Recent trends in extratropical lows and their rainfall over Australia
Acacia Pepler
A
Abstract
Low pressure systems are an important source of rainfall in southern Australia, particularly deep lows that extend from the surface to at least 500 hPa. This paper uses multiple reanalyses to assess long-term trends in lows over the period 1959–2023, and identifies statistically significant decreasing trends in the number of surface low pressure systems near southern Australia during May–October, linked to a decrease in cyclogenesis near south-western Western Australia. Trends in lows at 500 hPa are also negative but weaker than at the surface, and are less consistent between reanalyses owing to less consistent observations through time. The spatial pattern of observed declines during the cool season is consistent with trends using eight CMIP6 models, but global climate models systematically underestimate the magnitude of the observed decline in surface lows. Trends in rainfall associated with lows are also shown, including assessing the sensitivity of trends to the specific years used. Despite well above average numbers of lows and enhanced rainfall during recent La Niña years 2020–2022, total rainfall from low pressure systems is declining during the cool season in south-east Australia. Trends in rainfall from lows are largest on the east coast, where they explain more than 70% of observed rainfall changes since the 1960s.
Keywords: Australia, climate change, CMIP6, cyclone, extratropical low, extreme precipitation, rainfall, reanalysis, trend.
1.Introduction
Low pressure systems are some of the most important weather systems for generating heavy rainfall in Australia (Lavender and Abbs 2013; Dowdy et al. 2019; Pepler et al. 2020) and globally (Hawcroft et al. 2012; Dowdy and Catto 2017; Utsumi et al. 2017). Australian lows can have a range of synoptic characteristics, including extratropical lows which are either embedded in or cut off from the mid-latitude westerlies (sometimes called Southern Ocean lows), tropical lows including monsoon depressions and tropical cyclones, as well as ex-tropical cyclones which have undergone extratropical transition, and subtropical or hybrid lows. Near the east coast, lows locally known as east coast lows (ECLs) are a particular category of interest, which often develop in an ‘easterly dip’ and can exhibit rapid intensification close to populated regions (Dowdy et al. 2019).
Collectively, low pressure systems play a critical role in water security through their contribution to widespread heavy rainfall and inflows to catchments (Pepler and Rakich 2010), as well as being a major cause of extreme weather including flooding, strong winds, storm surge, and coastal erosion (Dowdy and Catto 2017; Dowdy et al. 2019). Low pressure systems are also projected to become less common in the southern hemisphere midlatitudes in a warming climate (Grieger et al. 2014; Pepler and Dowdy 2021a; Priestley and Catto 2022), contributing to projected decreases in cool season rainfall in these regions (Pepler and Dowdy 2022).
Australia has very large interannual rainfall variability, and is strongly influenced by global climate drivers such as the El Niño–Southern Oscillation (ENSO) and the Indian Ocean Dipole (IOD) (Risbey et al. 2009). Nonetheless, recent decades have seen increasing rainfall during the warmer half of the year, particularly in the northern tropics, and declines in cool season rainfall in the southern half of the country (Dey et al. 2019; McKay et al. 2023), which is broadly consistent with climate model projections for the 21st century (CSIRO and Bureau of Meteorology 2015; Grose et al. 2020). The declining trends in cool season rainfall in southern Australia have been attributed to a range of factors, including an increase in the frequency of positive IOD and Central Pacific El Niño events (Freund et al. 2019; Abram et al. 2020, 2021), an intensification of the eastern Australian subtropical ridge (Timbal and Drosdowsky 2013), a positive trend in the summer and autumn Southern Annular Mode (SAM) (Fogt and Marshall 2020), and anthropogenic climate change (Timbal et al. 2010; Rauniyar and Power 2020; Rauniyar et al. 2023). Several studies have also identified a decrease in the rainfall associated with low pressure systems, particularly in the south-east (Risbey et al. 2013a; Pepler et al. 2021).
South-east Australia experienced a particularly severe drought in the years 2017–2019, including record-low numbers of low pressure systems (Pepler 2020). This event, in part linked to interannual variability including a Central Pacific El Niño and an unusually weak polar stratospheric vortex (Lim et al. 2021), resulted in significant negative trends in low pressure systems when calculated over the period 1979–2019 (Pepler 2020). Declining trends in rainfall associated with lows have also been detected over periods ending in the relatively dry years of 2009 (Risbey et al. 2013a) or 2015 (Pepler et al. 2021), with future declines in lows projected by global and regional climate models (Dowdy et al. 2013; Pepler and Dowdy 2022). By contrast, the period 2020–2022 was very wet across Australia, associated with three consecutive La Niña years, including notable prolonged heavy rainfall in eastern Australia linked to cut off lows in the mid-troposphere (Barnes et al. 2023). This raises the question of how robust previously identified trends in low pressure systems and rainfall are to the years used in analysis, and whether trends remain detectable outside of historically dry periods.
This paper presents newly calculated global datasets of surface and 500-hPa low pressure systems between 1959 and 2023 based on three reanalyses: NCEP1, JRA55 and ERA5. It then uses these datasets to assess trends in low pressure systems and their associated rainfall around Australia over the period 1959–2023, with a focus on southern Australia.
2.Methods
2.1. Feature tracking
Lows are tracked on 6-hourly sea level pressure (SLP; hPa) and 500-hPa geopotential height (Z500; m) using The University of Melbourne low tracking scheme (Murray and Simmonds 1991; Simmonds et al. 1999; Pepler 2020). Although there are a large number of tracking methods in the literature, this tracking method performs well against other approaches (Neu et al. 2013) and is frequently used in Australia, where it has good skill in identifying manually detected extratropical lows (Pepler et al. 2015).
This method first regrids all data to a common polar stereographic grid with an effective resolution of 1.5 at 30°S, and applies 2° of spatial smoothing to reduce the sensitivity of low detection to small-scale pressure anomalies. The method then detects maxima in the Laplacian of the data, before associating these with a minimum in the data. Filtering of surface lows is also applied over elevated topography (>1000 m). The mean Laplacian is calculated within a 2° radius of this centre, and all lows that exceed a minimum value (0.5 hPa per degree of latitude squared for surface lows, 2 m per degree of latitude squared for Z500 lows) are combined into tracks. These data have been made available online at https://doi.org/10.6084/m9.figshare.24086898.v1. Surface anticyclones are also tracked using the same process but not shown, with Laplacian averaged over a 10° radius required to be below −0.075 hPa per degree of latitude squared, consistent with Pepler et al. (2019).
For the results presented in this paper, lows are required to have a closed circulation and exceed a higher minimum intensity threshold: 0.6 hPa per degree of latitude squared for surface lows and 5 m per degree of latitude squared for 500-hPa lows. These thresholds were selected by testing correlations and biases between the ERA5 and JRA55 reanalyses over the periods 1959–1978 and 1979–2022, and minimise the impact of inhomogeneities in trends when compared to stronger thresholds. Although the thresholds are weaker than those in Pepler and Dowdy (2021b, 2022), those studies also included open depressions, necessitated by the use of daily mean geopotential height instead of 6-hourly Z500 data in this study. No minimum duration or movement criteria are applied, to allow the inclusion of short-lived but hazardous surface lows, such as those that develop on the east coast of Australia (Hopkins and Holland 1997). Cyclogenesis is based on the first location a low is identified for each track, which includes cases where the initial low is open or weak.
There are a large range of proposed methods for classifying and grouping lows, including based on thermal structure, synoptic characteristics, and track patterns (Speer et al. 2009; Browning and Goodwin 2013; Catto 2016; Cavicchia et al. 2019; Pepler and Dowdy 2021a). We apply the method of Pepler and Dowdy (2021a) to distinguish between shallow single-level cyclones and vertically developed lows that span much of the troposphere, as this approach has been linked to the likelihood of a low generating heavy rainfall (Dowdy et al. 2013; Pepler and Dowdy 2020).
Deep (i.e. vertically developed) lows are identified following the approach of Pepler and Dowdy (2020, 2021a). First, for each identified surface low, we identify any 500-hPa low at the same time and within a 500-km radius that exceeded the minimum intensity threshold. Secondly, for each track, we identify if an upper low was present at any time during the track, as the low may initially be detected at only a single level and the heaviest rainfall tends to occur slightly prior to the low reaching its maximum intensity and vertical extent (Pepler and Dowdy 2020). Any low that is part of such a track is considered a ‘deep low,’ with the remaining unmatched lows considered ‘shallow.’ Similarly, upper lows are defined as ‘deep’ if a surface low was located within a 500-km radius at any time during the track.
When plotting low occurrence, we expand each identified low using a radius of 10°, which broadly corresponds to the area over which lows influence rainfall (Hawcroft et al. 2012; Booth et al. 2018; Pepler and Dowdy 2020). These areas are used to calculate the proportion of UTC days where at least one low is present. These are calculated independently for surface and upper lows, as well as for deep lows, where either a deep surface or a deep upper low is identified. Deep lows produce a considerably higher proportion of mean and extreme rainfall than shallow lows identified at a single level (Pepler and Dowdy 2021b, 2022). In some cases, we additionally plot shallow surface lows, where no deep low is identified but a shallow surface low is present, and shallow upper lows, where a shallow upper low is present but neither a deep low nor a shallow surface low. These datasets are also used for matching the occurrence of low pressure systems with daily 0.05° gridded rainfall from Jones et al. (2009), based on rainfall recorded over the 24 h to 09:00 hours local time on the subsequent day (between 22:00 and 01:00 hours UTC, depending on season and location).
Means are shown for the seasons May–October and November–April; the cross-year season is referred to by the first year, with the final year being 2022–2023 (referred to as 2022). Changes in the number of days with a cyclone focus on the spatial average over southern Australia (33–43°S, 110–155°E) (Fig. 1), which is the region in which the largest changes are observed. In addition, we look at trends in both the frequency of lows and cyclogenesis for three subregions of interest. ECLs are identified as lows within a region bound by 25–43°S, 160°E, and the east coast of Australia; this region is similar to that used in Speer et al. (2009) and Pepler and Dowdy (2021b) but extended southward to include lows relevant to the east coast of Tasmania. The remaining regions of southern Australia are divided into south-west Western Australia (SWWA; 33–43°S, 110–125°E), an area of large declines in rainfall (Rauniyar et al. 2023), and the remaining areas centred on the Great Australian Bight (GAB).
Regions used in this study. Coloured regions indicate land areas used for calculated changes in low-related rainfall: the Eastern Seaboard (ESB), south-east Australia (SEA), Tasmania (TAS) and south-west Western Australia (SWWA). The black dashed rectangle indicates the region used for calculating trends in frequency for southern Australia, with land areas inside this box also described as southern Australia for rainfall analysis. Additional polygons indicate subregions for assessing trends in cyclone frequency and cyclogenesis: the east coast low region in the Tasman Sea (ECL), SWWA and the Great Australian Bight (GAB).
