Microbial genes highlight different trends in short term for N cycling in historical alpine pastures
Salvatore Raniolo
A
Abstract
Alpine pastures are seminatural grasslands which play a crucial role in biodiversity conservation, service provisioning, and mountain livestock systems. The soil microbial communities of pasture are fundamental in ecosystem nutrient cycles, but they are relatively underexplored in European Alpine pastures.
We explored the many soil microbial genes encoding key functions in the nitrogen cycle in three historical alpine pastures grazed by dairy cattle, considering different soils, temporal dynamics, and exclusion of cattle grazing for one summer.
216 samples were collected across four sampling times. The abundance of genetic determinants involved in nitrogen fixation (nifH), nitrification (amoA bacterial and archaeal), and denitrification (nirK and nosZ) were quantified using real-time polymerase chain reaction.
The terminal denitrification nosZ gene was the most sensitive indicator and responded significantly to soil chemical composition and animal grazing. Sampling time affected nitrogen fixation nifH and intermediate denitrification nirK in relation to rainfall cumulation dynamics. The amoA nitrification genes showed high variability but no significant effects from the tested factors.
In spite of a general homeostatic trend occurring in these habitats and of the short term analysis, some genes acted as sensitive reporters of soil compositional differences, intraseasonal climatic variations, and grazing disturbance.
A stocking rate of >0.6 livestock units per hectare can be recommended, to combine animal production with conditions that favour complete denitrification, thus potentially reducing the nitrous oxide greenhouse gas emissions. Higher livestock grazing intensity can be withstood by the ecosystem without denitrification-related drawbacks when the preceding 10 days display a cumulated rainfall lower than 22 mm.
Keywords: alpine grassland, denitrification, grazing, microbial nitrogen function, nitrogen cycle, nitrification, pasture, real-time PCR.
Introduction
Soil is the basis of all terrestrial ecosystems and its microbial biodiversity supports many ecosystem processes through the unfolding of central biogeochemical cycles, such as those of carbon and nitrogen (Zhou et al. 2012; Cavicchioli et al. 2019). Nutrient cycles encompass many functions, based on enzyme-catalysed reactions running within the metabolic pathways of different microbial guilds (Rocca et al. 2015; Louca et al. 2018; Dong et al. 2020). In microbial communities, redundancy and diversity are fundamental for sustaining resilient biogeochemical processes (Louca et al. 2018; Maron et al. 2018). Thus, microbial communities should be considered as sets of functional groups in which species richness per se is less relevant than phenotypic traits for specific functions, which define the actual functional richness of the biochemical processes (Moonen and Bàrberi 2008; Bahram et al. 2018; Louca et al. 2018). Such functional richness ultimately relies on the genetic content of communities, basing on the central tenet of molecular biology, where biological information passes from genes to their transcripts and then to protein synthesis. Hence, enzyme-catalysed reactions depend on the presence of specific genes, which determine the metabolic niches of microbial communities and their potential functions (Rocca et al. 2015; Louca et al. 2018). The abundance of a gene reflects that of its carrying population, as its multiplication testifies the relevance of that microbial group within the ongoing local processes (De Boer and Kowalchuk 2001; Yergeau et al. 2007; Jia and Conrad 2009; Beule et al. 2019). For this reason, the relative frequency of a specific gene within amicrobial population can determine how widely the corresponding phenotype will be expressed, once reaction substrates of those enzymes become available under favourable boundary conditions. From this standpoint, microbial protein-encoding genes can be used both as reporters of the leading biochemical occurrences and as predictive indicators of potential for specific ecosystem functions to be performed (Wallenstein and Vilgalys 2005; Lindsay et al. 2010).
The soil gene pool potentially provides multiple functions, such as regulating (e.g. carbon sequestration) and supporting (e.g. nutrient cycles) services. These functions in soils are also linked to the modulation of greenhouse gases including carbon dioxide (CO2), methane (CH4) and nitrous oxide (N2O,) ruling the balance between their sequestration and emission via microbiota (Pereira et al. 2018). N2O is an ozone-depleting substance and a greenhouse gas (Eichner 1990) with a global warming potential 298-fold higher than CO2 over 100 years and an estimated contribution to global warming of 7.9% (Hackl et al. 2000; Wu et al. 2021). Grasslands are among the most important terrestrial ecosystems in the world, covering over 37% of our planet’s terrestrial areas (Zhong et al. 2015), and 23% of the European Alpine region (Ali et al. 2016). They represent the second largest terrestrial carbon sink (Ali et al. 2016; Yang et al. 2019), are key habitats for animal and plant biodiversity (Marini et al. 2009; Jerrentrup et al. 2016; Nota et al. 2020), and provide multiple ecosystem services (McSherry and Ritchie 2013; Rodríguez-Ortega et al. 2014). Grassland soils are also among the main sources of N2O (Henry et al. 2006; Zhong et al. 2015; Wu et al. 2021) and those used for agriculture (intended as pasture) contribute with more than 10% of the total N2O emissions of global agricultural land, equal to 40% of the 6–7 gt emitted in total per year (Bell et al. 2016). However, the estimation of N2O emissions from grassland is still uncertain due to a gap in information about nitrogen (N) dynamics in different contexts, considering both environmental drivers and microbial communities (López-Aizpún et al. 2020). In natural ecosystems, microbial pathways control not only nitrification (Schmidt 1982), but also nitrous oxide emission. This gas is generated mainly by incomplete denitrification or as a byproduct of nitrification (Tiedje 1988; Orellana et al. 2014; Di and Cameron 2018). In microbial communities, denitrification involves a sequential series of reactions. The intermediate step of denitrification, corresponding to the reduction of nitrite to nitric oxide, involves the nirS and nirK genes, which encode for nitrite reductase (Pauleta et al. 2013). The final step in denitrification is catalysed by nosZ, encoding for nitrous oxide reductase (N2OR), the specific enzyme catalysing N2O reduction to N2 (Pauleta et al. 2013). The role of this gene in bringing about complete denitrification was highlighted by different studies, which showed a negative correlation between nosZ and nitrous oxide emission (Du et al. 2019; Zhou et al. 2022). At the opposite corner of the N cycle, the gene amoA encodes for ammonia monooxygenase, the enzyme for the first and limiting reaction in the nitrification process (De Boer and Kowalchuk 2001; Di and Cameron 2018; Wrage-Mönnig et al. 2018). In terrestrial ecosystems, an important nitrogen input is the fixation of atmospheric N2 to biologically available ammonium. This is mediated by prokaryotes, both symbiotic and free-living (Rösch et al. 2002; Pereira e Silva et al. 2013; Regan et al. 2017). Among the free-living prokaryotes, the gene nifH encodes for the iron nitrogenase subunit, within the complex responsible for the nitrogen fixation, which is a fundamental process for the entire N cycle (Rösch et al. 2002). The abundance values of nifH, nirK, nosZ, and amoA genes have been used as indicators to assess potential nitrogen fixation, denitrification and nitrification through quantitative real-time polymerase chain reaction (qPCR) approaches (Morales et al. 2010; Verhamme et al. 2011; Jones et al. 2014; Yang et al. 2014; Hu et al. 2015; Rocca et al. 2015; Wrage-Mönnig et al. 2018). qPCR is used to quantify the number of copies in the soil of the starting target gene, which is used as a reporting measure for the potential of its corresponding ecosystem process (Henry et al. 2004). This analysis has been used in many different contexts: from the treatment of wastewater (Wakelin et al. 2008) to different agroecosystems, such as croplands (Colloff et al. 2008; Beule et al. 2019), forests (Lindsay et al. 2010), and grasslands (Wakelin et al. 2009).
Recent studies have started to fill the above mentioned gap in knowledge between the environmental chemistry and ecosystem biology, characterising the N dynamics in grasslands through microbial functional genes, such as nifH, nirK, nosZ, and amoA, in the context of Asian mountains (Du et al. 2019; Dong et al. 2020; Zhang et al. 2021), or European hill/low mountain areas (Chroňáková et al. 2009; Andrade-Linares et al. 2021; Raniolo et al. 2023). Much less information about N microbial functional genes has been reported as regards seminatural grasslands managed through grazing in European Alpine areas (Raniolo et al. 2023). For these agroecosystems, grazing by megaherbivores, such as cattle, can be considered a management practice as well as a prescribed disturbance factor in an ecological sense. In fact, grazing has both direct impacts on the vegetation and soil of grasslands by defoliation and trampling, and indirect ones because of the release of nutrients that affect microbial communities, with possible consequences in the long term. Through selection and predation of plant species, grazing modifies vegetation composition and causes rhizosphere alterations, which may have indirect effects on soil structure (Qu et al. 2016). Plant diversity can support microbial soil diversity with positive implications on species redundancy and on the use of the available resources (Mendes et al. 2015; Delgado-Baquerizo et al. 2016). For example, the nosZ gene seems positively influenced by perennial plant cover in agroecosystems (Morales et al. 2010). Moreover, predation by herbivores seems to induce plants to allocate more resources into root development and release of root exudates, with a consequent increase in soil carbon (Bardgett and Wardle 2003). Instead, in terms of soil conditions, grazing could contribute indirectly to an increase in heterotrophic nitrifiers by diminishing the aeration of soil due to trampling, and by increasing the supply of organic matter due to dung inputs (Chroňáková et al. 2009; Zhong et al. 2015; Du et al. 2019; Yin et al. 2020). These effects have direct consequences on the interspecific competition among microorganisms, especially in the rhizosphere (Hackl et al. 2000; Li et al. 2016). Grazing might also modify the species composition of microbial communities by introducing additional members from animal dung (NandaKafle et al. 2017). Additionally, soil microbial communities are influenced by a variety of soil chemical properties and land morphology features (Paul 2014; Bahram et al. 2018). Among the former, soil pH, moisture, organic carbon (SOC) and nitrogen contents are the most relevant (Fierer 2017; Bahram et al. 2018; Kuypers et al. 2018; Raniolo et al. 2023). Among the latter, slope can impact on microbial communities, for example by allowing the leaching of nutrients and cells through water flows (Unc and Goss 2004; Yang and van Elsas 2018), and altitude has been found to be positively correlated with nosZ gene abundance (Yang et al. 2014).
