Online screening assessment of lifetime exposure to traumatic brain injury: a pilot study of associations between exposure and health status
Karen A Sullivan
A
Abstract
This study aimed to conduct a pilot test of the feasibility and validity of administering an online screening measure of lifetime traumatic brain injury (TBI) exposure in Australia.
One hundred and fifty six adults (aged 18–65 years) were recruited from the community via snowball sampling (convenience sample). A cross-sectional online survey was deployed that included the Ohio State University Traumatic Brain Injury Identification Method (OSU TBI-ID) short form. Secondary measures assessed post-concussion symptoms (the Rivermead Post-Concussion Symptoms Questionnaire), negative affective states (depression, anxiety, and stress scales), and behavioural health risks (items from the 2014 Ohio Behavioural Risk Factor Surveillance System).
Online OSU TBI-ID feasibility was high (minimal missing data and attrition, high completion rate). A TBI history was successfully recorded by approximately 60% of participants. Validity testing, via investigation of expected associations with risk factors controlled, found that selected indices [Worst TBI, Multiple TBIs] were positively associated with worse post-concussion symptoms: P’s < 0.05, small–medium effects. Worst TBI was significantly related to one behavioural health risk, smoking. There were no other significant correlations between online OSU TBI-ID indices and secondary outcomes when accounting for covariates (P’s > 0.05).
Initial support was found for the feasibility and validity of an online screening measure of lifetime TBI exposure (LTE) in an Australian sample. Cautious interpretation is warranted because of study limitations, especially the small unrepresentative sample. Further studies could increase confidence in the feasibility and validity of online LTE screening.
Keywords: closed-head injury, concussion, head trauma, lifetime exposure, mild traumatic brain injury, minor head injury, online assessment, repetitive injury.
Introduction
Traumatic brain injury (TBI) is associated with lifelong consequences and is considered the leading cause of death and disability worldwide (Langlois et al. 2006). Even ‘mild’ TBI (mTBI) has been linked to long-term complications and negative consequences (Langlois et al. 2006). Predicting outcomes from one, let alone multiple TBIs, is challenging (Dennis et al. 2022). Further, cumulative TBI exposure is a suggested mechanism or risk factor for late life health consequences, including chronic traumatic encephalopathy (Dams-O’Connor et al. 2013). The very strong potential for serious long-term negative consequences from multiple TBIs has inspired discussion about the best methods for measuring lifetime TBI exposure (LTE; Dennis et al. 2022).
There are well-documented challenges in single TBI assessment and additional issues for lifetime assessment. These issues include weak consensus for defining TBI (Menon et al. 2010); reliance on symptom-based criteria (Dennis et al. 2022); selection biases in TBI research due to unrecognised, unreported, or untreated injuries (Dams-OʼConnor et al. 2014); and parameter variation (e.g. selection criteria; Corrigan et al. 2003). TBI effects can be masked, misattributed, or otherwise altered by numerous factors, such as incentives to over- (Jurick et al. 2016) or under-report symptoms (Meier et al. 2015), use of interviews vs questionnaires (Edmed and Sullivan 2014), and cognitive distortions (Voormolen et al. 2020). For LTE, there is also the challenge of retrospective assessment over an extended period, including childhood (McKinlay et al. 2016).
Despite the challenges, several LTE assessment methods have been proposed. These methods range from a single item (e.g. ‘How many times, if ever…have you had…[a] head injury?’) (Schofield et al. 2006b; Ilie et al. 2018); small-item sets (McKinlay et al. 2016); and structured, multi-item methods mimicking the clinical interview (e.g. Brain Injury Screening Questionnaire, Dams-OʼConnor et al. 2014); Ohio State University Traumatic Brain Injury Identification Method (OSU TBI-ID), Corrigan and Bogner 2007). A prior study (Dams-OʼConnor et al. 2014) reported that single item methods can miss >35% of cases identified by multi-item methods. Another study found that 15% of older adults (n = 559) screened positive for LTE using a multi-item method, about 80% of whom were previously unidentified (Schneider-Cline et al. 2019). Taken together, it seems that multi-item methods will be vital to advance understanding of LTE correlates for all TBIs (mild–severe).
The OSU TBI-ID short form is emerging as a leading LTE measure. This well-validated tool systematically elicits TBI history via a structured interview (Corrigan and Bogner 2007; Corrigan et al. 2013; McKinlay et al. 2017). The OSU TBI-ID can quantify TBI history in several ways via multiple indices, for example via a count of TBI exposures irrespective of severity. The OSU TBI-ID has been adopted in multi-centre, longitudinal TBI studies (Corrigan et al. 2013; Dams-O’Connor et al. 2013; Rabinowitz et al. 2020; Ohio State University Wexner Medical Centre 2022), and its usage is recommended by leading agencies (e.g. the National Institute of Neurological Disorder and Stroke 2019).
The findings from LTE studies that have used the OSU TBI-ID typically show that LTE is associated with increased behavioural health risks (BHRs), such as substance misuse, and poorer long-term health from a heightened risk of other conditions (Corrigan et al. 2013; Manchester et al. 2020). However, it remains unclear which indices are critical and whether increased BHRs are expected from exposure to any TBI or specific TBIs (e.g. severe injury or mild injury with loss of consciousness [LOC]; Rabinowitz et al. 2020). A recent study found that LTE metrics – with LOC- – were associated with increased risks, such as heavy drinking and poor general mental health (Feiss et al. 2022). Further studies must clarify the relation between LTE and BHRs, including which injuries (and by extension which OSU TBI-ID indices) show these relations.
A potential limiting factor for LTE research is that the OSU TBI-ID was designed as an in-person interview (Lequerica et al. 2018). To address this issue, a handful of studies have deployed other OSU TBI-ID formats, including a computer-assisted, telephone-administered version (Cuthbert et al. 2016) and online versions (Lequerica et al. 2018; Lequerica et al. 2021). These new formats could increase the ease of data collection from participants in distributed geographic regions (Lequerica et al. 2018). However, changing the OSU TBI-ID to other formats could introduce additional challenges. The interview can support conditional (follow-up/clarification) questions – a feature considered by some as critical for success (Ohio State University Wexner Medical Centre 2022) – and online surveys have specific biases (e.g. they may be susceptible to participant inattention and other data integrity threats; Oppenheimer et al. 2009; McKibben and Silvia 2017; Griffin et al. 2021).