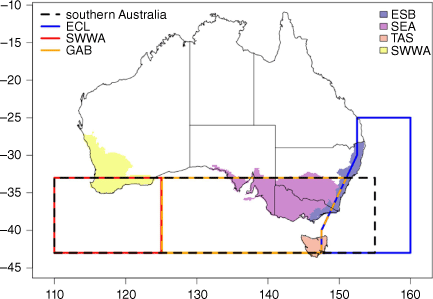
The average proportion of rainfall which can be associated with a cyclone within a 10° radius is reported for corresponding land areas south of 33°S as well as four subregions of Australia with different characteristic rainfall and trends, used for projections in Pepler and Dowdy (2022).
2.2. Datasets
Tracking was performed using three long-term reanalyses over the period 1959–2023: JRA55 (Kobayashi et al. 2015), ERA5 (Hersbach et al. 2020), and NCEP1 (Kalnay et al. 1996). Using multiple reanalyses increases the robustness of results, and helps to identify periods or regions where the reanalyses are not well constrained by the available observations. Although NCEP1 data are shown in timeseries plots and included in the dataset for legacy purposes, they are not used for detailed analysis owing to very large inhomogeneities in the frequency of upper lows prior to the start of the satellite era in 1979. Comparisons between reanalyses are separated into the satellite era (1979–2023) and the pre-satellite era (1959–1978). During the pre-satellite era there are fewer observations available to constrain the representation of lows within reanalyses, particularly at levels above the surface, potentially resulting in inhomogeneities in the derived datasets. Although these inhomogeneities would be less prevalent using centennial reanalysis ensembles such as the Twentieth Century Reanalysis (Slivinski et al. 2019), such reanalyses generally do not have data during the last decade and so cannot be used to understand recent variability or changes.
To put historical trends into context of trends expected from climate models, we supplement some analyses with lows tracked in eight global climate models (GCMs) from the 6th Coupled Model Intercomparison Project (CMIP6; Eyring et al. 2016). This represents all models with 6-hourly SLP and Z500 data available for the historical simulations over 1959–2014 on the Australian (NCI) node of the ESGF. Model details are listed in Table 1. In cases where multiple ensemble members were available, we prioritise those selected by Grose et al. (2023), as better representing the range of potential future changes in Australia. Six of the models used are priority models for Australian downscaling based on Grose et al. (2023); we additionally include MPI-ESM1-2-HR and MRI-ESM2-0.
Model | Ensemble member | Resolution | Reference | Surface lows (%) | 500-hPa lows (%) | |
---|---|---|---|---|---|---|
ERA5 | 0.25 × 0.25 | Hersbach et al. (2020) | 26 | 22 | ||
JRA55 | 1.25 × 1.25 | Kobayashi et al. (2015) | 25 | 20 | ||
NCEP1 | 2.0 × 2.0 | Kalnay et al. (1996) | 25 | 16 | ||
ACCESS-CM2 | r4i1p1f1 | 1.2 × 1.8 | Dix et al. (2019) | 16 | 10 | |
ACCESS-ESM1-5 | r6i1p1f1 | 1.2 × 1.8 | Ziehn et al. (2019) | 14 | 8 | |
CMCC-ESM2 | r1i1p1f1 | 0.9 × 1.3 | Lovato et al. (2021) | 21 | 11 | |
CNRM-ESM2-1 | r1i1p1f2 | 1.4 × 1.4 | Seferian (2018) | 19 | 12 | |
EC-Earth3 | r1i1p1f1 | 0.7 × 0.7 | EC-Earth Consortium (2019) | 19 | 16 | |
MPI-ESM1-2-HR | r1i1p1f1 | 0.9 × 0.9 | Jungclaus et al. (2019) | 23 | 15 | |
MRI-ESM2-0 | r1i1p1f1 | 1.1 × 1.1 | Yukimoto et al. (2019) | 23 | 12 | |
NorESM2-MM | r1i1p1f1 | 0.9 × 0.9 | Bentsen et al. (2019) | 23 | 12 |
For additional assessment of the influences of trends in surface lows in climate models, we make use of the ACCESS-ESM1.5 ensemble. This model has 10 ensemble members with 6-hourly SLP available over the historical period 1959–2014, with a range of simulated trends. This analysis is also supplemented by a small number of ensemble members with 6-hourly SLP data from the Detection and Attribution Model Intercomparison Experiment (DAMIP), including three ensemble members over the historical period with natural forcings only (hist-nat) and three members with greenhouse gas forcings only (hist-GHG). These members are used to assess the relationship between trends in lows and trends in other monthly mean climatological variables including temperature and zonal winds at 850, 700, 500 and 300 hPa and the derived static stability and Philips criterion measures (as per Frederiksen et al. 2016).
3.Climatology
Over the 1959–2014 period, the climatology of surface and 500-hPa lows is very similar between ERA5 and JRA55 (Fig. 2). Although lows at both levels occur most frequently in the extratropical storm tracks south of 45°S, both reanalyses capture the relatively high frequencies of both surface and 500-hPa lows over the Tasman Sea to the east of Australia, which is particularly pronounced during the cool months of May–October (Dowdy et al. 2019). This co-location results in a relatively high frequency of deep lows over south-eastern Australia, where they occur on ~20% of days and are responsible for more than 40% of total rainfall (Supplementary Fig. S1), consistent with Pepler and Dowdy (2022). All datasets also have a large frequency of lows over the west coast of Australia; these are primarily shallow lows during the warm half of the year typical of heat lows over a warm land surface. Upper level and deep lows are less common in northern Australia, particularly in JRA55, but the tracking method also detects cyclones with a deep warm core, including many tropical cyclones.
Average percentage of days during 1959–2014 with any 500-hPa low (a–d) or surface low (e–h) centre detected within a 10° radius, or a deep low at either level (i–l), in the ERA5 (a, e, i) and JRA55 (b, f, j) reanalyses and eight CMIP6 models (c, g, k). Ensemble mean difference (d, h, j) between the CMIP6 models (c, g, k) and ERA5 (a, e, i), with dots indicating where the bias is smaller than the difference between ERA5 and JRA55.
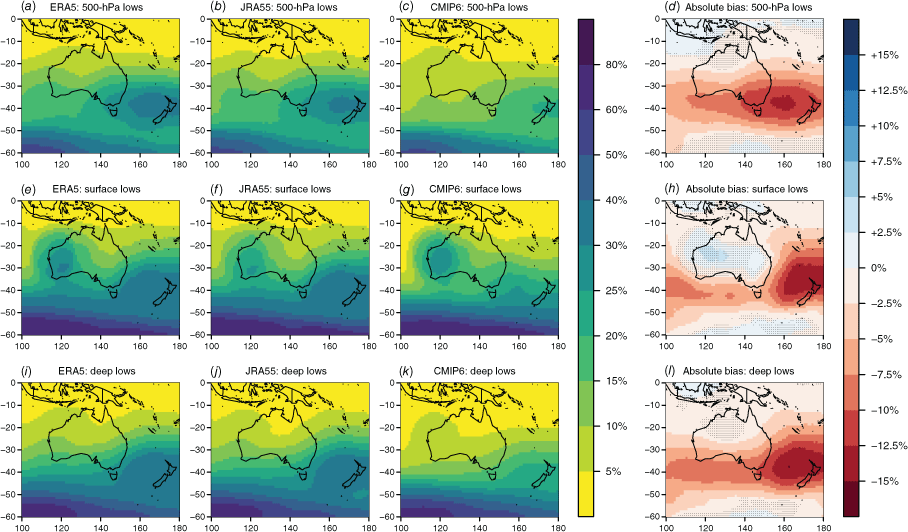
The average frequency of surface lows around southern Australia and the nearby oceans (110–155°E, 33–43°S) is broadly consistent among ERA5, JRA55, and NCEP1 (Fig. 3). Frequencies are within ±8% in both the satellite period 1979–2022 and the pre-satellite period 1959–1978, with interannual correlations between reanalyses of 0.75–0.88. The reanalyses agree well on interannual variability, including the presence of very large interannual variability in the frequency of 500-hPa lows in the early period 1959–1970 and during the most recent period. Notably, 2022 had the highest number of 500-hPa lows on record for both NCEP1 and JRA55, and the second-highest for ERA5, whereas 2019 was the lowest on record for both 500-hPa and deep lows in ERA5 and JRA55, as well as the fewest surface lows on record for all datasets – previously shown by Pepler (2020). The year 2019 was particularly notable for the absence of lows throughout the year; more recently, 2023 was among the three lowest years on record for all low types in May–October.
The percentage of days with a 500-hPa low (left), surface low (centre) or deep low (right) detected during 1959–2023 during May–October (a–c) and November–April (d–f), averaged across southern Australia (33–43°S, 110–155°E). Coloured lines indicate three reanalyses and grey lines the eight CMIP6 models, with black indicating the CMIP6 ensemble mean.
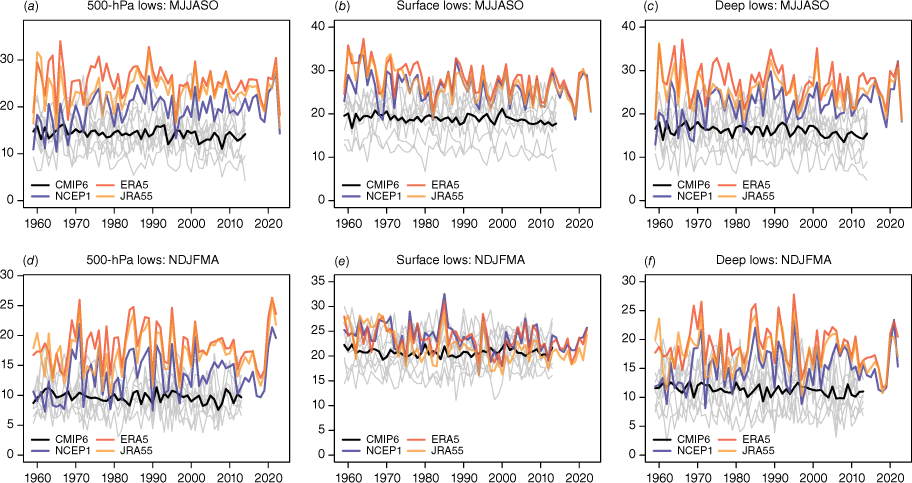
However, for 500-hPa lows there is a strong negative bias in NCEP1, which records on average 23% fewer upper lows than ERA5 during the satellite period and 33% fewer 500-hPa lows in the pre-satellite period, precluding its use for assessing long-term trends in upper lows due to lesser confidence in results. There are also larger differences between ERA5 and JRA55 during the pre-satellite period, with JRA55 identifying 10% fewer upper lows than ERA5 (v. 6% fewer in the satellite period). This is most evident during the cool season (Fig. 3a) and is particularly noticeable in the SWWA subregion (Supplementary Fig S2d), and means that JRA55 tends towards weaker decreasing trends or stronger increasing trends than ERA5. Previously, JRA55 has shown inhomogeneities in its representation of extratropical surface lows in the southern hemisphere, with trends that diverge from other reanalyses (Wang et al. 2016).
