Spatial and temporal variation of marine megafauna off coastal beaches of south-eastern Queensland, Australia
Maddison C. Cross
A
B
C
D
E
Abstract
Coastal beach environments provide habitats for marine megafauna, including turtles, rays, marine mammals and sharks. However, accessing these variable energy zones has been difficult for researchers by using traditional methods.
This study used drone-based aerial surveys to assess spatio-temporal variation of marine megafauna across south-eastern Queensland, Australia.
Drones were operated at five south-eastern Queensland beaches. Megafauna sightings and key variables including location, month and turbidity were analysed to assess variation across locations.
Overall, 3815 individual megafauna were detected from 3273 flights. There were significant differences in the composition of megafauna assemblages throughout the year and among beaches, with megafaunal sightings in >80% of flights conducted off North Stradbroke Island.
Strong temporal presence was found that is congruent with other studies examining seasonality. This supports the use of drones to provide ecological data for many hard-to-study megafauna species and help inform long-term sustainable management of coastal ecosystems.
Results indicated that environmental conditions can influence the probability of sighting marine megafauna during aerial surveys.
Keywords: aerial survey, beaches, continental shelf, drones, ecological, RPA, temporal, UAV.
Introduction
Marine megafauna off coastal beaches
Marine megafauna are key components of marine ecosystems and include many charismatic species that are socially, economically and culturally valued by humans worldwide (Pimiento et al. 2020). Herein, we refer to ‘megafauna’ as groups of large animals, including mammals, rays, sharks, turtles, seabirds and large pelagic fishes (Fuentes et al. 2016). Coastal beaches worldwide provide important nesting and feeding grounds for the seven sea turtle species, including the green (Chelonia mydas) and loggerhead turtle (Caretta caretta) (Limpus and Coffee 2019; Schofield et al. 2019). These waters also support a diverse assemblage of elasmobranchs, which act as high-level trophic predators critical to maintain the balance in coastal food webs (Barreto et al. 2021). The shallow and productive waters of coastal beaches provide critical habitat for both resident and migratory shark and ray species that use these areas as nursery and foraging grounds (DiGiacomo et al. 2020), including tiger sharks (Galeocerdo cuvier), shovelnose rays (Rhinopristiformes) and green turtles (Chelonia mydas) (Holmes et al. 2014; Limpus and Coffee 2019; Colefax et al. 2020a). Nursery habitats provide an advantage to young because they reduce early mortality rate by decreasing the risk of predation and generally provide an abundance of prey sources (Yokota and Lessa 2006). According to the International Union for Conservation and Nature (IUCN), approximately one-third of marine megafauna species are at risk of extinction (see https://www.iucnredlist.org, accessed 17 September 2023). As shore-based activities and coastal developments increase, baseline knowledge of megafauna that inhabit these ecosystems and the processes that manage their populations is sorely needed.
Influence of environmental factors on marine megafauna
To understand the spatial dynamics of marine megafauna in coastal ecosystems, it must be recognised that shallow-water environments are exposed to highly variable oceanic conditions. The patchy distribution of megafaunal species is influenced by biotic and abiotic environmental cues occurring at various temporal and spatial scales (Haulsee et al. 2015). At the scale of hours, weeks or years, these coastal ecosystems can experience major changes in temperature, depth, turbidity and bathymetry. Fluctuations in rainfall, tidal changes and seasonal weather patterns also occur on varied temporal scales (DiGiacomo et al. 2020). Hays et al. (2016) stated that these factors can interact both directly (physiology of megafauna) and indirectly (physiology of megafauna prey) with marine megafauna across varied temporal scales. As the majority of large marine animals has been documented to migrate long-distances, it is often thought that their directed movements are influenced by spatial and temporal variations in essential prey resources (Couturier et al. 2011).
Rainfall events and warmer temperatures are linked to increases in phytoplankton, a vital biological component that establishes ocean food webs (Lee et al. 2018). Blooms in phytoplankton enhance productivity, providing megafauna with food sources and favourable foraging grounds (Meynecke et al. 2006). The variation in marine megafaunal assemblages can also change because of spatial factors, such as distance from other habitats (coral reefs, seagrass beds and estuaries), proximity to continental shelf and the presence of marine protected areas (Barnes 2017). Some coastal waters are also heavily influenced by estuarine plumes, which make substantial nutrient and energy contributions to nearby habitats (Connolly et al. 2009). These inputs, and the resulting enhanced biological activity, may play a role in the variation of megafauna presence across time and space (Loneragan 1999).
Studying marine megafauna in coastal waters
Faunal assemblages off coastal beaches have been primarily assessed using boat-based fishing surveys, or other passive methods such as baited remote underwater video systems (BRUVS) (Olds et al. 2018). However, these methods are not always suitable for large marine animals because of their wide range, protection status and spatial presence in coastal ecosystems (Kelaher et al. 2020). Telemetry methods, such as satellite and acoustic tracking, offer efficient monitoring of species movement, location and aggregations (Dudgeon et al. 2008; Kock et al. 2013). However, both are costly and restricted by animal-capture considerations and tag-retention duration (Gredzens et al. 2014; Pereira et al. 2023). Effective sampling of air-breathing megafauna has traditionally been conducted by active surveillance (e.g. land- and vessel-based) (Hawkins and Gartside 2008). However, once underwater, accurate observation of behaviours is challenging, with animals hard to track until they re-surface. Traditional aerial surveys have typically been conducted using manned aircrafts (e.g. helicopters and light planes), consisting of a pilot and an observer (Kelaher et al. 2023). In recent times, remotely piloted aerial systems (RPAS), hereafter called drones, have become increasingly used as a tool for marine science research (Barreto et al. 2021; Kelaher et al. 2023).
Compared with traditional survey methods, drones are time-efficient, cost-effective and have shown significant value in detecting and observing fauna that may be dangerous or difficult to study (Butcher et al. 2021). Additionally, because many species of megafauna are threatened, drones provide a non-invasive approach to surveying these species with limited visual and auditory impact on the target individual (Bourke et al. 2023). Drones have been used in marine environments for quantifying fauna presence (Kelaher et al. 2020; Ayres et al. 2021) and behaviour (Torres et al. 2018; Fettermann et al. 2019), as well as for detecting and monitoring sharks as a method of shark control for beach users (Colefax et al. 2020b; Butcher et al. 2021). A recent study conducted by the Queensland Government Department of Agriculture and Fisheries (DAF) and Surf Life Saving Queensland (SLSQ) demonstrated this, when comparing this non-lethal measure with traditional, lethal shark-bite mitigation measures such as nets and drumline shark-culling measures (SharkSmart drone trial, see Mitchell et al. 2022). Overall, drone detection of marine megafauna has the potential to play a significant role in the conservation of threatened species, calculate population estimates (Rees et al. 2018), guide management decisions (Monteforte et al. 2022), contribute to citizen science (Dudgeon et al. 2019) and assist in the designation of future Marine Protected Areas (MPAs) (Gredzens et al. 2014).
Given the paucity of our understanding of the spatial and temporal patterns of marine megafauna species off coastal beaches in south-eastern Queensland (SEQ), 12 months of drone surveys conducted as part of the SharkSmart drone trial were utilised to (1) determine temporal and spatial variations in marine megafauna assemblages (specifically species abundance and richness) across several SEQ beaches and (2) quantify the influence of environmental conditions on the sightability of marine megafauna.