Considering all the above background, we chose to investigate the molecular basis for the dynamics of nitrification and denitrification functions in European Alpine grazed seminatural grasslands with the quantitative PCR (qPCR) approach, by targeting the prokaryotic nifH, nirK, nosZ and amoA genes as functional indicators and the general 16S rDNA bacterial abundance as a reference value. We selected a study area in the eastern Italian Alps where grasslands have been historically managed by ‘summer farms’ (Zanella et al. 2010), i.e. temporary farms located at high elevations, where livestock is moved to graze during summer from the permanent farms of the valleys’ bottoms. This traditional seasonal transhumance is a still a widespread practice in Alpine regions (Bunce et al. 2004; Zanella et al. 2010; Sturaro et al. 2013), and summer farms with their managed grasslands contribute to local economies and to the delivering of provisioning ecosystem services, but also to the conservation of a unique environment that is recognised as a source of biodiversity and a primary provider of such services (Chemini and Rizzoli 2003; Yuan et al. 2016; Schirpke et al. 2017; Nota et al. 2020). In this context, the selected genes were expected to possibly have relationships with the characteristics of single pastures such as the topology, the local soil physical and chemical conditions, with the additional influence of grazing animals. We thus considered the effects of these multiple characteristics on the presence of these genes to verify the following hypotheses: (1) selected genes may vary as a function of the prevailing environmental features, as well as the physical presence of grazing livestock in high summer pastures, and (2) variations in gene copy numbers for nitrogen fixation, nitrification, and denitrification functions are expected to occur as a consequence of animal disturbance (i.e. vegetation grazing, nutrient enrichment, and trampling) within the short period of transhumance; and the applied techniques could be suited to reveal its extent.
Materials and methods
Study area and sampling design
The study was performed in 2019 in three Alpine summer farms (‘Juribello’, ‘Vallazza’ and ‘Venegiota’, Fig. 1), in the Natural Park ‘Parco Naturale Paneveggio Pale di San Martino’, in the Trento province, eastern Italian Alps (46°18′28″N 11°44′38″E) (Fig. 1). Here the climate is alpine (Tattoni et al. 2010) with rainy and fresh summers (mean June–September 2000–2021: precipitation 147.5 mm ± 48.05 mm s.d.; temperature 10.9°C ± 3.9°C s.d.) and cold and long winters (mean October–April 2000–2021: precipitation 132.8 mm ± 295.3 mm s.d.; temperature −1.1°C ± 5.5° s.d.C). The pasture areas of the summer farms (Table 1) range between 124 ha and 180 ha, and are characterised by similar altitudinal and slope gradients (mean altitude ranged from 1947 ± 114 to 2038 ± 146 m above sea level, and mean slope from 14.3° ± 7.9° to 17.1° ± 8.5°. These were calculated with a resolution of 25 m from the Digital Terrain Model of the Trento province (https://siat.provincia.tn.it/stem/), using QGIS (ver. 3.10.4 ‘A Coruña’). The sites differ in soil type (http://wms.pcn.minambiente.it/ogc?map=/ms_ogc/WMS_v1.3/Vettoriali/Carta_ecopedologica.map). Juribello is characterised by a Cambisol (IUSS Working Group WRB 2022), a soil at the beginning of horizon differentiation with small quantities of illuviated clay and organic carbon; Vallazza by an Umbrisol (IUSS Working Group WRB 2022), a soil with an accumulation of organic matter on the surface and a small moisture deficit; Venegiota by a Leptosol (IUSS Working Group WRB 2022), a thin soil with substantial internal drainage which can cause drought.
Location of three summer farms and respective sampling sites (coloured dots) in the ‘Parco Naturale Paneveggio Pale di San Martino’ in the Trento province, eastern Italian Alps. Top left: Juribello; top right: Vallazza; bottom left: Venegiota; bottom right: location in Italian Alps.
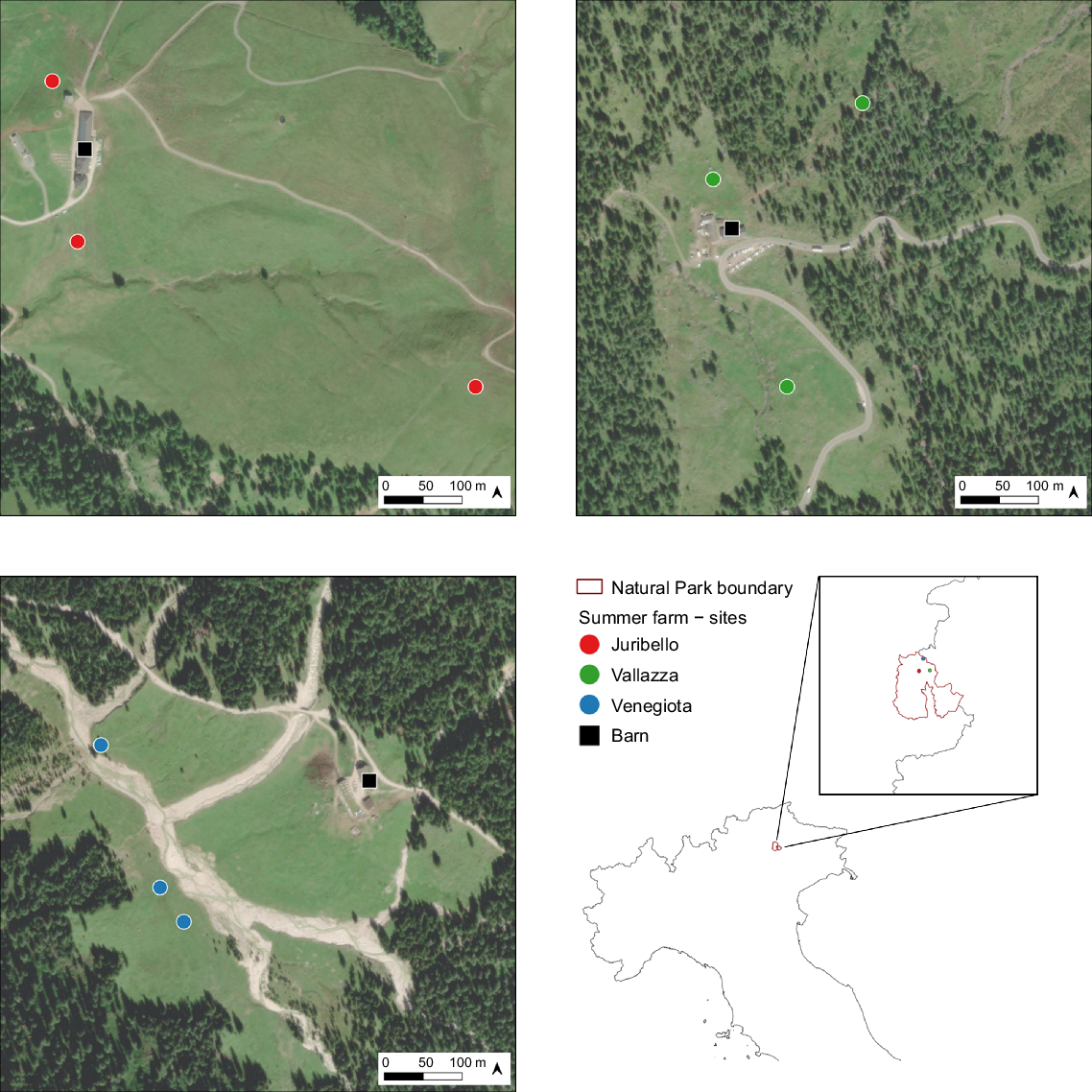
Summer farm | Grazed area (ha) | Slope (°) (mean ± s.d.) | Altitude (mean ± s.d.) | Soil | LU A | Stocking rate B | |
---|---|---|---|---|---|---|---|
Juribello | 180 | 14.3 ± 7.9 | 1950 ± 100 | Cambisol | 151 | 0.84 | |
Vallazza | 171 | 15.3 ± 7.9 | 2038 ± 146 | Umbrisol | 89 | 0.52 | |
Venegiota | 124 | 17.1 ± 8.5 | 1947 ± 114 | Leptosol | 78 | 0.63 |
These summer farms have a long history of continuous grazing (Zanella et al. 2010), and during the study period hosted herds of dairy cattle of mixed breeds (Brown Swiss, Simmental, Alpine Grey), with an extensive grazing management at low stocking rates (from 0.52 to 0.84 Livestock Units (LU)/ha; Table 1). Lactating cows composed most of the herds and were milked twice daily in the barn. Each day, after the morning milking, they were conducted by the shepherds to graze in a different section of the pasture area, where they were then left free until they returned to the barn for the evening milking. After that they were again released free to spend the night outdoors. During the milking, all cows received a concentrated supplement, a common practice in these systems (Zendri et al. 2016).