To date, there have been a few studies on the feasibility and validity of an online form of the OSU TBI-ID (O-OSU TBI-ID). These studies, mostly from North America, have evaluated the completion rate (Lequerica et al. 2018, 2021) and replicated expected associations (e.g. between LTE and post-concussion symptoms (PCS); Lequerica et al. 2018). In general, the findings have been favourable (Lequerica et al. 2018), but none have included the data integrity checks now commonly deployed in online surveys (Griffin et al. 2021).
In Australia, there is a paucity of research on LTE and its correlates. There are three prior studies with selected (criminal justice [CJ]; Perkes et al. 2011; Moore et al. 2014) or unselected (community) samples (Butterworth et al. 2004) and two with adults (cf. Moore et al. 2014). One study surveyed 200 men in the CJ system (Schofield et al. 2006a, 2006b) and a comparative sample (200 community-dwelling men; Perkes et al. 2011). Using a single-item LTE and follow-up questions for ≥five incidents, the study found a high prevalence of LTE with LOC (65% CJ vs 32% community) and all TBI exposures (82% CJ vs 72% community) as well as increased risk of neuropsychiatric sequalae when TBI exposed (CJ sample). Another cross-sectional survey (Anstey et al. 2004; Butterworth et al. 2004) in ~8000 randomly selected community members used a single-item LTE measure: ‘…ever had a serious head injury where you became unconscious for >15 minutes?’. Consistent with general population studies outside of Australia, LTE with LOC was associated with poorer general physical and mental health on screening tests (Anstey et al. 2004; Butterworth et al. 2004). Given that the Australian data are almost 20 years old and not obtained using a multi-item method or a current definition for ‘serious’ TBI, there is merit in revisiting this issue, including the methodology for LTE screening.
This study had two aims. First, to conduct an initial pilot investigation of O-OSU TBI-ID as a potential tool for LTE measurement in Australia via a small-scale replication and extension of the O-OSU TBI-ID feasibility and validity study by Lequerica et al. (2018). Thus, it was expected that the O-OSU TBI-ID would be feasible (i.e. data captured in all sections) and there would be a positive association between Worst TBI and PCS (Lequerica et al. 2018). The extension added data integrity checks and examined relations between other LTE indices (e.g. Multiple TBIs) and correlates (i.e. BHRs, PCS).
Method
Participants
Participants were recruited at a major metropolitan university, including via a for-credit, university-hosted research participation pool (SONA), and externally via electronic communication within the researchers’ networks. A snowball method was employed, whereby the recipients of study information were encouraged to share it with their networks. Eligible participants were people aged ≥18 years with a valid protocol.
Measures
Based on prior research (Butterworth et al. 2004; Ilie et al. 2015; Osborn et al. 2018), a SI-TBI history was assessed: ‘Have you ever had a Traumatic Brain Injury (TBI) also referred to as a head injury?’.
The OSU TBI-ID is a 3–5 min structured (three-step) tool to determine LTE (Corrigan and Bogner 2007). The interview form has strong inter-rater reliability and acceptable test/retest reliability (Corrigan and Bogner 2007). Step 1 assesses LTE and injury cause (five yes/no questions; e.g. ‘In your lifetime, have you ever injured your head or neck in a car accident or…crashing…a bicycle…?’ A yes at Step 1 triggers deployment of Step 2 (event details; e.g. ‘Were you knocked out…?’). All participants complete Step 3 about a history of multiple TBIs. Three summary indices of LTE were used in this study: Worst TBI [WTBI]; Multiple TBIs [MultiTBI]; TBI number by severity [TBINS] (Corrigan and Bogner 2007).
The RPQ assesses the frequency and severity of 16 PCS. The RPQ has good psychometric properties (King et al. 1995). An example RPQ item is: ‘…Do you now (i.e. over the last 24 hours) suffer from headaches?’ Participants indicate symptom frequency and severity (0 = not experienced at all to 4 = a severe problem). The wording of the introduction to the RPQ was altered slightly to ensure participants without a TBI history would also record their experience of these symptoms. The total score range is 0–64, with higher scores indicating greater problems (King et al. 1995). In this study the RPQ was found to be reliable (α = 0.96).
The DASS is a 42-item self-report measure of three negative emotional states with good to excellent internal consistency (Lovibond and Lovibond 1995). An example item is, ‘I felt that I had nothing to look forward to.’ Participants rate each item for personal applicability over the past week (0 = did not apply… to 3 = applied…very much, or most of the time). Prior OSU TBI-ID studies have used the Beck Depression Inventory (e.g. Konrad et al. 2011); however, since the DASS was developed and normed in Australia, it was chosen for this study. The DASS subscales are scored by summing the responses (total score range = 0–42). Higher scores indicate a more negative emotional state (Lovibond and Lovibond 1995). In this study, the Cronbach’s alpha for each DASS subscale was >0.80.
The BRFSS is a telephone survey of BHRs and chronic health conditions (Centres for Disease Control and Prevention 2014). Several studies (Bogner et al. 2020; Manchester et al. 2020; Feiss et al. 2022) have used specific BRFSS items to examine relationships between TBI exposure and BHRs (e.g. substance use, depression, and days of poor mental health; Bogner et al. 2020). The current study used selected 2014 BRFSS items from the following sections: Health Status, Healthy Days, Health-Related Quality of Life (HRQoL), Tobacco Use, Marijuana Use, and Alcohol Consumption, since they were previously used in LTE studies. An example HRQoL item is, ‘How many days in the past 30 days was your physical health…not good?’. BRFSS items are individually interpreted. Higher scores on the BHR items indicate increased health risk.