The CMIP6 ensemble consistently underestimates the frequency of 500-hPa lows across the southern hemisphere midlatitudes (Fig. 2, Table 1), consistent with results for CMIP5 in Pepler and Dowdy (2021a). Averaged over 33–43°S, 110–155°E, the annual mean bias compared to ERA5 during 1960–2014 ranges from −64% in ACCESS-ESM1-5 to −28% in EC-Earth3. Although all GCMs have higher spatial resolutions but fewer lows than the NCEP reanalysis, there is a negative correlation of −0.89 between GCM spatial resolution and the number of upper level lows, indicating that model resolution may play a role in these biases.
Biases are slightly smaller overall for surface lows, from −47% (ACCESS-ESM1-5) to −10% (MPI-ESM1-2-HR), but remain negatively correlated with GCM resolution (r = −0.79). This is primarily due to the ACCESS models which have the coarsest resolution and largest biases, as correlations between resolution and bias are weak across the other six models. Negative biases for surface lows are primarily present over oceans, with weaker or positive biases over the land; this reflects a tendency of GCMs to generate too many shallow surface lows over land areas but too few deep lows, particularly to the east of Australia (Pepler and Dowdy 2021a). These results are consistent with Priestley et al. (2020), who found that CMIP6 models underestimated the frequency of lows over Australia using a tracking method based on 850-hPa vorticity, although not in the adjacent oceans. This may be related to the stronger criteria on the movement and duration of lows in that paper, which resulted in fewer lows detected in this sector.
4.Trends in frequency
4.1. May–October
Fig. 4 shows spatial maps of the 1959–2023 linear trend in the May–October low frequency. Both ERA5 and JRA55 have declining trends in surface low frequency across parts of Australia (south of 33°S) and the surrounding oceans. This is statistically significant in much of this area, with a regional mean trend of −1.6 days per decade in ERA5 and −1.8 days per decade in JRA55 (P < 0.001), as well as a non-significant decrease in NCEP1 (−0.7 days per decade, Table 2). The trends in ERA5 and JRA55 are negative across almost all time periods, and are statistically significant for 94% of periods using 50 or more years of data (Supplementary Fig. S2). This includes periods ending in 2023, despite above average frequencies of lows during 2020–2022. This indicates a robust declining trend, which could not easily be detected using earlier datasets of less than 40 years given the large interannual variability. Trends are also negative across a range of time periods using the NCEP1 reanalysis, but generally non-significant.
Linear trends (1960–2023) in the frequency of May–October lows within a 10° radius (days per decade) for any 500-hPa low (a–c) or surface low (d–f) centre detected within a 10° radius, or a deep low at either level (g–i). In the ERA5 panels (a, d, g) and the JRA55 panels (b, e, h), dots indicate where trends are non-significant (P < 0.05); the CMIP6 panels (c, f, i) show the mean trend across eight CMIP6 models for the historical simulation (1960–2014), with dots indicating where at least seven models agree on the sign of the change.
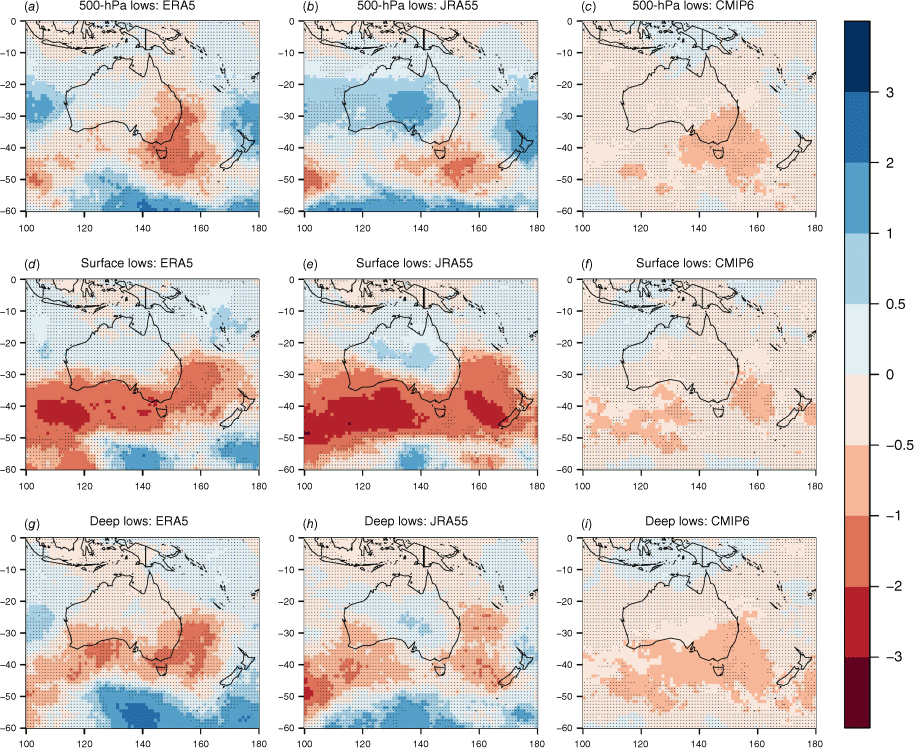
May–October | November–April | ||||||
---|---|---|---|---|---|---|---|
Model | Surface | 500 hPa | Deep | Surface | 500 hPa | Deep | |
ERA5 | −1.62 | −0.57 | −0.84 | −0.78 | 0.21 | −0.35 | |
JRA55 | −1.84 | 0.05 | −0.54 | −1.76 | 0.33 | −0.33 | |
NCEP1 | −0.73 | 1.21 | 1.18 | −0.88 | 1.00 | 0.62 | |
CMIP6 mean | −0.40 | −0.50 | −0.61 | −0.02 | −0.19 | −0.39 | |
ACCESS-CM2 | −0.31 | −0.76 | −0.82 | 0.27 | 0.47 | −0.02 | |
ACCESS-ESM1-5 | −0.95 | −0.85 | −0.99 | 0.11 | −0.38 | −0.06 | |
CMCC-ESM2 | −0.17 | −0.43 | −0.60 | 0.32 | 0.20 | −0.13 | |
CNRM-ESM2-1 | −0.42 | 0.15 | −0.66 | −0.59 | −1.25 | −1.39 | |
EC-Earth3 | −0.84 | −0.92 | −1.00 | 0.61 | −0.25 | −0.07 | |
MPI-ESM1-2-HR | −0.93 | −0.90 | −0.99 | −0.44 | −0.43 | −0.56 | |
MRI-ESM2-0 | −0.27 | −0.93 | −0.76 | −0.14 | 0.24 | −0.43 | |
NorESM2-MM | 0.67 | 0.66 | 0.93 | −0.32 | −0.17 | −0.51 |
The CMIP6 mean row provides the means for the rows below (ACCESS-CM2–NorESM2-MM). Bold indicates trends significant for P < 0.05, and italic for P < 0.1.
The average number of surface lows in 2000–2023 is 8% below the 1959–1999 mean in ERA5 and 9% below the 1959–1999 mean in JRA55, which is statistically significant using a t-test (P < 0.01). By contrast, trends are weak and non-significant over the shorter 1979–2023 period (Supplementary Table S1). Interannual variability is larger when southern Australia is divided into three subregions (Supplementary Fig. S3), but all three subregions have statistically significant negative trends in surface low occurrence in both JRA55 and ERA5 during 1959–2023. Declines in surface low frequency are particularly large during the early part of the season, April–August, with weaker trends in the ERA5 reanalysis during September and October (not shown).
The declining trend in surface lows is linked to a notable decline in cyclogenesis near SWWA in both reanalyses (Fig. 5). During the first part of this period, a large proportion of all lows in southern Australia had cyclogenesis near SWWA before moving eastward across the continent, with a second hotspot of cyclogenesis near the east coast contributing to high cyclone frequencies in the Tasman Sea. However, although there have been only small changes in cyclogenesis near the east coast, both reanalyses find a large and statistically significant decline in cyclogenesis near SWWA. The number of May–October cyclones with genesis in SWWA which cross the southern Australia region decreased from 9.1 per year in 1961–1990 to 5.9 per year in 1991–2023 for the ERA5 reanalysis. The linear trend over 1959–2023 is −1.6% per year in ERA5 and −1.5% per year in JRA55.
(left) Average frequency of surface cyclogenesis (centres per degree per decade) in May–October during 1961–1990 for the ERA5 (a–c) and JRA55 (d–f) reanalysis as well as the CMIP6 ensemble mean (g–i), with 2° of spatial smoothing applied to reduce noisiness. Genesis locations are only shown for lows which are identified in the southern Australian region (marked with a black polygon) for at least one instance. (centre) Average cyclogenesis frequency during 1991–2023 (or 1991–2014) for the CMIP6 ensemble. Differences between the frequency in the earlier and later periods (c, f, i), with red colours indicating a decrease in cyclogenesis. In panels (c) and (f), dots indicate changes that are statistically significant for P < 0.05 using a t-test. In panel (i), dots indicate where at least 20% of ensemble members agree on the sign of the change.
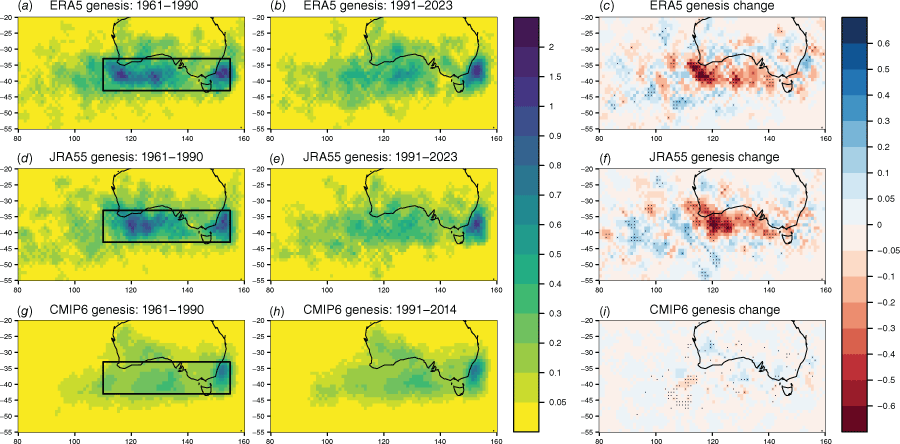
Statistically significant decreases in cyclogenesis are also seen in the GAB region, of −0.9% per year in JRA55 and −0.8% per year in ERA5. The overall decrease in cyclone frequency in southern Australia can be completely explained by the decrease in cyclogenesis in these two regions. Although these changes in cyclogenesis could be partially linked to poorer representation of weak surface lows over the Indian and Southern Oceans during the pre-satellite period, there is no significant increase in the frequency of lows in southern Australia from cyclogenesis outside the region. Changes in cyclogenesis in the ECL region are smaller, with non-significant declines between 1961–1990 and 1991–2023 of 5–10%.
Trends in 500-hPa lows are not significant for southern Australia over 1959–2023, with a weakly negative trend in ERA5 (−0.6 days per decade) including a significant trend in the ECL subregion (−1.1 days per decade) but no trend in JRA55. This results in weaker declining trends in deep low days of −0.8 days per decade in ERA5 (P = 0.1) and −0.5 days per decade in JRA55 (P = 0.24). In contrast to surface lows, trends in 500-hPa lows are generally stronger over the 1979–2023 period (Supplementary Table S1, Fig. S2), with larger uncertainty between datasets over longer periods. Cyclogenesis at 500-hPa declines broadly across the southern Australian region, with different spatial patterns in ERA5 v. JRA55 (Supplementary Fig. S4). In contrast to surface lows, trends in upper lows in ERA5 are most notable towards the end of the cool season, between July and October.
Significant declining trends in surface lows, in addition to non-significant declining trends in 500-hPa and deep lows, are evident in ERA5 for a wide range of intensity thresholds (Supplementary Table S2). Near-zero trends are found for the most intense lows; however, this may be an artefact of lower skill at representing the intensity of strong lows during the pre-satellite era rather than a true climate signal (Wang et al. 2016). This shift is more notable for JRA55, which has a large drop in the frequency of strong upper lows prior to 1985, resulting in large increasing trends in strong upper lows over the period 1959–2023. The CMIP6 ensemble has declines in both surface and upper lows for all intensity thresholds (not shown).
The ERA5 trend in 500-hPa lows is consistent with the CMIP6 ensemble, which has declining trends in six models and an ensemble-mean decline of −0.5 days per decade over 1959–2014. In both the models and reanalyses, the strongest trends are in south-east Australia and the adjacent oceans (Fig. 4). In the region 140–155°E, 33–43°S the ensemble mean decline is −0.8 days per decade and four models have staistically significant declines of more than −1.2 days per decade, although NorESM2-MM has a strong increasing trend of +0.9 days per decade. Additionally, ERA5 has strong declining trends in this region for both 500-hPa lows (−1.2 days per decade, P = 0.06) and deep lows (−1.0 days per decade, P = 0.13), although trends are weaker in JRA55.
By contrast, whereas the spatial pattern of trends in surface lows in the CMIP6 models is consistent with observations, the magnitude of the modelled trend is much weaker than in observations. Although seven of the eight models have a declining trend in surface lows in southern Australia, the ensemble mean trend is just −0.4 days per decade, with no models producing trends comparable in magnitude to the observed trend. Whereas weaker absolute trends might be expected given biases in mean cyclone frequency (e.g. Fig. 2h), only ACCESS-ESM1.5 has relative trends comparable to observations, with a 10% decline in frequency between 1960–1990 and 1991–2014 and declines in all three subregions. The CMIP6 ensemble mean consequently has a −0.6 days per decade decline in deep lows, which is similar in magnitude to JRA55 and weaker than the trend in ERA5.
4.2. Drivers of cool season trends
The strength of the historical trends in cool season lows, particularly at the surface level, alongside the weaker but spatially consistent trends across the historical CMIP6 ensemble, raises questions as to the relative role of natural variability and anthropogenic forcing in the observed changes.
To investigate the role of natural variability, we first assessed detrended correlations between cyclone frequency and the three largest sources of interannual variability in this region: ENSO, IOD and SAM. Surprisingly, there are no significant correlations with the seasonal SAM at either level, with weakly positive correlations during August–October at 500 hPa (+0.35) but no significant correlations for surface lows or during the early season, where trends are largest. Correlations with the Southern Oscillation Index (SOI), an indicator of ENSO, are positive near the surface (+0.45) but weak at upper levels, but this index has no significant trends over the period of interest. There are stronger correlations for the Dipole Mode Index (DMI), an indicator of IOD, of −0.57 at the surface and −0.39 at 500 hPa. This results in a strong correlation of −0.48 with deep lows, but the DMI also shows no significant trend over the available dataset (beginning in 1982).
In the ERA5 reanalysis, detrended interannual variability in surface lows is negatively correlated (Supplementary Fig. S5) with mean 700-hPa zonal wind speeds to the south of Australia (r = −0.64 for 110–155°E, 40–50°S), with similar correlations also with the frequency of 500-hPa lows (r = −0.62). Surface lows are also positively correlated with wind speeds in the subtropics (r = +0.40 for 110–155°E, 20–30°S), and negatively correlated with 700-hPa geopotential height over Australia. This appears as an intensification of the eddy-driven jet during years with fewer low pressure systems, but weaker westerlies in the subtropics, conditions previously linked to a decrease in rainfall from cold fronts (Pepler and Rudeva 2023). The period 1959–2023 has seen an intensification of the westerlies near Tasmania and an increase in 700-hPa geopotential height over Australia; however, the magnitude of observed trends in mean climate variables explains less than 20% of the observed trend in lows based on linear regressions, and trends in the mean state may themselves be partially due to changes in weather systems.
The ACCESS-ESM1.5 model ensemble can be used to identify potential links between simulated trends in extratropical lows and changes in mean state variables. This model has 10 historical simulations with 6-hourly SLP data, of which the ensemble member used in this paper (r4i1p1f1) is the closest in magnitude to the observed trend of any CMIP6 model tested (Table 2). This member also has the strongest trends of any member of the ACCESS-ESM1.5 ensemble, with a decrease in the number of surface lows of −1.1% per year during 1960–2014. Four additional ensemble members have strong negative trends of at least −0.5% per year, but only one member has a weakly increasing trend of +0.1% per year.
Six-hourly SLP data are also available from ACCESS-ESM1.5 for a small number of DAMIP experiments, including three hist-GHG and three hist-nat simulations. Both ensembles have a wide range of trends, between −0.3 and +0.4% per year in hist-nat and between −0.7 and +0.01% per year in hist-GHG. These variations between ensemble members are unsurprising given the large interannual variability in cyclone counts (Fig. 3), and offer the potential to identify links between the magnitude of modelled trends in lows and other relevant climate variables.
Across the 10-member historical ensemble, there is no strong relationship between trends in the number of lows and trends in the zonally symmetric SAM (not shown), and only weak relationships between trends in lows and trends in baroclinicity over the same region, as measured by the Phillips Criterion (Supplementary Fig. S6). However, ensemble members with stronger decreases in the frequency of lows tend to have stronger increases in both mean temperature and geopotential height at 700 hPa near SWWA (Supplementary Fig. S6), which is not identified from ERA5. These models also tend to have stronger increases in the strength of westerly zonal winds near Tasmania, although the region is slightly offset from the latitudes with strongest correlations in the ERA5 reanalysis.
Trends in 700-hPa winds and temperature near SWWA are both strongly correlated with the magnitude of trends in southern Australian lows across the ACCESS-ESM1.5 ensemble, but also consistently stronger in the historical and hist-GHG ensembles than hist-nat (Fig. 6a, b), indicating a potential anthropogenic forcing (Fig. 6). Compared to the model ensemble, trends in climate variables near SWWA in ERA5 are consistent in sign and larger in magnitude than in any ACCESS-ESM1.5 ensemble member, and the relationship between observed trends in lows and SWWA climate variables are consistent with that seen across the ACCESS-ESM1.5 ensemble. By contrast, trends in zonal winds near Tasmania in the ERA5 reanalysis are within the middle of the ensemble spread (Fig. 6), and are less useful for explaining observed trends.
Trend in the number of low centres identified in southern Australia during May–October (x-axis, counts per decade) during 1960–2023 for the ERA5 reanalysis and 1960–2014 for a 16-member ACCESS-ESM1.5 ensemble, compared to seasonal mean climate variables (y-axis): (a) mean 700-hPa geopotential height averaged over 100–120°E, 35–45°S (metres per decade); (b) mean 700-hPa temperature averaged over 100–120°E, 35–45°S (Kelvin per century); and (c) mean 700-hPa zonal wind averaged over 140–160°E, 35–45°S (metres per second per century).
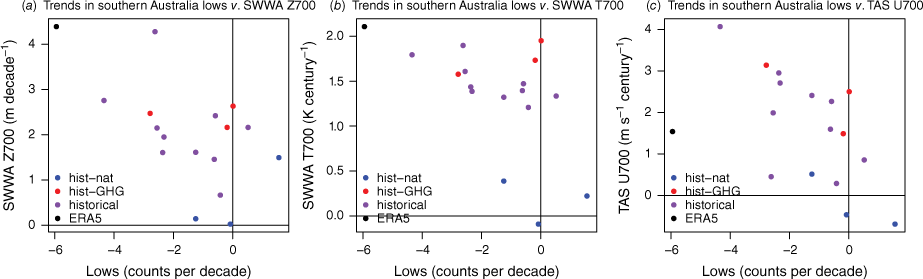
4.3. November–April
All three reanalyses also show statistically significant declines in the frequency of surface lows in November–April over southern Australia between 1959–1960 and 2022–2023 (Table 2), with strongest trends in the south-east (Fig. 7d, e, Supplementary Fig. S7). The average decline is −0.9 days per decade in ERA5 (P = 0.05), with much larger declines in JRA55 (−2.1 days per decade). These observed declines extend further north than trends expected from the CMIP6 ensemble (Fig. 7), which has no robust change in surface lows over southern Australia, but large and robust declines over higher latitudes (~45–55°S). Interestingly, both the CMIP6 ensemble and the reanalyses also show an increasing trend in surface lows over parts of Western Australia during the warm months, which likely reflects an increase in heat lows. Trends are weaker and less significant for surface lows identified using stronger intensity thresholds (Supplementary Table S3).
Linear trends (1960–1961 to 2022–2023) in the frequency of November–April lows within a 10° radius (days per decade) for any 500-hPa low (a–c) or surface low (d–f) centre detected within a 10° radius, or a deep low at either level (g–i). In the ERA5 panels (a, d, g) and JRA55 panels (b, e, h), dots indicate where trends are non-significant (P > 0.05); the CMIP6 panels (c, f, i) show the mean trend across eight CMIP6 models for the historical simulation (1960–2013), with dots indicating where at least seven models agree on the sign of the change.
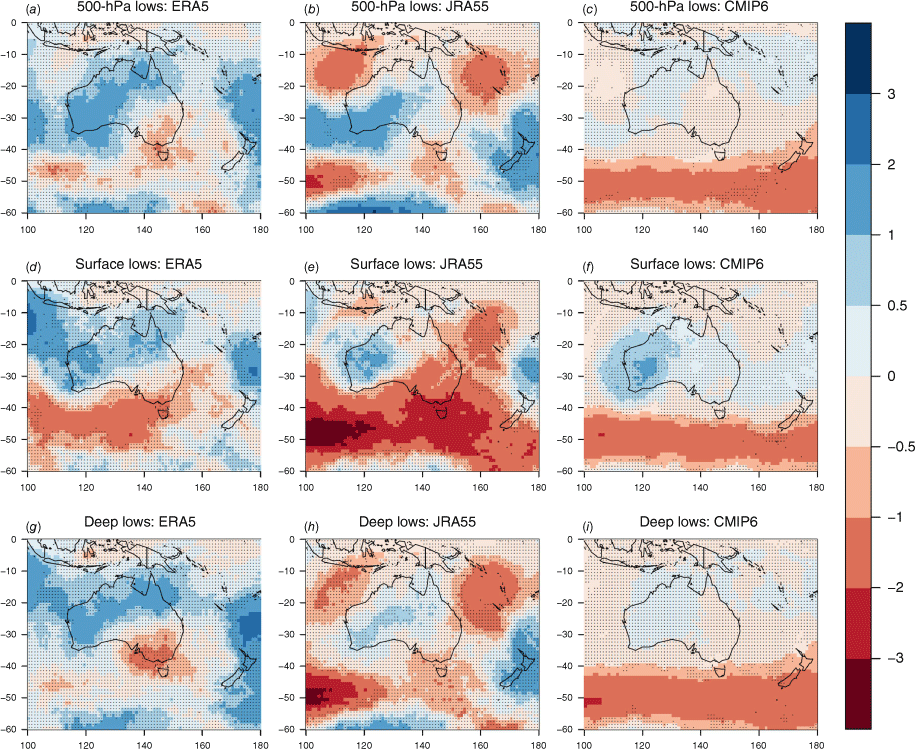
Trends in 500-hPa lows over southern Australia are not statistically significant for any intensity threshold (Supplementary Table S3). Following near-record low frequencies in 2017–18, 500-hPa lows were the highest on record in ERA5 during 2021–22 (Fig. 3), contributing to very high rainfall totals during that year (Barnes et al. 2023). Despite this, both ERA5 and JRA55 have a small but non-significant decline in the frequency of deep lows during November–April, which is close to −1 day per decade near south-east Australia and Tasmania (Fig. 7). Interestingly, trends in 500-hPa lows also differ between ERA5 and JRA55 over the 1979–2022 period, as JRA55 has markedly fewer 500-hPa lows than ERA5 during the early 1980s (Fig. 3d).
In northern Australia there are statistically significant increasing trends in the frequency of surface and upper lows in ERA5 but decreasing trends in JRA55, particularly over the ocean to the north-east of Australia. This is due to a divergence between the two reanalyses in recent decades, with ERA5 recording an increased frequency of lows since 2000 but JRA55 recording a decrease. Consequently, ERA5 has 17% more lows in the region 120–150°E, 10–20°S than JRA55 in 1959–1999, but 57% more lows in 2000–2021, including more than twice as many lows in 2004–2005, 2007–2008, and 2016–2017. These diverging results give low confidence around trends in this region, particularly as the tracking method used for this paper was designed for extratropical lows and may not necessarily detect all tropical cyclones. There are known issues for ERA5 in simulating tropical cyclones (Bell et al. 2021), which may contribute to errors in this region, noting that the frequency of tropical cyclones has been declining in the Australian region (Chand et al. 2019, 2022).
5Trends in rainfall
As surface and upper lows commonly interact in the generation of rainfall, rather than showing trends separately for surface and 500-hPa lows, we instead show rainfall associated with deep lows, shallow surface lows, and shallow upper lows, consistent with Pepler and Dowdy (2022), with a focus on results using ERA5 (Fig. 8). Deep cyclones are responsible for 45% of total rainfall and 58% of days above the 99th percentile when averaged across southern Australia and have the heaviest mean rainfall intensities, whereas shallow surface (10%) and shallow upper (3%) make smaller contributions to rainfall (Supplementary Fig. S1).
Linear trend in total rainfall in May–October (a–e) and November–April (f–j) over 1959–2023, in millimetres per decade. Also shown are linear trends in the total seasonal rainfall accumulated across days with no low, a deep low, a shallow surface low or a shallow upper low in ERA5.
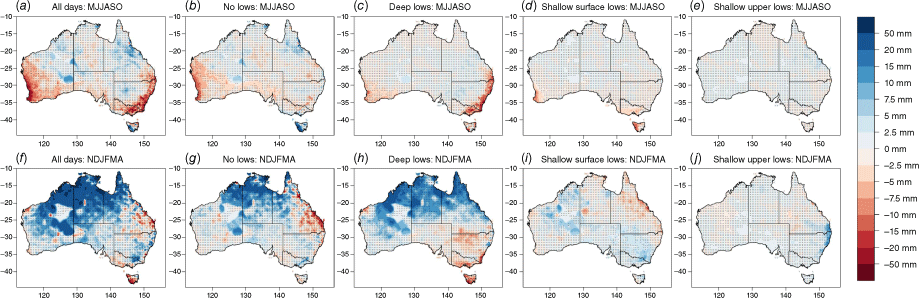
Rainfall is spatially heterogeneous and exhibits large interannual variability, so linear trends are typically not statistically significant at the gridpoint level, with the exception of parts of SWWA during the cool months and the northern tropics during the warm months. In parts of southern Australia with declining rainfall trends during the cool season, these are predominantly associated with deep lows in ERA5. Equivalent plots for JRA55 are shown in Supplementary Fig. S8; this reanalysis also attributed rainfall trends primarily to rainfall from lows, but with a larger proportion due to shallow surface lows.
Warm season rainfall increases are linked to a combination of increasing rain from deep lows in parts of the north and west, as well as rain on days when no lows are detected. This increase in rainfall on days with no low pressure system are consistent with observed increases in rainfall from thunderstorms (Dowdy 2020) and a shift towards rainfall events of shorter duration (Dey et al. 2020). The increasing trend in rainfall from deep lows in northern Australia may relate to changes in monsoon depressions (Lavender and Abbs 2013; Hurley and Boos 2015), as tropical cyclone frequency is decreasing over Australia (Chand et al. 2019). However, it is important to note that ERA5 shows stronger increasing trends in deep cyclones than JRA55 in this region (Fig. 4), so there is less confidence in this change than for southern Australia. There is also an indication of an increase in warm season rainfall linked to shallow upper lows in part of the east coast, which is an area that was strongly influenced by upper lows during recent record-breaking rain and flooding (Barnes et al. 2023).
To better assess changes in low-related rainfall, we accumulate rainfall across land areas south of 33°S (southern Australia), as well as four regions used for rainfall projections in Pepler and Dowdy (2022): SWWA, Tasmania, the Eastern Seaboard (ESB), and inland south-east Australia (SEA) (Fig. 1). Total rainfall in these regions has large interannual variability, particularly in recent years: 2022 was the wettest year on record for the ESB, and the second-wettest year for southern Australia overall; by contrast, 2019 was the driest year on record for the ESB and well below average in southern Australia. These result in very different apparent trends in rainfall depending on the years used, so linear trends are calculated across a range of time periods to assess the robustness of inferred changes.
For southern Australia as a whole, cool season rainfall has a non-significant decline of −0.6 mm per decade during 1959–2023 (P = 0.20, Fig. 9a). Declines are most robust in SWWA (−1.1 mm per year, P = 0.002) and on the ESB (−1.2 mm per year, P = 0.06), but are not significant in SEA (−0.44 mm per decade, P = 0.36). Although average rainfall in SEA during 2000–2023 was 11% lower than during 1959–1999 period (P = 0.11), the two wettest May–October periods on record for SEA were in 2022 and 2016. Trends are particularly weak when calculated over periods ending in these wet years, and are stronger when calculated over periods beginning in the wetter 1970s than during the 1960s. This highlights the influence of interannual variability on the detection of trends.
Linear trend (mm per year) in total seasonal rainfall in southern Australia (a, b) during MJJASO (a, c, e, g, i) and NDJFMA (b, d, f, h, j), as well as the trend in rainfall linked to three categories of low (e–j) and days with no lows (c, d) using the ERA5 reanalysis. Trends are shown for a range of periods between 1959 and 2022 of 30 years or longer, with the y-axis denoting the first year used for each trend calculation and the x-axis denoting the final year. Solid circles indicate trends that are statistically significant at P < 0.05 and crosses at P < 0.1. The longest period, 1959–2023, is shown in the bottom right corner.
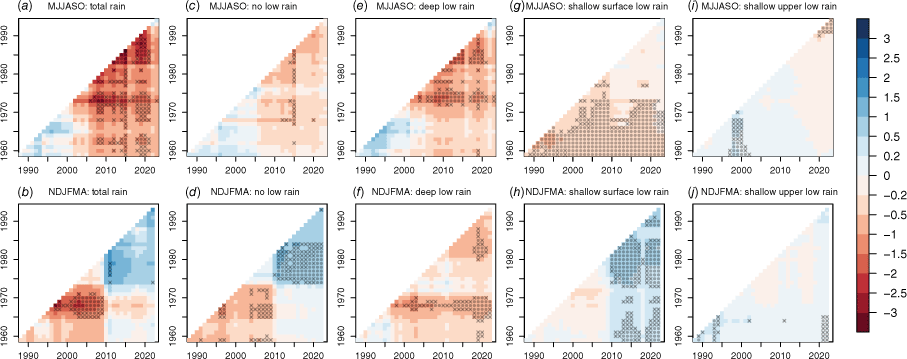
When rainfall is separated into the components from different weather systems, 60% of the trend in southern Australian rainfall is attributed to deep lows using ERA5, with an additional 36% attributed to shallow surface lows (Fig. 9e, g), suggesting most of the change in rainfall can be linked to lows. This is consistent with Pepler et al. (2021), who found that most of the change in south-east Australian rainfall between 1979–1996 and 1997–2015 could be linked to lows and associated fronts. However, although JRA55 has a similar decline in rainfall from shallow lows, it does not find a trend in rainfall from deep lows, leading to a corresponding larger decline in rainfall from days with no associated low (Supplementary Fig. S8). During the warm half of the year, there is a trend towards increasing rainfall from shallow surface lows in ERA5 (Fig. 9h), but not in JRA55 (Supplementary Fig. S9), which has a much larger change in surface low frequency during the warm months (Fig. 7e).
Rainfall in south-east Australia is strongly influenced by climate variability, with correlations of 0.57 between SOI and total rainfall in May–October and 0.43 in November–April, so long-term trends are sensitive to the period used. Over periods beginning in the 1970s, including the satellite period from 1979, there are predominantly declining trends in rainfall associated with deep lows during the cool season (Fig. 10e), including a linear trend of −1 mm per year over 1979–2019 (P = 0.06). Trends are stronger and more significant when calculated over periods ending in dry years such as 2015 and 2019, and not significant for periods ending in wet years such as 2016 and 2022. However, the 1970s were a notably wet decade across southern Australia and, although declining rainfall from lows is the largest component of rainfall trends for 1959–2023 (−0.3 mm per year, 67% of total decline), it is not statistically significant. This subregion also has a small decreasing trend in rainfall from shallow surface lows during the cool half of the year, and an increasing trend in rainfall from surface lows during the warm half of the year in ERA5 (Supplementary Fig. S10g, h).
Linear trend (mm per year) in average seasonal rainfall associated with deep lows in MJJASO (a, c, e, g, i) and NDJFMA (b, d, f, h, j) for five subregions of Australia: southern Australia (SAust) (a, b), ESB (c, d), SEA (e, f), TAS (g, h) and SWWA (i, j). Trends are shown for a range of periods between 1959 and 2022 of 30 years or longer, with solid circles indicating P < 0.05 and crosses P < 0.1.
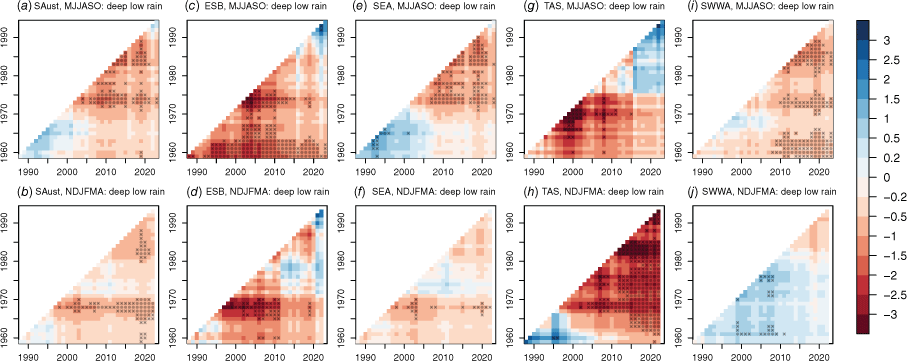
The relationship between rainfall and ENSO is weaker on the ESB, with a correlation of 0.36 between deep cyclone rainfall and SOI in May–October, which is weaker for shallow cyclones. Declining May–October rainfall on the ESB is primarily due to declining rainfall from deep low pressure systems, which explain 89% of the total rainfall trend in ERA5 (P = 0.02) and 72% of the declining trend in JRA55. The declining trend in rainfall from deep lows in this region is robust to a large range of periods (Fig. 10c), particularly over longer periods beginning in the 1960s. The ESB had near-record high rainfall from deep lows in 2022, 64% above the historical average and behind only 1974 and 1967 in magnitude, leading to non-significant rainfall trends over the 1959–2022 period. However, that was followed by record low rainfall from deep lows in 2023, just 31% of the historical average, with statistically significant trends when calculated over 1959–2023.
There are no strong trends in warm season rainfall on the ESB, with record-high totals in 2021–22 linked to very much above average rainfall from both deep lows and shallow upper lows. There is an indication of an increasing trend in rainfall from shallow upper lows (Supplementary Fig. S11), with a 0.5 mm per year increase over 1959–2022 (P = 0.09). Rainfall from non-cyclone days during the warm season increases during La Niña years (r = 0.52), but correlations are weaker for cyclone rainfall (r = 0.23–0.29).
There is a statistically significant decline in cool season rainfall for SWWA of −1.1 mm per year over 1959–2023 (P < 0.01). This trend is associated with a range of weather systems (Supplementary Fig. S12) including deep lows (−0.4 mm per year, P = 0.051), shallow surface lows (−0.2 mm per year, P = 0.005), and days with no low (−0.5 mm per year, P = 0.055), which is most likely rain from cold fronts (Hope et al. 2006; Risbey et al. 2013b; Pepler and Rudeva 2023). The proportion of the trend arising from days with no low is higher in JRA55 (−0.8 mm per year). During the warm half of the year, trends in rainfall in SWWA are weakly positive but non-significant.
The reverse is true of Tasmania, which has declining rainfall from deep lows during the warm season over a large range of time periods, including a −1.6 mm per year change over 1965–2022 (P = 0.02), with similar declines in JRA55 (−1.3 mm per year, P = 0.06). However, trends are weaker over the full period beginning in 1959 (−1.0 mm per year, P = 0.11), as low-related rainfall was below average in the years 1961–1963. There is a weaker and non-significant decreasing trend in rainfall from deep lows during the cool season, along with a significant and robust decreasing trend in rainfall from shallow surface lows (Supplementary Fig. S13g), which is weaker in JRA55. However, this is balanced by an increasing trend in rainfall on days with no lows (Supplementary Fig. S13c) by 1.4 mm per decade over 1959–2023 (P = 0.02), particularly on the exposed west coast (Fig. 8b). This is consistent with projected future increases in non-cyclone rain in western Tasmania from Pepler and Dowdy (2022).
Along with decreasing trends in total rainfall, all regions have a declining trend in the number of days with more than 1 mm of rain over 1959–2023. Consistent with trends in total rainfall, this trend is strongest in the warm season for Tasmania (−1.1 days per decade, P = 0.02), whereas all other regions are dominated by statistically significant declines of at least 1 day per decade in the cool season. For southern Australia as a whole, 64% of the annual decline in rain days is due to declining rain days from deep cyclones using ERA5 (P = 0.02), with smaller contributions from days with shallow surface lows or no cyclone.
By contrast, no regions have statistically significant changes in the frequency of heavy rain days greater than or equal to the 99th percentile, although there are small increases in the numbers of heavy rain days linked to shallow surface lows in SEA (+0.6 days per century, P = 0.059) and shallow upper lows in most regions (+0.2 days per century for southern Australia, P = 0.006), predominantly during the warm half of the year. The increase in heavy rainfall from shallow upper lows in southern Australia is also seen over other periods such as 1959–2019 (+0.16 days per century, P = 0.04), so is not solely due to the very high frequencies of upper lows in 2022. Although this trend is significant, it is relatively unimportant, reflecting a change from shallow upper lows explaining 2% of all southern Australian heavy rain days in 1960–1989 to 4% in 1990–2019, and an increase from 7.1 to 10.9% of heavy rain days on the ESB.
6Discussion and conclusions
The frequency of both surface and 500-hPa lows has large interannual variability, which is particularly notable in recent years. The years 2017–2019 were a very dry period in southern Australia, with a record lack of both surface and upper lows in 2019, as previously noted in Pepler (2020). This was followed by three wet years, including record-high numbers of 500-hPa lows in 2022, contributing to the wettest year since the beginning of this dataset on the ESB. By contrast, 2023 experienced a lack of low pressure systems and associated rainfall during the cool season, particularly on the ESB. This large interannual variability, partly linked to ENSO and IOD, makes the detection of trends over periods less than 50 years challenging (e.g. Pepler et al. 2015, Pepler and Dowdy 2021b), and may result in artificially inflated trend strengths over periods ending in dry years.
The recent extension of the ERA5 reanalysis to 1959 supplements the earlier JRA55 reanalysis to allow assessment of trends over more than 60 years. Using these data, we find a statistically significant decline in the frequency of cool season surface lows over southern Australia between 1959 and 2023, which is significant for nearly all cool season periods of at least 50 years. Declines in surface lows are also detected over parts of south-east Australia during the warm months November–April, particularly near Tasmania and in the JRA-55 reanalysis, with areas of increasing frequency over parts of Western Australia. Trends in both reanalyses are stronger and more significant when using the 1959–2023 period than in shorter periods starting in 1979, due to high frequencies of surface lows in southern Australia during the 1960s. This is consistent with previous studies using the Twentieth Century Reanalysis, which found higher frequencies of lows in south-east Australia during the 1960s and 1970s than the early 21st century using a range of methods (Pepler et al. 2017; Ji et al. 2018). Trends are weaker using the NCEP reanalysis, which has diverging (lower) cyclone frequencies in the early data, particularly at upper levels; although this decreases confidence in the detected trends, it may indicate inhomogeneities which make this dataset less suitable for assessing long-term changes near Australia.
The observed decline in surface cyclone frequency near southern Australia is linked to a decline in cyclogenesis near SWWA. Using the ACCESS-ESM1.5 historical model ensemble, members with strong trends in cyclone frequency are also identified to have larger mean state changes near SWWA, including increases in 700-hPa geopotential height and air temperature, with strong trends also identified in reanalysis data. The SWWA subregion is an area of rapid climate change, including very large decreases in rainfall during the cool half of the year; whereas climate models give high confidence that changes in this region are partially anthropogenically forced, models underestimate the magnitude of the historical changes (Rauniyar et al. 2023). Although targeted model experiments are needed to understand the detailed dynamics, these results suggest that changes in the mean state near SWWA may be affecting cyclone frequency across the whole of southern Australia.
The frequency of both surface and upper cyclones in southern Australia are related to the strength of 700-hPa zonal winds in the midlatitudes in reanalyses and model simulations, suggesting a possible role of a strengthening eddy-driven jet in recent trends. Changes in Australian-region westerly winds, including weakening of the subtropical westerlies and strengthening in the midlatitudes, has also been identified previously as a possible mechanism behind decreases in frontal rainfall in southern Australia (Pepler and Rudeva 2023). However, the ACCESS-ESM1.5 ensemble mean change in this region is similar to the observed trends, so this cannot explain the underestimation of observed declines in cyclone frequency by climate models.
Lows at 500 hPa are less consistent between the ERA5 and JRA55 reanalyses. Although both reanalyses have decreases during May–October in parts of south-east Australia and Tasmania, these are stronger and cover a larger area in ERA5, whereas increasing frequencies are simulated over parts of central Australia in JRA55. This means that only ERA5 has a decreasing trend for southern Australia over 1959–2023, with stronger declines in both reanalyses over 1979–2023. Differences are also evident in the warm season, particularly over tropical ocean regions where the signs of trends differ between reanalyses. Lower consistency between reanalyses, particularly at upper levels, is to be expected during earlier decades when reanalyses are poorly constrained by limited upper air data. However, poorly constrained reanalyses are more likely to underestimate the true frequency of strong low pressure systems in earlier periods (Wang et al. 2016; Pepler et al. 2017); this would have the effect of weakening or removing negative trends, such that the observed declines are more likely to be underestimated than overestimated. This effect is more noticeable when trends are calculated over lows of higher intensity, with opposing trends in ERA5 and JRA55 in some cases.
During the cool half of the year, the observed decline in 500-hPa lows, particularly from ERA5, is consistent with both the spatial pattern and magnitude of trends in the CMIP6 model ensemble. The consistency between historical trends, model simulations, and projected changes suggest that this could be an emerging signal of anthropogenic change, noting that upper level lows are projected to decline in frequency across the southern hemisphere midlatitudes in a warming climate (Pepler et al. 2021a).
The spatial pattern of observed cool season trends in surface lows is also well simulated by the CMIP6 ensemble, and consistent with projected future changes for this region (Grieger et al. 2014; Pepler and Dowdy 2021a, 2022; Priestley and Catto 2022). However, the magnitude of trends is underestimated, with trends also underestimated across the ACCESS-ESM1.5 model ensemble. These trends cannot be explained by changes in climate variability, and are not present in ‘hist-nat’ simulations without anthropogenic forcing, indicating a likely anthropogenic role in observed changes. Although some possible mechanisms have been identified, further research and targeted model simulations are needed to understand the drivers of these trends, including whether climate models are underestimating the magnitude of the forced signal.
Trends in rainfall associated with lows are more sensitive to time period and reanalysis dataset than low frequency, and are more strongly influenced by drivers of interannual variability such as ENSO. This may also be more affected by other aspects of a warming climate including increasing moisture availability for extreme events (Pepler and Dowdy 2022; Wasko et al. 2024). Consistent with declines in low pressure systems, the total rainfall from deep lows is generally declining over Australia south of 33°S during the cool half of the year. This is the main driver of declines in total rainfall, which has also been shown for future projections of 21st century rainfall (Pepler and Dowdy 2022). During very wet years, such as 2016 and 2022, these trends are non-significant. However there is a strong declining trend in rainfall from deep lows on the ESB over 1959–2023 which could not be detected over the shorter 1979–2020 period in Pepler and Dowdy (2021b), highlighting the value of extended historical reanalyses. By contrast, previously identified declining trends in cyclone rainfall during the satellite era in south-eastern Australia (Pepler et al. 2021) may have been overestimated and are less apparent using a longer period.
This paper presents a new dataset of low pressure systems between 1959 and 2023. Over this period, there are robust declining trends in extratropical lows near southern Australia during the cool season, particularly near the surface, which are likely contributing to long-term declines in cool season rainfall. However, the drivers of these long-term trends remain unclear, including the relative contributions of natural variability and anthropogenic forcings. Although there is little relationship between ENSO, IOD or SAM with either observed or simulated trends, underestimation of observed trends in GCMs as well as a lack of subdaily data from DAMIP experiments make attributing observed trends to anthropogenic climate change more challenging than for other variables such as temperature. Given the importance of low pressure systems for rainfall in southern Australia, particularly during the cool months of the year, there is a need to better understand the drivers of observed trends, including by better identifying the relationship between changes in low pressure frequency and other fingerprints of global climate change such as changing large-scale mean circulation patterns, meridional temperature gradients or land–sea contrast. Analysis of these factors including targeted experiments to understand the mechanisms behind the observed trends and the relative role of anthropogenic climate change will be critical to understanding future climate in this region.
Data availability
This paper presents data for surface and 500-hPa lows over the Australian region, with a focus on the ERA5 reanalysis. However, the full global dataset, including high pressure systems, for the ERA5, JRA55 and NCEP1 reanalyses are available for research use at https://doi.org/10.6084/m9.figshare.24086898.v1. The CMIP6 tracks are available upon contacting the author.
Declaration of funding
This work was supported by the Climate Systems Hub of the Australian National Environmental Science Program, with computing resources from the National Computational Infrastructure.
Acknowledgements
The author acknowledges the helpful comments and advice from Irina Rudeva, Andrew Dowdy, and three anonymous reviewers, who improved this manuscript.
References
Abram NJ, Wright NM, Ellis B, Dixon BC, Wurtzel JB, England MH, et al. (2020) Coupling of Indo-Pacific climate variability over the last millennium. Nature 579(7799), 385-392.
| Crossref | Google Scholar | PubMed |
Abram NJ, Henley BJ, Sen Gupta A, Lippmann TJR, Clarke H, Dowdy AJ, et al. (2021) Connections of climate change and variability to large and extreme forest fires in southeast Australia. Communications Earth & Environment 2(1), 8.
| Crossref | Google Scholar |
Barnes MA, King M, Reeder M, Jakob C (2023) The dynamics of slow‐moving coherent cyclonic potential vorticity anomalies and their links to heavy rainfall over the eastern seaboard of Australia. Quarterly Journal of the Royal Meteorological Society 149, 2233-2251.
| Crossref | Google Scholar |
Bell B, Hersbach H, Simmons A, Berrisford P, Dahlgren P, Horányi A, et al. (2021) The ERA5 global reanalysis: preliminary extension to 1950. Quarterly Journal of the Royal Meteorological Society 147(741), 4186-4227.
| Crossref | Google Scholar |
Bentsen M, Oliviè DJL, Seland Ø, Toniazzo T, et al. (2019) NCC NorESM2-MM model output prepared for CMIP6 CMIP historical. Version 20190514. (Earth System Grid Federation) [Dataset] doi:10.22033/ESGF/CMIP6.8040
Booth JF, Naud CM, Jeyaratnam J (2018) Extratropical cyclone precipitation life cycles: a satellite-based analysis. Geophysical Research Letters 2009, 8647-8654.
| Crossref | Google Scholar |
Browning SA, Goodwin ID (2013) Large-scale influences on the evolution of winter subtropical maritime cyclones affecting Australia’s east coast. Monthly Weather Review 141(7), 2416-2431.
| Crossref | Google Scholar |
Catto JL (2016) Extratropical cyclone classification and its use in climate studies. Reviews of Geophysics 54(2), 486-520.
| Crossref | Google Scholar |
Cavicchia L, Pepler A, Dowdy A, Walsh K (2019) A physically based climatology of the occurrence and intensification of Australian east coast lows. Journal of Climate 32(10), 2823-2841.
| Crossref | Google Scholar |
Chand SS, Dowdy AJ, Ramsay HA, Walsh KJE, Tory KJ, Power SB, et al. (2019) Review of tropical cyclones in the Australian region: Climatology, variability, predictability, and trends. Wiley Interdisciplinary Reviews: Climate Change 10(5), 1-17.
| Crossref | Google Scholar |
Chand SS, Walsh KJE, Camargo SJ, Kossin JP, Tory KJ, Wehner MF, et al. (2022) Declining tropical cyclone frequency under global warming. Nature Climate Change 12(7), 655-661.
| Crossref | Google Scholar |
CSIRO, Bureau of Meteorology (2015) Climate change in Australia: projections for Australia’s NRM regions. Technical report. (CSIRO and Bureau of Meteorology) Available at https://www.climatechangeinaustralia.gov.au/media/ccia/2.2/cms_page_media/168/CCIA_2015_NRM_TechnicalReport_WEB.pdf
Dey R, Lewis SC, Arblaster JM, Abram NJ (2019) A review of past and projected changes in Australia’s rainfall. Wiley Interdisciplinary Reviews: Climate Change 10(3), e577.
| Crossref | Google Scholar |
Dey R, Gallant AJE, Lewis SC (2020) Evidence of a continent-wide shift of episodic rainfall in Australia. Weather and Climate Extremes 29, 100274.
| Crossref | Google Scholar |
Dix M, Bi D, Dobrohotoff P, Fiedler R, et al. (2019) CSIRO-ARCCSS ACCESS-CM2 model output prepared for CMIP6 CMIP historical. Version 20210607. (Earth System Grid Federation) [Dataset] doi:10.22033/ESGF/CMIP6.4271
Dowdy AJ (2020) Climatology of thunderstorms, convective rainfall and dry lightning environments in Australia. Climate Dynamics 54(5), 3041-3052.
| Crossref | Google Scholar |
Dowdy AJ, Catto JL (2017) Extreme weather caused by concurrent cyclone, front and thunderstorm occurrences. Scientific Reports 7, srep40359.
| Crossref | Google Scholar |
Dowdy AJ, Mills GA, Timbal B, Wang Y (2013) Changes in the risk of extratropical cyclones in eastern Australia. Journal of Climate 26(4), 1403-1417.
| Crossref | Google Scholar |
Dowdy AJ, Pepler A, Di Luca A, Cavicchia L, Mills G, Evans J, et al. (2019) Review of Australian east coast low pressure systems and associated extremes. Climate Dynamics 53, 4887.
| Crossref | Google Scholar |
EC-Earth Consortium (2019) EC-Earth-Consortium EC-Earth3 model output prepared for CMIP6 CMIP historical. Version 20200310. (Earth System Grid Federation) [Dataset] doi:10.22033/ESGF/CMIP6.4700
Eyring V, Bony S, Meehl GA, Senior CA, Stevens B, Stouffer RJ, Taylor KE (2016) Overview of the Coupled Model Intercomparison Project Phase 6 (CMIP6) experimental design and organization. Geoscientific Model Development 9(5), 1937-1958.
| Crossref | Google Scholar |
Fogt RL, Marshall GJ (2020) The Southern Annular Mode: variability, trends, and climate impacts across the Southern Hemisphere. Wiley Interdisciplinary Reviews: Climate Change 11(4), e652.
| Crossref | Google Scholar |
Frederiksen CS, Frederiksen JS, Sisson JM, Osbrough SL (2016) Trends and projections of Southern Hemisphere baroclinicity: the role of external forcing and impact on Australian rainfall. Climate Dynamics 48(9–10), 3261-3282.
| Crossref | Google Scholar |
Freund MB, Henley BJ, Karoly DJ, McGregor HV, Abram NJ, Dommenget D (2019) Higher frequency of Central Pacific El Niño events in recent decades relative to past centuries. Nature Geoscience 12(6), 450-455.
| Crossref | Google Scholar |
Grieger J, Leckebusch GC, Donat MG, Schuster M, Ulbrich U (2014) Southern Hemisphere winter cyclone activity under recent and future climate conditions in multi-model AOGCM simulations. International Journal of Climatology 34(12), 3400-3416.
| Crossref | Google Scholar |
Grose MR, Narsey S, Delage FP, Dowdy AJ, Bador M, Boschat G, et al. (2020) Insights from CMIP6 for Australia’s future climate. Earth’s Future 8, e2019EF001469.
| Crossref | Google Scholar |
Grose MR, Michael R, Narsey S, Trancoso R, Mackallah C, Delage F, Dowdy A, et al. (2023) A CMIP6-based multi-model downscaling ensemble to underpin climate change services in Australia. Climate Services 30, 100368.
| Crossref | Google Scholar |
Hawcroft MK, Shaffrey LC, Hodges KI, Dacre HF (2012) How much Northern Hemisphere precipitation is associated with extratropical cyclones? Geophysical Research Letters 39, L24809.
| Crossref | Google Scholar |
Hersbach H, Bell B, Berrisford P, Hirahara S, Horányi A, Muñoz‐Sabater J, et al. (2020) The ERA5 global reanalysis. Quarterly Journal of the Royal Meteorological Society 146(730), 1999-2049.
| Crossref | Google Scholar |
Hope PK, Drosdowsky W, Nicholls N (2006) Shifts in the synoptic systems influencing southwest Western Australia. Climate Dynamics 26(7), 751-764.
| Crossref | Google Scholar |
Hopkins LC, Holland GJ (1997) Australian heavy-rain days and associated east coast cyclones: 1958–92. Journal of Climate 10(4), 621-634.
| Crossref | Google Scholar |
Hurley JV, Boos WR (2015) A global climatology of monsoon low-pressure systems. Quarterly Journal of the Royal Meteorological Society 141(689), 1049-1064.
| Crossref | Google Scholar |
Ji F, Pepler AS, Browning S, Evans JP, Di Luca A (2018) Trends and low frequency variability of east coast lows in the twentieth century. Journal of Southern Hemisphere Earth System Science 68, 1-15.
| Crossref | Google Scholar |
Jones DA, Wang W, Fawcett R (2009) High-quality spatial climate data-sets for Australia. Australian Meteorological and Oceanographic Journal 58(4), 233-248.
| Crossref | Google Scholar |
Jungclaus J, Bittner M, Wieners K, Wachsmann F, et al. (2019) MPI-M MPI-ESM1.2-HR model output prepared for CMIP6 CMIP historical. Version 20190815. (Earth System Grid Federation) [Dataset] doi:10.22033/ESGF/CMIP6.6594
Kalnay E, Kanamitsu M, Kistler R, Collins W, Deaven D, Gandin L, et al. (1996) The NCEP/NCAR 40-Year Reanalysis Project. Bulletin of the American Meteorological Society 77(3), 437-471.
| Crossref | Google Scholar |
Kobayashi S, Ota Y, Harada Y, Ebita A, Moriya M, Onoda H, et al. (2015) The JRA-55 reanalysis: general specifications and basic characteristics. Journal of the Meteorological Society of Japan. Ser. II 93(1), 5-48.
| Crossref | Google Scholar |
Lavender SL, Abbs DJ (2013) Trends in Australian rainfall: contribution of tropical cyclones and closed lows. Climate Dynamics 40(1–2), 317-326.
| Crossref | Google Scholar |
Lim EP, Hendon HH, Butler AH, Thompson DWJ, Lawrence ZD, Scaife AA, et al. (2021) The 2019 southern hemisphere stratospheric polar vortex weakening and its impacts. Bulletin of the American Meteorological Society 102(6), E1150-E1171.
| Crossref | Google Scholar |
Lovato T, Peano D, Butenschön M (2021) CMCC CMCC-ESM2 model output prepared for CMIP6 CMIP historical. Version 20210114. (Earth System Grid Federation) [Dataset] doi:10.22033/ESGF/CMIP6.13195
McKay RC, Boschat G, Rudeva I, Pepler A, Purich A, Dowdy A, et al. (2023) Can southern Australian rainfall decline be explained? A review of possible drivers. WIREs Climate Change 14, e820.
| Crossref | Google Scholar |
Murray RJ, Simmonds I (1991) A numerical scheme for tracking cyclone centres from digital data. Part I: development and operation of the scheme. Australian Meteorological Magazine 39(3), 155-166.
| Google Scholar |
Neu U, Akperov MG, Bellenbaum N, Benestad R, Blender R, Caballero R, et al. (2013) Imilast: A community effort to intercompare extratropical cyclone detection and tracking algorithms. Bulletin of the American Meteorological Society 94(4), 529-547.
| Crossref | Google Scholar |
Pepler A (2020) Record lack of cyclones in southern Australia during 2019. Geophysical Research Letters 47(13), e2020GL088488.
| Crossref | Google Scholar |
Pepler A, Dowdy A (2020) A three-dimensional perspective on extratropical cyclone impacts. Journal of Climate 33(13), 5635-5649.
| Crossref | Google Scholar |
Pepler A, Dowdy A (2021a) Fewer deep cyclones projected for the midlatitudes in a warming climate, but with more intense rainfall. Environmental Research Letters 16(5),.
| Crossref | Google Scholar |
Pepler A, Dowdy A (2021b) Intense east coast lows and associated rainfall in eastern Australia. Journal of Southern Hemisphere Earth Systems Science 71, 110-122.
| Crossref | Google Scholar |
Pepler AS, Dowdy AJ (2022) Australia’s future extratropical cyclones. Journal of Climate 35, 7795-7810.
| Crossref | Google Scholar |
Pepler AS, Rakich CS (2010) Extreme inflow events and synoptic forcing in Sydney catchments. IOP Conference Series: Earth and Environmental Science 11(1), 12010.
| Crossref | Google Scholar |
Pepler AS, Rudeva I (2023) Anomalous subtropical zonal winds drive decreases in southern Australian frontal rain. Weather and Climate Dynamics 4(1), 175-188.
| Crossref | Google Scholar |
Pepler AS, Di Luca A, Ji F, Alexander LV, Evans JP, Sherwood SC (2015) Impact of identification method on the inferred characteristics and variability of Australian east coast lows. Monthly Weather Review 143(3), 864-877.
| Crossref | Google Scholar |
Pepler AS, Fong J, Alexander LV (2017) Australian east coast mid-latitude cyclones in the 20th Century reanalysis ensemble. International Journal of Climatology 37(4), 2187-2192.
| Crossref | Google Scholar |
Pepler A, Dowdy A, Hope P (2019) A global climatology of surface anticyclones, their variability, associated drivers and long-term trends. Climate Dynamics 52, 5397.
| Crossref | Google Scholar |
Pepler AS, Dowdy AJ, van Rensch P, Rudeva I, Catto JL, Hope P (2020) The contributions of fronts, lows and thunderstorms to southern Australian rainfall. Climate Dynamics 55(5–6), 1489-1505.
| Crossref | Google Scholar |
Pepler AS, Dowdy AJ, Hope P (2021) The differing role of weather systems in southern Australian rainfall between 1979–1996 and 1997–2015. Climate Dynamics 56(7–8), 2289-2302.
| Crossref | Google Scholar |
Priestley MDK, Catto JL (2022) Future changes in the extratropical storm tracks and cyclone intensity, wind speed, and structure. Weather and Climate Dynamics 3(1), 337-360.
| Crossref | Google Scholar |
Priestley MDK, Ackerley D, Catto JL, Hodges KI, McDonald RE, Lee RW (2020) An overview of the extratropical storm tracks in CMIP6 historical simulations. Journal of Climate 33(15), 6315-6343.
| Crossref | Google Scholar |
Rauniyar SP, Power SB (2020) The impact of anthropogenic forcing and natural processes on past, present, and future rainfall over Victoria, Australia. Journal of Climate 33(18), 8087-8106.
| Crossref | Google Scholar |
Rauniyar SP, Hope P, Power SB, Grose M, Jones D (2023) The role of internal variability and external forcing on southwestern Australian rainfall: prospects for very wet or dry years. Scientific Reports 13(1), 21578.
| Crossref | Google Scholar | PubMed |
Risbey JS, Pook MJ, McIntosh PC, Wheeler MC, Hendon HH (2009) On the remote drivers of rainfall variability in Australia. Monthly Weather Review 137(10), 3233-3253.
| Crossref | Google Scholar |
Risbey JS, Mcintosh PC, Pook MJ (2013a) Synoptic components of rainfall variability and trends in southeast Australia. International Journal of Climatology 33(11), 2459-2472.
| Crossref | Google Scholar |
Risbey JS, Pook MJ, McIntosh PC (2013b) Spatial trends in synoptic rainfall in southern Australia. Geophysical Research Letters 40(14), 3781-3785.
| Crossref | Google Scholar |
Seferian R (2018) CNRM-CERFACS CNRM-ESM2-1 model output prepared for CMIP6 CMIP historical. Version 20181206. (Earth System Grid Federation) [Dataset] doi:10.22033/ESGF/CMIP6.4068
Simmonds I, Murray RJ, Leighton RM (1999) A refinement of cyclone tracking methods with data from FROST. Australian Meteorological Magazine SP, 35-49.
| Google Scholar |
Slivinski LC, Compo GP, Whitaker JS, Sardeshmukh PD, Giese BS, McColl C, Allan R, Yin X, Vose R, Titchner H, Kennedy J, Spencer LJ, Ashcroft L, Brönnimann S, Brunet M, Camuffo D, Cornes R, Cram TA, Crouthamel R, Domínguez‐Castro F, Freeman JE, Gergis J, Hawkins E, Jones PD, Jourdain S, Kaplan A, Kubota H, Blancq FL, Lee T, Lorrey A, Luterbacher J, Maugeri M, Mock CJ, Moore GWK, Przybylak R, Pudmenzky C, Reason C, Slonosky VC, Smith CA, Tinz B, Trewin B, Valente MA, Wang XL, Wilkinson C, Wood K, Wyszyński P (2019) Towards a more reliable historical reanalysis: improvements for version 3 of the Twentieth Century reanalysis system. Quarterly Journal of the Royal Meteorological Society 145(724), 2876-2908.
| Crossref | Google Scholar |
Speer M, Wiles P, Pepler A (2009) Low pressure systems off the New South Wales coast and associated hazardous weather: establishment of a database. Australian Meteorological and Oceanographic Journal 58(01), 29-39.
| Crossref | Google Scholar |
Timbal B, Drosdowsky W (2013) The relationship between the decline of southeastern Australian rainfall and the strengthening of the subtropical ridge. International Journal of Climatology 33(4), 1021-1034.
| Crossref | Google Scholar |
Timbal B, Arblaster J, Braganza K, Fernandez E, Hendon H, Murphy B, et al. (2010) Understanding the anthropogenic nature of the observed rainfall decline across south eastern Australia. CAWCR Technical Report Number 26, July 2010. (CSIRO and Bureau of Meteorology: Melbourne, Vic., Australia) Available at http://www.bom.gov.au/research/publications/cawcrreports/CTR_026.pdf
Utsumi N, Kim H, Kanae S, Oki T (2017) Relative contributions of weather systems to mean and extreme global precipitation. Journal of Geophysical Research: Atmospheres 122, 152-167.
| Crossref | Google Scholar |
Wang XL, Feng Y, Chan R, Isaac V (2016) Inter-comparison of extra-tropical cyclone activity in nine reanalysis datasets. Atmospheric Research 181, 133-153.
| Crossref | Google Scholar |
Wasko C, Westra S, Nathan R, Pepler A, Raupach TH, Dowdy A, Johnson F, Ho M, McInnes KL, Jakob D, Evans J, Villarini G, Fowler HJ (2024) A systematic review of climate change science relevant to Australian design flood estimation. Hydrology and Earth System Sciences 28(5), 1251-1285.
| Crossref | Google Scholar |
Yukimoto S, Koshiro T, Kawai H, Oshima N, et al. (2019) MRI MRI-ESM2.0 model output prepared for CMIP6 CMIP historical, Version 20190722. (Earth System Grid Federation) [Dataset] doi:10.22033/ESGF/CMIP6.6842
Ziehn T, Chamberlain M, Lenton A, Law R (2019) CSIRO ACCESS-ESM1.5 model output prepared for CMIP6 CMIP historical, Version 20200529. (Earth System Grid Federation) [Dataset] doi:10.22033/ESGF/CMIP6.4272