Utilisation of local emission inventory data for forecasting PM10 using the WRF-Chem model in the Bandung Basin
Prawira Yudha Kombara
A
B
Abstract
The 2015 local emission data from the Ministry of Environment and Forestry of the Republic of Indonesia is used as the anthropogenic emission input for the WRF-Chem model to forecast particulate matter with a size of 10 μm or less (PM10). The research examines the model’s performance when the anthropogenic emission data were replaced from global to local. The study focuses on the Bandung Basin, running the model for both dry and wet seasons. Two scenarios are conducted for each season: the first (control scenario) uses global emissions, whereas the second (updated scenario) utilises local emissions. The results indicate that the WRF-Chem model’s performance improved slightly when regional emissions replaced global emissions in either season. When the model’s output was compared with ground station data, the PM10 pattern of the second scenario followed the pattern of the observation data. Regarding the Pearson correlation and root mean square error (RMSE), the wet season result exhibits a better score than the dry season result. Though the RMSE for both seasons shows an unsatisfactory score, the Pearson Correlation shows a good score. Both scenarios produce a poor RMSE score, and these results reveal that anthropogenic emissions updated with local emissions still cannot produce a high accuracy of PM10 prediction in the Bandung Basin.
Keywords: air pollution, Bandung Basin, dry season, forecasting, Indonesia, local emissions, PM10, wet season, WRF-Chem.
1.Introduction
Bandung is a capital city located in the centre of West Java province. Its topography resembles a bowl because mountains surround it. According to the IQAir report of 2022, the air quality technology company of Switzerland states that air pollution conditions in Bandung are not as severe as in Jakarta (IQAir 2023). However, anticipatory action should be taken, and forecasting air pollution is a critical step for formulating effective policies, minimizing economic losses, and improving planning.
Forecasting air pollution can be conducted by using numerical models such as the Weather Research Forecasting (WRF)-Chem model. The WRF-Chem model is one of the numerical models that has been used widely by the academic community and research institutions around the world to forecast air pollution. WRF-Chem is a WRF model that is equipped with a chemistry module to calculate atmospheric chemistry (Grell et al. 2005; Fast et al. 2006; Baklanov et al. 2014; Powers et al. 2017). To run the WRF-Chem model, it is necessary to provide additional input data, such as emission and background chemistry data, to compute the atmospheric chemistry components. Emission data refers to the estimated amount of air pollutants released from various emission sources into the atmosphere. These data are typically expressed in units of mass, for example, kilograms or tonnes (Mg), per unit of time, for example, months or years. These data are crucial for understanding the impact of different emission sources, such as industrial facilities, vehicles, or natural processes, on air quality and for developing strategies to mitigate air pollution.
Generally, emission data are already available globally and comprise sectors such as transportation, industry, and agriculture. One repository of global emission data is the Emission Database for Global Atmospheric Research (EDGAR), and the latest version of data that can be used for WRF-Chem input is Ver. 5 (2015) (Crippa et al. 2019).
Several previous studies have used the EDGAR emission data as emission input for running the WRF-Chem model in the Indonesian region. One of those studies was done by Kombara and Komala (2023). Their research used EDGAR Ver. 5 as WRF-Chem input and simulated particulate matter with a diameter of 2.5 μm or less (PM2.5) and ozone from 2 until 4 August 2021 for Jakarta and its surrounding area. They compared the results of the model with data from three ground stations at Jakarta, Bekasi and Serang. Based on their research, the simulation results still did not produce a satisfactory output. The Pearson correlation and root mean square error (RMSE) results revealed that the use of EDGAR Ver. 5 did not help the model produce accurate results (Kombara and Komala 2023).