Materials and methods
Drone-trial locations
Drones were operated at five SEQ beaches as part of the Queensland SharkSmart drone trial. A total of 3273 drone surveys occurred at two beaches on the Gold Coast (Southport Main Beach and Burleigh Beach), two beaches on the Sunshine Coast (Alexandra Headland and Coolum North) and one beach on North Stradbroke Island (Main Beach) (Fig. 1). Trial sites were selected on the basis of the recommendations of the Cardno report of alternative approaches to shark control in Queensland (Cardno 2019), and Civil Aviation Safety Authority (CASA) regulations regarding restricted airspace and other no-fly areas, for example, within 5.5 km of an airport. Other key factors that determined the selection of these locations were the presence of Surf Life Saving Queensland (SLSQ) services, high year-round visitation of beach users, high water clarity, proximity to river mouths and historical catch of potentially dangerous sharks (Mitchell et al. 2022) (see Table 1 for location characteristics).
Map of beach locations surveyed by the Queensland SharkSmart drone trial in south-eastern Queensland, Australia. The solid brown line indicates the edge of the continental shelf.
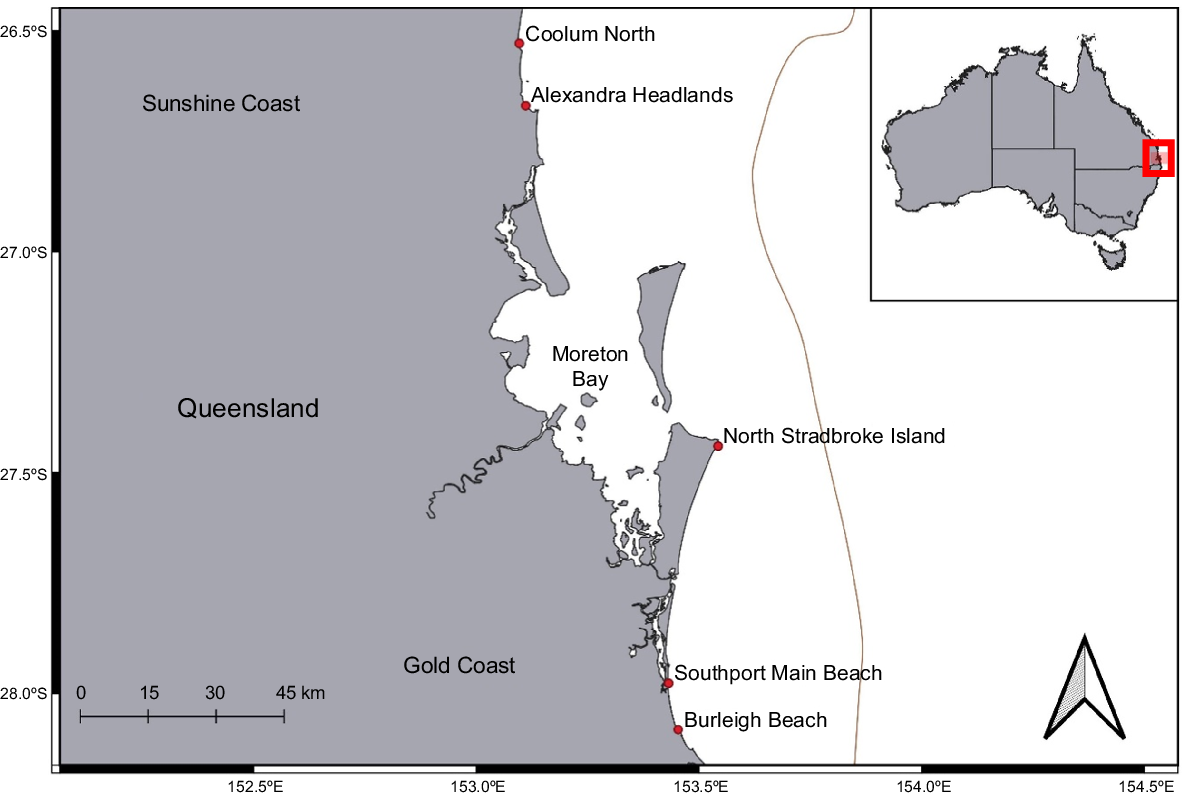
Location | Site characteristics | ||||
---|---|---|---|---|---|
Proximity to river mouths (km) | Proximity to the edge of the continental shelf (km) | Proximity to nearest town (km) | Available habitats | ||
Coolum North | 1.12 | 58 | 0.64 | Shallow rocky reefs, sandy beach | |
Alexandra Headland | 2.11 | 53 | 0.32 | Shallow rocky reefs, sandy beach | |
North Stradbroke Island | 39 | 23 | 1.08 | Rocky reefs, Point Lookout Headland, adjacent Moreton Bay, sandy beach | |
Southport Main Beach | 9.8 | 42 | 0.51 | Sandy beach | |
Burleigh Beach | 1.48 | 39 | 0.47 | Shallow rocky reefs, sandy beach |
Experimental design
DJI Mavic 2 Enterprise Zoom drones were operated by certified SLSQ pilots from September 2020 to October 2021 on weekends, public holidays and school holidays to coincide with periods of highest public use of beaches. The lens of theses drones had a field of view of ~77° and a sensor of 20 million effective pixels. No filters were added to the drones during the trial. Drones were deployed at 30-min intervals from when the beach opened (usually 07:00–08:00 hours) until 12:00 hours, because higher wind speeds typically occur in the afternoon in SEQ (>20 knots, ~37 km h−1), conditions in which these particular drones could not be operated. This resulted in six to eight flights per day. Flights conducted twice per hour allowed time in between for pilots to record environmental and flight log data and change drone batteries.
Flights lasted between 15 and 20 min, with flight paths being designed as 400-m transects behind the surf break (Fig. 2) (see Mitchell et al. 2022 for detailed drone transects). Flights were conducted with manual control as weather and tide variables significantly changed the position of the surf break. To stay within visual line of sight of the drone, flight paths covered a section of each beach close to the SLSQ swimming flags. Drones were flown at ~10–20 km h−1 at a constant altitude of 60 m from where they were deployed, with the camera set at a 45° angle facing the direction of travel. These values provided a balanced height for identifying marine life, a field of view of ~110 m and helped reduce glare. Drones were set to record continuously in 4K video to maximise the resolution for detecting megafauna and to allow identification to the species level.
Data collection
Videos were reviewed at double speed using VideoLan VLC media player (ver. 3.0.16, see https://www.videolan.org/vlc/index.html) until marine megafauna were sighted. Once fauna were sighted, the footage was analysed, using frame by frame view if necessary to identify and count individual animals. During the review of video footage, all marine megafauna observed were recorded and identified to the lowest possible taxonomic resolution. In some cases, this was to a species level (e.g. Indo-Pacific leopard shark, Stegostoma tigrinum), whereas others were identified to genus level (e.g. whaler shark, Carcharhinus spp.). All marine megafauna were individually counted per flight conducted using MaxN statistic, where abundance is calculated as the maximum number of individuals of each taxa observed in a single frame of view (Shah Esmaeili et al. 2021). Megafauna sightings (presence or absence) were recorded at the beach level to enable abundance and richness comparison among locations.
No animal ethics approval was required because the study was observational only.
Environmental data were recorded once per flight for each location. Specific environmental parameters included air temperature (°C), sea state (Beaufort scale), wind speed (km h−1), wind direction, turbidity (%), cloud cover (Oktas), barometric pressure (hPa), sea-surface temperature (°C) and chlorophyll concentration (mg L−1). Surface temperature and chlorophyll data were collected by satellite oceanography (Integrated Marine Observing System, Australian Ocean Data Network, see https://portal.aodn.org.au/search, accessed 20 December 2022), but high cloud cover and subsequent light reflectance resulted in data being unavailable for many days of this study. Rainfall data for the preceding 7 days for each beach location was downloaded from The Bureau of Meteorology (BOM) website (see http://www.bom.gov.au/climate/data/index.shtml, accessed 19 September 2022). This enabled an assessment of the influence of turbidity on sightability of megafauna at time periods >1 day because there can be a time lag between rain falling over land and the subsequent increase in turbidity from river outflows.
Statistical analysis
Generalised linear models (GLMs) were applied to determine which key environmental variables influenced the probability of sighting marine megafauna (Table 2). Response variables for analysis included megafauna species sightability (separated into air breathing v. non-air-breathing fauna), calculated as the presence or absence of individuals, and megafauna species abundance (specifically for leopard sharks, S. tigrinum, and turtles, Cheloniidae). These two groups were selected for abundance because a large number of them were sighted. Species richness, calculated as the number of different species found in a location, was also used to examine the variation in species composition across each location.
Variable or predictor | Unit of measurement | Rationale | Data source | |
---|---|---|---|---|
Location | Beach | The five locations have differences in depth, habitat type, level of exposure and continental shelf distance, which can influence the behaviour and movement of megafauna | Recorded by pilot | |
Time of day | Flight number (1–8) | The behaviour and movement of megafauna vary with the time of day. Time of day also affects the angle of sun, which can influence the intensity of glare thus limit visibility into the water column. | Recorded by pilot | |
Month | January–December | In south-eastern Queensland, there are seasonal changes in weather patterns, for example, high pressure systems occur in winter and bring low winds, dry weather and still sea states. | Recorded by pilot | |
Air temperature | °C | Rising temperatures are associated with some weather patterns and can lead to heavy rainfall, higher winds and affect the amount of dissolved oxygen in the water column (Garcia-Soto et al. 2021). | Bureau of Meteorology | |
Rainfall | Cumulative rainfall of previous week (mm) | Cumulative rainfall over the previous week can influence turbidity levels, for example, high rainfall can lead to large plumes of organic materials from rivers, therefore influencing the probability of sighting megafauna. | Bureau of Meteorology | |
Turbidity | 0–100% | Visibility into the water column from a drone is affected by turbidity. | Estimated by pilot | |
Wind speed | km h−1 | Wind speed is associated with most waves, for example, higher winds can lead to increased surface disturbance and therefore inhibit the likelihood of sighting megafauna. Drones could operate safely with winds up to 20 km h−1. | Bureau of Meteorology | |
Sea state | Beaufort scale (low = 1, high = 12) | Surface disturbance and turbidity of the water column is affected by sea state. | Bureau of Meteorology | |
Cloud cover | Oktas | Cloud cover affects the amount of sunlight that penetrates the water column, thus influencing megafauna sightability. | Estimated by pilot | |
Glare | 1 (low)−5 (high) scale | Visibility into the water column from drones can be affected by the amount of sun glare on the water surface. | Estimated by pilot | |
Atmospheric pressure | hPa | Weather patterns are affected by atmospheric pressure, for example, low pressure systems can cause high winds, rough sea states and heavy rain. Barometric pressure can also influence the movement and behaviour of megafauna (Heupel et al. 2003). | Bureau of Meteorology |
Response variables in the GLMs were modelled using a Poisson distribution. The distributions of predictor variables were checked, and a log + 1 transformation was applied to rainfall to account for overdispersion. The correlation of predictor variables were also checked using the cor() function to ensure all variable combinations had a Pearson correlation coefficient of <0.4. A series of models were run following a backwards stepwise model selection to drop predictor variables. The model of best fit was indicated by having the lowest Akaike information criterion (AIC, Akaike 1974). All statistical analyses were performed in RStudio (ver. 1.4.1711, Posit Software, PBC, Boston, MA, USA, see https://posit.co/products/open-source/rstudio/) in R (ver. 4.0.5, R Foundation for Statistical Computing, Vienna, Austria, see https://www.r-project.org/) . GLMs were implemented with the R package lme4 (ver. 1.1-30, see https://CRAN.R-project.org/package=lme4; Bates et al. 2015). The tidyverse package (ver. 1.3.2, see https://cran.r-project.org/package=tidyverse; Wickham et al. 2019) was also used in analysis for organising and plotting data.
Results
Marine megafauna sightings
In total, 3815 megafauna were observed across the five locations (Table 3). The number of sightings varied substantially across beaches, ranging from 227 megafauna sightings at Alexandra Headland, to 2273 sightings at North Stradbroke Island. Maximum megafaunal richness was eight across all locations, varying from three at both Alexandra Headland and Southport Main Beach, to eight at North Stradbroke Island. In total, megafauna were sighted on 23.3% of all flights. Megafauna sighting rates were highly variable across locations, ranging from 11.3% at Alexandra Headland and Southport Main Beach, to 84% at North Stradbroke Island (Table 3).
Location | Total megafauna abundance | Maximum megafaunal richness | Percentage of flights with megafauna sighted (%) | Total number of flights conducted at each location | |
---|---|---|---|---|---|
Coolum North | 358 | 4 | 18.3 | 727 | |
Alexandra Headland | 227 | 3 | 11.3 | 825 | |
North Stradbroke Island | 2273 | 8 | 84 | 325 | |
Southport Main Beach | 327 | 3 | 11.3 | 698 | |
Burleigh Beach | 630 | 4 | 26.2 | 698 | |
All SEQ locations combined | 3815 | 8 | 23.3 | 3273 |
Overall, megafauna sightings and the abundance of rays, sharks, turtles, cetacean and fish varied substantially across locations (Table 4). The most abundant and diverse marine megafauna assemblages were observed at North Stradbroke Island, followed by Burleigh Beach, whereas Alexandra Headland and Southport Main Beach had the least abundant and rich assemblages. Turtles (Cheloniidae) were the most commonly sighted megafauna, occurring in 49.5% of flights at North Stradbroke Island, 9.4% at Coolum North and 7.9% at Alexandra Headland. This was followed by stingrays (Dasyatidae), which occurred in 40.9% of flights at North Stradbroke Island, 14.6% at Burleigh Beach and 4.8% at Coolum North. North Stradbroke Island had significantly higher sightings of the majority of megafauna taxa despite having the fewest number of flights (Table 3).