In each summer farm’s pasture area, we collected sample cores from the first 10 cm of soil (using a borer with a diameter of 1 cm) within three different georeferenced areas, selected according to interviews with farmers to represent sites with a high and constant presence of cows, but differing in altitude and slope. In each of the sampling areas, and before transhumance, we installed an exclusion cage of 1 m2 to prevent animals from grazing. Soil samples were collected in three replicates both within (‘ungrazed’) and outside (‘grazed’) the cages on four sampling times before (19 June 2019) and during (2, 16 July 2019 and 23 August 2019) the grazing period. Therefore, we collected 18 samples per summer farm at each sampling time, leading to a total of 216 samples at the end of the study period. In addition, soil depth was determined for each sampling site as an average of 5 probes using an auger of 1.2 m. The cumulative rainfall and the number of days with precipitation events were recorded over a 10-day period, starting from the sampling times, from the nearest weather stations to the study areas located at Passo Rolle (46°17′52.5″N; 11°47′13.6″E) and Passo Valles (46°20′18.2″N; 11°47′59.2″E).
Soil analyses
Before physicochemical analyses and DNA extraction, soil samples were air-dried at room temperature for 2 weeks (Wang et al. 2021), then ground by mortar and pestle and sieved through a 0.5 mm mesh to remove fine root portions and rocky debris. Drying was preferred to freezing as the latter method has been reported to alter DNA-based data as regards the Bacteroidetes phylum (Dorsaz et al. 2020), which can account for part of the denitrifier taxa. All analyses were expressed on an air-dried basis.
Chemical analyses
The analysed parameters included pH (Conyers and Davey 1988), electrical conductivity (EC, dS/cm) (Rhoades 1996) and cation exchange capacity (CEC, molc(+)/kg) estimated with a buffer solution of barium chloride and triethanolamine (Chapman 1965). Micronutrients (Cu and Fe) and extractable potassium (K ext, mg/kg) were determined with the Mehlich III method through inductively coupled plasma optical emission spectroscopy (Mehlich 1984); assimilable phosphorus (P_as, mg/kg) was estimated with the Olsen method (Olsen 1954). Total nitrogen (N_tot, % dry mass [DM]) and total C (C_tot, % DM) were determined with a VarioMacro combustion analyser (Elementar, Langenselbold, Germany), and total carbon (C_tot, % DM) was determined with an automatic Skalar Primacs SNC-100 analyser. The organic fraction of carbon (C_org, % DM) was calculated by subtracting from C_tot the inorganic fraction of carbon (C_ing, % DM) lost upon heating at 550°C, and the carbon to nnitrogen ratio (C_org/N_tot) was calculated as an index of available itrogen (Wang and Hou 2018; Risch et al. 2019).
Microbial genes analyses
The total soil DNA was extracted from 0.25 g of dried soil using the Qiagen DNeasy PowerSoil Pro Kit (Qiagen, Hilden, Germany) as described by the manufacturer’s protocol. The extracted DNA was quantified with a Qubit 3.0 fluorometer (Thermo Fisher Scientific, Carlsbad, CA) using the Qubit™ DNA HS Assay Kit (Thermo Fisher Scientific, Carlsbad, CA) and stored at −20°C.
To analyse the purified DNA, qPCR amplifications were carried out to quantify multiple target genes: 16S, nifH, nirK, nosZ, archaeal amoA (AOA) and eubacterial amoA (AOB). The primers used are shown in Table 2. A QuantStudio 5 System (Thermo Fisher Scientific, Carlsbad, CA) was used to perform the qPCR analyses in a final reaction volume of 5 μL, composed of 1 μL of template DNA and 4 μL of reaction mix. The mix contained 1.2 μL PCR-grade water, 0.15 μL each of Forward and Reverse primers (Table 2) and 2.5 μL Power SYBR Green PCR Master Mix (Thermo Fisher Scientific, Carlsbad, CA), which includes the Taq polymerase. The thermal conditions for each qPCR corresponded to a predenaturing stage at 95°C for 10 min, followed by 40 cycles with denaturation step at 95°C for 15 s, annealing step at 57°C for 60 s and extension at 72°C for 60 s. A no template control (NTC) of ultrapure PCR-grade water was run for each amplification in three technical replicates. The threshold cycle (Ct) values were transformed into gene copies using the equation by Dong et al. (2020), considering undetermined Ct equal or greater than 40 to equate to 0 genes copies by the transformation.
Primer | Function | Sequence | Amplicon length | References | |
---|---|---|---|---|---|
16S F | – | ATGGYTGTCGTCAGCTCGTG | 1550 bp | Johnson et al. (2016) | |
16S R | – | GGGTTGCGCTCGTTGC | |||
Archaeal amoA – AOA F | Ammonia oxidation | STAATGGTCTGGCTTAGACG | 635 bp | Francis et al. (2005) | |
Archaeal amoA – AOA R | Ammonia oxidation | GCGGCCATCCATCTGTATGT | |||
Bacterial amoA – AOB F | Ammonia oxidation | GGGGTTTCTACTGGTGGT | 500 bp | Rotthauwe et al. (1997) | |
Bacterial amoA – AOB R | Ammonia oxidation | CCCCTCKGSAAAGCCTTCTTC | |||
nifH F | Nitrogen fixation | AAAGGYGGWATCGGYAARTCCACCAC | 432 bp | Rösch et al. (2002) | |
nifH R | Nitrogen fixation | TTGTTSGCSGCRTACATSGCCATCAT | |||
nosZ F | Nitrous oxide reduction | CGYTGTTCMTCGACAGCCAG | 706 bp | Rösch et al. (2002) | |
nosZ R | Nitrous oxide reduction | CATGTGCAGNGCRTGGCAGAA | |||
nirK F | Nitrite reduction | ATYGGCGGVCAYGGCGA | 160 bp | Henry et al. (2004) | |
nirK R | Nitrite reduction | RGCCTCGATCAGRTTRTGGTT |
Statistical analysis
We conducted all analyses in R 4.2.0 (R Core Team 2016) with the ‘vegan’ (Dixon 2003), ‘factoextra’ (Kassambara and Mundt 2017) and ‘lmperm’ (Bates et al. 2020) libraries. All the soil physicochemical parameters were standardised as z-scores. We first examined the correlations between these parameters and the genes abundances with the Spearman coefficient for nonparametric distributions (Legendre and Legendre 2012). Further, we used a clustering approach to identify associations of levels of soil physicochemical variables, after checking for clustering tendency with the Hopkins’ statistic testing spatial randomness of the data (Banerjee and Davé 2004). We applied hierarchical clustering using the Ward method on the dissimilarity matrix of sites, computed considering all soil parameters; we verified the optimal number of clusters with the silhouette method (Rousseeuw 1987). The obtained clusters were used as categorical fixed effects in the subsequent analysis of factors affecting gene abundance. The use of the term cluster hereafter refers to such hierarchically coherent sets of soil properties. Gene abundances were analysed after log transformation, including Kendall’s correlations and ANOVA based on permutation test after log transformation to base 10. The correlation analysis was performed separately for genes and for pedological conditions, as well as for both together. The permutation test-based ANOVA was performed using the ‘aovp’ function from the ‘lmPerm’ library (Wheeler and Torchiano 2010) as a function of ‘sampling time’ (T1, T2, T3, and T4), ‘cluster’ (Ju, Va, and Ve; the previously identified clusters of soil chemical and physical properties), ‘grazing’ (grazed – Yes vs not grazed – No), and the two-way interactions between ‘cluster’ and ‘grazing’. The significant differences among levels within single factors were tested by posthoc test using the ‘pairwisePermutationTest’ function from the ‘rcompanion’ library (Mangiafico and Mangiafico 2017).
Results
Cluster analysis
The hierarchical clustering presented three clusters (Fig. 2a) according to the optimal number of clusters among sampling sites estimated with the Silhouette method (Supplementary Fig. S1). The dendrogram of hierarchical clustering (Fig. 2a) indicated that each cluster coincided with a different summer farm, with a certain similarity between the clusters Venegiota and Juribello in terms of soil conditions, whereas Vallazza was the most isolated. The profiles of the clusters (Fig. 2b) showed clear differences in terms of chemical soil properties, especially for pH, EC, CEC, macronutrients (N, C, and P), and micronutrients (Fe and Cu). pH, EC, and CEC presented the highest values in Venegiota, intermediate in Juribello, and the lowest in Vallazza. The macronutrients showed similar concentrations between Venegiota and Juribello, but not with Vallazza, which presented the highest values. A similarity between macronutrients between Venegiota and Juribello was also confirmed for the C/N ratio but not for the C/P, which had similar values between Juribello and Vallazza. Regarding the micronutrients, Cu showed a different concentration among the clusters with the highest values in Juribello and the lowest in Vallazza, whereas Fe presented the highest in Juribello but the lowest in Venegiota. Additionally, in terms of morphological characteristics (slope and altitude), the clusters showed clear differences with the minimum values for Venegiota and the maximum values for Juribello and Vallazza (Fig. 2b).
(a) Dendrogram of hierarchical clustering based on the soil chemical variables of the three pastures (J, Juribello pasture; V, Venegiota pasture; Z, Vallazza pasture). (b) boxplots of the corresponding soil variables for the three sites (boxplot whiskers extend to 1.5 times the interquartile range from the quartiles).
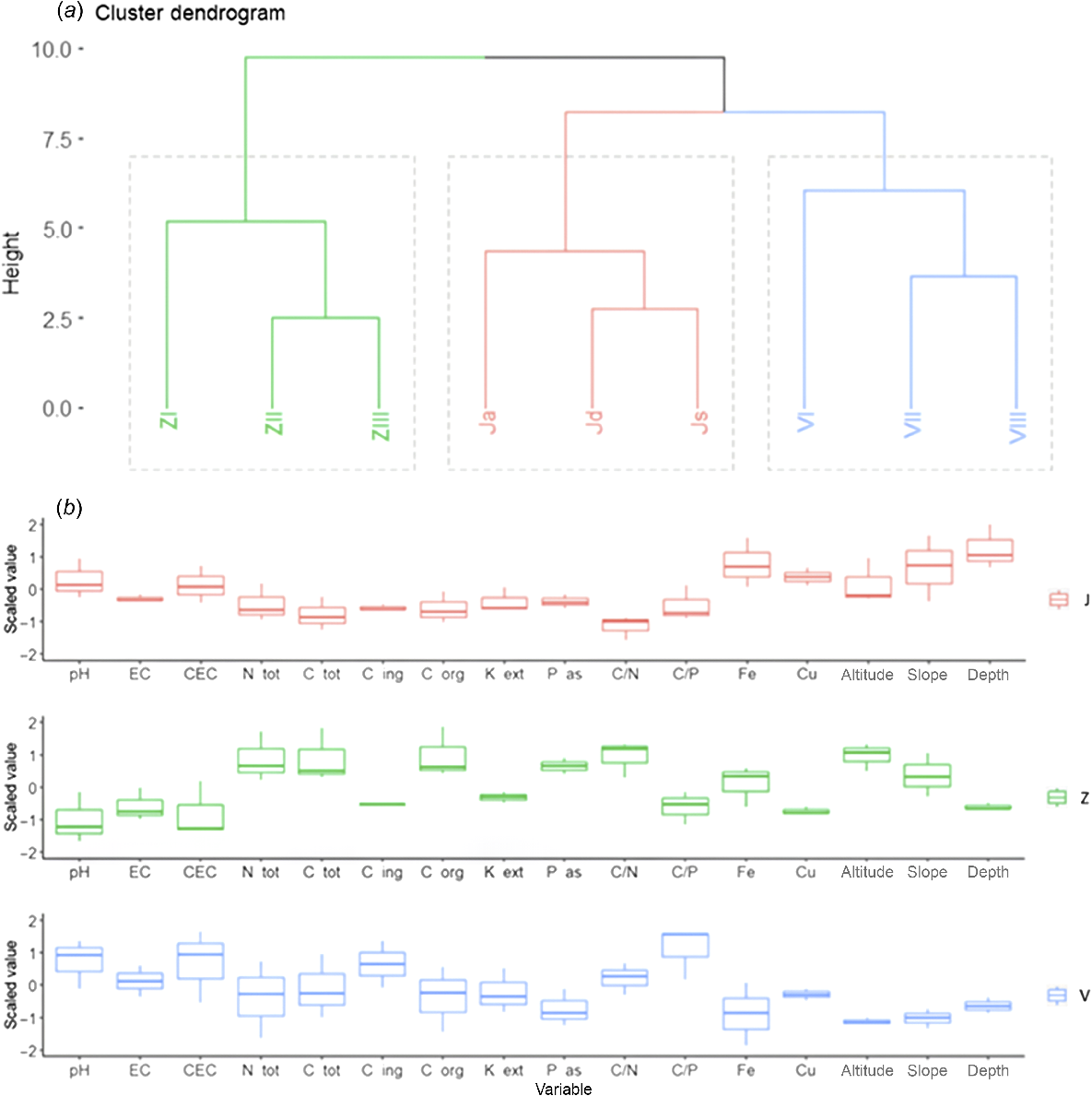
Soil physicochemical parameters
The correlation analysis among the chemical and physical variables (Fig. S2) revealed that CEC and EC, and N_tot and C_org, were basically equivalent to substitutes of each other (ρ = 0.93). Other remarkable positive correlations (ρ ranging from 0.5 to 0.73) were found for K_ext and P_as with N_tot and C_org, and for slope with altitude and depth. The pH showed remarkable negative correlations with P_as, N_tot, C_org and altitude (ρ ranging from −0.75 to −0.6). Altitude was also negatively correlated with EC and C_ing. The genes showed multiple significant correlations with soil parameters, (Fig. 3) except nifH whose only significance was with total N, that is consistent with its expected ecological service. The16S gene was strongly and positively significantly correlated with N_tot, C_tot, C_org, K_ext, P_as, Fe; and negatively with C/P. The nirK gene was significantly correlated with EC, CEC and K_ext. The nosZ gene was strongly significantly correlated with EC and CEC. The amoA AOB gene was strongly and positively correlated with Cu, which is in line with the role of this cation as cofactor for the ammonia oxidase enzyme.
The rainfall distribution across the four sampled periods is shown in Table 3. This is a factor whose intensity and frequency can affect soil redox potential as a function of its moisture content, and in turn have influence on the oxidative (nitrification) or reductive (denitrification, nitrogen fixation) metabolisms whose underlying genes are analysed.
Effect of pasture site, grazing, and sampling time on gene abundance
The permutation test-based ANOVA results are presented in Table S1. The abundance of 16S was not significantly affected by any factor. The abundances of nifH and nirK were significantly affected by the period (nifH: P < 0.001; nirK: P < 0.05), where T3 showed a significant decrease compared to the other times. The abundance of nosZ was significantly affected by the presence of grazing (P < 0.05) and by pasture area (P < 0.01), where Vallazza was significantly different from both Juribello and Venegiota. Neither amoA AOA nor amoA AOB were significantly affected by any factors but presented high variability. The abundance data for the six genes analysed, with respect to presence or absence of animal grazing, pasture area and sampling time are shown in Figs 4 and 5.
Boxplots showing log abundance of the number of copies per gram of soil dry weight for the following genes: 16S rDNA (a–c), nifH (d–f) and nirK (g–i) in relation to grazing (a, d, g), pasture area (b, e, h), and sampling time (c, f, i). Boxes sharing the same letter are not significantly different for P < 0.05.
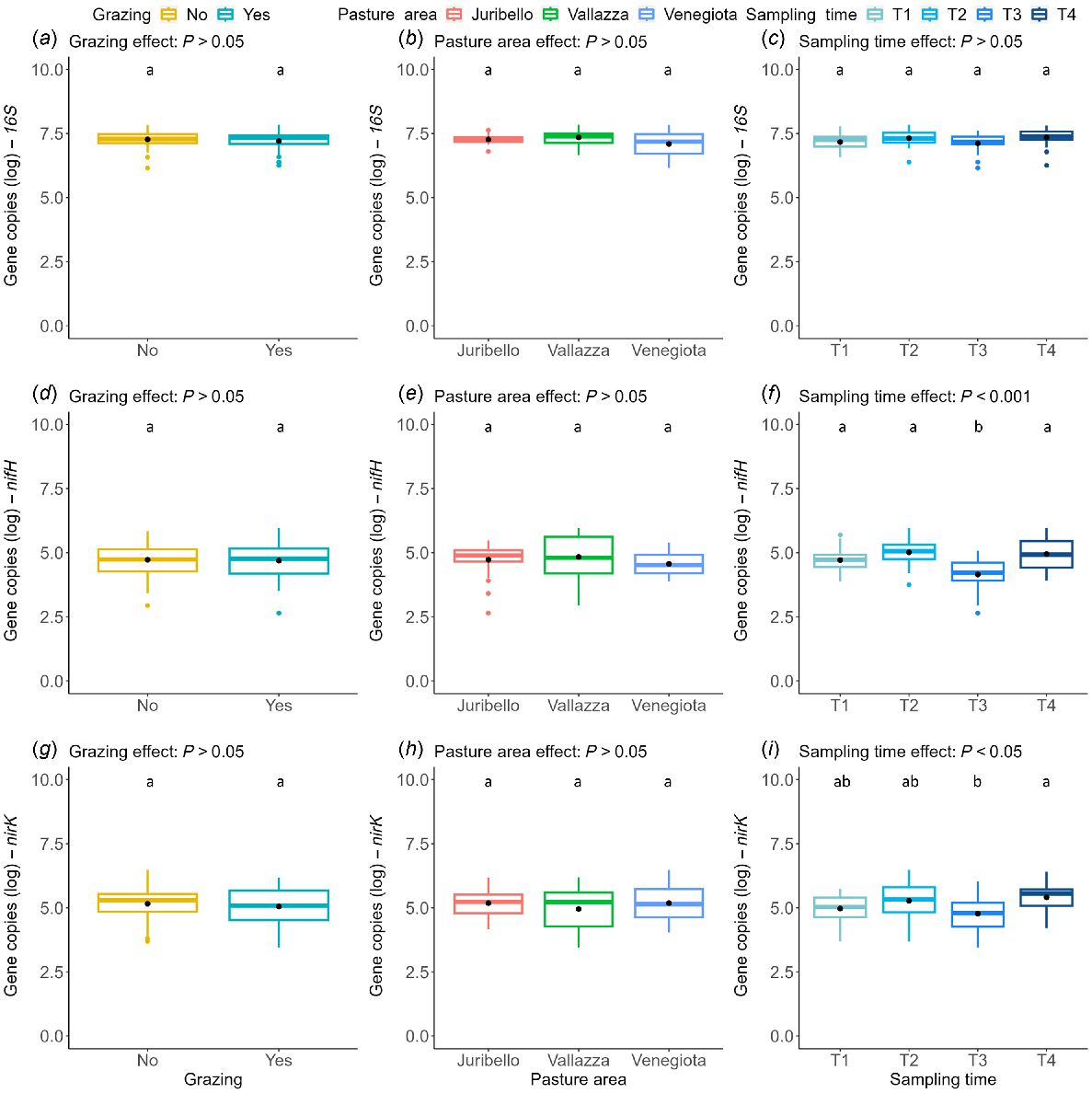
Boxplots showing log abundance of the number of copies per gram of soil dry weight for the following genes: nosZ (a–c), amoA AOA (d–f) and amoA AOB (g–i) in relation to grazing (a, d, g), pasture area (b, e, h), and sampling time (c, f, i). Boxes sharing the same letter are not significantly different for P < 0.05.
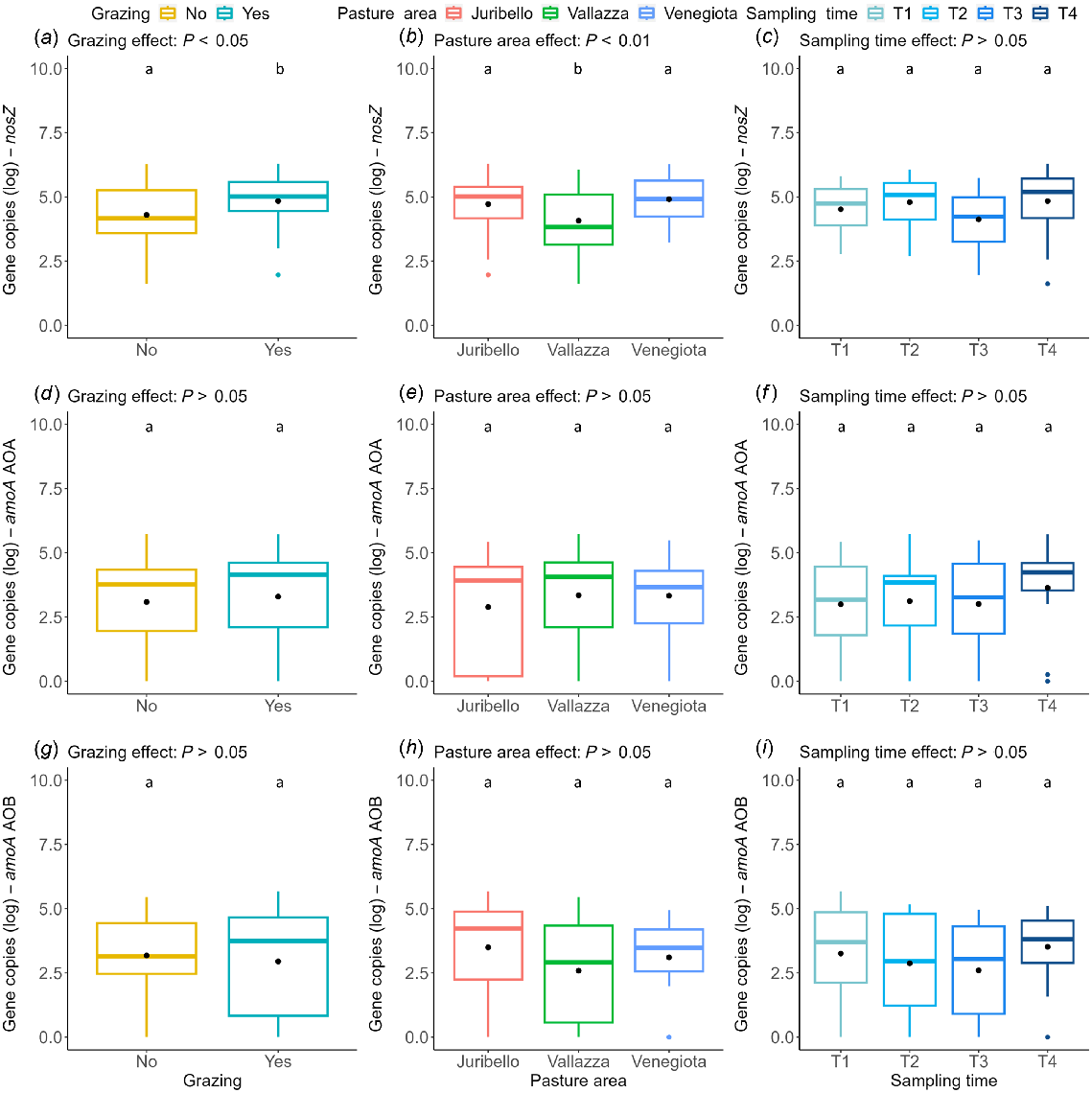
The Least Significant Difference and the significance values for the data presented in Figs 4 and 5 are tabulated in Table 4.
Comparison | Log 16S | Log nirK | Log nosZ | Log nifH | Log amoAb | Log amoAa | |||||||
---|---|---|---|---|---|---|---|---|---|---|---|---|---|
Sampling time | Stat | P value | Stat | P value | Stat | P value | Stat | P value | Stat | P value | Stat | P value | |
T1 – T2 | −1.26 | 0.21n.s. | −1.34 | 0.18n.s. | −0.89 | 0.37n.s. | −1.61 | 0.11n.s. | 0.59 | 0.55n.s. | −0.22 | 0.83n.s. | |
T1 – T3 | 0.46 | 0.64n.s. | 0.97 | 0.33n.s. | 1.18 | 0.24n.s. | 2.52 | 0.01* | 1.04 | 0.30n.s. | −0.02 | 0.98n.s. | |
T1 – T4 | −1.47 | 0.14n.s. | −2.19 | 0.03* | −0.88 | 0.38n.s. | −1.24 | 0.22n.s. | −0.47 | 0.64n.s. | −1.12 | 0.26n.s. | |
T2 – T3 | 1.56 | 0.12n.s. | 1.97 | 0.05 . | 1.91 | 0.06 . | 3.40 | 0.001*** | 0.43 | 0.66n.s. | 0.18 | 0.85n.s. | |
T2 –T 4 | −0.23 | 0.82n.s. | −0.59 | 0.55n.s. | −0.12 | 0.90n.s. | 0.34 | 0.74n.s. | −1.14 | 0.25n.s. | −0.91 | 0.36n.s. | |
T3 – T4 | −1.74 | 0.08n.s. | −2.72 | 0.01* | −1.78 | 0.07n.s. | −3.15 | 0.002** | −1.66 | 0.10n.s. | −1.03 | 0.30n.s. | |
Pasture cluster | |||||||||||||
Juribello – Vallazza | −1.09 | 0.28n.s. | 1.16 | 0.25n.s. | 1.96 | 0.05 . | −0.48 | 0.63n.s. | 1.62 | 0.11n.s. | −0.85 | 0.40n.s. | |
Juribello – Venegiota | 1.55 | 0.12n.s. | 0.00 | 1.00n.s. | −0.73 | 0.47n.s. | 1.01 | 0.31n.s. | 0.81 | 0.42n.s. | −0.87 | 0.38n.s. | |
Vallazza – Venegiota | 1.99 | 0.05 . | −1.05 | 0.29n.s. | −2.61 | 0.01* | 1.35 | 0.18n.s. | −1.03 | 0.30n.s. | 0.04 | 0.97n.s. | |
Grazing presence | |||||||||||||
No – Yes | 0.75 | 0.46n.s. | 0.67 | 0.50n.s. | −2.15 | 0.03* | 0.23 | 0.82n.s. | 0.54 | 0.59n.s. | −0.51 | 0.61n.s. |
n.s. P > 0.05, P ≈ 0.05, *P < 0.05, **P < 0.01, ***P < 0.001.
Discussion
We found significant effects despite the relatively low number of samples, the small control areas (i.e. exclusion cages), and the short period available because of the transhumance cycle. The general topsoil communities, marked by the 16S gene, were constant among pastures, periods, and presence of grazing (Fig. 4a–c), showing a moderate positive relation with all considered nutrients (C org, N tot, K ext, P as, Fig. 3). The diazotrophs, marked by the nifH gene, were only affected by time period (Fig. 4f). The denitrifiers, represented by nirK and nosZ genes, were the most affected guild, with different responses in relation to the time period, pasture areas and presence of grazing (Figs 4i and 5a, b). The nitrifiers (amoA genes) showed the largest variability in terms of presence and abundance (Fig. 5d–f), in agreement with other studies (Colloff et al. 2008; Wakelin et al. 2009; Lindsay et al. 2010; Zhou et al. 2022). Only one gene, nosZ encoding the denitrification endpoint enzyme, was sensitive enough to respond to the exclusion of grazing, which qualified as a strong factor in spite of the likely suboptimal size of the exclusion surfaces and of the nutrient runoff/leaching that inevitably the slope of the mountain terrain makes impossible to avoid. Indeed, although in theory the availability of 10 m × 10 m areas and possibly with no grazed zone on the uphill sides, could have offered a more robust setup, that would have been however, rather difficult to arrange. However, having observed for the most sensitive and responsive gene nosZ, a significant difference between the grazed and ungrazed blocks (Fig. 5, top row, left panel), which stood out in a very evident manner across all the three sites, we can state that the grazing variable appears to have a major role in shaping the abundance of denitrifiers.
The nosZ was the only gene that showed a significant difference as a function of grazing presence, with an increase in grazed areas. This gene encodes for nitrous oxide reductase, the final enzyme in the denitrification pathway, suggesting that in grazed areas the complete denitrification pathway can be enhanced. This increase in nosZ can be attributed to the local enrichment of nutrients reintroduced by grazing animals, which can support nitrification and then denitrification processes (Zhu et al. 2020; Zhang et al. 2021). Also, trampling by heavy animals such as dairy cattle can compact the soil, decreasing air permeability and hydraulic conductivity; thus creating a more anaerobic environment, which may stimulate denitrification (Brůček et al. 2009; Zhong et al. 2015). The nosZ variability as a function of grazing presence could be also related to the different development of local vegetation inside the exclusion cage, where plants presented a consistent and undisturbed cover as they were not consumed by animals. Thus, our results seem to contrast the positive relation of nosZ gene with perennial plant cover in agroecosystems found by Morales et al. (2010). Plant consumption by herbivores can induce changes in the availability of soil nutrients, facilitating a rapid N turnover. Effects on total denitrification may be due to a diminished assimilation of nitrogen by plants (Uchida et al. 2008). Thus, plants not affected by animal feeding may assimilate more nitrogen to support continual aboveground development (Wang et al. 2017); whereas the ones enduring consumption can invest more in the root system, stimulating the local microbial communities more (López-Mársico et al. 2015; Chung and Rudgers 2016; Yan et al. 2021). Moreover, the nosZ showed a significant decrease in the Vallazza cluster compared to the others, despite its weak correlations with pedological variables. The difference could be attributed to the different animal load, which is the minimum in Vallazza, and/or by vegetation, which influenced the nosZ gene according to other studies (Wang et al. 2017; Xu et al. 2019). The Vallazza cluster presented the lowest value of pH, which is one of the most important drivers for denitrification (Jones et al. 2014), and a different, suitably adapted vegetation compared to the other two clusters. Those differences, combining with the low animal loads, can induce more an intermediate denitrification than a total denitrification.
In general, the analysed genes did not show significant differences between the end and the beginning of the summer, except nifH and nirK, which showed significant decreases during the third period followed by an increase in the last one. Also, the other genes showed a weak decrease during the third period, but it was not significant due to their variability. These differences may reveal a peculiar dynamic of the nitrogen cycle in Alpine grasslands as a function of local availability of chemical substrates. The decrease of nifH and nirK during the second half of summer may be due to vegetative sprouting, which can affect the availability of nutrients for microbial communities, as the dynamics of the N cycle appear tied to vegetation phenology (Liu et al. 2016; Song et al. 2019). During early summer, the vegetation uptake due to its growth may reduce the amount of total available nitrogen for microorganisms, especially in the rhizosphere (Kuzyakov and Xu 2013). In late summer, the availability of nitrogen can increase thanks to enrichment of the litter layer from plant remains (Chen et al. 2014) and the accumulation of animal excreta (Bilotta et al. 2007), despite the increase of plant–microorganism competition due to the complete development of the root system (Liu et al. 2016). Thus, the reduction of nifH during the second half of summer may be due to the increase of ammonium from plant litter and animal dung, which can inhibit the N fixation with a decrease of nifH, according with the reports of Pereira e Silva et al. (2013) and Regan et al. (2017). The decrease of nirK during the third period may be due to an increase of nitrate consumption by the plants, which can also influence marginally the total denitrification according with the negative trend of nosZ during the same period. The rainfall distribution over the months of animal presence in the sites showed that the third period was the one with the lowest cumulative rainfall (Table 3). This difference is strongly supportive of an explanation of the behaviour of the only two genes (nifH and nirK) that displayed a diminished number of copies exactly and only in that period (Fig. 4f, i, respectively). This observation is concordant with the fact that the encoded functions are both reductions, and a lower level of soil moisture is associated with more aerated states. However nosZ, in spite of also encoding a reduction (as nitrous oxide reductase), was by contrast not significantly diminished. This can be interpreted in relation to this enzyme’s reaction, which has a lower electronegative redox potential when compared to that of the nitrite reductase nirK, located upstream on the same pathway.
As mentioned, except for nosZ, the study revealed the absence of significant effects of grazing for all the other genes (16S, nifH, nirK, amoA AOB and amoA AOA), indicating a possible resistance to changes of the microbial community that would require longer periods to start showing differences. In other words, a single year of exclusion could not be enough to reverse the effect of a century-old pasture use. What is meant here is that a practice might need to be applied for periods that are longer than those afforded by experimental campaigns, in which variables start to be manipulated on purpose for a duration of few years. Soil conditions in these historically established pastures, as Juribello, Vallazza and Venegiota, could be actually slower to modify than those occurring in other more recently established agroecosystems. In addition, the long adaptation to cyclic, transhumant management may have shaped the microbial functional community favouring a relevant resistance with respect to grazing conditions through a long-term ‘soil memory’ of the biotic component. With respect to this theory, grazing can contribute to reaching and maintaining ecosystem stability in pastures with historical presence of animals (Beneduzi et al. 2019). This agrees also with the Intermediate Disturbance Hypothesis (Connell 1979), where the summer grazing of animals can be considered a temporal disturbance needed to maintain the variety of ecological niches and thus the ecosystem equilibrium (Beneduzi et al. 2019).
The absence of significant effect of identified hierarchical clusters of features for almost all genes may be tied to geographical proximity, which may facilitate the phenomenon of dispersion of microorganisms increasing the homogeneity between communities (Albright et al. 2018; Louca 2022), also in terms of genes. In these terms, the historical use of grassland as pastures may shape the microbial communities supporting the dispersion through the passage of animals with the consequent decrease in the differences among close areas, such as our clusters. Despite this possible homogenisation of microbial communities, the pastures of the three summer farms had distinct physicochemical and morphological identities, as the cluster analysis revealed. The similarity between Juribello and Venegiota clusters and the difference of Vallazza agree with their uneven geographical proximities. These dissimilarities among sites were also supported by the correlation analysis among soil properties (Fig. S2), and essentially they were consistent only with the trend of nosZ abundances, which were significantly affected by pasture areas despite their weak correlations with pedological conditions, except with EC and CEC. The other genes were not affected by the site (farms) according to their weak to moderate correlations with pedological conditions. In terms of correlations, only 16S showed significant moderate and positive correlations with nutrients, both macro and micro. These correlations may reveal the positive contribution of multiple nutrients’ availability on microbial community diversity and composition, as multiple soil nutrients may support microorganisms with different metabolisms and niche specialisation (Fan et al. 2014; Zhang et al. 2021). As regards correlation among genes, we found significant but weak correlations between only nosZ and amoA AOB (data not shown). Furthermore, we found a moderate correlation (0.4) between nirK and nifH, the gene responsible for nitrogen fixation, a process able to increase ammonium levels in the soil. This correlation could be expected, as nitrogen fixation is one way to introduce ammonium,supporting nitrification and then denitrification processes. However, this type of analysis involving pairwise correlations between genes may be sensitive to effects of generalised increase or decrease of the whole bacterial community without meaningful nexuses. Concerning chemical variables, we found a weak positive significance linking Fe and Cu, two of the main cofactors of our target gene enzymes (Pauleta et al. 2013; Musiani et al. 2020). These correlations suggest a possible greater sensitivity of these two genes to the concentrations of micronutrients than in the rest of selected genes.
Coming to an attempt to draw applied information from all the above data and to extract the connections that appear most supportive to interpret the data and to formulate advice for the actual managers of the pastures, the following two lines of rationale can be proposed.
The Vallazza pasture site, as mentioned, was the one adopting the lowest animal stocking rate among the three farms (Table 1) and has significantly fewer gene copies of the terminal denitrification (N2O-detoxifying) nosZ gene (Fig. 4 top row, middle panel), which is also the gene most negatively correlated with C/N ratio (Fig. 3). The effect is independently confirmed by the significant reduction of nosZ gene copies across all farms, in relation to grazing exclusion under the caged areas (Fig. 5, top row, left panel). On the applied side of the research output, a practical use of this significant result is to recommend farmers managing the pastures to attain a stocking rate of >0.6 livestock units per hectare. The aim is to ensure sufficient nitrogen from the animals’ excretions to ultimately serve as an electron acceptor throughout the complete true denitrification pathway. This solution represents a suitable compromise to reconcile animal production in mountain areas with minimising the downsides of denitrification, as it will mitigate the premature gaseous emissions of nitrous oxide. A second applied deliverable, in the form of a recommendable practice for pasture farming, is derived from the effect of sampling time. In periods accumulating <22 mm of rain in the preceding 10 day time window, the risk of denitrification-linked (nitric oxide and nitrous oxide) emissions is mitigated (there are reduced amounts of nitrite reductase and unaffected levels of nitrous oxide reductase) and thus a higher livestock grazing intensity can be allowed as the ecosystem will tolerate the extra pressure.
Conclusions
In this work, we indirectly examined the potentialities for different stages of the nitrogen cycle of alpine grasslands historically used as pastures, using various target genes as indicators. Overall, in most cases the quantified genes displayed a moderate variability, which suggests the prevalence of a homeostatic maintenance of an ecosystem equilibrium. Grazing presence did not have a significant effect on most of the targeted genes, indicating a possible resistance of the microbial community to long-term grazing disturbances. However, significant variations during the presence of livestock were found in genes for nitrogen fixation (nifH) and intermediate denitrification (nirK) among periods, suggesting temporal dynamics of these processes that could be interpreted in relation to local vegetation phenology, nutrient availability, and rainfall. The last factor was actually supported by the precipitation data as coinciding with the differences as discussed above. The terminal denitrification determinant (nosZ) was the most affected proxy, with significant variations as a function of hierarchically clustered soil properties, despite its low correlations with single pedological parameters, and a significant increase in grazed areas. This increase confirmed the direct effects of soil compaction and nutrient enrichment linked to animal presence on the full denitrification pathway representation in pastures. Conversely, no significant differences for the nitrification genes were found despite the high variability for both amoA AOA and amoA AOB.
Two take home lessons and ensuing recommended practices for mountain pasture management stem from these data: first, a stocking rate higher than 0.6 LU/ha appears compatible with the compromise of mountain productivity at the same time as fostering the presence of bacterial guilds that can reduce nitrogen oxides GHG emissions. Second, the fact that nitrite reductase turned out to be more oxygen-sensitive than nitrous oxide reductase, as the former was reduced in the driest period, permits an increase of the stocking rate when the soil has received less than 22 mm of rain in the prior 10 day period.
We underscore the need for further research to explore the effects of different vegetation types and their phenology and a gradient of animal stocking rates, obtained through the integration of GPS tracking. The characterisation of ecosystem functions of soil microbial community, such as N cycling potentials, may be fundamental to reach a real sustainable management and improve the conservation of alpine grasslands.
Data availability
The datasets generated during and/or analysed during the current study are available from the corresponding author on reasonable request.
Declaration of funding
The research was supported by BIRD PROJECT ‘Relationships between livestock grazing, soil microbiology and nutrient cycles in different agroecosystems’ (University of Padova, grant number 199773/19). The grant of Salvatore Raniolo was funded by the PNRR research activities of the consortium iNEST (Interconnected North-Est Innovation Ecosystem) funded by the European Union Next-GenerationEU (Piano Nazionale di Ripresa e Resilienza (PNRR) – Missione 4 Componente 2, Investimento 1.5 – D.D. 1058 23/06/2022, ECS_00000043).
Author contributions
All authors contributed to the study conception and design. Material preparation, data collection and analysis were performed by Salvatore Raniolo and Laura Maretto. The first draft of the manuscript was written by Salvatore Raniolo, Andrea Squartini, Maurizio Ramanzin, Enrico Sturaro, Piergiorgio Stevanato and Giuseppe Concheri, and all authors commented on previous versions of the manuscript. All authors read and approved the final manuscript.
Acknowledgements
We thank all the farmers involved during the sampling for their availability; and the Breeders Association of Trento and the Parco Naturale Paneveggio Pale di San Martino for their support.
References
Albright MBN, Johansen R, Lopez D, Gallegos-Graves LV, Steven B, Kuske CR, Dunbar J (2018) Short-term transcriptional response of microbial communities to nitrogen fertilization in a pine forest soil. Applied and Environmental Microbiology 84(15), e00598–18.
| Crossref | Google Scholar | PubMed |
Ali I, Cawkwell F, Dwyer E, Barrett B, Green S (2016) Satellite remote sensing of grasslands: from observation to management. Journal of Plant Ecology 9, 649-671.
| Crossref | Google Scholar |
Andrade-Linares DR, Zistl-Schlingmann M, Foesel B, Dannenmann M, Schulz S, Schloter M (2021) Short term effects of climate change and intensification of management on the abundance of microbes driving nitrogen turnover in montane grassland soils. Science of The Total Environment 780, 146672.
| Crossref | Google Scholar |
Bahram M, Hildebrand F, Forslund SK, Anderson JL, Soudzilovskaia NA, Bodegom PM, Bengtsson-Palme J, Anslan S, Coelho LP, Harend H, Huerta-Cepas J, Medema MH, Maltz MR, Mundra S, Olsson PA, Pent M, Põlme S, Sunagawa S, Ryberg M, Tedersoo L, Bork P (2018) Structure and function of the global topsoil microbiome. Nature 560, 233-237.
| Crossref | Google Scholar | PubMed |
Banerjee A, Davé RN (2004) Validating clusters using the Hopkins statistic. In ‘Institute of Electrical and Electronics Engineers International Conference on Fuzzy Systems. Vol. 1’. pp. 149–153. (IEEE) Available at https://doi.org/10.1109/FUZZY.2004.1375706
Bardgett RD, Wardle DA (2003) Herbivore-mediated linkages between aboveground and belowground communities. Ecology 84, 2258-2268.
| Crossref | Google Scholar |
Bates D, Maechler M, Bolker B, Walker S, Christensen RHB, Singmann H, Dai B, Scheipl F, Grothendieck G, Green P, Fox J (2020) Linear mixed-effects model using “Eigen” and S4, R Package Version 1.1-23. Available at https://github.com/lme4/lme4/
Bell MJ, Cloy JM, Topp CFE, Ball BC, Bagnall A, Rees RM, Chadwick DR (2016) Quantifying N2O emissions from intensive grassland production: the role of synthetic fertilizer type, application rate, timing and nitrification inhibitors. The Journal of Agricultural Science 154, 812-827.
| Crossref | Google Scholar |
Beneduzi A, dos Anjos Borges LG, Alvarenga SM, Faoro H, de Souza EM, Vargas LK, Passaglia LMP (2019) Distinct grazing pressure loads generate different impacts on bacterial community in a long-term experiment in Pampa biome. Applied Soil Ecology 137, 167-177.
| Crossref | Google Scholar |
Beule L, Corre MD, Schmidt M, Göbel L, Veldkamp E, Karlovsky P (2019) Conversion of monoculture cropland and open grassland to agroforestry alters the abundance of soil bacteria, fungi and soil-N-cycling genes. PLoS ONE 14, e0218779.
| Crossref | Google Scholar | PubMed |
Bilotta GS, Brazier RE, Haygarth PM (2007) The impacts of grazing animals on the quality of soils, vegetation, and surface waters in intensively managed grasslands. In ‘Advances in agronomy. Vol. 94’. (Ed. DL Sparks) pp. 237–280. (Academic Press) doi:10.1016/S0065-2113(06)94006-1
Brůček P, Šimek M, Hynšt J (2009) Long-term animal impact modifies potential production of N2O from pasture soil. Biology and Fertility of Soils 46, 27-36.
| Crossref | Google Scholar |
Cavicchioli R, Ripple WJ, Timmis KN, Azam F, Bakken LR, Baylis M, Behrenfeld MJ, Boetius A, Boyd PW, Classen AT, Crowther TW, Danovaro R, Foreman CM, Huisman J, Hutchins DA, Jansson JK, Karl DM, Koskella B, Mark Welch DB, Martiny JBH, Moran MA, Orphan VJ, Reay DS, Remais JV, Rich VI, Singh BK, Stein LY, Stewart FJ, Sullivan MB, van Oppen MJH, Weaver SC, Webb EA, Webster NS (2019) Scientists’ warning to humanity: microorganisms and climate change. Nature Reviews Microbiology 17, 569-586.
| Crossref | Google Scholar | PubMed |
Chapman HD (1965) Cation-exchange capacity. In ‘Methods of soil analysis: Part 2. Chemical and microbiological properties. Vol. 9’. (Ed. AG Norman) pp. 891–901. (American Society of Agronomy) doi:10.2134/agronmonogr9.2.c6
Chemini C, Rizzoli A (2003) Land use change and biodiversity conservation in the Alps. Journal of Mountain Ecology 7, 1-7.
| Crossref | Google Scholar |
Chen B, Liu E, Tian Q, Yan C, Zhang Y (2014) Soil nitrogen dynamics and crop residues. A review. Agronomy for Sustainable Development 34, 429-442.
| Crossref | Google Scholar |
Chroňáková A, Radl V, Čuhel J, Šimek M, Elhottová D, Engel M, Schloter M (2009) Overwintering management on upland pasture causes shifts in an abundance of denitrifying microbial communities, their activity and N2O-reducing ability. Soil Biology and Biochemistry 41, 1132-1138.
| Crossref | Google Scholar |
Chung YA, Rudgers JA (2016) Plant–soil feedbacks promote negative frequency dependence in the coexistence of two aridland grasses. Proceedings of the Royal Society B: Biological Sciences 283(1835), 20160608.
| Crossref | Google Scholar |
Colloff MJ, Wakelin SA, Gomez D, Rogers SL (2008) Detection of nitrogen cycle genes in soils for measuring the effects of changes in land use and management. Soil Biology and Biochemistry 40, 1637-1645.
| Crossref | Google Scholar |
Connell JH (1979) Response: intermediate-disturbance hypothesis. Science 204(4399), 1345.
| Google Scholar |
Conyers MK, Davey BG (1988) Observations on some routine methods for soil pH determination. Soil Science 145(1), 29-36.
| Crossref | Google Scholar |
De Boer W, Kowalchuk GA (2001) Nitrification in acid soils: micro-organisms and mechanisms. Soil Biology and Biochemistry 33, 853-866.
| Crossref | Google Scholar |
Delgado-Baquerizo M, Maestre FT, Reich PB, Jeffries TC, Gaitan JJ, Encinar D, Berdugo M, Campbell CD, Singh BK (2016) Microbial diversity drives multifunctionality in terrestrial ecosystems. Nature Communications 7, 10541.
| Crossref | Google Scholar |
Di HJ, Cameron KC (2018) Ammonia oxidisers and their inhibition to reduce nitrogen losses in grazed grassland: a review. Journal of the Royal Society of New Zealand 48, 127-142.
| Crossref | Google Scholar |
Dixon P (2003) VEGAN, a package of R functions for community ecology. Journal of Vegetation Science 14(6), 927-930.
| Crossref | Google Scholar |
Dong S, Li Y, Ganjurjav H, Gao Q, Gao X, Zhang J, Yan Y, Zhang Y, Liu S, Hu G, Wang X, Wu H, Li S (2020) Grazing promoted soil microbial functional genes for regulating C and N cycling in alpine meadow of the Qinghai-Tibetan Plateau. Agriculture, Ecosystems & Environment 303, 107111.
| Crossref | Google Scholar |
Dorsaz S, Charretier Y, Girard M, Gaïa N, Leo S, Schrenzel J, Harbarth S, Huttner B, Lazarevic V (2020) Changes in microbiota profiles after prolonged frozen storage of stool suspensions. Frontiers in Cellular and Infection Microbiology 10, 77.
| Crossref | Google Scholar |
Du Y, Shu K, Guo X, Pengjin Z (2019) Moderate grazing promotes grassland nitrous oxide emission by increasing ammonia-oxidizing archaea abundance on the Tibetan Plateau. Current Microbiology 76, 620-625.
| Crossref | Google Scholar | PubMed |
Eichner MJ (1990) Nitrous oxide emissions from fertilized soils: summary of available data. Journal of Environmental Quality 19(2), 272-280.
| Crossref | Google Scholar |
Fan F, Yin C, Tang Y, Li Z, Song A, Wakelin SA, Zou J, Liang Y (2014) Probing potential microbial coupling of carbon and nitrogen cycling during decomposition of maize residue by 13C-DNA-SIP. Soil Biology and Biochemistry 70, 12-21.
| Crossref | Google Scholar |
Fierer N (2017) Embracing the unknown: disentangling the complexities of the soil microbiome. Nature Reviews Microbiology 15, 579-590.
| Crossref | Google Scholar | PubMed |
Francis CA, Roberts KJ, Beman JM, Santoro AE, Oakley BB (2005) Ubiquity and diversity of ammonia-oxidizing archaea in water columns and sediments of the ocean. Proceedings of the National Academy of Sciences USA 102, 14683-14688.
| Crossref | Google Scholar |
Hackl E, Zechmeister-Boltenstern S, Kandeler E (2000) Nitrogen dynamics in different types of pasture in the Austrian Alps. Biology and Fertility of Soils 32, 321-327.
| Crossref | Google Scholar |
Henry S, Baudoin E, López-Gutiérrez JC, Martin-Laurent F, Brauman A, Philippot L (2004) Quantification of denitrifying bacteria in soils by nirK gene targeted real-time PCR. Journal of Microbiological Methods 59, 327-335.
| Crossref | Google Scholar | PubMed |
Henry S, Bru D, Stres B, Hallet S, Philippot L (2006) Quantitative detection of the nosZ gene, encoding nitrous oxide reductase, and comparison of the abundances of 16S rRNA, narG, nirK, and nosZ genes in soils. Applied and Environmental Microbiology 72, 5181-5189.
| Crossref | Google Scholar | PubMed |
Hu H-W, Chen D, He J-Z (2015) Microbial regulation of terrestrial nitrous oxide formation: understanding the biological pathways for prediction of emission rates. FEMS Microbiology Reviews 39, 729-749.
| Crossref | Google Scholar | PubMed |
Jerrentrup JS, Klimek S, Marchiori E, Bittante G, Ramanzin M, Sturaro E, Marini L (2016) Impact of dairy farming on butterfly diversity in Alpine summer pastures. Agriculture, Ecosystems & Environment 232, 38-45.
| Crossref | Google Scholar |
Jia Z, Conrad R (2009) Bacteria rather than Archaea dominate microbial ammonia oxidation in an agricultural soil. Environmental Microbiology 11, 1658-1671.
| Crossref | Google Scholar | PubMed |
Johnson TA, Stedtfeld RD, Wang Q, Cole JR, Hashsham SA, Looft T, Zhu Y-G, Tiedje JM, Gillings M, Davies JE (2016) Clusters of antibiotic resistance genes enriched together stay together in swine agriculture. mBio 7(2),.
| Crossref | Google Scholar |
Jones CM, Spor A, Brennan FP, Breuil M-C, Bru D, Lemanceau P, Griffiths B, Hallin S, Philippot L (2014) Recently identified microbial guild mediates soil N2O sink capacity. Nature Climate Change 4, 801-805.
| Crossref | Google Scholar |
Kuypers MMM, Marchant HK, Kartal B (2018) The microbial nitrogen-cycling network. Nature Reviews Microbiology 16, 263-276.
| Crossref | Google Scholar | PubMed |
Kuzyakov Y, Xu X (2013) Competition between roots and microorganisms for nitrogen: mechanisms and ecological relevance. New Phytologist 198, 656-669.
| Crossref | Google Scholar |
Legendre P, Legendre L (2012) ‘Numerical ecology.’ 2nd edn. (Elsevier) https://doi.org/10.1017/CBO9781107415324.004
Li Y, Lin Q, Wang S, Li X, Liu W, Luo C, Zhang Z, Zhu X, Jiang L, Li X (2016) Soil bacterial community responses to warming and grazing in a Tibetan alpine meadow. FEMS Microbiology Ecology 92, fiv152.
| Crossref | Google Scholar |
Lindsay EA, Colloff MJ, Gibb NL, Wakelin SA (2010) The abundance of microbial functional genes in grassy woodlands is influenced more by soil nutrient enrichment than by recent weed invasion or livestock exclusion. Applied and Environmental Microbiology 76, 5547-5555.
| Crossref | Google Scholar | PubMed |
Liu Q, Qiao N, Xu X, Xin X, Han JY, Tian Y, Ouyang H, Kuzyakov Y (2016) Nitrogen acquisition by plants and microorganisms in a temperate grassland. Scientific Reports 6(1), 22642.
| Crossref | Google Scholar |
López-Aizpún M, Horrocks CA, Charteris AF, Marsden KA, Ciganda VS, Evans JR, Chadwick DR, Cárdenas LM (2020) Meta-analysis of global livestock urine-derived nitrous oxide emissions from agricultural soils. Global Change Biology 26, 2002-2013.
| Crossref | Google Scholar | PubMed |
López-Mársico L, Altesor A, Oyarzabal M, Baldassini P, Paruelo JM (2015) Grazing increases below-ground biomass and net primary production in a temperate grassland. Plant and Soil 392, 155-162.
| Google Scholar |
Louca S (2022) The rates of global bacterial and archaeal dispersal. The ISME Journal 16(1), 159-167.
| Google Scholar |
Louca S, Polz MF, Mazel F, Albright MBN, Huber JA, O’Connor MI, Ackermann M, Hahn AS, Srivastava DS, Crowe SA, Doebeli M, Parfrey LW (2018) Function and functional redundancy in microbial systems. Nature Ecology & Evolution 2, 936-943.
| Crossref | Google Scholar | PubMed |
Mangiafico S, Mangiafico MS (2017) Package ‘rcompanion’. Cran Repos 20, 1-71.
| Google Scholar |
Marini L, Fontana P, Klimek S, Battisti A, Gaston KJ (2009) Impact of farm size and topography on plant and insect diversity of managed grasslands in the Alps. Biological Conservation 142, 394-403.
| Crossref | Google Scholar |
Maron P-A, Sarr A, Kaisermann A, Lévêque J, Mathieu O, Guigue J, Karimi B, Bernard L, Dequiedt S, Terrat S, Chabbi A, Ranjard L (2018) High microbial diversity promotes soil ecosystem functioning. Applied and Environmental Microbiology 84, e02738-17.
| Crossref | Google Scholar |
McSherry ME, Ritchie ME (2013) Effects of grazing on grassland soil carbon: a global review. Global Change Biology 19, 1347-1357.
| Crossref | Google Scholar | PubMed |
Mehlich A (1984) