs), that consider the expected socio-economic, technological growth and associated greenhouse gas emissions in the scenarios of future climatic conditions (Fan et al. 2020; HamadAmin and Khwarahm 2023). To characterise future climate conditions, we obtained bioclimatic data projected under two IPCC concentration pathways emissions scenarios, SSP2.45 (optimistic) and SSP3.70 (high middle) (HamadAmin and Khwarahm 2023). SSP1.45 was excluded from the model as emissions have already exceeded this scenario (Hausfather and Peters 2020; Nazarenko et al. 2022). For the Euclidean distance to watercourses, we used the present-day data for all future projections under the premise that these geological features are not likely to change significantly within the next 80 years.
Using the optimal MaxEnt model described above, we predicted S. eungellensis habitat suitability across the Eungella study area for SSP2.45 and SSP3.70 scenarios across four time periods (2040, 2060, 2080, 2100). Maps of predicted future pre-clearing habitat suitability were created using QGIS (QGIS Development Team 2022). To estimate area of potentially suitable habitat, binary predictions for each scenario and time period were created using a threshold that maximises sensitivity and specificity (Muñoz et al. 2022; Torkkola et al. 2022a).
Ethical standards
This research was conducted in accordance with the Queensland state wildlife permits issues by the Department of Environment and Science (within protected areas: P-PTUKI-100483094, P-PTC-100483098, P-SPP-100483101, and non-protected areas: WA0056270) and a Monash University Animal Ethics Committee approval (Approval number: 39971).
Results
Individuals observed
We detected 103 S. eungellensis individuals during the survey period, comprising 49 adults (22 female, 9 male, 18 indeterminant), 51 juveniles and 3 indeterminant. A total of 56 individuals were captured allowing for measurements of morphology and body temperature. Adult males (n = 9) were slightly, but significantly, larger than adult females (n = 22) for mass, tail length, head length and head width (Table S3 and S4).
Thermal biology
Saproscincus eungellensis displayed low active body temperatures (n = 56, ~23–26°C; Fig. 3). The mean Tb of the species was higher than the mean of all the environmental variables (22–23°C) but was lower than the observed maximum values of Tsurf and TsurfMax (Table S5). Model V performed best out of the tested models (Table 3). According to this optimal model, Tsurf (P = 0.02348) had a significant positive effect on the Tb of S. eungellensis, whereas average potential refuge temperature (P = 0.04847) had a significant negative effect (Table 4). Additionally, TsurfMax (P = 0.07772) had a marginally significant positive effect (Table 4). Both air temperature (°C) and solar radiation (W/m2) had insignificant effects on lizard Tb and were removed in the step wise process (Table 3).
Bar chart showing distribution of observed Saproscincus eungellensis field active body temperatures (Tb; °C).
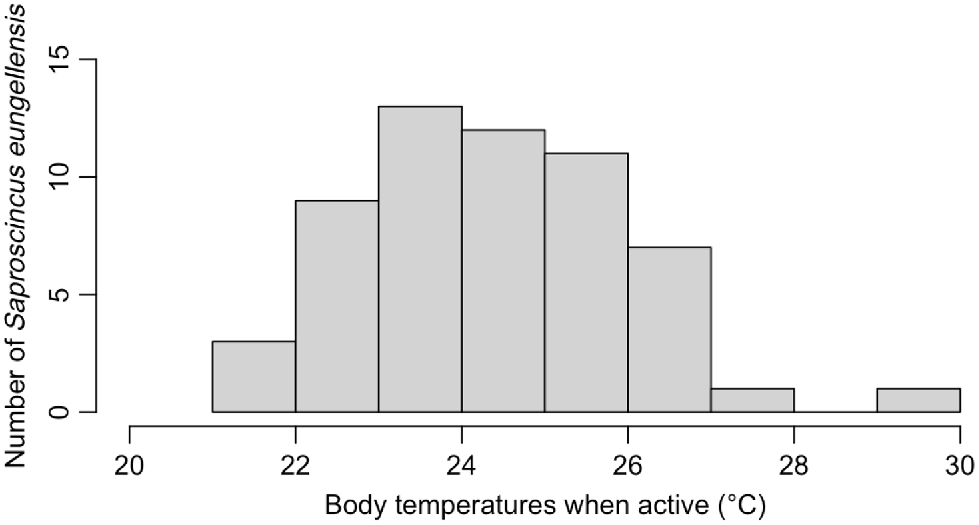
Model | AIC | BIC | d.f. | |
---|---|---|---|---|
M I. Saturated model with all predictor variables | 133.04 | 148.3 | 26 | |
M II. Model excluding air temperature | 129.56 | 143.3 | 27 | |
M III. Model excluding mass, air temperature | 128.56 | 140.78 | 28 | |
M IV. Model excluding solar radiation, mass, air temperature | 119.62 | 130.3 | 29 | |
M V. Model excluding snout – vent length, solar radiation, mass, air temperature | 113.88 | 123.04 | 30 |
Chosen model indicated in bold.
AIC, Akaike information criterion; BIC, Bayesian Information Criterion; d.f., degrees of freedom.
Factor | Coefficient | s.e. | t | P | |
---|---|---|---|---|---|
Intercept | 21.854 | 6.3495 | 3.442 | 0.00234 | |
Tsurf | 0.5673 | 0.2355 | 2.408 | 0.02348 | |
TsurfMax | 0.2096 | 0.1146 | 1.830 | 0.07772 | |
Average refuge temperature | −0.7708 | 0.3710 | −2.077 | 0.04847 |
Restricted maximum likelihood (REML): 101.9 with 30 degrees of freedom.
Significant results (P < 0.05) marked in bold.
Habitat
The PCA of habitat structure variables indicated two principal components (PC1 and PC2) explaining a cumulative total of 94.58% of the variation in habitat structure within creek and forest sites (Fig. 4). PC1 explained a high amount of variation (85.63%), describing a gradient of increased water area, rock and flood debris count (Table 5). PC2 explained 8.95% of the variation and described a gradient of increasing woody debris and bare ground. A PERMANOVA was conducted on PC1 only (as only this PC had an eigenvalue >1; Jackson 1993), this revealed a significant difference in habitat structure between creek and forest sites (F1,6 = 32.509, R2 = 0.844, P = 0.02).
Principal Component Analysis performed on the mean habitat structure variables at each high elevation survey site, with groups indicating habitat type (creek vs forest). Coloured ovals around each group display 95% confidence ellipses. The first principal component (PC1) describes increases in water, rock and flood debris cover, and decreases in variables related to forest (i.e. canopy percent, litter).
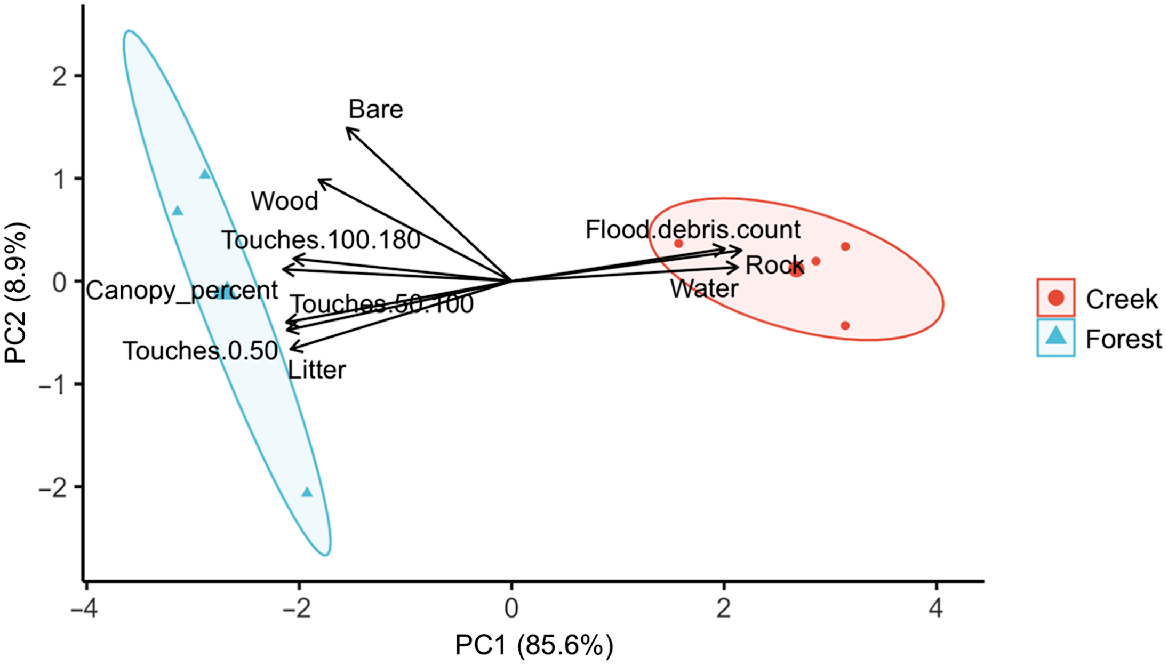
Variable | PC1 | PC2 | |
---|---|---|---|
Proportion of variance | 0.85 | 0.09 | |
Eigenvalue | 8.56 | 0.89 | |
Rock | 0.34 | 0.15 | |
Wood | −0.28 | 0.48 | |
Litter | −0.32 | −0.32 | |
Bare | −0.24 | 0.72 | |
Water | 0.33 | 0.07 | |
Flood debris count | 0.31 | 0.15 | |
Touch pole touches 0–50 cm | −0.33 | −0.23 | |
Touch pole touches 50–100 cm | −0.33 | −0.19 | |
Touch pole touches 100–180 cm | −0.32 | 0.11 | |
Canopy cover (%) | −0.34 | 0.06 |
All but two S. eungellensis individuals were found active in shade. Microhabitat preferences for S. eungellensis were predominantly rocks and fallen palm fronds, but they were also found on woody and flood debris and on leaf litter (Table S6). Creek site mean potential refuge temperatures were lower than those of adjacent rainforest sites (Fig. S8A); however, the mean air temperature for creek sites was higher than adjacent rainforest sites (Fig. S8B; refuge, P < 0.0001; air, P = 0.03811). The thermal regime of Eungella NP varies across elevation with the mean temperatures of both air and refuge being much lower at higher elevations (Fig. S9; P < 0.0001).
Distribution
All individuals were found within 25 m of a stream (Fig. S10) at elevations between 700 and 1000 m (Fig. S11). No more than 25 individuals were found at any site. Using both the species’ historic and new occurrence points, the EOO and AOO were calculated to be 81.7 km2 and 36 km2, respectively (Fig. S4).
Estimate of current population size
The minimum and maximum adult population size estimates for S. eungellensis were 17,333 (high range; >950 m elevation), 4667 (low range; >950 m elevation), and 379 (700–950 m) individuals per km2, respectively (Table S11). Area calculations from buffered high elevation creek lines between 700 and 950 m (A = 15.88 km2) and above 950 m (A = 6.01 km2) resulted in a total A value of 21.9 km2. By inputting these values into the d × A × p formula, along with the P-value of 0.48, minimum 16,352 (4667 × 6.01 × 0.48 + 379 × 15.88 × 0.48) and maximum 52,892 (17,333 × 6.01 × 0.48 + 379 × 15.88 × 0.48) numbers of mature individuals were calculated for S. eungellensis.
Species distributional modelling
The optimal MaxEnt model (regularisation multiplier of one and Linear feature class) performed well, with an AUC of 0.94. The difference between the training and testing AUC (i.e. AUCDIFF) was small (0.05) suggesting that the optimal model generalises well to novel data and is not overfitted (Warren and Seifert 2011; Farquhar et al. 2023a). Both the OR10 (0.17) and ORMTP (0.15) scores for the model were also small, further indicating minimal model overfitting (Fielding and Bell 1997). Full model performance metrics of the candidate MaxEnt models are in Table S8. The geographical prediction of the current distribution of potentially suitable pre-clearing habitat for S. eungellensis is displayed in Fig. 5.
The optimal MaxEnt model predictions displaying currently climatically suitable areas for the potential occurrence of Saproscincus eungellensis across the Eungella study area. Maps show (a) probability of climatic habitat suitability, and (b) a binary prediction based on a probability threshold optimised for ROC; areas highlighted red (where probability of habitat suitability >0.27%) provide the species’ approximate realised niche, given that the model included climate variables aligning with the species ecological needs (e.g. temperature, rainfall).
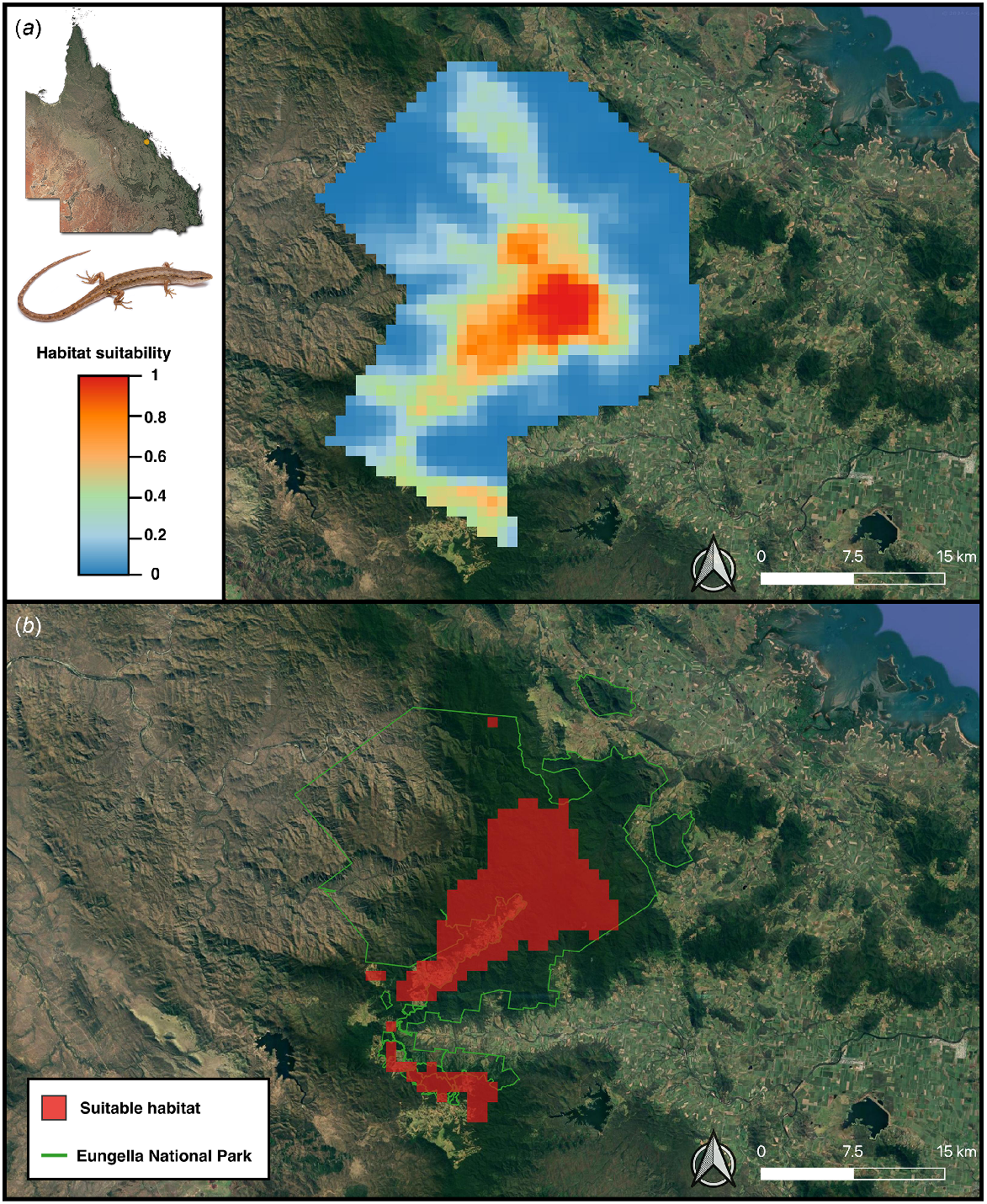
The most important predictors of habitat suitability were annual mean temperature (Bio1; ~70% contribution) and annual precipitation (Bio12; ~25% contribution) (Fig. S12). The model predicted high habitat suitability for relatively low values of annual mean temperatures and high values of annual precipitation. Warm annual temperatures and low annual precipitation were associated with low predicted habitat suitability for the species. Response curves of the environmental variables are supplied in Fig. S13.
Future potential distribution
Projecting models to future climate scenarios predicted large-scale reductions in the extent of potentially suitable habitat for S. eungellensis (Figs 6 and 7). The species is projected to lose all available habitat under emission scenario SSP3.70 and SSP2.45 by 2080 and 2100, respectively (Figs 6 and 7).
MaxEnt model predictions of future climatically suitable areas for the occurrence of Saproscincus eungellensis in the Eungella study area. Maps show continuous probability of pre-clearing habitat suitability modelled at different time periods under (a) Scenario SPP2.45 and (b) Scenario SPP3.70.
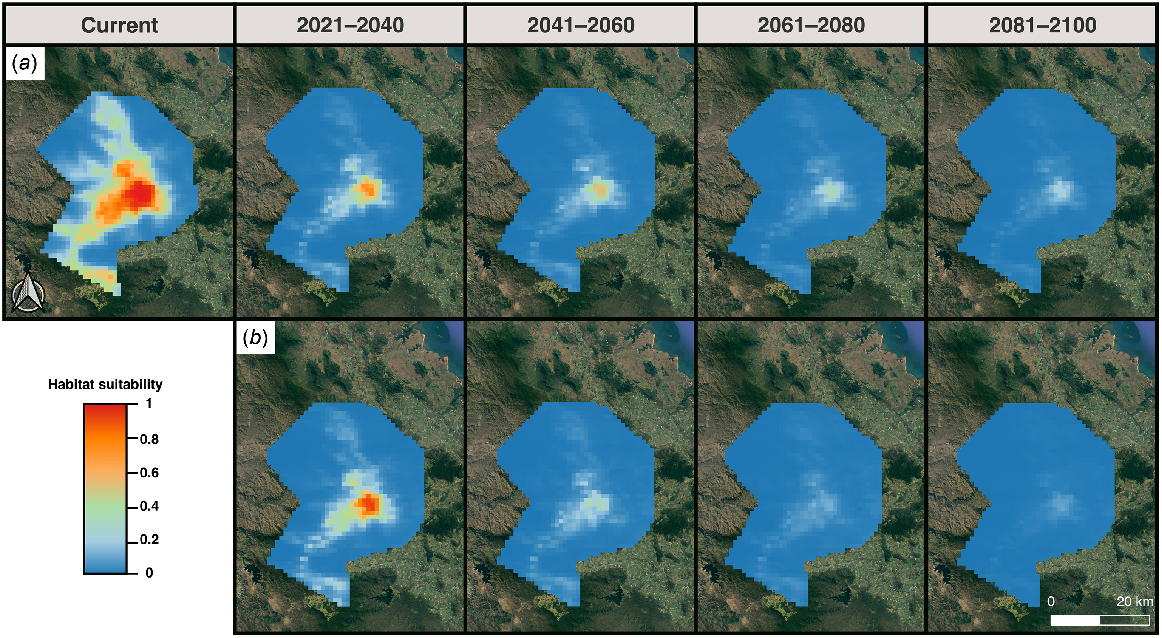
MaxEnt climate model predictions of future climatically suitable areas for the occurrence of Saproscincus eungellensis within the Eungella study area. Maps show binary predictions of pre-clearing habitat suitability determined by a probability threshold that optimises ROC; areas highlighted red (where probability of habitat suitability >0.27%) provide the species’ approximate realised niche, given that the model included climate variables aligning with the species ecological needs (e.g. temperature, rainfall); The orange represents an approximation of the species future realised niche modelled at different time periods under (a) Scenario SPP2.45 and (b) Scenario SPP3.70.
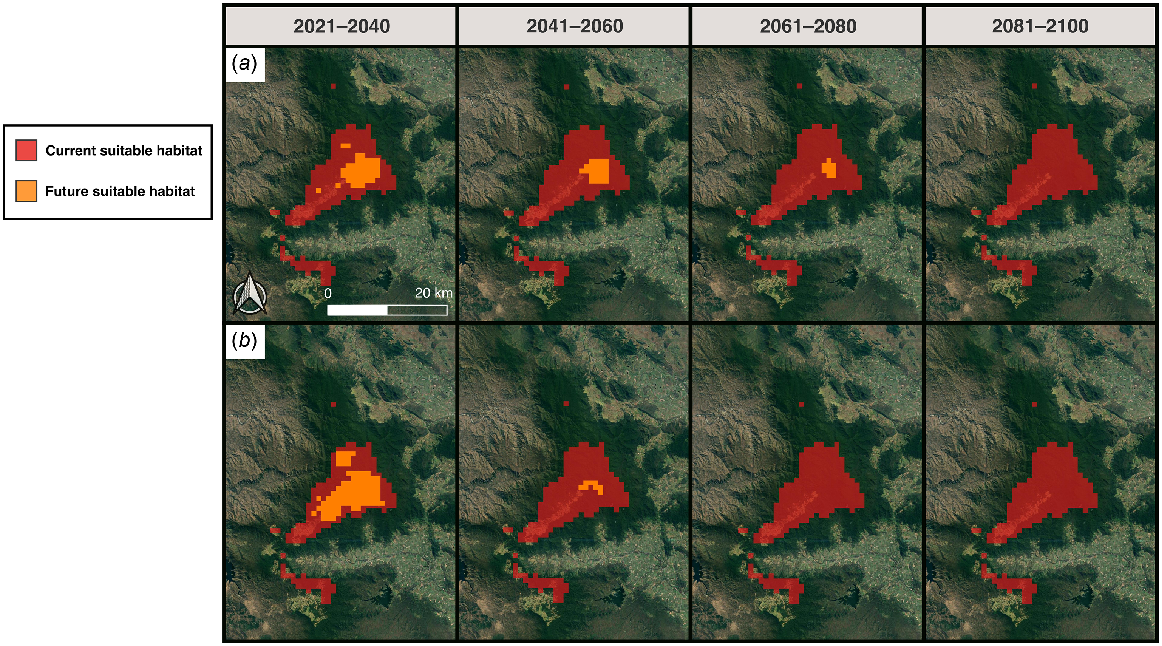
Threat analysis
We observed evidence of fire, invasive species (feral cats and feral pigs) and climate change at sites where Saproscincus eungellensis occurred. The fires of 2018 burned habitat at, and close to, S. eungellensis occurrences (Fig. S14). The extent of the fires within Eungella NP (~209 km2; Hines et al. 2020) burned areas over two times greater and five times greater than the species’ EOO and AOO respectively (for more information about threats refer to Fig. S14, Tables S7 and S9).
Conservation status assessment
Based on assessment against IUCN Red List criteria (see Tables S7 and S9–S11) S. eungellensis meets criteria for A3(c) for Endangered, B1ab(iii) for Critically Endangered, B2ab(iii) for Endangered, Vulnerable D2, and E for Vulnerable. The species is not eligible for listing under criterion C. The Criterion A listing is based on projected population reductions ≥50% in 10 years. The Criterion B listings are based on EOO <100 km2, restricted to one location threatened by climate change, and an inferred continuing decline in area/extent/quality of habitat. The Criterion D listing is based on ≤5 locations and a threat (climate change) that can drive the species to extinction in a short time period. The Criterion E listing was based on ≥0% extinction likelihood in 100 years.
Discussion
This study addresses a key problem in conservation biology: clarifying the conservation status of DD species. We show here how a targeted conservation assessment guided by core aspects of the IUCN Red List criteria can collect sufficient data on a poorly-known species to inform conservation assessments. By undertaking targeted field work we confirmed that S. eungellensis is a highly specialised, range-restricted species, with a likely high susceptibility to predicted climate change. Predictions of habitat suitability in the future under climate change scenarios indicate that the species will completely lose all suitable habitat by 2080. Below we discuss the implications of our results for the conservation of S. eungellensis and the corresponding Red List category that the species is eligible for.
Saproscincus eungellensis is restricted to high elevation areas of Eungella NP
Our study has demonstrated that S. eungellensis is likely restricted to elevations above 700 m in Eungella NP that are annually subject to relatively cold temperatures and high precipitation. The species distribution model accords broadly with modelling conducted by Moussalli et al. (2009) showing that Saproscincus czechurai (a related high-elevation species) is dependent on relatively cool and wet conditions. Annual temperature and precipitation have been identified as significant contributing variables influencing distributional limits in other ectotherms (Kearney and Porter 2004; Penman et al. 2010; Sopniewski et al. 2022). The persistence of short-range endemic taxa is thought to be a result of environmental stability, ecological specialisation, and localised abundance (VanDerWal et al. 2009; Torkkola et al. 2022a). For example, Torkkola et al. (2022b) argued that the persistence of Calorodius thorntonensis is a result of small stable upland rainforest habitats acting as an evolutionary refuge. High elevation rainforest refuges provide a stable but typically localised environment, permitting the continued existence of many short-range endemic species. Similarly, Williams et al. (2009) found endemic vertebrate biodiversity in the Wet Tropics bioregion to be highest in upland areas. Thus, species distributional modelling indicates that climatic variables likely have a high degree of influence on S. eungellensis distributional limits, likely via direct effects of climate on the species’ physiological tolerances. High elevations of Eungella NP offer cooler and wetter climatic conditions than lower elevations (Figs. S9A and S9B). Consequently, the relative stability of climatic variables within high elevations of Eungella NP over evolutionary time scales likely shaped the small potential habitat suitability of the species observed through modelling.
Saproscincus eungellensis is a riparian zone specialist with cold-adapted thermal biology
Saproscincus eungellensis was found to mostly occur in close proximity (<25 m) to creek lines, with the vast majority of observations within 5 m of a water course. Riparian habitats are known to be key refugia for many taxa (Faria et al. 2019), as they generally have high humidity and are thermally buffered. As such, riparian habitats often support disproportionately high levels of both species’ abundance and richness in ectotherms (Warren and Schwalbe 1985; Faria et al. 2019; Bateman and Merritt 2020). The reliance of S. eungellensis on riparian habitat accords with the hypothesised association between mesic herpetofauna and habitats with a heterogenous structure that provide ample microhabitat refuges (Farquhar et al. 2023b). Farquhar et al. (2023b) showed that range-edge populations of Lampropholis delicata (delicate skink) are associated with habitats that offer deep leaf litter, logs, and rocks. Such microhabitat structure is critical to skink ecology, impacting species ability to avoid predation, hunt and thermoregulate (Neel et al. 2021).
Thermoregulation, along with high humidity, may be a key factor explaining the reliance of S. eungellensis on riparian habitat. Morgan (1988) suggests that in heavily shaded environments (such as rainforests), thermally discrete microclimates are critically important for lizard thermoregulation. Complex microhabitat structures related to riparian habitat provide a varying thermal gradient allowing access to distinct thermal regimes. For example, coarse woody debris has been shown to provide North American forest reptiles and amphibians a substrate for both thermoregulation and refuge use that is distinct from ambient air temperatures (Whiles and Grubaugh 1996). High elevation forest areas are of relatively low thermal quality (Díaz 1997; Brazeau 2016), but rocks within riparian systems provide higher substrate temperatures than other microhabitats, allowing high elevation ectothermic species to achieve higher desired body temperatures. For example, Muñoz and Losos (2018) found that in an elevation-stratified assemblage of lizards (Anolis) high elevation individuals were more frequently encountered on rock substrates whereas low elevation individuals were encountered on trees. Closed habitats such as highly shaded forests (where S. eungellensis are uncommon) strongly filter sunlight (Scheffers et al. 2017) and exhibit little thermal heterogeneity, posing challenges to the maintenance of optimal body temperatures by ectotherms (Huey 1974; Fitzgerald et al. 2003). For example, Huang et al. (2014), found high elevation forests are too cold to provide suitable habitat to support populations of a high elevation lizard species, Takydromus hsuehshanensis. Thus, although S. eungellensis is not an active basker, it may be seeking out the warmer parts of its microhabitat to achieve its thermal requirements.
Saproscincus eungellensis displayed relatively low field active body temperatures compared to other high elevation skink species (Table 1). This accords with observations that other species of Saproscincus, and other thigmothermic species, have lower CTMax values than active basking lizard species occupying similar environments (Table 1; Greer 1980, 1989; Muñoz et al. 2016). For example, Muñoz et al. (2016) showed a difference of 10°C in the CTMax values of two Australian rainforest lizard species occurring in the same locality, Saproscincus czechurai (35.1°C) and Carlia rubrigularis (45.2°C). The low field active body temperature of S. eungellensis may be explained by considering aspects of their ecology. In the field we found substrate temperature to be the most significant predictor of S. eungellensis body temperature, whereas both air temperature and solar radiation were unrelated to body temperature. Further, we only observed two S. eungellensis individuals active outside of shade. Saproscincus eungellensis belongs to a genus of shade-seeking scincid lizards, which regulate body temperatures via conduction of heat from bodily contact with surface structures (i.e. thigmothermy) rather than direct sunlight (heliothermy; Muñoz et al. 2016; Sazima 2023). Heat tolerance in rainforest lizard species likely evolved in two distinct directions, with edge habitat species displaying significantly higher heat tolerances than shade-seeking species (Muñoz et al. 2016). Thus, specialisation to use cool and mesic microhabitats likely contributed to the relatively low field active body temperatures displayed by S. eungellensis. Future studies should determine the CTMax and thermal preference of S. eungellensis. However, Grigg and Buckley (2013) found that thermal tolerances are conserved within evolutionary history rather than being determined by dispersal or local adaptation. Thus, we hypothesise that S. eungellensis has both a relatively low CTMax and thermal preference in line with its congeners; indeed, this may explain why the species is geographically restricted to high-elevation creek lines.
Threats to the persistence of Saproscincus eungellensis
Saproscincus eungellensis is affected by numerous threats; in the field we observed evidence of invasive species (feral cats and feral pigs), fire and climate change (additional potential threats to the species are outlined in Tables S7 and S10). These threats are known to interact; for example, both fire and feral pigs simplify habitats by removing vegetation and refuges, which in turn creates conditions favouring cat predation (Doupé et al. 2010; McGregor et al. 2016). Cats are particularly problematic for Australian native reptile species and it is estimated that cats kill 466 million Australian native reptiles per year (Woinarski et al. 2018). The observed threats for S. eungellensis are consistent with threat analyses reported by Tingley et al. (2019) and Geyle et al. (2021), who highlighted invasive species, habitat destruction (i.e. through development), and climate change as major threats acting on terrestrial squamates.
Saproscincus eungellensis displays many characteristics consistent with species considered especially vulnerable to climate change. First, the species is highly specialised and restricted to high-elevation riparian habitat. Tropical high-elevation specialists have been identified as among the species most likely to be impacted by climate change. For example, Cordier et al. (2020) predicted total losses for two microendemic high elevation frog species in response to climate change. Similarly, highly specialised species are predicted to be highly susceptible to climate change. For example, Hagger et al. (2013) identified habitat specialisation as the second most important predictor of climate sensitivity in an assemblage of subtropical vertebrates. Second, S. eungellensis is a relatively recently described narrow-range endemic, impacted by multiple threats. Wotherspoon et al. (2024) determined that these traits characterised many Australian threatened squamates. Finally, S. eungellensis is a thigmothermic species and consequently has a relatively low CTMax. Muñoz et al. (2016) showed closely related shade-seeking Saproscincus species with relatively low CTMax’s are especially susceptible to climate warming due to low tolerance of heat. Consequently, S. eungellensis is likely highly susceptible to future climatic changes.
Despite using an optimistic model (CMCC-CM2) and relatively optimistic scenarios (SSP2.45 and SSP3.70; Grose et al. 2023; HamadAmin and Khwarahm 2023), future climatic modelling for S. eungellensis showed complete loss of climatically suitable habitat for both climate emission scenarios SSP2.45 and SSP3.70 by 2100 and 2080, respectively (Figs S12 and S13). Our modelling aligns with previous work by Williams et al. (2003), which showed extensive losses for Australian tropical high elevation rainforest species in response to climate change. Similarly, Williams et al. (2008) predicted that globally many high elevation tropical climates would disappear in response to global warming, essentially leaving these species with no available habitat. In a similar vein Laurance (2015) predicted temperature increases of just 2°C would be enough to drive 1/8th of endemic montane vertebrates in tropical Queensland extinct. Modelling of palaeo-distributions for a related and ecologically similar species (Saproscincus czechurai) by Moussalli et al. (2009), indicated a larger distribution when colder and wetter climates were much more extensive in the Pliocene. The differences between prediction of past, present and future distributions of high elevation species highlight the magnitude of the potential impacts of climate warming on these species’ persistence. There is some potential that the riparian microhabitat that S. eungellensis occurs in may buffer the impacts of climate change, and this process may explain why high elevation areas of the Eungella region host several evolutionarily old faunal lineages (Mahony 2020). However, the speed and trajectory of current climate warming exceeds that most organisms have experienced in their recent evolutionary history, and S. eungellensis also has a very narrow niche. For these reasons we argue that our dire predictions for the future of this species under a rapidly and markedly warming climate are realistic and defendable.
In addition, although future climate modelling for S. eungellensis predicted drastic losses of climatically suitable areas, it is important to note that other synergistic processes are of concern. First, while CMIP6 climate models predict factors that influence fire likelihood, the model may not show the actual extent and severity of potential future fires (Li et al. 2024). A more severe fire weather climate is predicted for Eungella NP (Climate Change in Australia 2020), and fire has already severely impacted high elevation areas of Eungella NP, burning areas in close proximity to core S. eungellensis habitat (Hines et al. 2020). Modelling by Torkkola et al. (2022a) estimated that the 2019–2020 fires burnt about half of the predicted distribution of Harrisoniascincus zia, an Australian skink with a similar preference for cool wet upland forest habitats. Second, the modelling does not consider the arrival of lower elevation predators, competitors and pathogens. Warming pushes climatic envelopes towards the poles or higher elevation, warmer climate species are able to track these changes and shift their ranges to accommodate them (Walther et al. 2002; Colwell et al. 2008; Ashton et al. 2016). In the field we saw evidence of skink assemblage composition changes in the Eungella area, with different species occurring in distinct bands of elevation. If these species track climatic envelopes to higher elevations, they may compete with or predate S. eungellensis, further contributing to extinction risk. The arrival of lowland competitors has already been observed affecting other Australian high-elevation skink species (DCCEEW 2023c).
Saproscincus eungellensis meets the criteria for recognition as Critically Endangered
Saproscincus eungellensis met the criteria for Critically Endangered (under Criterion B), the most severe IUCN Red List category before extinction. The species also met the criteria for Endangered (A3, B2), and Vulnerable (D2, E), highlighting that the species is severely impacted by multiple aspects of its ecology and threats. As a Critically Endangered species likely highly susceptible to climate change, conservation (e.g. population monitoring, and mitigation of feral species) for this species should be considered an urgent priority.
These results highlight issues with the IUCN DD category. First, we know that DD species are often at risk of extinction, but have not yet received such diagnosis (Bland et al. 2017; González-del-Pliego et al. 2019; Caetano et al. 2022). Second, the IUCN Red List has only considered climate change as the main threatening process for 4% of assessed skink species (Chapple et al. 2021; Torkkola et al. 2022a). The case of S. eungellensis highlights limitations in the IUCN framework as a previously DD species severely impacted by climate change. Unfortunately, there are many species still listed as DD on the IUCN Red List. The methods used in this study can act as a framework allowing future researchers to survey and assess DD species to remove them from the problematic DD category. Further, this research can act as a case study applying the methods outlined by Mancini et al. (2024) to assess the impacts of climate change under subcriterion A3.
Conclusion
With rapidly occurring changes in climate, many of the 21,000 species within the DD category of the IUCN may be faced with the prospect of total habitat loss and corresponding extinction. It is therefore paramount that conservation scientists focus on species in this category to identify those most at risk and better resolve the true conservation status of DD species. The findings from our research add to the growing body of evidence that both DD and high elevation range-restricted species are likely highly susceptible to climatic changes and at a heightened risk of extinction. The methods outlined in this paper can be used as a framework for future researchers to undertake relatively rapid field studies coupled with species distributional modelling of DD species, thereby allowing them to be assigned to a data sufficient IUCN Red List category. In turn, these methods will help to enable conservation managers to target conservation and recovery efforts to the species that require it most.
Declaration of funding
The project was supported by a grant from the Australian Research Council (FT200100108; to DGC), a scholarship from Biosis PTY LTD (to NS) and by funding from the Queensland Department of Environment and Science.
Acknowledgements
We would like to acknowledge the traditional custodians of Eungella NP, the Birri-Gubba and Wirri people. Justin Wright assisted with fieldwork, and Janne Torkkola assisted with the climate modelling. We thank Ben Fredrickson for providing access through his land so we could get to our study sites more effectively.
References
Aiello-Lammens ME, Boria RA, Radosavljevic A, Vilela B, Anderson RP (2015) spThin: an R package for spatial thinning of species occurrence records for use in ecological niche models. Ecography 38(5), 541-545.
| Crossref | Google Scholar |
ALA (Atlas of Living Australia) (2023) Saproscincus eungellensis. Available at https://bie.ala.org.au/species/https://biodiversity.org.au/afd/taxa/c5881bf2-26fb-4c58-8504-4f0515eda375 [accessed 8 April 2023]
Anderson MJ (2017) Permutational multivariate analysis of variance (PERMANOVA). In ‘Wiley StatsRef: statistics reference online’. (Eds N Balakrishnan, T Colton, B Everitt, W Piegorsch, F Ruggeri, JL Teugels) pp. 1–15. (John Wiley & Sons, Ltd: Auckland, New Zealand) doi:10.1002/9781118445112.stat07841
Ashton LA, Odell EH, Burwell CJ, Maunsell SC, Nakamura A, McDonald WJF, Kitching RL (2016) Altitudinal patterns of moth diversity in tropical and subtropical Australian rainforests. Austral Ecology 41(2), 197-208.
| Crossref | Google Scholar |
Bachman S, Moat J (2012) GeoCAT – an open source tool for rapid red list assessments. BGjournal 9(1), 11-13.
| Google Scholar |
Bachman S, Moat J, Hill AW, de la Torre J, Scott B (2011) Supporting Red List threat assessments with GeoCAT: geospatial conservation assessment tool. ZooKeys 150, 117-126.
| Crossref | Google Scholar |
Bateman HL, Merritt DM (2020) Complex riparian habitats predict reptile and amphibian diversity. Global Ecology and Conservation 22, e00957.
| Crossref | Google Scholar |
Bland LM, Bielby J, Kearney S, Orme CDL, Watson JEM, Collen B (2017) Toward reassessing data-deficient species. Conservation Biology 31(3), 531-539.
| Crossref | Google Scholar | PubMed |
Borgelt J, Dorber M, Høiberg MA, Verones F (2022) More than half of data deficient species predicted to be threatened by extinction. Communications Biology 5(1), 679.
| Crossref | Google Scholar | PubMed |
Braby MF (2018) Threatened species conservation of invertebrates in Australia: an overview. Austral Entomology 57(2), 173-181.
| Crossref | Google Scholar |
Brazeau D (2016) Habitat selection in the Common Five-lined Skink near the northern extent of its range. Master Thesis, Lakehead University, Ontario, Canada. Available at https://knowledgecommons.lakeheadu.ca/handle/2453/4239
Burnham KP, Anderson DR (2004) Multimodel Inference: understanding AIC and BIC in model selection. Sociological Methods & Research 33(2), 261-304.
| Crossref | Google Scholar |
Butchart SHM, Bird JP (2010) Data Deficient birds on the IUCN Red List: what don’t we know and why does it matter? Biological Conservation 143(1), 239-247.
| Crossref | Google Scholar |
Caetano GHdO, Chapple DG, Grenyer R, Raz T, Rosenblatt J, Tingley R, Böhm M, Meiri S, Roll U (2022) Automated assessment reveals that the extinction risk of reptiles is widely underestimated across space and phylogeny. PLoS Biology 20(5), e3001544.
| Crossref | Google Scholar | PubMed |
Chapple DG, Roll U, Böhm M, Aguilar R, Amey AP, Austin CC, Baling M, Barley AJ, Bates MF, Bauer AM, Blackburn DG, Bowles P, Brown RM, Chandramouli SR, Chirio L, Cogger H, Colli GR, Conradie W, Couper PJ, Cowan MA, Craig MD, Das I, Datta-Roy A, Dickman CR, Ellis RJ, Fenner AL, Ford S, Ganesh SR, Gardner MG, Geissler P, Gillespie GR, Glaw F, Greenlees MJ, Griffith OW, Grismer LL, Haines ML, Harris DJ, Hedges SB, Hitchmough RA, Hoskin CJ, Hutchinson MN, Ineich I, Janssen J, Johnston GR, Karin BR, Keogh JS, Kraus F, LeBreton M, Lymberakis P, Masroor R, McDonald PJ, Mecke S, Melville J, Melzer S, Michael DR, Miralles A, Mitchell NJ, Nelson NJ, Nguyen TQ, de Campos Nogueira C, Ota H, Pafilis P, Pauwels OSG, Perera A, Pincheira-Donoso D, Reed RN, Ribeiro-Júnior MA, Riley JL, Rocha S, Rutherford PL, Sadlier RA, Shacham B, Shea GM, Shine R, Slavenko A, Stow A, Sumner J, Tallowin OJS, Teale R, Torres-Carvajal O, Trape J-F, Uetz P, Ukuwela KDB, Valentine L, Van Dyke JU, van Winkel D, Vasconcelos R, Vences M, Wagner P, Wapstra E, While GM, Whiting MJ, Whittington CM, Wilson S, Ziegler T, Tingley R, Meiri S (2021) Conservation status of the world’s skinks (Scincidae): taxonomic and geographic patterns in extinction risk. Biological Conservation 257, 109101.
| Crossref | Google Scholar |
Cherchi A, Fogli PG, Lovato T, Peano D, Iovino D, Gualdi S, Masina S, Scoccimarro E, Materia S, Bellucci A, Navarra A (2019) Global mean climate and main patterns of variability in the CMCC-CM2 coupled model. Journal of Advances in Modeling Earth Systems 11(1), 185-209.
| Crossref | Google Scholar |
Climate Change in Australia (2020) Super Clusters. Available at https://www.climatechangeinaustralia.gov.au/en/projections-tools/regional-climate-change-explorer/super-clusters/ [accessed 22 August 2023]
Colles A, Liow LH, Prinzing A (2009) Are specialists at risk under environmental change? Neoecological, paleoecological and phylogenetic approaches. Ecology Letters 12(8), 849-863.
| Crossref | Google Scholar | PubMed |
Colwell RK, Brehm G, Cardelús CL, Gilman AC, Longino JT (2008) Global warming, elevational range shifts, and lowland biotic attrition in the wet tropics. Science 322(5899), 258-261.
| Crossref | Google Scholar | PubMed |
Cordier JM, Lescano JN, Ríos NE, Leynaud GC, Nori J (2020) Climate change threatens micro-endemic amphibians of an important South American high-altitude center of endemism. Amphibia-Reptilia 41(2), 233-243.
| Crossref | Google Scholar |
Cox N, Young BE, Bowles P, Fernandez M, Marin J, Rapacciuolo G, Böhm M, Brooks TM, Hedges SB, Hilton-Taylor C, Hoffmann M, Jenkins RKB, Tognelli MF, Alexander GJ, Allison A, Ananjeva NB, Auliya M, Avila LJ, Chapple DG, Cisneros-Heredia DF, Cogger HG, Colli GR, de Silva A, Eisemberg CC, Els J, Fong GA, Grant TD, Hitchmough RA, Iskandar DT, Kidera N, Martins M, Meiri S, Mitchell NJ, Molur S, Nogueira CdC, Ortiz JC, Penner J, Rhodin AGJ, Rivas GA, Rödel M-O, Roll U, Sanders KL, Santos-Barrera G, Shea GM, Spawls S, Stuart BL, Tolley KA, Trape J-F, Vidal MA, Wagner P, Wallace BP, Xie Y (2022) A global reptile assessment highlights shared conservation needs of tetrapods. Nature 605(7909), 285-290.
| Crossref | Google Scholar | PubMed |
Department of Environment, Science and Innovation, Queensland (2024a) Wetland mapping — Calen 100K map tile — 8656, Wetland Info website. Available at https://wetlandinfo.des.qld.gov.au/wetlands/facts-maps/tile-100k-calen/ [accessed 10 April 2024]
Department of Environment, Science and Innovation, Queensland (2024b) Wetland mapping — Mirani 100K map tile — 8656, Wetland Info website. Available at https://wetlandinfo.des.qld.gov.au/wetlands/facts-maps/tile-100k-mirani/ [accessed 10 April 2024]
Díaz JA (1997) Ecological correlates of the thermal quality of an ectotherm’s habitat: a comparison between two temperate lizard populations. Functional Ecology 11(1), 79-89.
| Crossref | Google Scholar |
Doupé RG, Mitchell J, Knott MJ, Davis AM, Lymbery AJ (2010) Efficacy of exclusion fencing to protect ephemeral floodplain lagoon habitats from feral pigs (Sus scrofa). Wetlands Ecology and Management 18(1), 69-78.
| Crossref | Google Scholar |
Ebach MC, Michaux B (2020) Neotectonics and Australian biogeography. In ‘Biotectonics: tectonics as the driver of bioregionalisation’. (Eds MC Ebach, B Michaux) pp. 33–47. (Springer International Publishing: Cham) 10.1007/978-3-030-51773-1_3
Eyring V, Bony S, Meehl GA, Senior CA, Stevens B, Stouffer RJ, Taylor KE (2016) Overview of the coupled model intercomparison project phase 6 (CMIP6) experimental design and organization. Geoscientific Model Development 9(5), 1937-1958.
| Crossref | Google Scholar |
Fan X, Duan Q, Shen C, Wu Y, Xing C (2020) Global surface air temperatures in CMIP6: historical performance and future changes. Environmental Research Letters 15(10), 104056.
| Crossref | Google Scholar |
Faria AS, Menin M, Kaefer IL (2019) Riparian zone as a main determinant of the structure of lizard assemblages in upland Amazonian forests. Austral Ecology 44(5), 850-858.
| Crossref | Google Scholar |
Farquhar JE, Carlesso A, Pili A, Gale N, Chapple DG (2023a) Capturing uncatalogued distribution records to improve conservation assessments of data-deficient species: a case study using the glossy grass skink. Animal Conservation 27(1), 124-137.
| Crossref | Google Scholar |
Farquhar JE, Russell W, Chapple DG (2023b) Identifying the abiotic factors that determine the inland range limits of a mesic-adapted lizard species. Integrative and Comparative Biology 64(1), 55-66.
| Crossref | Google Scholar |
Fielding AH, Bell JF (1997) A review of methods for the assessment of prediction errors in conservation presence/absence models. Environmental Conservation 24(1), 38-49.
| Crossref | Google Scholar |
Fitzgerald M, Shine R, Lemckert F (2003) A reluctant heliotherm: thermal ecology of the arboreal snake Hoplocephalus stephensii (Elapidae) in dense forest. Journal of Thermal Biology 28(6–7), 515-524.
| Crossref | Google Scholar |
Fox J, Weisberg S (2019) ‘An R companion to applied regression,’ 3rd edn. (Sage: Thousand Oaks, CA) Available at https://www.john-fox.ca/Companion/
Geyle HM, Tingley R, Amey AP, Cogger H, Couper PJ, Cowan M, Craig MD, Doughty P, Driscoll DA, Ellis RJ, Emery J-P, Fenner A, Gardner MG, Garnett ST, Gillespie GR, Greenlees MJ, Hoskin CJ, Keogh JS, Lloyd R, Melville J, McDonald PJ, Michael DR, Mitchell NJ, Sanderson C, Shea GM, Sumner J, Wapstra E, Woinarski JCZ, Chapple DG (2021) Reptiles on the brink: identifying the Australian terrestrial snake and lizard species most at risk of extinction. Pacific Conservation Biology 27(1), 3-12.
| Crossref | Google Scholar |
Godínez-Alvarez H, Herrick JE, Mattocks M, Toledo D, Van Zee J (2009) Comparison of three vegetation monitoring methods: their relative utility for ecological assessment and monitoring. Ecological Indicators 9(5), 1001-1008.
| Crossref | Google Scholar |
González-del-Pliego P, Freckleton RP, Edwards DP, Koo MS, Scheffers BR, Pyron RA, Jetz W (2019) Phylogenetic and trait-based prediction of extinction risk for Data-Deficient amphibians. Current Biology 29(9), 1557-1563.E3.
| Crossref | Google Scholar | PubMed |
Grady KC, Laughlin DC, Ferrier SM, Kolb TE, Hart SC, Allan GJ, Whitham TG (2013) Conservative leaf economic traits correlate with fast growth of genotypes of a foundation riparian species near the thermal maximum extent of its geographic range. Functional Ecology 27(2), 428-438.
| Crossref | Google Scholar |
Graham KA, Mahony SV, Chapple DG, Farquhar JE (2023) The long unknown: rediscovery of the long sunskink, Lampropholis elongata (Squamata: Scincidae) – after almost a decade, and after 50 years of data deficiency. Austral Ecology 48(5), 877-884.
| Crossref | Google Scholar |
Greer AE (1980) Critical thermal maximum temperatures in Australian scincid lizards: their ecological and evolutionary significance. Australian Journal of Zoology 28(1), 91-102.
| Crossref | Google Scholar |
Greer AE (1989) ‘The biology and evolution of Australian lizards.’ Surrey Beatty. Available at https://cir.nii.ac.jp/crid/1130282268970495616
Grigg JW, Buckley LB (2013) Conservatism of lizard thermal tolerances and body temperatures across evolutionary history and geography. Biology Letters 9(2), 20121056.
| Crossref | Google Scholar | PubMed |
Grose MR, Narsey S, Trancoso R, Mackallah C, Delage F, Dowdy A, Di Virgilio G, Watterson I, Dobrohotoff P, Rashid HA, Rauniyar S, Henley B, Thatcher M, Syktus J, Abramowitz G, Evans JP, Su C-H, Takbash A (2023) A CMIP6-based multi-model downscaling ensemble to underpin climate change services in Australia. Climate Services 30, 100368.
| Crossref | Google Scholar |
Gumbs R, Gray CL, Böhm M, Hoffmann M, Grenyer R, Jetz W, Meiri S, Roll U, Owen NR, Rosindell J (2020) Global priorities for conservation of reptilian phylogenetic diversity in the face of human impacts. Nature Communications 11(1), 2616.
| Crossref | Google Scholar | PubMed |
Hagger V, Fisher D, Schmidt S, Blomberg S (2013) Assessing the vulnerability of an assemblage of subtropical rainforest vertebrate species to climate change in south-east Queensland. Austral Ecology 38(4), 465-475.
| Crossref | Google Scholar |
HamadAmin BA, Khwarahm NR (2023) Mapping impacts of climate change on the distributions of two endemic tree species under Socioeconomic Pathway Scenarios (SSP). Sustainability 15(6), 5469.
| Crossref | Google Scholar |
Hamilton K, Goulet CT, Drummond EM, Senior AF, Schroder M, Gardner MG, While GM, Chapple DG (2021) Decline in lizard species diversity, abundance and ectoparasite load across an elevational gradient in the Australian alps. Austral Ecology 46, 8-19.
| Crossref | Google Scholar |
Hausfather Z, Peters GP (2020) Emissions – the ‘business as usual’ story is misleading. Nature 577(7792), 618-620.
| Crossref | Google Scholar | PubMed |
Hijmans RJ, Cameron SE, Parra JL, Jones PG, Jarvis A (2005) Very high resolution interpolated climate surfaces for global land areas. International Journal of Climatology 25(15), 1965-1978.
| Crossref | Google Scholar |
Hines HB, Brook M, Wilson J, McDonald WJF, Hargreaves J (2020) The extent and severity of the MacKay highlands 2018 wildfires and the potential impact on natural values, particularly in the mesic forests of the Eungella-crediton area. Proceedings of the Royal Society of Queensland 125, 139-157.
| Crossref | Google Scholar |
Hoskin C, Amey A, Couper P, Vanderduys E (2018) Saproscincus eungellensis. The IUCN Red List of Threatened Species 2018: e.T109481233A109481236. Available at doi:https://dx.doi.org/10.2305/IUCN.UK.2018-1.RLTS.T109481233A109481236.en [accessed 11 October 2024]
Huang S-P, Porter WP, Tu M-C, Chiou C-R (2014) Forest cover reduces thermally suitable habitats and affects responses to a warmer climate predicted in a high-elevation lizard. Oecologia 175(1), 25-35.
| Crossref | Google Scholar | PubMed |
Huey RB (1974) Behavioral thermoregulation in lizards: importance of associated costs. Science 184(4140), 1001-1003.
| Crossref | Google Scholar |
Hughes L (2011) Climate change and Australia: key vulnerable regions. Regional Environmental Change 11(S1), 189-195.
| Crossref | Google Scholar |
IUCN (2024a) Summary statistics. IUCN Red List. Available at https://www.iucnredlist.org/resources/summary-statistics [accessed 20 March 2024].
IUCN (2024b) Guidelines for using the IUCN red list categories and criteria [online]. Available at https://nc.iucnredlist.org/redlist/content/attachment_files/RedListGuidelines.pdf [accessed 28 February 2024].
Jackson DA (1993) Stopping rules in principal components analysis: a comparison of heuristical and statistical approaches. Ecology 74(8), 2204-2214.
| Crossref | Google Scholar |
Jarić I, Courchamp F, Gessner J, Roberts DL (2016) Potentially threatened: a Data Deficient flag for conservation management. Biodiversity and Conservation 25(10), 1995-2000.
| Crossref | Google Scholar |
Kass JM, Muscarella R, Galante PJ, Bohl CL, Pinilla-Buitrago GE, Boria RA, Soley-Guardia M, Anderson RP (2021) ENMeval 2.0: redesigned for customizable and reproducible modeling of species’ niches and distributions. Methods in Ecology and Evolution 12(9), 1602-1608.
| Crossref | Google Scholar |
Kassambara A, Mundt F (2020) Factoextra: extract and visualize the results of multivariate data analyses. R Package Version 1.0.7. Available at https://CRAN.R-project.org/package=factoextra
Kearney M, Porter WP (2004) Mapping the fundamental niche: physiology, climate, and the distribution of a nocturnal lizard. Ecology 85(11), 3119-3131.
| Crossref | Google Scholar |
Kramer-Schadt S, Niedballa J, Pilgrim JD, Schröder B, Lindenborn J, Reinfelder V, Stillfried M, Heckmann I, Scharf AK, Augeri DM, Cheyne SM, Hearn AJ, Ross J, Macdonald DW, Mathai J, Eaton J, Marshall AJ, Semiadi G, Rustam R, Bernard H, Alfred R, Samejima H, Duckworth JW, Breitenmoser-Wuersten C, Belant JL, Hofer H, Wilting A (2013) The importance of correcting for sampling bias in MaxEnt species distribution models. Diversity and Distributions 19(11), 1366-1379.
| Crossref | Google Scholar |
Laurance WF (2015) Emerging threats to tropical forests. Annals of the Missouri Botanical Garden 100(3), 159-169.
| Crossref | Google Scholar |
Le Breton TD, Zimmer HC, Gallagher RV, Cox M, Allen S, Auld TD (2019) Using IUCN criteria to perform rapid assessments of at-risk taxa. Biodiversity and Conservation 28(4), 863-883.
| Crossref | Google Scholar |
Lê S, Josse J, Husson F (2008) FactoMineR: an R package for multivariate analysis. Journal of Statistical Software 25(1), 1-18.
| Crossref | Google Scholar |
Lees DM, Watchorn DJ, Driscoll DA, Doherty TS (2022) Microhabitat selection by small mammals in response to fire. Australian Journal of Zoology 69(3), 67-79.
| Crossref | Google Scholar |
Li F, Song X, Harrison S, Lin Z (2024) Evaluation of global fire simulations in CMIP6 Earth system models. In ‘EGU General Assembly 2024, EGU24-14202. Copernicus Meetings’. Geoscientific Model Development Discussions. 10.5194/egusphere-egu24-14202
Lindenmayer DB, Wood JT, McBurney L, MacGregor C, Youngentob K, Banks SC (2011) How to make a common species rare: a case against conservation complacency. Biological Conservation 144(5), 1663-1672.
| Crossref | Google Scholar |
Mahony MJ (2020) The amphibian fauna of Eungella and their important role in unravelling the evolutionary history of the Australian east coast closed forest biota. Proceedings of the Royal Society of Queensland 125, 81-96.
| Google Scholar |
Mancini G, Santini L, Cazalis V, Akçakaya HR, Lucas PM, Brooks TM, Foden W, Di Marco M (2024) A standard approach for including climate change responses in IUCN Red List assessments. Conservation Biology 38(3), e14227.
| Crossref | Google Scholar |
Martin TG, Nally S, Burbidge AA, Arnall S, Garnett ST, Hayward MW, Lumsden LF, Menkhorst P, McDonald-Madden E, Possingham HP (2012) Acting fast helps avoid extinction. Conservation Letters 5(4), 274-280.
| Crossref | Google Scholar |
Masson-Delmotte VP, Zhai P, Pirani SL, Connors C, Péan S, Berger N, Caud Y, Chen L, Goldfarb MI, Scheel Monteiro PM (2021) IPCC, 2021: Summary for Policymakers. In: Climate Change 2021: The Physical Science Basis. Contribution of Working Group I to the Sixth Assessment Report of the Intergovernmental Panel on Climate Change. Report. Cambridge University Press, Cambridge, United Kingdom and New York, NY, USA. Available at https://researchspace.csir.co.za/dspace/handle/10204/12710
McGregor HW, Cliff HB, Kanowski J (2016) Habitat preference for fire scars by feral cats in Cape York Peninsula, Australia. Wildlife Research 43(8), 623-633.
| Crossref | Google Scholar |
Meinshausen M, Nicholls ZRJ, Lewis J, Gidden MJ, Vogel E, Freund M, Beyerle U, Gessner C, Nauels A, Bauer N, Canadell JG, Daniel JS, John A, Krummel PB, Luderer G, Meinshausen N, Montzka SA, Rayner PJ, Reimann S, Smith SJ, van den Berg M, Velders GJM, Vollmer MK, Wang RHJ (2020) The shared socio-economic pathway (SSP) greenhouse gas concentrations and their extensions to 2500. Geoscientific Model Development 13(8), 3571-3605.
| Crossref | Google Scholar |
Meiri S, Chapple DG, Tolley KA, Mitchell N, Laniado T, Cox N, Bowles P, Young BE, Caetano G, Geschke J, Böhm M, Roll U (2023) Done but not dusted: reflections on the first global reptile assessment and priorities for the second. Biological Conservation 278, 109879.
| Crossref | Google Scholar |
Meyer EA, Hines HB, Clarke JM, Hoskin CJ (2020) An update on the status of wet forest stream-dwelling frogs of the Eungella region. The Proceedings of the Royal Society of Queensland 125, 97-115.
| Google Scholar |
Morais AR, Siqueira MN, Lemes P, Maciel NM, De Marco P, Jr, Brito D (2013) Unraveling the conservation status of Data Deficient species. Biological Conservation 166, 98-102.
| Crossref | Google Scholar |
Morgan KR (1988) Body temperature, energy metabolism, and stamina in two neotropical forest lizards (ameiva, teiidae). Journal of Herpetology 22(2), 236-241.
| Crossref | Google Scholar |
Moussalli A, Moritz C, Williams SE, Carnaval AC (2009) Variable responses of skinks to a common history of rainforest fluctuation: concordance between phylogeography and palaeo-distribution models. Molecular Ecology 18(3), 483-499.
| Crossref | Google Scholar | PubMed |
Muñoz MM, Losos JB (2018) Thermoregulatory behavior simultaneously promotes and forestalls evolution in a tropical lizard. The American Naturalist 191(1), E15-E26.
| Crossref | Google Scholar | PubMed |
Muñoz MM, Langham GM, Brandley MC, Rosauer DF, Williams SE, Moritz C (2016) Basking behavior predicts the evolution of heat tolerance in Australian rainforest lizards. Evolution 70(11), 2537-2549.
| Crossref | Google Scholar | PubMed |
Muñoz MM, Feeley KJ, Martin PH, Farallo VR (2022) The multidimensional (and contrasting) effects of environmental warming on a group of montane tropical lizards. Functional Ecology 36(2), 419-431.
| Crossref | Google Scholar |
Muscarella R, Galante PJ, Soley-Guardia M, Boria RA, Kass JM, Uriarte M, Anderson RP (2014) ENMeval: an R package for conducting spatially independent evaluations and estimating optimal model complexity for Maxent ecological niche models. Methods in Ecology and Evolution 5(11), 1198-1205.
| Crossref | Google Scholar |
Nazarenko LS, Tausnev N, Russell GL, Rind D, Miller RL, Schmidt GA, Bauer SE, Kelley M, Ruedy R, Ackerman AS, Aleinov I, Bauer M, Bleck R, Canuto V, Cesana G, Cheng Y, Clune TL, Cook BI, Cruz CA, Del Genio AD, Elsaesser GS, Faluvegi G, Kiang NY, Kim D, Lacis AA, Leboissetier A, LeGrande AN, Lo KK, Marshall J, Matthews EE, McDermid S, Mezuman K, Murray LT, Oinas V, Orbe C, García-Pando CP, Perlwitz JP, Puma MJ, Romanou A, Shindell DT, Sun S, Tsigaridis K, Tselioudis G, Weng E, Wu J, Yao M-S (2022) Future climate change under SSP emission scenarios with GISS-E2.1. Journal of Advances in Modeling Earth Systems 14(7), e2021MS002871.
| Crossref | Google Scholar |
Neel LK, Logan ML, Nicholson DJ, Miller C, Chung AK, Maayan I, Degon Z, DuBois M, Curlis JD, Taylor Q, Keegan KM, McMillan WO, Losos JB, Cox CL (2021) Habitat structure mediates vulnerability to climate change through its effects on thermoregulatory behavior. Biotropica 53(4), 1121-1133.
| Crossref | Google Scholar |
Ortega-Andrade HM, Rodes Blanco M, Cisneros-Heredia DF, Guerra Arévalo N, López de Vargas-Machuca KG, Sánchez-Nivicela JC, Armijos-Ojeda D, Cáceres Andrade JF, Reyes-Puig C, Quezada Riera AB, Székely P, Rojas Soto OR, Székely D, Guayasamin JM, Siavichay Pesántez FR, Amador L, Betancourt R, Ramírez-Jaramillo SM, Timbe-Borja B, Gómez Laporta M, Webster Bernal JF, Oyagata Cachimuel LA, Chávez Jácome D, Posse V, Valle-Piñuela C, Padilla Jiménez D, Reyes-Puig JP, Terán-Valdez A, Coloma LA, Pérez Lara MB, Carvajal-Endara S, Urgilés M, Yánez Muñoz MH (2021) Red List assessment of amphibian species of Ecuador: a multidimensional approach for their conservation. PLoS ONE 16(5), e0251027.
| Crossref | Google Scholar | PubMed |
Ortega Z, Pérez-Mellado V (2016) Seasonal patterns of body temperature and microhabitat selection in a lacertid lizard. Acta Oecologica 77, 201-206.
| Crossref | Google Scholar |
Parsons ECM (2016) Why IUCN should replace “Data Deficient” conservation status with a precautionary “Assume Threatened” status – a cetacean case study. Frontiers in Marine Science 3, 193.
| Crossref | Google Scholar |
Penman TD, Pike DA, Webb JK, Shine R (2010) Predicting the impact of climate change on Australia’s most endangered snake, Hoplocephalus bungaroides. Diversity and Distributions 16(1), 109-118.
| Crossref | Google Scholar |
Peters RL, Darling JDS (1985) The greenhouse effect and nature reserves: global warming would diminish biological diversity by causing extinctions among reserve species. BioScience 35(11), 707-717.
| Crossref | Google Scholar |
Phillips SJ, Anderson RP, Schapire RE (2006) Maximum entropy modeling of species geographic distributions. Ecological Modelling 190(3–4), 231-259.
| Crossref | Google Scholar |
Powney GD, Grenyer R, Orme CDL, Owens IPF, Meiri S (2010) Hot, dry and different: Australian lizard richness is unlike that of mammals, amphibians and birds. Global Ecology and Biogeography 19(3), 386-396.
| Crossref | Google Scholar |
QGIS Development Team (2022) QGIS Geographic Information System. Open Source Geospatial Foundation Project. Available at http://qgis.osgeo.org
R Core Team (2023) ‘R: a language and environment for statistical computing.’ (R Foundation for Statistical Computing: Vienna, Austria) Available at https://www.R-project.org/
Román-Palacios C, Wiens JJ (2020) Recent responses to climate change reveal the drivers of species extinction and survival. Proceedings of the National Academy of Sciences 117(8), 4211-4217.
| Crossref | Google Scholar |
Sadlier RA, Couper P, Colgan DJ, Vanderduys E, Rickard E (2005) A new species of scincid lizard, Saproscincus eungellensis, from mid-eastern Queensland. Memoirs of the Queensland Museum 51(2), 559-571.
| Google Scholar |
Sannolo M, Mangiacotti M, Sacchi R, Scali S (2014) Keeping a cool mind: head–body temperature differences in the common wall lizard. Journal of Zoology 293(2), 71-79.
| Crossref | Google Scholar |
Sazima I (2023) Thigmothermic behaviour on paved paths after sunset by the weasel skink Saproscincus mustelinus. The Herpetological Bulletin 166, 40-42.
| Crossref | Google Scholar |
Scheffers BR, Edwards DP, Macdonald SL, Senior RA, Andriamahohatra LR, Roslan N, Rogers AM, Haugaasen T, Wright P, Williams SE (2017) Extreme thermal heterogeneity in structurally complex tropical rain forests. Biotropica 49(1), 35-44.
| Crossref | Google Scholar |
Scoccimarro E, Peano D, Gualdi S, Bellucci A, Lovato T, Fogli PG, Navarra A (2021) Extreme events representation in CMCC-CM2 high and very-high resolution general circulation models. Geoscientific Model Development Discussions 1-18.
| Crossref | Google Scholar |
Seastedt TR, Oldfather MF (2021) Climate change, ecosystem processes and biological diversity responses in high elevation communities. Climate 9(5), 87.
| Crossref | Google Scholar |
Sopniewski J, Scheele BC, Cardillo M (2022) Predicting the distribution of Australian frogs and their overlap with Batrachochytrium dendrobatidis under climate change. Diversity and Distributions 28(6), 1255-1268.
| Crossref | Google Scholar |
Tingley R, Macdonald SL, Mitchell NJ, Woinarski JCZ, Meiri S, Bowles P, Cox NA, Shea GM, Böhm M, Chanson J, Tognelli MF, Harris J, Walke C, Harrison N, Victor S, Woods C, Amey AP, Bamford M, Catt G, Clemann N, Couper PJ, Cogger H, Cowan M, Craig MD, Dickman CR, Doughty P, Ellis R, Fenner A, Ford S, Gaikhorst G, Gillespie GR, Greenlees MJ, Hobson R, Hoskin CJ, How R, Hutchinson MN, Lloyd R, McDonald P, Melville J, Michael DR, Moritz C, Oliver PM, Peterson G, Robertson P, Sanderson C, Somaweera R, Teale R, Valentine L, Vanderduys E, Venz M, Wapstra E, Wilson S, Chapple DG (2019) Geographic and taxonomic patterns of extinction risk in Australian squamates. Biological Conservation 238, 108203.
| Crossref | Google Scholar |
Torkkola JJ, Chauvenet ALM, Hines H, Oliver PM (2022a) Distributional modelling, megafires and data gaps highlight probable underestimation of climate change risk for two lizards from Australia’s montane rainforests. Austral Ecology 47(2), 365-379.
| Crossref | Google Scholar |
Torkkola JJ, Wilmer JW, Hutchinson MN, Couper PJ, Oliver PM (2022b) Die on this hill? A new monotypic, microendemic and montane vertebrate genus from the Australian Wet Tropics. Zoologica Scripta 51(5), 483-497.
| Crossref | Google Scholar |
VanDerWal J, Shoo LP, Williams SE (2009) New approaches to understanding late Quaternary climate fluctuations and refugial dynamics in Australian wet tropical rain forests. Journal of Biogeography 36(2), 291-301.
| Crossref | Google Scholar |
Walther G-R, Post E, Convey P, Menzel A, Parmesan C, Beebee TJC, Fromentin J-M, Hoegh-Guldberg O, Bairlein F (2002) Ecological responses to recent climate change. Nature 416(6879), 389-395.
| Crossref | Google Scholar | PubMed |
Warren DL, Seifert SN (2011) Ecological niche modeling in Maxent: the importance of model complexity and the performance of model selection criteria. Ecological Applications 21(2), 335-342.
| Crossref | Google Scholar | PubMed |
Williams SE, Bolitho EE, Fox S (2003) Climate change in Australian tropical rainforests: an impending environmental catastrophe. Proceedings of the Royal Society of London. Series B: Biological Sciences 270(1527), 1887-1892.
| Crossref | Google Scholar |
Williams SE, Shoo LP, Isaac JL, Hoffmann AA, Langham G (2008) Towards an integrated framework for assessing the vulnerability of species to climate change. PLoS Biology 6(12), e325.
| Crossref | Google Scholar | PubMed |
Williams SE, Williams YM, VanDerWal J, Isaac JL, Shoo LP, Johnson CN (2009) Ecological specialization and population size in a biodiversity hotspot: how rare species avoid extinction. Proceedings of the National Academy of Sciences 106(supplement_2), 19737-19741.
| Crossref | Google Scholar |
Woinarski JCZ, Murphy BP, Palmer R, Legge SM, Dickman CR, Doherty TS, Edwards G, Nankivell A, Read JL, Stokeld D (2018) How many reptiles are killed by cats in Australia? Wildlife Research 45(3), 247-266.
| Crossref | Google Scholar |
Wotherspoon L, de Oliveira Caetano GH, Roll U, Meiri S, Pili A, Tingley R, Chapple DG (2024) Inferring the extinction risk of Data Deficient and not evaluated Australian squamates. Austral Ecology 49(2), e13485.
| Crossref | Google Scholar |