Taxon | Location | |||||
---|---|---|---|---|---|---|
Coolum North | Alexandra Headland | North Stradbroke Island | Southport Main Beach | Burleigh Beach | ||
Stingray (Dasyatidae) | 4.8 | 1 | 40.9 | 4.4 | 14.6 | |
Manta and devil ray (Mobulidae) | 1.4 | 0.1 | 14.8 | 0 | 0.3 | |
Eagle ray (Myliobatidae) | 3.3 | 1.6 | 32.6 | 2 | 5.2 | |
Shovelnose ray and wedgefish (Rhinopristiformes) | 0 | 0 | 17.5 | 0 | 0.7 | |
Cownose ray (Rhinoptera neglecta) | 0.1 | 0.1 | 2.2 | 1.3 | 5.2 | |
Leopard shark (Stegostoma tigrinum) | 0 | 0 | 22.5 | 0.4 | 0.3 | |
Hammerhead shark (Sphyrna spp.) | 0 | 0.1 | 0.3 | 0 | 0 | |
White shark (Carcharodon carcharias) | 0 | 0 | 0 | 0.1 | 0 | |
Whaler shark (Carcharhinus spp.) | 0 | 0.4 | 19.1 | 0 | 6 | |
Turtle (Cheloniidae) | 9.4 | 7.9 | 49.5 | 1.3 | 0.7 | |
Dolphin (Delphinidae) | 0.6 | 0.1 | 19.1 | 0.6 | 0.1 | |
Dugong (Dugong dugong) | 0 | 0 | 0.6 | 0 | 0 | |
Humpback whale (Megaptera novaeangliae) | 0 | 0 | 1.2 | 0 | 0 | |
Large fish (e.g. tuna and cobia) | 5.4 | 3 | 22.8 | 3.2 | 4.7 |
Leopard sharks were the most frequently sighted shark species at North Stradbroke Island, being sighted in 22.5% of flights. Whaler sharks (Carcharhinus spp.) were the second-most frequently sighted sharks at North Stradbroke Island, occurring in 19.1% of flights, followed by 6% of flights at Burleigh Beach. Marine mammals were predominantly sighted at North Stradbroke Island, with dolphins (Delphinidae), dugongs (D. dugong) and humpback whales (M. novaeangliae) occurring in 19.1, 0.6 and 1.2% of flights respectively (Table 4).
Temporal variation of marine megafauna
The majority of megafauna groups exhibited temporal variation. Stingrays were sighted at most locations throughout the year. However, July had the highest number of sightings at North Stradbroke Island, whereas more were sighted at Burleigh in the January (Fig. 3). The highest sighting rates of cownose (R. neglecta) and devil rays (Mobula spp.) occurred at Burleigh during December and January respectively. Reef manta rays (M. alfredi) were most sighted at North Stradbroke Island during September and decreased notably in number as the year passed. This species also had more sightings at Coolum in June. Eagle (Myliobatidae) and shovelnose ray (Rhinopristiformes) sightings were highest at North Stradbroke Island during summer (January and December respectively), whereas at Burleigh sightings were highest in April (Fig. 3).
The sighting rate of dolphins was highest at North Stradbroke Island during January (Fig. 4). Dolphins were sighted at Coolum and Alexandra Headland only in winter (June–August). Dugongs were sighted only in August and June at North Stradbroke Island, along with the highest sightings of humpback whales, although a small number of whales were sighted in September. Sightings of large fish were similar year-round for all locations; however, North Stradbroke Island had the highest sighting rate during September. Higher sightings of fish were observed at the other four locations during autumn and winter (Fig. 4).
The most important factor influencing sightability of leopard sharks (S. tigrinum) and turtles (Cheloniidae) was month, explaining 58 and 22% of the deviance in the response variable respectively (Fig. 5, see Supplementary Tables S3 and S4 for further model outputs). Models including this variable had the lowest AIC. The probability of sighting leopard sharks was highest in December (0.1 ± 0.04) and lowest in August (<0.001 ± <0.001). The probability of sighting turtles was highest in November (0.95 ± 0.15) and lowest in March (0.07 ± 0.04).
Environmental factors influencing the sightability of marine megafauna
Generalised linear model outputs indicated that turbidity, month and location were the most important factors influencing the sightability of air-breathing marine megafauna (mammals and turtles), explaining 42% of the deviance in the response variable (see Table S1 for further model outputs). The model including these variables had the lowest AIC. Turbidity had a negative linear effect on the probability of sighting air-breathing megafauna, decreasing from 0.86 (±0.15 s.e.) when turbidity was 0%, to 0.39 (±0.11) when turbidity was highest (Fig. 6a). The likelihood of sighting air-breathing fauna was highest during October (0.91 ± 0.16) and lowest during May (0.35 ± 0.13) (Fig. 6b). The probability of sighting megafauna was highest at North Stradbroke Island (0.69 ± 0.11), followed by Coolum North and Alexandra Headland. The lowest probability of sighting megafauna was at Burleigh Beach (0.01 ± 0.004), followed by Southport Main Beach (Fig. 6c).
Influence of predictor variables on the sightability of air-breathing marine megafauna: (a) turbidity, (b) month and (c) location. Solid black lines indicate model-fitted values. Grey shaded areas indicate 95% confidence intervals.
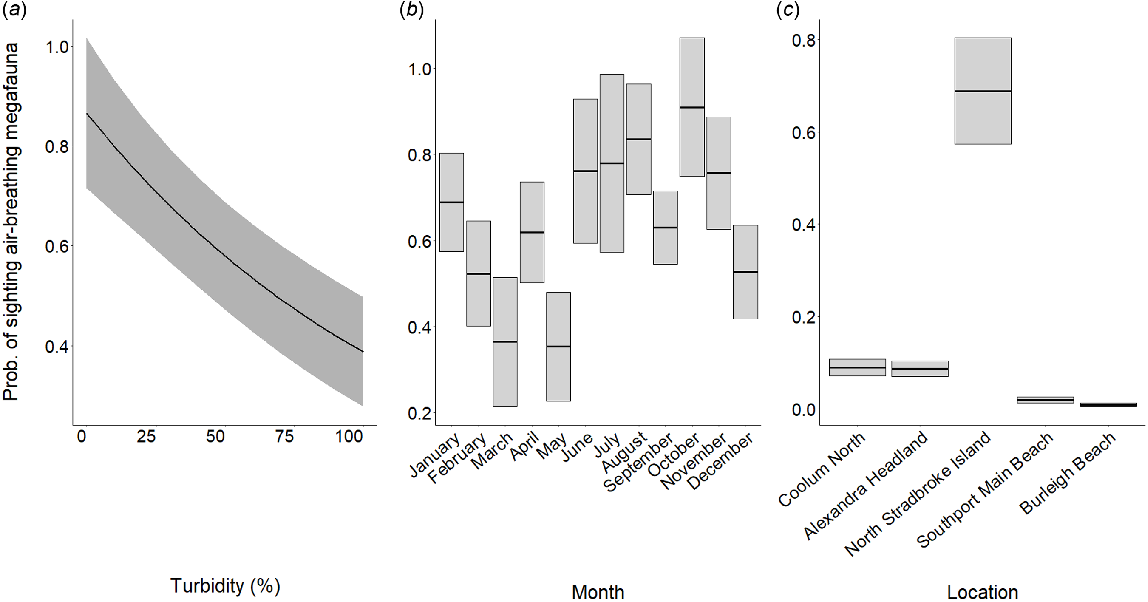
Model selection based on AIC for sightability of non-air-breathing megafauna (elasmobranchs and fish) included rainfall, turbidity, location and month, which collectively accounted for 44% of the deviance in the response variable (see Table S2 for further model outputs). Rainfall had a negative linear effect on the relative probability of sighting non-air-breathing megafauna, decreasing from 3.78 (±0.46 s.e.) when rainfall was lowest, to 1.49 (±0.17) when rainfall was 6 mm (Fig. 7a). A similar trend was seen with turbidity, ranging from 3.4 (±0.32) when there was no turbidity, to 1.46 (±0.25) when turbidity was at 100% (Fig. 7b). The relative probability of sighting megafauna was highest at North Stradbroke Island (2.67 ± 0.25), followed by Burleigh Beach and Coolum North. Alexandra Headland (0.11 ± 0.02) had the lowest likelihood of sighting megafauna, followed by Southport Main Beach (Fig. 7c). The relative likelihood of sighting non-air-breathing fauna was highest during March (2.78 ± 0.46) and lowest during September (0.8 ± 0.08) (Fig. 7d).
Discussion
Drone surveys found that assemblages of marine megafauna varied substantially among the sandy beaches surveyed and also by month. Spatiotemporal variation was identified in commonly sighted species including leopard sharks and reef manta rays, with some information being provided for rare faunal groups including dugongs.
Spatial variation of megafauna
North Stradbroke Island was found to have the highest detection of megafauna, as well as the greatest species richness and abundance of species compared with the other locations. There could be several explanations for the differences in megafaunal assemblages across these coastal beaches, such as the exposure to adjacent urbanisation or physical characteristics (e.g. wave climate), both of which are known to influence key aspects of beach ecology (Schlacher et al. 2007; Olds et al. 2018). Nevertheless, the availability of other habitats and the proximity to the edge of the continental shelf (23 v. >39 km) are plausible contributors to the high detection of all fauna species and groups at North Stradbroke Island (refer to Table 1 for site characteristics). The presence of shallow rocky reefs and the Point Lookout headland on North Stradbroke Island create one of the most prominent and complex habitats for the region. This habitat is likely to be abundant with potential prey items (Chilvers et al. 2005), possibly explaining the high detection of marine megafauna (Amir et al. 2005; Kelaher et al. 2020). This availability of complex habitats may additionally provide megafauna with zones used as cleaning stations (Couturier et al. 2011; Couturier et al. 2014), refuges from predators (DiGiacomo et al. 2020), breeding and nursery grounds (Shah Esmaeili et al. 2021). Turtles and dugongs are resident inside adjacent Moreton Bay, which has extensive seagrass meadows (Lanyon 2003; Limpus and Coffee 2019). The variable detection of these species on the ocean side of North Stradbroke Island is likely to be due to the absence of seagrass beds in these high-energy environments (Barnes 2017). Instead, these zones are likely to be selected for reproduction purposes for turtles, and thermoregulation may partially explain the occurrence of dugongs off this sandy beach. Although few dugongs were recorded here, they have been documented previously to temporarily leave the protected bay to adjacent oceanic waters that are significantly warmer, so as to minimise heat loss to the environment (Zeh et al. 2018).
Proximity to continental shelfs is known to attract animals closer to beaches, because it serves as a navigational aid during their migrations (e.g. sharks (Holmes et al. 2014) and humpback whales (Noad et al. 2006)). The shelf also facilitates the convergence of the warm East Australian Current (EAC) and colder oceanic currents (Armstrong et al. 2020). The convergence of these currents provides essential biophysical conditions, as well as periodic nutrient enrichment through upwellings, to support high concentrations of megafauna (Sleeman et al. 2007; Jaine et al. 2014). Key species including manta rays (Couturier et al. 2011), turtles (Limpus and Coffee 2019) and whales (Bolin et al. 2020) are known to use the EAC to facilitate their migrations to the southern Great Barrier Reef.
High sighting rates of megafauna also occurred at Burleigh Beach on the Gold Coast, which may be due to the close proximity to a productive river estuary, Tallebudgera Creek. The substantial contributions of nutrients and organic materials provided by estuarine plumes promotes primary and secondary production in nearby ecosystems, including sandy beaches (Connolly et al. 2009). These inputs and resulting enhanced biological activity may increase prey availability for megafauna (Loneragan 1999). Elasmobranchs were the most dominant group sighted off this location, with their movements possibly driven by factors associated with foraging (DiGiacomo et al. 2020), reproduction (Yokota and Lessa 2006; Schluessel et al. 2010) and anti-predatory strategies (Schlaff et al. 2014). Specifically, proximity to nearby rivers may explain the high occurrence of cownose rays off Gold Coast locations. This result is similar to the findings from the New South Wales trial, which identified that the beach adjacent to a river mouth had the highest abundance of cownose rays (Kelaher et al. 2020; Tagliafico et al. 2020).
Excluding turtles, the low probability of sighting large marine animals off Sunshine Coast locations may be due to the distance to the continental shelf (>53 km), and less suitable habitat (refer to Table 1 for location characteristics). In contrast, turtles are known to nest in small densities on Sunshine Coast beaches (Limpus 1993) and show site fidelity to these zones (Limpus et al. 1994).
Assessing the temporal variation of megafauna
The abundance of most megafauna groups peaked in austral spring–summer (September–February). This was possibly due to the increase in water temperature and related productivity (Meynecke et al. 2006), although chlorophyll concentrations and surface temperature could not be included in our analyses because of restrictions with data. In SEQ, dramatic increases in phytoplankton biomass have been reported to occur each spring, with little inter-annual variability; thus, these regular spring blooms may attract large marine animals (Lee et al. 2018). Furthermore, as most elasmobranchs are ectothermic (excluding lamnid sharks), the energy requirements of thermoregulation would be reduced by remaining within their thermal preference (Lee et al. 2018; Elston et al. 2022). Owing to similar morphologies within some taxonomic groups of elasmobranchs, the ability to identify to a species level was limited; thus, analysis of temporal variation was restricted to more generalised taxa.
Manta rays and leopard sharks exhibited strong temporal variation, with high detections in September and December respectively. These results are consistent with those of Couturier et al. (2011) and Dudgeon et al. (2008, 2013), who reported strong seasonal aggregations over the austral spring and summer in SEQ from photo-identification surveys and acoustic tracking. It is apparent that these species show the same temporal preference in the photo-ID work and acoustic tracking as they do in drone sightings, providing valid resolution for seasonal comparisons. Given the congruence of the leopard shark and manta ray data, this provides support for temporal patterns shown in the drone data for other species.
Indo-Pacific bottlenose dolphins are resident to North Stradbroke Island (Chilvers and Corkeron 2003) and, therefore, the increased sightings in spring–summer may be associated with mother–calf pods, because an increase in calving generally occurs at this time of year (Hawkins and Gartside 2008). Additionally, the variation (composition, size, movement) of dolphin pods may change routinely depending on the availability of prey, habitat type, reproductive state, behaviour and time of day (Chilvers and Corkeron 2003; Kelaher et al. 2020).
In general, this study found limited temporal influence on large pelagic fish assemblages off the coast of SEQ, Australia. Peaks in large fish abundance occurred in September at North Stradbroke Island. More research and ability to identify fish down to a species level is required to better understand the spatio-temporal variation in this region.
Environmental factors influencing the sightability of marine megafauna
Unless optimal conditions (e.g. calm, clear, shallow water <5 m deep) occur in all drone flights, the presence and detectability of megafauna can be influenced by environmental factors. Environmental factors including turbidity, rainfall, month and location can influence the probability of sighting marine megafauna off sandy beaches during aerial surveys (Fiori et al. 2017). Cumulative rainfall over the previous week can influence turbidity levels, contributing to a lag in correlation. High rainfall can lead to large plumes of organic materials from rivers and stir up bottom sediments, thus decreasing visibility into the water column. Therefore, detection probabilities of benthic organisms (e.g. rays) and fauna exhibiting diving behaviour are reduced (Hodgson et al. 2013). A similar result was reported in a recent New South Wales study, which indicated that turbidity had a strong negative influence on megafauna detectability (Butcher et al. 2019). Although rainfall had a negative relationship with megafauna sightability because of the connection with turbidity, it is known that rainfall has positive links with the coastal environment by increasing productivity (Schlacher et al. 2008; Connolly et al. 2009).
Warmer temperature and relative productivity during summer are plausible contributors to the higher detection of non-air-breathing megafauna during these months. The slightly higher sightings of air-breathing fauna during winter may be linked with annual whale migrations (Noad et al. 2006), and warmer ocean temperatures than in the sheltered Moreton Bay (Zeh et al. 2018). Temperature has been found to affect megafauna sighting rates in other drone-based studies, with higher rates being observed with an increasing temperature (Ayres et al. 2021; Elston et al. 2022). However, in the current study this did not have a significant influence on the sightability of marine megafauna. Other influencing factors include sea state, cloud cover, glare and time of day, although these effects were also not significant in this study (Hodgson et al. 2013; Colefax et al. 2018). Group size can also play a role in detecting megafauna (Dudgeon et al. 2018), because groups are more likely to be detected by pilots than individuals.
Future applications
Although drone technology offers new opportunities for marine megafauna detection off coastal beaches, there are a number of caveats in the data that must be acknowledged. The inability to determine whether it is the same animal being sighted on multiple flights or different animals is a limitation in this study, because drones cannot identify individual animals. The impact of water clarity on faunal sightability is another limitation faced. Although no megafauna may be detected on days with low clarity, it is possible that these animals are still present.
Owing to the wide range of marine megafauna observed in this drone study, there is a significant opportunity to conduct further research on specific species or groups. The ability to identify temporal trends to a valid resolution with drones also provides a useful tool that can be applied globally to document spatio-temporal patterns of megafauna. Additionally, the implementation of drone image-analysis software on a global scale can provide further insights into the habitat use of threatened species. This knowledge plays an important role in the conservation of threatened species, because it can assist in population estimates, guide management decisions, contribute to citizen science and inform the designation of marine protected areas by identifying critical habitats used by marine megafauna.
Data availability
The corresponding author can provide the datasets used in this study upon reasonable request.
Conflicts of interest
Christine Dudgeon is an editor for Marine and Freshwater Research but did not at any stage have editor-level access to this manuscript while in peer review, as is the standard practice when handling manuscripts submitted by an editor to this journal. Marine and Freshwater Research encourages its editors to publish in the journal and they are kept totally separate from the decision-making processes for their manuscripts. The authors have no further conflicts of interest to declare.
Declaration of funding
The SharkSmart drone trial was funded and delivered by the Queensland Government Department of Agriculture and Fisheries in collaboration with SLSQ.
Acknowledgements
The authors thank the SLSQ staff and pilots who contributed to this study by managing all aspects of drone operations and data collection at the beaches. We acknowledge the traditional custodians and Elders past, present and emerging, of the lands and waters on which this study was conducted. Dr Paul Butcher from the New South Wales Department of Primary Industries, and Dr Andrew Colefax and Dr Justin Meager are thanked for providing scientific advice on the design and implementation of the Queensland SharkSmart drone trial.
References
Akaike H (1974) A new look at the statistical model identification. IEEE Transactions on Automatic Control 19, 716-723.
| Crossref | Google Scholar |
Amir OA, Berggren P, Ndaro SGM, Jiddawi NS (2005) Feeding ecology of the Indo-Pacific bottlenose dolphin (Tursiops aduncus) incidentally caught in the gillnet fisheries off Zanzibar, Tanzania. Estuarine, Coastal and Shelf Science 63, 429-437.
| Crossref | Google Scholar |
Armstrong AJ, Armstrong AO, Bennett MB, McGregor F, Abrantes KG, Barnett A, Richardson AJ, Townsend KA, Dudgeon CL (2020) The geographic distribution of reef and oceanic manta rays (Mobula alfredi and Mobula birostris) in Australian coastal waters. Journal of Fish Biology 96(3), 835-840.
| Crossref | Google Scholar | PubMed |
Ayres KA, Ketchum JT, González-Armas R, Galván-Magaña F, Hearn A, Elorriaga-Verplancken FR, Hoyos-Padilla EM, Kajiura SM (2021) The use of an unoccupied aerial vehicle to survey shark species over sand and rocky-reef habitats in a marine protected area. Journal of Fish Biology 99(5), 1735-1740.
| Crossref | Google Scholar | PubMed |
Barnes RSK (2017) Patterns of benthic invertebrate biodiversity in intertidal seagrass in Moreton Bay, Queensland. Regional Studies in Marine Science 15, 17-25.
| Crossref | Google Scholar |
Barreto J, Cajaíba L, Teixeira JB, Nascimento L, Giacomo A, Barcelos N, Fettermann T, Martins A (2021) Drone-monitoring: improving the detectability of threatened marine megafauna. Drones 5(1), 14.
| Crossref | Google Scholar |
Bates D, Mächler M, Bolker B, Walker S (2015) Fitting linear mixed-effects models using lme4. Journal of Statistical Software 67(1), 1-48.
| Crossref | Google Scholar |
Bolin JA, Schoeman DS, Pizà-Roca C, Scales KL (2020) A current affair: entanglement of humpback whales in coastal shark-control nets. Remote Sensing in Ecology and Conservation 6(2), 119-128.
| Crossref | Google Scholar |
Bourke E, Raoult V, Williamson JE, Gaston TF (2023) Estuary stingray (Dasyatis fluviorum) behaviour does not change in response to drone altitude. Drones 7(3), 164.
| Crossref | Google Scholar |
Butcher PA, Piddocke TP, Colefax AP, Hoade B, Peddemors VM, Borg L, Cullis BR (2019) Beach safety: can drones provide a platform for sighting sharks? Wildlife Research 46(8), 701-712.
| Crossref | Google Scholar |
Butcher PA, Colefax AP, Gorkin RA, Kajiura SM, López NA, Mourier J, Purcell CR, Skomal GB, Tucker JP, Walsh AJ, Williamson JE, Raoult V (2021) The drone revolution of shark science: a review. Drones 5(1), 8.
| Crossref | Google Scholar |
Chilvers BL, Corkeron PJ (2003) Abundance of Indo-Pacific bottlenose dolphins, Tursiops aduncus, off point lookout, Queensland, Australia. Marine Mammal Science 19(1), 85-095.
| Crossref | Google Scholar |
Chilvers BL, Lawler IR, Macknight F, Marsh H, Noad M, Paterson R (2005) Moreton Bay, Queensland, Australia: an example of the co-existence of significant marine mammal populations and large-scale coastal development. Biological Conservation 122(4), 559-571.
| Crossref | Google Scholar |
Colefax AP, Butcher PA, Kelaher BP (2018) The potential for unmanned aerial vehicles (UAVs) to conduct marine fauna surveys in place of manned aircraft. ICES Journal of Marine Science 75(1), 1-8.
| Crossref | Google Scholar |
Colefax AP, Butcher PA, Pagendam DE, Kelaher BP (2020a) Comparing distributions of white, bull, and tiger sharks near and away from the surf break using three tech-based methods. Ocean & Coastal Management 198, 105366.
| Crossref | Google Scholar |
Colefax AP, Kelaher BP, Pagendam DE, Butcher PA (2020b) Assessing white shark (Carcharodon carcharias) behavior along coastal beaches for conservation-focused shark mitigation. Frontiers in Marine Science 7, 268.
| Crossref | Google Scholar |
Connolly RM, Schlacher TA, Gaston TF (2009) Stable isotope evidence for trophic subsidy of coastal benthic fisheries by river discharge plumes off small estuaries. Marine Biology Research 5(2), 164-171.
| Crossref | Google Scholar |
Couturier LIE, Jaine FRA, Townsend KA, Weeks SJ, Richardson AJ, Bennett MB (2011) Distribution, site affinity and regional movements of the manta ray, Manta alfredi (Krefft, 1868), along the east coast of Australia. Marine and Freshwater Research 62(6), 628-637.
| Crossref | Google Scholar |
Couturier LIE, Dudgeon CL, Pollock KH, Jaine FRA, Bennett MB, Townsend KA, Weeks SJ, Richardson AJ (2014) Population dynamics of the reef manta ray Manta alfredi in eastern Australia. Coral Reefs 33(2), 329-342.
| Crossref | Google Scholar |
DiGiacomo AE, Harrison WE, Johnston DW, Ridge JT (2020) Elasmobranch use of nearshore estuarine habitats responds to fine-scale, intra-seasonal environmental variation: observing coastal shark density in a temperate estuary utilizing unoccupied aircraft systems (UAS). Drones 4(4), 74.
| Crossref | Google Scholar |
Dudgeon CL, Noad MJ, Lanyon JM (2008) Abundance and demography of a seasonal aggregation of zebra sharks Stegostoma fasciatum. Marine Ecology Progress Series 368, 269-281.
| Crossref | Google Scholar |
Dudgeon CL, Lanyon JM, Semmens JM (2013) Seasonality and site fidelity of the zebra shark, Stegostoma fasciatum, in southeast Queensland, Australia. Animal Behaviour 85(2), 471-481.
| Crossref | Google Scholar |
Dudgeon CL, Dunlop RA, Noad MJ (2018) Modelling heterogeneity in detection probabilities in land and aerial abundance surveys in humpback whales (Megaptera novaeangliae). Population Ecology 60(4), 371-387.
| Crossref | Google Scholar |
Dudgeon CL, Kilpatrick C, Armstrong A, Armstrong A, Bennett MB, Bowden D, Richardson AJ, Townsend KA, Hawkins E (2019) Citizen science photographic identification of marine megafauna populations in the Moreton Bay Marine Park. In ‘Moreton Bay Quandamooka & Catchment: past, present, and future’. (Eds IR Tibbetts, PC Rothlisberg, DT Neil, TA Homburg, DT Brewer, AH Arthington) pp. 475–490. (Moreton Bay Foundation: Brisbane, Qld, Australia)
Elston C, Cowley PD, von Brandis RG, Lea J (2022) Stingray habitat use is dynamically influenced by temperature and tides. Frontiers in Marine Science 8, 754404.
| Crossref | Google Scholar |
Fettermann T, Fiori L, Bader M, Doshi A, Breen D, Stockin KA, Bollard B (2019) Behaviour reactions of bottlenose dolphins (Tursiops truncatus) to multirotor Unmanned Aerial Vehicles (UAVs). Scientific Reports 9, 8558.
| Crossref | Google Scholar |
Fiori L, Doshi A, Martinez E, Orams MB, Bollard-Breen B (2017) The use of unmanned aerial systems in marine mammal research. Remote Sensing 9(6), 543.
| Crossref | Google Scholar |
Fuentes MMPB, Chambers L, Chin A, Dann P, Dobbs K, Marsh H, Poloczanska ES, Maison K, Turner M, Pressey RL (2016) Adaptive management of marine mega-fauna in a changing climate. Mitigation and Adaptation Strategies for Global Change 21(2), 209-224.
| Crossref | Google Scholar |
Garcia-Soto C, Cheng L, Caesar L, Schmidtko S, Jewett EB, Cheripka A, Rigor I, Caballero A, Chiba S, Báez JC, Zielinski T, Abraham JP (2021) An overview of ocean climate change indicators: sea surface temperature, ocean heat content, ocean pH, dissolved oxygen concentration, arctic sea ice extent, thickness and volume, sea level and strength of the AMOC (Atlantic Meridional Overturning Circulation). Frontiers in Marine Science 8, 642372.
| Crossref | Google Scholar |
Gredzens C, Marsh H, Fuentes MMPB, Limpus CJ, Shimada T, Hamann M (2014) Satellite tracking of sympatric marine megafauna can inform the biological basis for species co-management. PLoS ONE 9(6), e98944.
| Crossref | Google Scholar |
Haulsee DE, Breece MW, Miller DC, Wetherbee BM, Fox DA, Oliver MJ (2015) Habitat selection of a coastal shark species estimated from an autonomous underwater vehicle. Marine Ecology Progress Series 528, 277-288.
| Crossref | Google Scholar |
Hawkins ER, Gartside DF (2008) Social and behavioural characteristics of indo-pacific bottlenose dolphins (Tursiops aduncus) in northern New South Wales, Australia. Australian Mammalogy 30, 71-82.
| Crossref | Google Scholar |
Hays GC, Ferreira LC, Sequeira AMM, Meekan MG, Duarte CM, Bailey H, Bailleul F, Bowen WD, Caley MJ, Costa DP, Eguíluz VM, Fossette S, Friedlaender AS, Gales N, Gleiss AC, Gunn J, Harcourt R, Hazen EL, Heithaus MR, Heupel M, Holland K, Horning M, Jonsen I, Kooyman GL, Lowe CG, Madsen PT, Marsh H, Phillips RA, Righton D, Ropert-Coudert Y, Sato K, Shaffer SA, Simpfendorfer CA, Sims DW, Skomal G, Takahashi A, Trathan PN, Wikelski M, Womble JN, Thums M (2016) Key questions in marine megafauna movement ecology. Trends in Ecology and Evolution 31(6), 463-475.
| Crossref | Google Scholar | PubMed |
Heupel MR, Simpfendorfer CA, Hueter RE (2003) Running before the storm: blacktip sharks respond to falling barometric pressure associated with Tropical Storm Gabrielle. Journal of Fish Biology 63(5), 1357-1363.
| Crossref | Google Scholar |
Hodgson A, Kelly N, Peel D (2013) Unmanned Aerial Vehicles (UAVs) for surveying marine fauna: a dugong case study. PLoS ONE 8(11), e79556.
| Crossref | Google Scholar |
Holmes BJ, Pepperell JG, Griffiths SP, Jaine FRA, Tibbetts IR, Bennett MB (2014) Tiger shark (Galeocerdo cuvier) movement patterns and habitat use determined by satellite tagging in eastern Australian waters. Marine Biology 161(11), 2645-2658.
| Crossref | Google Scholar |
Jaine FRA, Rohner CA, Weeks SJ, Couturier LIE, Bennett MB, Townsend KA, Richardson AJ (2014) Movements and habitat use of reef manta rays off eastern Australia: offshore excursions, deep diving and eddy affinity revealed by satellite telemetry. Marine Ecology Progress Series 510, 73-86.
| Crossref | Google Scholar |
Kelaher BP, Colefax AP, Tagliafico A, Bishop MJ, Giles A, Butcher PA (2020) Assessing variation in assemblages of large marine fauna off ocean beaches using drones. Marine and Freshwater Research 71(1), 68-77.
| Crossref | Google Scholar |
Kelaher BP, Monteforte KI, Morris SG, Schlacher TA, March DT, Tucker JP, Butcher PA (2023) Drone-based assessment of marine megafauna off wave-exposed sandy beaches. Remote Sensing 15(16), 4018.
| Crossref | Google Scholar |
Kock A, O’Riain MJ, Mauff K, Meÿer M, Kotze D, Griffiths C (2013) Residency, habitat use and sexual segregation of white sharks, Carcharodon carcharias in False Bay, South Africa. PLoS ONE 8(1), e55048.
| Crossref | Google Scholar | PubMed |
Lanyon JM (2003) Distribution and abundance of dugongs in Moreton Bay, Queensland, Australia. Wildlife Research 30, 397-409.
| Crossref | Google Scholar |
Lee KA, Roughan M, Harcourt RG, Peddemors VM (2018) Environmental correlates of relative abundance of potentially dangerous sharks in nearshore areas, southeastern Australia. Marine Ecology Progress Series 599, 157-179.
| Crossref | Google Scholar |
Limpus CJ (1993) The green turtle, Chelonia mydas, in Queensland: breeding males in the southern Great Barrier Reef. Wildlife Research 20(4), 513-523.
| Crossref | Google Scholar |
Limpus CJ, Couper PJ, Read MA (1994) The green turtle, Chelonia mydas in Queensland: population structure in a warm temperate feeding area. Memoirs of the Queensland Museum 35, 139-154.
| Google Scholar |
Loneragan NR (1999) River flows and estuarine ecosystems: implications for coastal fisheries from a review and a case study of the Logan River, southeast Queensland. Australian Journal of Ecology 24(4), 431-440.
| Crossref | Google Scholar |
Meynecke J-O, Lee SY, Duke NC, Warnken J (2006) Effect of rainfall as a component of climate change on estuarine fish production in Queensland, Australia. Estuarine, Coastal and Shelf Science 69(3-4), 491-504.
| Crossref | Google Scholar |
Monteforte KIP, Butcher PA, Morris SG, Kelaher BP (2022) The relative abundance and occurrence of sharks off ocean beaches of New South Wales, Australia. Biology 11, 1456.
| Crossref | Google Scholar | PubMed |
Noad MJ, Dunlop RA, Paton D, Cato DH (2006) Absolute and relative abundance estimates of Australian east coast humpback whales (Megaptera novaeangliae). Journal of Cetacean Research and Management (Special Issue) 3, 243-252.
| Crossref | Google Scholar |
Olds AD, Vargas-Fonseca E, Connolly RM, Gilby BL, Huijbers CM, Hyndes GA, Layman CA, Whitfield AK, Schlacher TA (2018) The ecology of fish in the surf zones of ocean beaches: a global review. Fish and Fisheries 19(1), 78-89.
| Crossref | Google Scholar |
Pereira JM, Clay TA, Reisinger RR, Ropert-Coudert Y, Sequeira AMM (2023) Editorial: Tracking marine megafauna for conservation and marine spatial planning. Frontiers in Marine Science 9, 1119428.
| Crossref | Google Scholar |
Pimiento C, Leprieur F, Silvestro D, Lefcheck JS, Albouy C, Rasher DB, Davis M, Svenning J-C, Griffin JN (2020) Functional diversity of marine megafauna in the Anthropocene. Science Advances 6(16), eaay7650.
| Crossref | Google Scholar |
Rees AF, Avens L, Ballorain K, Bevan E, Broderick AC, Carthy RR, Christianen MJA, Duclos G, Heithaus MR, Johnston DW, Mangel JC, Paladino F, Pendoley K, Reina RD, Robinson NJ, Ryan R, Sykora-Bodie ST, Tilley D, Varela MR, Whitman ER, Whittock PA, Wibbels T, Godley BJ (2018) The potential of unmanned aerial systems for sea turtle research and conservation: a review and future directions. Endangered Species Research 35, 81-100.
| Crossref | Google Scholar |
Schlacher TA, Dugan J, Schoeman DS, Lastra M, Jones A, Scapini F, McLachlan A, Defeo O (2007) Sandy beaches at the brink. Diversity and Distributions 13(5), 556-560.
| Crossref | Google Scholar |
Schlacher TA, Skillington AJ, Connolly RM, Robinson W, Gaston TF (2008) Coupling between marine plankton and freshwater flow in the plumes off a small estuary. International Review of Hydrobiology 93, 641-658.
| Crossref | Google Scholar |
Schlaff AM, Heupel MR, Simpfendorfer CA (2014) Influence of environmental factors on shark and ray movement, behaviour and habitat use: a review. Reviews in Fish Biology and Fisheries 24(4), 1089-1103.
| Crossref | Google Scholar |
Schluessel V, Bennett MB, Collin SP (2010) Diet and reproduction in the white-spotted eagle ray Aetobatus narinari from Queensland, Australia and the Penghu Islands, Taiwan. Marine and Freshwater Research 61, 1278-1289.
| Crossref | Google Scholar |
Schofield G, Esteban N, Katselidis KA, Hays GC (2019) Drones for research on sea turtles and other marine vertebrates – a review. Biological Conservation 238, 108214.
| Crossref | Google Scholar |
Shah Esmaeili Y, Corte GN, Checon HH, Gomes TRC, Lefcheck JS, Amaral ACZ, Turra A (2021) Comprehensive assessment of shallow surf zone fish biodiversity requires a combination of sampling methods. Marine Ecology Progress Series 667, 131-144.
| Crossref | Google Scholar |
Sleeman JC, Meekan MG, Wilson SG, Jenner CKS, Jenner MN, Boggs GS, Steinberg CC, Bradshaw CJA (2007) Biophysical correlates of relative abundances of marine megafauna at Ningaloo Reef, Western Australia. Marine and Freshwater Research 58(7), 608-623.
| Crossref | Google Scholar |
Tagliafico A, Butcher PA, Colefax AP, Clark GF, Kelaher BP (2020) Variation in cownose ray Rhinoptera neglecta abundance and group size on the central east coast of Australia. Journal of Fish Biology 96(2), 427-433.
| Crossref | Google Scholar | PubMed |
Torres LG, Nieukirk SL, Lemos L, Chandler TE (2018) Drone Up! Quantifying whale behavior from a new perspective improves observational capacity. Frontiers in Marine Science 5, 319.
| Crossref | Google Scholar |
Wickham H, Averick M, Bryan J, Chang W, McGowan LD, François R, Grolemund G, Hayes A, Henry L, Hester J, Kuhn M, Pedersen TL, Miller E, Bache SM, Müller K, Ooms J, Robinson D, Seidel DP, Spinu V, Takahashi K, Vaughan D, Wilke C, Woo K, Yutani H (2019) Welcome to the tidyverse. Journal of Open Source Software 4(43), 1686.
| Crossref | Google Scholar |
Yokota L, Lessa RP (2006) A nursery area for sharks and rays in Northeastern Brazil. Environmental Biology of Fishes 75(3), 349-360.
| Crossref | Google Scholar |
Zeh DR, Heupel MR, Hamann M, Jones R, Limpus CJ, Marsh H (2018) Evidence of behavioural thermoregulation by dugongs at the high latitude limit to their range in eastern Australia. Journal of Experimental Marine Biology and Ecology 508, 27-34.
| Crossref | Google Scholar |