Besides global emission data, there are other emission databases for small regions. These emission databases are usually built by each country or research organisation for implementation in a specific area. Several studies have used their emission data to simulate air pollution in their region (Marrapu et al. 2014; Kuik et al. 2016; Cifuentes et al. 2021; Spiridonov et al. 2021; Qiu et al. 2023). In Indonesia, emission data from several sectors have been collected every year by the Republic of Indonesia’s Kementrian Lingkungan Hidup dan Kehutanan (KLHK, the Department of Air Pollution Control, Ministry of Environment and Forestry). The KLHK emission data have been used for simulating air pollution prediction in Indonesia using the WRF-Chem model (Faisal and Sofyan 2019; Pratama and Sofyan 2020). Pratama and Sofyan (2020) used KLHK emission data to predict PM10 in the Bandung Basin. Their research tried to predict PM10 in the Bandung Basin using the WRF-Chem model in the dry season and wet season of 2017. However, their result was not verified with observation data, specifically for PM10 parameters. Therefore, we wanted to use the KLHK emission data as WRF-Chem model input to predict air pollution in the Bandung Basin and verify the result with observation data. This is an important step to explore so that we can assess the performance of WRF-Chem when we use local emission data in Indonesia. However, because of our limited access to observation weather data from the Meteorological Agency of Indonesia, we were not able to use the data assimilation method for predicting weather parameters as in Pratama and Sofyan (2020). Therefore, instead of the assimilation method used in Pratama and Sofyan (2020), in the present study, we only executed the WRF-Chem with default configurations and used the KLHK emission data to predict PM10 concentrations in the Bandung Basin during two recent seasons, specifically the dry season in 2021 and the wet season in 2022. PM10 is an example of several dangerous pollutants in the atmosphere that affect human health, especially the respiratory system. Equally or more dangerous for human health is PM2.5, owing to its smaller size (Fitri et al. 2021). We should predict PM2.5; however, in this case, we only had the PM10 parameter from the KLHK emission data. In addition, the reason we chose 2021 and 2022 was because of our limited access to KLHK ground station data to evaluate the model results.
2.Methods
2.1. Study area
According to Aldrian and Dwi Susanto (2003), Bandung is an area that has a monsoon climate type. This type has the characteristic of two seasons, namely the wet and the dry seasons, with peak rainfall in December, January, and February and minimum rainfall in June, July, and August (Aldrian and Dwi Susanto 2003). The differences in characteristics between these two seasons can affect the concentration of pollutants in the area. Further, meteorological conditions in one place are also influenced by other factors, such as topographic characteristics. Bandung is an Indonesian city with distinctive topographic characteristics. This is due to the city being surrounded by higher plains and mountains, forming a basin, as shown in Fig. 1. Such topography affects Bandung’s atmospheric circulation and pollutants. Fig. 1 shows the topography of the city of Bandung from the Shuttle Radar Topography Mission (SRTM), with a spatial resolution of 30 m.
2.2. Model setup
For this research, we used the WRF-Chem model ver. 4.2.2 with meteorological input from Global Forecast System (GFS) data. The GFS data are the global weather predictions produced by the National Oceanic and Atmospheric Administration (NOAA). These data are usually used as input data or initial conditions in numerical weather prediction models, and we downloaded the GFS data from rda.ucar.edu. The specifications of the GFS in this study were 0.25° or ~27 km for the spatial resolution, 3 h for the time resolution, and 00 for the cycle. To forecast PM10 in the Bandung Basin, we created two nesting domains, as shown in Fig. 1. The spatial resolution for the first domain is 5 km and 1 km for the second domain. We ran the model with 50 layers of vertical levels. To run the WRF-Chem model, several parameterisation schemes can be used. The details of the parameterisation schemes used in this study are presented in Table 1.