Procedure
Project applications were lodged, reviewed, and approved by the Queensland University of Technology (ethical clearance no: 4145; health and safety clearance no: 1782). The survey was hosted on the online platform Qualtrics™ (Provo, UT) and opened from October 2021 to May 2022. An opt-in method (e.g. activating a link) provided access to study information (e.g. risks, benefits). Consent was probed with a forced-choice item (agree [to continue] or disagree [to exit]). Continuing participants completed a validity check (reCAPTCHA plugin1) and the SI-TBI, O-OSU TBI-ID, RPQ, DASS, and BRFSS. Skip logic was programed for conditional questions, including O-OSU TBI-ID Step 2. The duration of LOC was presented with three options (i.e. no LOC, <30 min–24 h, and >24 h). The participants quit the study directly or after opting in to receive a study summary and/or small reimbursement (i.e. prize draw entry [chance to win one of two $100 shopping vouchers] or 0.5% course credit [if eligible]).
Data analysis
The data were transferred to the Statistical Package for the Social Sciences (IBM SPSS Statistics™ version 28) and inspected for missing values, input errors, eligibility, and invalidity. Feasibility was established through examination of completed sections (see Lequerica et al. 2018). Analysis of Covariance (ANCOVA) tested for group differences in symptoms (RPQ or DASS subscale [dependent variables]) based on LTE, while controlling for age, gender, and membership of a TBI ‘at risk’ group (e.g. contact-sport players). The ANCOVA independent variable (group) was LTE (i.e. WTBI, four levels: no TBI, mTBI (no LOC), mild–moderate TBI [LOC < 30 min–24 h], or severe TBI [LOC > 24 h]; MultiTBI, three levels: 0, 1, or 2+ TBIs; or TBINS, three levels: multiple mTBIs [no LOC], multiple mild–moderate TBIs [LOC < 30 min–24 h], or multiple severe TBI [LOC > 24 h]). ANCOVA assumptions (Field 2018) were met with the exception of normality violations (RPQ, DASS subscales, positive skew, Shapiro–Wilk, P’s < 0.001). A square-root (RPQ, DASS-Depression, DASS-Stress) or logarithmic transformation (DASS-Anxiety) yielded approximately normal distributions. Analyses were run with and without transformations, with no differences noted. Therefore, non-transformed data were reported. Multivariable binomial logistic regression determined the association between LTE and BHRs while controlling for ‘at risk’ status, age, and gender. The reference group was ‘No TBI’ (WTBI, MultiTBI, TBINS). As per precedent (Bogner et al. 2020; Waltzman et al. 2021), frequencies and weighted percentages were estimated for BHRs and compared across subgroups. A significance level of <0.05 was used.
Results
Data screening
Nine participants were excluded because of extreme missing data, an invalid protocol (failed integrity check), or an implausible survey completion time of <3 min (a value we could only obtain with indiscriminate responding). One underage (17 years) and one older participant (outlier aged >95% trimmed sample mean age) were also excluded. The selection of participants is shown in Fig. 1.
Participant selection and study flow showing the participant exclusions (and reasons), completion of OSU TBI-ID sections, and study exit choices. Notes: OSU TBI-ID = Ohio State University Traumatic Brain Injury Identification Method. * = accessed the survey (e.g., by clicking the survey link). a = significant missing data, i.e., no response to almost all questions; 3.10% missing data); b = rushed response, survey duration <3 minutes (minimum pilot response time); c = study summary 16.6%, 2022; d = reimbursement with either course credit (n = 81) or entry (n = 62) for a prize draw 91.6%, 2022. Sixty-two participants entered the prize draw, which was drawn on 20 June 2022; 81 participants were awarded course credit (29 April 2022), and the study summary was provided to twenty-six participants (20 June 2022).
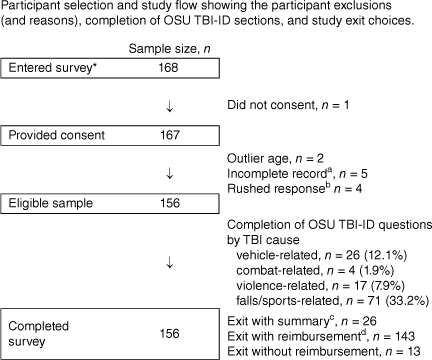
The completion rate for the OSU TBI-ID was examined with controls applied (e.g. where skip logic was used). Questions about TBI from all causes were answered (see Fig. 1). The average survey completion time (OSU TBI-ID plus other measures) was approximately 148 min (5% trimmed mean, range ~4–5398 min). The lengthy completions are likely due to the failure to close the survey browser.
Descriptive statistics: sample characteristics and LTE
The sample comprised 156 young–middle-aged adults (Mage = 25.27, s.d. = 9.89, range = 18–65 years, 73% women). Most participants were studying (68.6%). Most participants (~70%) were at risk for TBI, primarily from playing contact sport (62.2%). Approximately 60% reported at least one TBI (9%, SI-TBI; 59.6% OSU TBI-ID). This estimate was dependent on the assessment method, χ2(2) = 18.42, P < 0.001, with one participant recording a TBI on the SI-TBI only. The most common TBI cause was ‘falls/sport’ (33.2%). Of the sample, 46.8% recorded a mTBI (no LOC), 13.5% mild—moderate TBI (with LOC), and 0% severe TBI. Meanwhile, 44.2% reported 1 TBI and 16% reported 2+ TBIs. The percentage of men vs women with 2+ TBIs was 18.4% vs 14.9%, χ2(4) = 3.26, P = 0.515. The percentage of women vs men with mild–moderate TBI (with LOC) exposure was 12.2% vs 18.4%, but this difference was not significant; χ2(4) = 2.72, P = 0.606. Demographic information is shown in Table 1. Average scores for RPQ, DASS, and BRFSS are shown in Table 2. Table 3 displays BHR frequency data by three measures of LTE.
Characteristic | n | % sample | |
---|---|---|---|
Gender A | |||
Male | 38 | 24.4 | |
Female | 114 | 73.1 | |
Age (years) | |||
18–25 | 101 | 64.7 | |
26–35 | 38 | 24.4 | |
36–45 | 7 | 4.5 | |
45+ | 9 | 5.8 | |
Country of birth | |||
Australia | 124 | 79.5 | |
Other | 30 | 19.2 | |
Highest level of education completed | |||
High school graduate | 71 | 45.5 | |
TAFE/Certificate/Diploma/Advanced Diploma | 35 | 22.4 | |
3-year or 4-year undergraduate degree B | 38 | 24.4 | |
Postgraduate degree C | 10 | 6.4 | |
Primary occupation A | |||
Working | 43 | 27.6 | |
Studying | 107 | 68.6 | |
History of membership of an ‘at risk’ population | 112 | 71.8 | |
Contact sport | 97 | 62.2 | |
Learning disability | 8 | 5.1 | |
Drug or alcohol use | 7 | 4.5 | |
Psychiatric condition | 38 | 24.4 | |
Military service history | 7 | 4.5 | |
Contact with the criminal justice system | 5 | 3.2 | |
Lifetime TBI exposure | |||
Single-item measure (SI-TBI) D | |||
Yes | 14 | 9 | |
Maybe | 14 | 9 | |
No | 125 | 80.1 | |
Missing | 3 | 1.9 | |
Multi-item measure (OSU TBI-ID) | |||
Worst TBI | |||
No TBI | 62 | 39.7 | |
Mild (no LOC) | 73 | 46.8 | |
Mild–Moderate (LOC <30 min–24 h) | 21 | 13.5 | |
Severe (LOC >24 h) | 0 | 0 | |
Multiple TBI (Number, any severity) | |||
No TBI | 62 | 39.7 | |
1 TBI | 69 | 44.2 | |
2+ TBIs | 25 | 16 | |
TBI number by severity | |||
Multiple mTBIs (no LOC) | 23 | 14.7 | |
Multiple mild–moderate TBIs (LOC <30 min–24 h) | 2 | 1.3 | |
Multiple severe TBIs (LOC >24 h) | 0 | 0 | |
TBI cause/situation E | |||
Vehicle-related | 26 | 12.1 | |
Combat-related | 4 | 1.9 | |
Violence-related | 17 | 7.9 | |
Fall/sports-related | 71 | 33.2 | |
Presence of TBI in each ‘at risk’ subgroup F | |||
Contact sports | 61 | 62.9 | |
Learning disability | 3 | 37.5 | |
Drug or alcohol use | 5 | 71.4 | |
Psychiatric condition | 26 | 68.4 | |
Military service history | 7 | 100 | |
Contact with the criminal justice system | 3 | 60 |
Notes: N = 156 TBI = traumatic brain injury; LOC = loss of consciousness, duration in minutes or hours; TAFE = Technical and Further Education.
M | s.d. | Minimum | Maximum | ||
---|---|---|---|---|---|
RPQ, post-concussion symptoms | 31.79 | 12.73 | 16 | 69 | |
DASS, negative emotional states | |||||
Depression | 9.03 | 10.09 | 0 | 41 | |
Anxiety | 7.53 | 6.89 | 0 | 28 | |
Stress | 9.72 | 7.78 | 0 | 34 | |
BRFSS, behavioural health risk factors and outcomes | |||||
Poor physical health, n (days)* | 5.86 | 8.09 | 0 | 30 | |
Poor mental health, n (days)* | 11.38 | 9.72 | 0 | 30 | |
Binge drinking, ≥5 (men) or 4 alcoholic drinks (women) on an occasion** | 1.56 | 3.63 | 0 | 29 | |
Smoked cigarettes*** | 0.12 | 0.40 | 0 | 2 | |
Used marijuana or cannabis, n (days)* | 0.43 | 2.63 | 0 | 25 |
N = 156. RPQ = Rivermead Post-concussion Symptoms Questionnaire. DASS = depression, anxiety, and stress scales. BRFSS = 2014 Behavioural Risk Factor Surveillance System. Item stem: *No. of days in the last 30 days…. **How many times in the past 30 days did you have… ***Item response scale: 0 = not at all, 1 = some days, 2 = every day.
Multiple TBIs | Worst TBI | TBI number by severity | ||||||||||||
---|---|---|---|---|---|---|---|---|---|---|---|---|---|---|
2014 BRFSS item | N days | No TBI | 1 TBI | 2 + TBIs | % (2 + TBIs) | Mild (no LOC) | % | Mild–Moderate (LOC > 30 min–24 h) | % | Multiple Mild (no LOC) | % | Multiple Mild–Moderate (LOC > 30 min–24 h) | % | |
Poor physical health | 0 | 20 | 19 | 13 | 25 | 24 | 46.2 | 8 | 15.3 | 12 | 23.1 | 1 | 1.9 | |
>2 | 30 | 36 | 10 | 13.2 | 37 | 48.7 | 9 | 11.8 | 9 | 11.8 | 1 | 1.3 | ||
>14 | 12.5 | 10 | 12 | 2 | 11 | 41.7 | 3 | 12.5 | 2 | 8.3 | 0 | 0 | ||
Poor mental health | 0 | 9 | 9 | 2 | 10 | 7 | 35 | 4 | 20 | 2 | 10 | 0 | 0 | |
>2 | 23 | 33 | 15 | 21.1 | 40 | 56.3 | 8 | 11.3 | 13 | 18.3 | 2 | 2.8 | ||
>14 | 26 | 25 | 8 | 13.6 | 25 | 42.4 | 8 | 13.6 | 8 | 13.6 | 0 | 0 | ||
Smoking behaviour | Not at all | 60 | 57 | 22 | 0.6 | 63 | 45.3 | 16 | 11.5 | 20 | 14.4 | 2 | 1.4 | |
Some days | 1 | 8 | 2 | 1.3 | 8 | 72.7 | 2 | 18.2 | 2 | 18.2 | 0 | 0 | ||
Everyday | 0 | 4 | 1 | 13.5 | 2 | 40 | 3 | 60 | 0 | 0 | 0 | 0 | ||
Binge drinking | 0 | 35 | 36 | 17 | 19.3 | 41 | 46.6 | 12 | 13.6 | 17 | 19.3 | 0 | 0 | |
1–4 | 18 | 22 | 5 | 11.2 | 22 | 48.9 | 5 | 11.2 | 4 | 8.9 | 1 | 2.2 | ||
5–10 | 2 | 9 | 1 | 8.3 | 8 | 66.7 | 2 | 16.7 | 1 | 8.3 | 0 | 0 | ||
>10 | 2 | 0 | 0 | 0 | 0 | 0 | 0 | 0 | 0 | 0 | 0 | 0 | ||
Cannabis use | 0 | 55 | 60 | 25 | 17.9 | 67 | 47.9 | 18 | 12.9 | 23 | 16.4 | 2 | 1.4 | |
>2 | 0 | 3 | 0 | 0 | 2 | 66.7 | 1 | 33.3 | 0 | 0 | 0 | 0 | ||
>14 | 1 | 1 | 0 | 0 | 0 | 0 | 1 | 50 | 0 | 0 | 0 | 0 |
Notes: TBI, traumatic brain injury; LOC, loss of consciousness; mTBI, mild TBI; BRFSS, Behavioural Risk Factor Surveillance System;O-OSU TBI-ID, Online Ohio State University TBI Identification Method.
There was a statistically significant small–medium relationship between WTBI and PCS, F(2,148) = 3.39, P = 0.036, partial η2 = 0.044 (Table 4). Follow-up tests found one significant pairwise comparison; participants with a mild–moderate LTE (LOC <30 min–24 h) had significantly higher RPQ scores (Mdiff = 8.37, s.e. = 3.22, P = 0.031) than the ‘no TBI’ group. There was a marginal (small–medium) effect of MultiTBI on PCS, F(2,148) = 2.91, P = 0.05, partial η2 = 0.038. There was one significant follow-up pairwise comparison; participants with 2+ TBIs had significantly higher RPQ scores (Mdiff = 7.15 s.e. = 2.97, P = 0.05) than the ‘no TBI’ group. There was no significant effect of TBINS on PCS, F(2,147) = 1.95, P = 0.125. There were no significant group differences on DASS subscales (all P’s >0.05).
Model | Source | Sums of squares | d.f. | Mean square | F | P-value | |
---|---|---|---|---|---|---|---|
1 | Age | 45.75 | 1 | 45.75 | 0.31 | 0.578 | |
Gender | 739.36 | 1 | 739.36 | 5.02 | 0.027 | ||
Risk | 912.73 | 1 | 912.73 | 6.19 | 0.014 | ||
Worst TBI | 998.67 | 2 | 499.33 | 3.39 | 0.036 | ||
2 | Age | 18.34 | 1 | 18.34 | 0.12 | 0.726 | |
Gender | 656.85 | 1 | 656.85 | 4.43 | 0.037 | ||
Risk | 1003.27 | 1 | 1003.27 | 6.77 | 0.010 | ||
Multiple TBIs | 860.99 | 2 | 430.49 | 2.91 | 0.05 | ||
3 | Age | 21.03 | 1 | 21.03 | 0.14 | 0.708 | |
Gender | 663.22 | 1 | 663.22 | 4.45 | 0.037 | ||
Risk | 989.04 | 1 | 989.04 | 6.63 | 0.011 | ||
TBI number by severity | 870.99 | 3 | 327.14 | 1.95 | 0.125 |
Notes: ANCOVA, Analysis of Covariance; RPQ, Rivermead Post-Concussion Symptoms Questionnaire; d.f., degrees of freedom; TBI, traumatic brain injury; Worst TBI and Multiple TBIs are index scores from the O-OSU TBI-ID = Online Ohio State University TBI Identification Method.
Multivariable binomial logistic regression found that WTBI was related to one BHR, smoking, χ2(10) = 18.06, P = 0.05. Upon examination of the likelihood ratio rests and the covariates (age, gender, at risk group membership), WTBI was a significant contributor to smoking over and above the covariates, χ2(4) = 11.35, P = 0.023. Multivariable binomial logistic regression for the other indices (MultiTBI and TBINS) were not related to BHRs (Table 5).
O-OSU TBI-ID Index | BHRs | −2 Log likelihood | χ 2 | d.f. | P-value | |
---|---|---|---|---|---|---|
Worst TBI | Poor physical health | 213.23 | 11.82 | 10 | 0.297 | |
Poor mental health | 198.94 | 22.62 | 10 | 0.012 | ||
Smoking behaviour | 89.41 | 18.06 | 10 | 0.05 | ||
Binge drinking | 172.09 | 17.66 | 15 | 0.281 | ||
Cannabis use | 27.49 | 14.54 | 10 | 0.150 | ||
Multiple TBIs | Poor physical health | 213.92 | 16.12 | 10 | 0.097 | |
Poor mental health | 194.44 | 22.63 | 10 | 0.012 | ||
Smoking behaviour | 86.73 | 17.15 | 10 | 0.071 | ||
Binge drinking | 166.13 | 21.09 | 15 | 0.134 | ||
Cannabis use | 24.52 | 13.36 | 10 | 0.204 | ||
TBI number by severity | Poor physical health | 212.47 | 16.56 | 12 | 0.167 | |
Poor mental health | 193.20 | 25.25 | 12 | 0.014 | ||
Smoking behaviour | 85.91 | 17.97 | 12 | 0.117 | ||
Binge drinking | 164.09 | 24.51 | 18 | 0.139 | ||
Cannabis use | 24.52 | 13.36 | 12 | 0.343 |
Notes: d.f., degrees of freedom; TBI, traumatic brain injury; Worst TBI and Multiple TBIs are index scores from the Ohio State University TBI Identification Method; administered in online format for this study (O-OSU TBI-ID).
All three indices (WTBI, MultiTBI, and TBINS) were significantly related to poor mental health: overall model, WTBI, χ2(10) = 22.62, P = 0.012, MultiTBI, χ2(10) = 22.63, P = 0.012 and TBINS, χ2(12) = 25.25, P = 0.014; however, the likelihood ratio test showed this was due to the covariates and not LTE. All other analyses determined no significant relationships between indices WTBI, MultiTBIs and TBINS and BHRs, when accounting for covariates.
Discussion
This study had two aims. The primary aim was to conduct an initial pilot investigation of O-OSU TBI-ID as a potential tool for LTE screening in Australia. This was done via a small-scale replication and extension of Lequerica et al. (2018). We also performed an extension of Lequerica et al. (2018) by adding data integrity checks and examining novel relations between LTE indices (e.g. MultiTBIs) and the outcomes used in other LTE studies (e.g. BHRs). In broad terms, this study found initial support for the O-OSU TBI-ID.
We examined the feasibility of the O-OSU TBI-ID via the inspection of completion rates as per Lequerica et al. (2018). The O-OSU TBI-ID prompted participants to recall and record details about TBIs from all causes. Further, a data verification check was trialled with no issues reported. Although this study could not determine if this inclusion improved the data integrity, it did prevent bot completion. Adding this type of feature in future O-OSU TBI-ID applications would reflect best practice in online data collection (McKibben and Silvia 2017; Griffin et al. 2021).
We also tested for known associations between LTE indices and correlates, such as PCS. We found a positive association between WTBI and PCS, as previously demonstrated (Lequerica et al. 2018). Compared to people with no history of TBI, when covariates were controlled (age, sex, at risk status) a history of mild—moderate TBI (with LOC) was associated with worse PCS. Further, in the extension with the original controls (Lequerica et al. 2018), a lifetime history of TBI (2+ TBIs) was associated with worse PCS. However, lifetime TBINS was not significantly related to PCS, and no indices were significantly related to negative emotional states (depression, anxiety, or stress). A history of mild—moderate TBI (with LOC) (WTBI) was associated with one BHR (smoking). This analysis reveals further support for selected O-OSU TBI-ID indices (WTBI, MultiTBI), since they were correlated as expected with PCS.
To our knowledge, this study was the first deployment of the OSU TBI-ID in any format in Australia. We showed that about 1 in 10 people (13.5%) experienced at least one TBI with LOC (up to 24 h) in their lifetime. Prior North American OSU TBI-ID studies in larger samples have reported LTE rates that are higher (1 in 5 adults; Corrigan et al. 2018), lower (1 in 20 adults; Corrigan et al. 2013), or similar (1 in 15 older adults; Schneider-Cline et al. 2019). Our rate is higher than the prior Australian study with community members (i.e. between 5 and 6% prevalence; Anstey et al. 2004; Butterworth et al. 2004) and lower than the selected men-only sample (~32%; Perkes et al. 2011). If our findings are replicated, it could indicate that Australia has a higher potential burden from LTE than previously modelled. This could be due to changes in the injury rate, reporting practices, or a research artefact (ie. use of a multi-item vs single item LTE measure).
This study has several limitations. Online surveys carry a specific risk of bias. This survey deployed bot detection; but other validation techniques should be trialled (e.g. ‘honey pots’; Griffin et al. 2021). A true equivalence test would compare the LTE estimate from an online- vs interview-administration of the OSU TBI-ID, and the self-reported LTE estimate could also be cross-checked with LTE data from another source (e.g. medical history, if available). These comparisons are suggested for future research. The LTE data are self-reported, conferring a risk of error and inaccuracy (e.g. recall bias, uncorroborated injury). Some measures referred to ‘head injury’ when the intent was to measure TBI, including our single-item measure and parts of the original OSU-TBI. The wording of our single-item measure could have been confusing for some participants, and this may have reduced the validity of some measures. This study used an online version of the BRFSS, which to our knowledge has not been tried before. This change in the BRFSS administration format from the original telephone interview could affect our results. Cross-sectional studies cannot answer questions of causation; thus, the findings do not show that LTE caused poorer mental health, only that it was associated with it. Some O-OSU TBI-ID scores will differ from those in other studies (i.e. we could not distinguish between mild vs a moderate TBI (with LOC) because of administrative error; and our WTBI measure did include severe TBI). The small sample size and composition (largely female university students) is a limitation, and we excluded a handful of responses as ‘invalid attempts’ (e.g. extremely quick completions or mostly blank protocols), whereas these responses could in fact signal a problem with feasibility (i.e. that the measure could not actually be completed by all of the participants). Whilst the sample may be sufficient for an initial Australian pilot test of the O-OSU TBI-ID, including its feasibility, it does not support generalisable conclusions about LTE nor does it show how the test would function in specific groups with a heightened TBI exposure (e.g. adolescent contact-sports players or unsteady, older persons). Future studies with larger, representative samples are now needed to determine if the O-OSU TBI-ID can be used as a population screening tool for LTE.
This study suggests that estimates of LTE prevalence depend on assessment method (single- vs multi-item). Whichever method is used, the implementation should address the potential for expectancy bias or iatrogenesis (Dams-OʼConnor et al. 2014). A suggested strategy is to provide participants with information about this risk, including that taking the survey does not prove causation (Dams-OʼConnor et al. 2014).
There is strong interest in understanding LTE effects on health and functioning. The current study finds initial support for the feasibility of the O-OSU TBI-ID, including with added data validation, and in a new context (Australian sample). We successfully replicated some expected associations (Lequerica et al. 2018) and recommend further studies to identify key indices. However, the study limitations must also be kept in mind when considering the implications, including that this study was a small pilot investigation. The results of this pilot trial of this tool in Australia gives some confidence that the O-OSU TBI-ID could be successfully deployed in a larger population survey. In the longer term, this could eventually support improved LTE screening and understanding of LTE correlates, which in turn could improve resource planning and supports for people with TBI exposure.
Data availability
The data that support this study will be shared upon reasonable request to the corresponding author.
Acknowledgements
The authors thank study participants for volunteering their time to participate in this study and Ms Catherine Kennon for assistance with data integrity measures. The authors acknowledge A/Prof Obst for her statistical review of this manuscript. Both authors contributed to the conception, execution, and write up of this research.
References
Anstey KJ, Butterworth P, Jorm AF, Christensen H, Rodgers B, Windsor TD (2004) A population survey found an association between self-reports of traumatic brain injury and increased psychiatric symptoms. Journal of Clinical Epidemiology 57(11), 1202-1209.
| Crossref | Google Scholar | PubMed |
Bogner J, Corrigan JD, Yi H, Singichetti B, Manchester K, Huang L, Yang J (2020) Lifetime history of traumatic brain injury and behavioral health problems in a population-based sample. Journal of Head Trauma and Rehabilitation 35(1), E43-E50.
| Crossref | Google Scholar | PubMed |
Butterworth P, Anstey K, Jorm AF, Rodgers B (2004) A community survey demonstrated cohort differences in the lifetime prevalence of self-reported head injury. Journal of Clinical Epidemiology 57(7), 742-748.
| Crossref | Google Scholar | PubMed |
Corrigan JD, Bogner J (2007) Initial reliability and validity of the Ohio State University TBI Identification Method. Journal of Head Trauma and Rehabilitation 22(6), 318-329.
| Crossref | Google Scholar | PubMed |
Corrigan JD, Harrison-Felix C, Bogner J, Dijkers M, Terrill MS, Whiteneck G (2003) Systematic bias in traumatic brain injury outcome studies because of loss to follow-up. Archives of Physical Medicine and Rehabilitation 84, 153-160.
| Crossref | Google Scholar | PubMed |
Corrigan JD, Bogner J, Mellick D, Bushnik T, Dams-O’Connor K, Hammond FM, et al. (2013) Prior history of traumatic brain injury among persons in the Traumatic Brain Injury Model Systems National Database. Archives of Physical Medicine and Rehabilitation 94(10), 1940-1950.
| Crossref | Google Scholar | PubMed |
Corrigan JD, Yang J, Singichetti B, Manchester K, Bogner J (2018) Lifetime prevalence of traumatic brain injury with loss of consciousness. Injury Prevention 24(6), 396-404.
| Crossref | Google Scholar | PubMed |
Cuthbert JP, Whiteneck GG, Corrigan JD, Bogner J (2016) The reliability of a computer-assisted telephone interview version of the Ohio State University Traumatic Brain Injury Identification Method. Journal of Head Trauma and Rehabilitation 31(1), E36-E42.
| Crossref | Google Scholar | PubMed |
Dams-O’Connor K, Spielman L, Singh A, Gordon WA, Lingsma HF, Maas AI, et al. (2013) The impact of previous traumatic brain injury on health and functioning: a TRACK-TBI study. Journal of Neurotrauma 30(24), 2014-2020.
| Crossref | Google Scholar | PubMed |
Dams-OʼConnor K, Cantor JB, Brown M, Dijkers MP, Spielman LA, Gordon WA (2014) Screening for traumatic brain injury: findings and public health implications. Journal of Head Trauma and Rehabilitation 29(6), 479-489.
| Crossref | Google Scholar | PubMed |
Dennis EL, Baron D, Bartnik-Olson B, Caeyenberghs K, Esopenko C, Hillary FG, et al. (2022) ENIGMA brain injury: Framework, challenges, and opportunities. Journal of Human Brain Mapping 43(1), 149-166.
| Crossref | Google Scholar | PubMed |
Edmed SL, Sullivan KA (2014) Method of symptom assessment influences cognitive, affective and somatic post-concussion-like symptom base rates. Brain Injury 28(10), 1277-1282.
| Crossref | Google Scholar | PubMed |
Feiss R, Corrigan JD, Ding K, Beaulieu CL, Bogner J, Yang J (2022) Rural-urban differences in behavioral outcomes among adults with lifetime history of traumatic brain injury with loss of consciousness: 2016-2019 Ohio BRFSS. International Journal of Environmental Research and Public Health 19(3), 1678.
| Crossref | Google Scholar | PubMed |
Griffin M, Martino RJ, LoSchiavo C, Comer-Carruthers C, Krause KD, Stults CB, Halkitis PN (2021) Ensuring survey research data integrity in the era of internet bots. Quality & Quantity 56, 2841-2852.
| Crossref | Google Scholar | PubMed |
Ilie G, Adlaf EM, Mann RE, Ialomiteanu A, Hamilton H, Rehm J, Asbridge M, Cusimano MD (2015) Associations between a History of Traumatic Brain Injuries and Current Cigarette Smoking, Substance Use, and Elevated Psychological Distress in a Population Sample of Canadian Adults. Journal of Neurotrauma 32(14), 1130-1134.
| Crossref | Google Scholar |
Ilie G, Adlaf EM, Mann RE, Ialomiteanu A, Hamilton H, Rehm J, et al. (2018) Associations between self-reported lifetime history of traumatic brain injuries and current disability assessment in a population sample of Canadian adults. PLoS One 13(1), e0188908.
| Crossref | Google Scholar | PubMed |
Jurick SM, Twamley EW, Crocker LD, Hays CC, Orff HJ, Golshan S, et al. (2016) Postconcussive symptom overreporting in Iraq/Afghanistan Veterans with mild traumatic brain injury. Journal of Rehabilitation Research 53(5), 571-584.
| Crossref | Google Scholar | PubMed |
King NS, Crawford S, Wenden FJ, Moss NEG, Wade DT (1995) The Rivermead Post Concussion Symptoms Questionnaire: a measure of symptoms commonly experienced after head injury and its reliability. Journal of Neurology 242(9), 587-592.
| Crossref | Google Scholar | PubMed |
Konrad C, Geburek AJ, Rist F, Blumenroth H, Fischer B, Husstedt I, Arolt V, Schiffbauer H, Lohmann H (2011) Long-term cognitive and emotional consequences of mild traumatic brain injury. Psychological Medicine 41(6), 1197-1211.
| Crossref | Google Scholar |
Langlois JA, Rutland-Brown W, Wald MM (2006) The epidemiology and impact of traumatic brain injury: A brief overview. Journal of Head Trauma and Rehabilitation 21(5), 375-378.
| Crossref | Google Scholar | PubMed |
Lequerica AH, Lucca C, Chiaravalloti ND, Ward I, Corrigan JD (2018) Feasibility and Preliminary Validation of an Online Version of the Ohio State University Traumatic Brain Injury Identification Method. Archives of Physical Medicine and Rehabilitation 99(9), 1811-1817.
| Crossref | Google Scholar | PubMed |
Lequerica AH, Arango-Lasprilla JC, Krch D, Corrigan JD (2021) Assessing lifetime exposure to traumatic brain injury among an international sample of Spanish speakers. NeuroRehabilitation 48(1), 109-117.
| Crossref | Google Scholar | PubMed |
Lovibond PF, Lovibond SH (1995) The structure of negative emotional states: comparison of the Depression Anxiety Stress Scales (DASS) with the Beck Depression and Anxiety Inventories. Behaviour Research and Therapy 33(3), 335-343.
| Crossref | Google Scholar | PubMed |
Manchester K, Corrigan JD, Singichetti B, Huang L, Bogner J, Yi H, Yang J (2020) Current health status and history of traumatic brain injury among Ohio adults. Injury Prevention 26(2), 129-137.
| Crossref | Google Scholar | PubMed |
McKibben WB, Silvia PJ (2017) Evaluating the distorting effects of inattentive responding and social desirability on self‐report scales in creativity and the arts. The Journal of Creative Behavior 51(1), 57-69.
| Crossref | Google Scholar |
McKinlay A, Horwood L, Fergusson D (2016) Accuracy of self-report as a method of screening for lifetime occurrence of traumatic brain injury events that resulted in hospitalization. Journal of the International Neuropsychological Society 22, 717-23.
| Crossref | Google Scholar | PubMed |
McKinlay A, Corrigan JD, Bogner JA, Horwood LJ (2017) Obtaining a history of childhood traumatic brain injury using the Ohio State University TBI Identification Method to elicit adult recall. Journal of Head Trauma and Rehabilitation 32(6), E24-E28.
| Crossref | Google Scholar | PubMed |
Meier TB, Brummel BJ, Singh R, Nerio CJ, Polanski DW, Bellgowan PS (2015) The underreporting of self-reported symptoms following sports-related concussion. Journal of Science and Medicine in Sport 18(5), 507-511.
| Crossref | Google Scholar | PubMed |
Menon DK, Schwab K, Wright DW, Maas AI (2010) Position statement: Definition of traumatic brain injury. Archives of Physical Medicine and Rehabilitation 91(11), 1637-1640.
| Crossref | Google Scholar | PubMed |
Moore E, Indig D, Haysom L (2014) Traumatic Brain Injury, Mental Health, Substance Use, and Offending Among Incarcerated Young People. Journal of Head Trauma Rehabilitation 29(3), 239-247.
| Crossref | Google Scholar | PubMed |
National Institute of Neurological Disorder and Stroke (2019) Ohio State University TBI Identification Method Short Form. NINDS Common Data Elements. Retrieved from https://commondataelements.ninds.nih.gov/report-viewer/22972/Ohio%20State%20University%20TBI%20Identification%20Method%20Short%20Form
Ohio State University Wexner Medical Centre (2022) Screening for TBI Using the OSU TBI-ID Method. Retrieved from https://wexnermedical.osu.edu/neurological-institute/neuroscience-research-institute/research-centers/ohio-valley-center-for-brain-injury-prevention-and-rehabilitation/for-professionals/screening-for-tbi
Oppenheimer DM, Meyvis T, Davidenko N (2009) Instructional manipulation checks: Detecting satisficing to increase statistical power. Journal of Experimental Social Psychology 45(4), 867-872.
| Crossref | Google Scholar |
Osborn AJ, Mathias JL, Fairweather-Schmidt AK, Anstey KJ (2018) Traumatic Brain Injury and Depression in a Community-Based Sample: A Cohort Study Across the Adult Life Span. Journal of Head Trauma Rehabilitation 33(1), 62-72.
| Crossref | Google Scholar |
Perkes I, Schofield PW, Butler T, Hollis SJ (2011) Traumatic brain injury rates and sequelae: A comparison of prisoners with a matched community sample in Australia. Brain Injury 25(2), 131-141.
| Crossref | Google Scholar | PubMed |
Rabinowitz AR, Chervoneva I, Hart T, O’Neil-Pirozzi TM, Bogner J, Dams-O’Connor K, et al. (2020) Influence of prior and intercurrent brain injury on 5-year outcome trajectories after moderate to severe traumatic brain injury. Journal of Head Trauma and Rehabilitation 35(4), E342-E351.
| Crossref | Google Scholar | PubMed |
Schneider-Cline W, Bush E, McKelvey M (2019) Using the OSU TBI-ID method for screening rural, older adults: a mixed methods feasibility study. Brain Injury 33(7), 899-915.
| Crossref | Google Scholar | PubMed |
Schofield PW, Butler TG, Hollis SJ, Smith NE, Lee SJ, Kelso WM (2006a) Neuropsychiatric correlates of traumatic brain injury (TBI) among Australian prison entrants. Brain Injury 20(13-14), 1409-1418.
| Crossref | Google Scholar |
Schofield PW, Butler TG, Hollis SJ, Smith NE, Lee SJ, Kelso WM (2006b) Traumatic brain injury among Australian prisoners: Rates, recurrence and sequelae. Brain Injury 20(5), 499-506.
| Crossref | Google Scholar |
Voormolen DC, Cnossen MC, Spikman J, Polinder S, Iverson GL, de Koning M, van der Naalt J (2020) Rating of pre-injury symptoms over time in patients with mild traumatic brain injury: the good-old-days bias revisited. Brain Injury 34(8), 1001-1009.
| Crossref | Google Scholar | PubMed |
Waltzman D, Daugherty J, Sarmiento K, Proescholdbell S (2021) Lifetime history of traumatic brain injury with loss of consciousness and the likelihood for lifetime depression and risk behaviors: 2017 BRFSS North Carolina. Journal of Head Trauma and Rehabilitation 36(1), E40-E49.
| Crossref | Google Scholar | PubMed |