Schemes | First domain (5 km) | Second domain (1 km) | |
---|---|---|---|
Cumulus | Grell 3D | – | |
Microphysics | Purdue Lin | Purdue Lin | |
Shortwave radiation | Dudhia | Dudhia | |
Longwave radiation | Rapid Radiative Transfer Model (RRTM) | RRTM | |
Boundary layer | Yonsei University (YSU) | YSU | |
Chemistry | Carbon Bond Mechanism version Z (CBMZ) chemical mechanism and MOSAIC using four sectional aerosol bins | CBMZ chemical mechanism and MOSAIC using four sectional aerosol bins |
As mentioned in the previous section, to run the WRF-Chem model, emission data are necessary, particularly anthropogenic emission data. This simulation used two kinds of anthropogenic emission data, namely the EDGAR emission and the KLHK or local emission data. We utilised EDGAR Emission ver. 2015, which can be downloaded by accessing this URL: https://www2.acom.ucar.edu/wrf-chem. EDGAR Emission ver. 5 is produced from emission measurements of several sectors, such as transportation, aviation, energy, shipping, food, agriculture, industry, and manufacturing. Then, the emission data are provided in grid form using technology-specific emission factors. In addition, the spatial and time resolution for these data is 0.1° or ~11 km and monthly (Crippa et al. 2021; Thunis et al. 2021). However, the original version of KLHK emission data are presented in point, line and area data separately for each sector. Thus, we had to combine and calculate the total from all sectors to find the total PM10 emissions in the Bandung Basin area. Then, we converted the KLHK emission data from ASCII type to raster so that the WRF-Chem model could use it (Pratama and Sofyan 2020). Additionally, the KLHK emission data also comprised several sectors, such as activities in hotels, crematoriums, industry, shopping centres, hospitals and activities on every road in Bandung City. Also, to calculate the possibility of aerosol supply from sea salt, we turned on the seasalt emission option (seas_opt = 2).
We ran the model two times in each season because we wanted to know how the model performed in different seasons when we replaced the emission input. We ran the model for the dry season on 18 July 2021 and 28 January 2022 for the wet season sample. For both simulations, we provided 24 h for spin-up time. The selection of the date was considered based on the completeness of the observation data. Then, in the first experiment, we used global emission data as anthropogenic emission input in both domains, and we called it the control scenario. Next, in the second experiment, we used the KLHK emission data for anthropogenic emission input in the second domain, and we called it the updated scenario. The reason the second domain used the KLHK emission data is because of the limitations in regional emission data we had at the time we wrote this study. Then, the emission data from KLHK were converted to micrograms per second per metre squared (µg s–1 m–2) for WRF-Chem emission input.
Further, we used the biogenic emission data in these simulations from the Model of Emissions of Gases and Aerosols from Nature (MEGAN). The source of biogenic emission data was the old version, and we did not update it.
2.3. Model evaluation
To evaluate the prediction result, we compared the pollutant output from the model with the ground station data of KLHK. This ground station is at −6.901417°S, 107.625601°E, Bandung, West Java. By contrast, to evaluate the meteorological parameters such as surface temperature and 10-m wind speed, we used observation data from the automatic weather station (AWS) located on the rooftop of the office of Badan Riset dan Inovasi Nasional (BRIN, the National Research and Innovation Agency) in Bandung with the coordinates −6.895°S, 107.587°E. The Pearson correlation coefficient (r) and RMSE methods were used to evaluate the prediction results.
The Pearson correlation coefficient (r) is a method used to confirm the correlation between two variables. Here, we compare the prediction and observation variables to identify linearity and similarity patterns. The value of r ranges from −1 to +1. To calculate the value of r, we used Eqn 2:
where F is the forecast or model variable, and O is the observation data.
The RMSE is used to investigate the difference in magnitude between the model or prediction and the observation data. We calculate the RMSE using Eqn 3:
3.Results
3.1. Meteorological variable evaluation
For meteorological parameter evaluation, we selected the 2-m air temperature, surface pressure and 10-m surface wind speed parameters. To evaluate the 2-m air temperature and surface pressure, the model output was compared with observation data and is presented in a time series shown in Fig. 2. Further, Table 2 shows the results of Pearson correlation (r) and root mean square error (RMSE) calculations. The model results for 2-m air temperature exhibit a similar pattern to the observation data for both seasons. These results are confirmed by the r score reaching 0.99 for the dry season and 0.97 for the wet season. Furthermore, the RMSE for 2-m temperature has a low score, which shows good agreement between the model and observations.
The comparison of 2-m temperature (a, b), and surface pressure (c, d) from model result and observation in the dry season (a, c) and wet season (b, d).
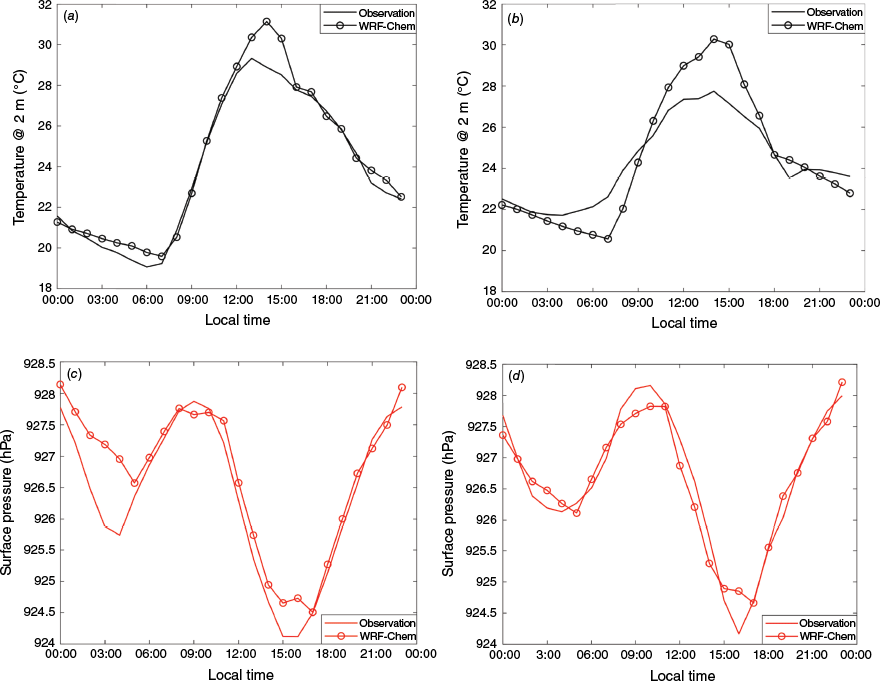
Dry season | Wet season | ||||
---|---|---|---|---|---|
Temperature @ 2 m | Surface pressure | Temperature @ 2 m | Surface pressure | ||
Pearson correlation | 0.99 | 0.95 | 0.97 | 0.97 | |
RMSE | 0.72 | 0.488 | 1.28 | 0.28 |
The second parameter is the surface pressure and this parameter shows excellent performance. This is confirmed by the r score of 0.95 for the dry season and 0.97 for the wet season. The pattern of surface pressure from the model output is very similar to the observation data for both seasons, and the model output and observation data present a semidiurnal pattern. Additionally, the RMSE score for surface pressure is lower than 0.5 for both seasons.
The next meteorological parameter is 10-m surface wind. To evaluate 10-m surface wind, this study uses a visual comparison presenting the parameter in a wind rose diagram, shown in Fig. 3. In the wet season, the prevailing wind in the Bandung basin is a westerly wind that is caused by the Asian winter monsoon. Both the model result and the observation data exhibit a similar pattern, as presented in Fig. 3a, c. Then, for the dry season, the prevailing wind usually blows from the south-east or can be said to be an easterly wind as the Asian summer monsoon is active. The model result and observation data show the same pattern, seen in Fig. 3b, d. Therefore, based on the 2-m air temperature, surface pressure and the 10-m surface wind result, the model shows impressive performance in predicting the meteorological parameters in the Bandung region.
3.2. Local and global emission of PM10 data in Bandung Basin
The local inventory of PM10 emissions carried out in this research comes from various sources, namely point, area and line sources. As mentioned in Section 2, these data come from generator activities in hotels, crematoriums, industry, shopping centres and hospitals, activity on every road in Bandung City and household activities. The total number of point sources inventoried was 365 sources and 205 roads in Bandung City. The data were digitised and converted to raster grid format with a resolution of 1 km.
PM10 emissions from points, areas and line sources were added to get the total emission of PM10 in Bandung City. From Fig. 4, it is seen that line sources make the most significant contribution to PM10 emission compared with the other sources. The most significant sources are in the south (red line), namely the Padaleunyi Toll Road and the Soekarno–Hatta Road. The emission amount from both sources is between 180 and 430 Mg year–1 for PM10. In addition, other regions, such as Setiabudhi Road, Ahmad Yani Road and Pasteur Toll Road, also contributed substantially to PM10 emissions.
A comparison between the local and global emissions of PM10 can be seen in Fig. 5. It presents the spatial plots of PM10 for dry and wet seasons, showing a strong discrepancy between the local and global emissions. For global emissions, we can see there is a slight discrepancy between dry and wet seasons in Bandung City, particularly in the southern region (Fig. 5a, c). In the wet season, the magnitude of PM10 emission is higher than in the dry season for the southern region of Bandung City. However, for local emissions, there are no differences between dry and wet seasons in the Bandung City area (Fig. 5b, d). This shows that there are no monthly variations of emission magnitude for local emission data; in other words, the local emissions only have one value for a year from all sectors. By contrast, PM10 emissions from the global data have variations every month from all sectors, although it is only a slight variation. This discrepancy gives different model responses in predicting the PM10 in the Bandung Basin.
3.3. Dry season
The evaluation and comparison of the model results with the KLHK station for the dry season can be seen in Fig. 6. Fig. 6a shows the time series of model results (control and updated) and the KLHK station for PM10 in the dry season. We see that the pattern of model results for the updated scenario follows the observation data pattern, although it is not too similar. However, we can say that the updated result is better than the control scenario as it follows the pattern of the observation data.
Time series of PM10 consisting of KLHK observation data, the control scenario, and the updated scenario in (a) the dry season, and (b) the wet season.
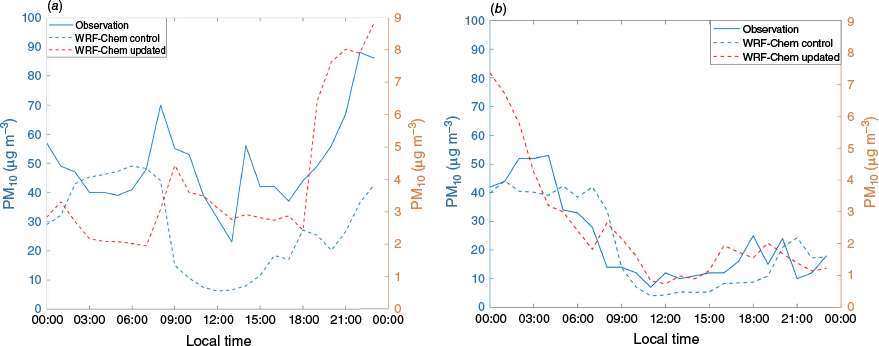
The observation data do not exhibit a perfect diurnal pattern, but there are several peaks in 1 day. Note that the location of the measurement station is near a main street. The measurement result has several peaks associated with the volume of vehicles. Aside from that, both PM10 from the control and updated models have a lower magnitude than the KLHK data, or we can say that the model produces an underestimate of PM10. In particular, the magnitude of the updated result is the smallest and weakest. Then, as shown in Table 3, the updated scenario has a higher correlation (r) value than the control scenario. The r-value of the control scenario is 0.29, whereas the r-value of the updated scenario is 0.73. This result confirms that the updated scenario has a better performance in the similarity of patterns.
3.4. Wet season
For the wet season, the model output shows a better result than the dry season. Based on Table 3, we can see that the r value in the wet season is higher than in the dry season. Table 3 shows the control scenario, which has an r value of ~0.83 and 0.8 for the updated scenario. Further, based on Fig. 6b, generally, we can see the model result exhibits a similar pattern to the observation data. The figures and tables indicate that the wet season results follow the observation pattern better than the dry season, and the RMSE value is smaller than in the dry season. The control scenario has an RMSE value of ~8.99 and 24.92 for the updated scenario. However, the performance of the control scenario is still slightly better than the updated scenario whereas it is the opposite with the dry season result. In addition, the peak of PM10 occurs before sunrise, and the minimum concentration occurs c. 10:00 or 11:00 hours. Despite the good performance of the PM10 diurnal cycle pattern, similarly to the dry season, the wet season also underestimates the observed PM10 values by orders of magnitude. This suggests the model has limitations in simulating PM10 accurately.
4.Discussion
In this section, we discuss the reasons for the slight improvement in model performance for predicting PM10 after changing the global anthropogenic emission to regional emission data. Several studies show that the use of regional or local emission data can increase model performance to predict air pollution. For example, Fountoukis et al. (2022) used local anthropogenic emission data to predict air pollutants in the Qatar area. They stated that the use of local emission data decreased the error of the model from −51 to 8% for PM2.5 and −88 to 20% for NOx prediction (Fountoukis et al. 2022). Another example is the study carried out by Spiridonov et al. (2021), who used regional emissions collected every year by the Ministry of Environmental and Physical Planning of Macedonia. Further, they also updated their regional emission data with seasonal variability so that there was variation in each season. The seasonal variability of emissions was obtained from mobile emissions measurements. The results showed that the model could capture the PM10 pattern well and present PM10 values more realistically (Spiridonov et al. 2021).
The control scenario in our simulation used EDGAR emission data for both domains in the WRF-Chem model. EDGAR emission is anthropogenic global emission data that have monthly time resolution so that they have monthly and seasonal variation (Crippa et al. 2019, 2020). However, the regional or local emission data from KLHK only have one value in 2015 for each sector, as mentioned in Section 3.2 and in Fig. 5. KLHK emission data are an average of 1-year measurement, so the final product only has one value for each pollutant and sector. We suspect this is the reason why the prediction result of the updated scenario still has a very large RMSE score. However, we changed the global anthropogenic emissions to local emissions and turned on the sea-salt emission option. Because the EDGAR data have monthly and seasonal variations, they can give a lower RMSE score. Although we believe the lack of monthly and seasonal variations is the reason for the high RMSE and underestimated PM10 values obtained in this study, further investigation is needed to understand the cause of this discrepancy. In addition, the EDGAR ver. 5 data is 2015 data; thus, it is old data and if we use it for predicting current conditions, it is difficult for the model to simulate the PM10 accurately. As we know, air pollution is influenced by atmospheric conditions, as the atmosphere changes with time. Thus, if we use old emissions input, there is a possibility that the prediction results will be less accurate. Therefore, we argue that local emission data should be used to estimate daily PM10. A further improvement would be the inclusion of seasonal variability in the local emission data input, as used by Spiridonov et al. (2021).
5.Conclusion
KLHK or local anthropogenic emission data from 2015 were used in this project as the WRF-Chem model input for predicting PM10 in the Bandung Basin during dry and wet seasons. We conducted two scenarios for this experiment; the first or control scenario used global emission EDGAR data, and the second or updated scenario used the KLHK emissions data. Based on the PM10 prediction results in this simulation, the replacement of the global anthropogenic emissions with local emission data still does not enhance model performance overall. The updated scenario has a better Pearson correlation value than the control scenario, particularly in the dry season. However, the RMSE is too high in the updated scenario. In addition, when we compare the results between the two seasons, the wet season prediction is better simulated than the dry season, as confirmed by the relatively higher correlation coefficient and lower RMSE. Further investigation is needed to elucidate the reason for this difference in the season predictions. In conclusion, the use of local emission data in our study did not improve PM10 prediction in the Bandung Basin significantly. The reasons for this remain unclear but it may be attributed to the data temporal resolution and discrepancies in data quality. Future research may want to consider the inclusion of monthly and seasonal emission data as well as more up-to-date datasets of local emissions.
Data availability
The GFS data used in this research can be found at: https://nomads.ncep.noaa.gov/pub/data/nccf/com/gfs/prod/.
Declaration of funding
The authors received funding for this research from Rumah Program Kebencanaan DIPA OR KM BRIN number SP DIPA-124.01.1.690501/2023.
Acknowledgements
We express our gratitude to Rumah Program Kebencanaan DIPA ORKM BRIN for funding research support. We also acknowledge the use of the KLHK emission and ground observation data for the Bandung region.
References
Aldrian E, Dwi Susanto R (2003) Identification of three dominant rainfall regions within Indonesia and their relationship to sea surface temperature. International Journal of Climatology 23, 1435-1452.
| Crossref | Google Scholar |
Baklanov A, Schlünzen K, Suppan P, Baldasano J, Brunner D, Aksoyoglu S, Carmichael G, Douros J, Flemming J, Forkel R, Galmarini S, Gauss M, Grell G, Hirtl M, Joffre S, Jorba O, Kaas E, Kaasik M, Kallos G, Kong X, Korsholm U, Kurganskiy A, Kushta J, Lohmann U, Mahura A, Manders-Groot A, Maurizi A, Moussiopoulos N, Rao ST, Savage N, Seigneur C, Sokhi RS, Solazzo E, Solomos S, Sørensen B, Tsegas G, Vignati E, Vogel B, Zhang Y (2014) Online coupled regional meteorology chemistry models in Europe: current status and prospects. Atmospheric Chemistry and Physics 14, 317-398.
| Crossref | Google Scholar |
Cifuentes F, González CM, Aristizábal BH (2021) Dataset for evaluating WRF-Chem sensitivity to biogenic emission inventories in a tropical region. Global online model (MEGAN) vs local offline model (BIGA). Data Break 38, 107438.
| Crossref | Google Scholar |
Crippa M, Guizzardi D, Muntean M, Schaaf E, Oreggioni G (2019) EDGAR v5.0 Global Air Pollutant Emissions. (European Commission, Joint Research Centre) Available at http://data.europa.eu/89h/377801af-b094-4943-8fdc-f79a7c0c2d19 [Dataset, verified 10 November 2023]
Crippa M, Solazzo E, Huang G, Guizzardi D, Koffi E, Muntean M, Schieberle C, Friedrich R, Janssens-Maenhout G (2020) High resolution temporal profiles in the Emissions Database for Global Atmospheric Research. Scientific Data 7, 121,.
| Crossref | Google Scholar | PubMed |
Crippa M, Guizzardi D, Pisoni E, Solazzo E, Guion A, Muntean M, Florczyk A, Schiavina M, Melchiorri M, Hutfilter AF (2021) Global anthropogenic emissions in urban areas: patterns, trends, and challenges. Environmental Research Letters 16, 074033.
| Crossref | Google Scholar |
Faisal I, Sofyan DA (2019) Analisis Pengaruh Variasi Musiman Terhadap Dispersi NO2 Di Kota Tangerang Dengan Menggunakan Model WRF-Chem. Analysis of the seasonal variation influence on the NO2 dispersion in the tangerang city by utilizing the WRF-Chem Model. Jurnal Teknik Lingkungan 25(1), 1-14 [In Indonesian with title, abstract and keywords in Indonesian and English].
| Google Scholar |
Fast JD, Gustafson WI, Easter RC, Zaveri RA, Barnard JC, Chapman EG, Grell GA, Peckham SE (2006) Evolution of ozone, particulates, and aerosol direct radiative forcing in the vicinity of Houston using a fully coupled meteorology–chemistry–aerosol model. Journal of Geophysical Research 111, D21305.
| Crossref | Google Scholar |
Fitri DW, Afifah N, Anggarani SMD, Chamidah N (2021) Prediction concentration of PM2.5 in Surabaya using ordinary Kriging method. AIP Conference Proceedings 2329, 060030.
| Crossref | Google Scholar |
Fountoukis C, Mohieldeen Y, Pomares L, Gladich I, Siddique A, Skillern A, Ayoub MA (2022) Assessment of high-resolution local emissions and land-use in air quality forecasting at an urban, coastal, desert environment. Aerosol and Air Quality Research 22, 220001.
| Crossref | Google Scholar |
Grell GA, Peckham SE, Schmitz R, McKeen SA, Frost G, Skamarock WC, Eder B (2005) Fully coupled ‘online’ chemistry within the WRF model. Atmospheric Environment 39, 6957-6975.
| Crossref | Google Scholar |
IQAir (2023) 2022 World Air Quality Report: Region & City PM2.5 Ranking. (Greenpeace) Available at https://www.greenpeace.org/static/planet4-thailand-stateless/2023/03/d1d69c24-2022_world_air_quality_report_en.pdf
Kombara PY, Komala N (2023) Utilization EDGAR fifth version as input emission of WRF-Chem model for simulating ozone and PM2.5 over Jakarta and its surroundings area. In ‘Proceedings of the International Conference on Radioscience, Equatorial Atmospheric Science and Environment and Humanosphere Science. INCREASE 2022. Springer Proceedings in Physics, Vol 290’. (Eds A Basit, et al.) pp. 161–171. (Springer: Singapore) 10.1007/978-981-19-9768-6_16
Kuik F, Lauer A, Churkina G, Denier Van Der Gon HAC, Fenner D, Mar KA, Butler TM (2016) Air quality modelling in the Berlin–Brandenburg region using WRF-Chem v3.7.1: sensitivity to resolution of model grid and input data. Geoscientific Model Development 9, 4339-4363.
| Crossref | Google Scholar |
Marrapu P, Cheng Y, Beig G, Sahu S, Srinivas R, Carmichael GR (2014) Air quality in Delhi during the Commonwealth Games. Atmospheric Chemistry and Physics 14, 10619-10630.
| Crossref | Google Scholar |
Powers JG, Klemp JB, Skamarock WC, Davis CA, Dudhia J, Gill DO, Coen JL, Gochis DJ, Ahmadov R, Peckham SE, Grell GA, Michalakes J, Trahan S, Benjamin SG, Alexander CR, Dimego GJ, Wang W, Schwartz CS, Romine GS, Liu Z, Snyder C, Chen F, Barlage MJ, Yu W, Duda MG (2017) The weather research and forecasting model: overview, system efforts, and future directions. Bulletin of the American Meteorological Society 98, 1717-1737.
| Crossref | Google Scholar |
Pratama A, Sofyan A (2020) Analisis dispersi pencemar udara PM10 di Kota Bandung menggunakan WRFCHEM data asimilasi. PM10 air pollution dispersion analysis in Bandung City using WRFCHEM data assimilation. Jurnal Teknik Lingkungan 26, 19-36 [In Indonesian with title, abstract and keywords in Indonesian and English].
| Google Scholar |
Qiu Y, Feng J, Zhang Z, Zhao X, Li Z, Ma Z, Liu R, Zhu J (2023) Regional aerosol forecasts based on deep learning and numerical weather prediction. npj Climate and Atmospheric Science 6, 71.
| Crossref | Google Scholar |
Spiridonov V, Ancev N, Jakimovski B, Velinov G (2021) Improvement of chemical initialization in the air quality forecast system in north Macedonia, based on WRF-Chem model. Air Quality, Atmosphere and Health 14, 283-290.
| Crossref | Google Scholar |
Thunis P, Crippa M, Cuvelier C, Guizzardi D, de Meij A, Oreggioni G, Pisoni E (2021) Sensitivity of air quality modelling to different emission inventories: a case study over Europe. Atmospheric Environment: X 10, 100111.
| Crossref | Google Scholar |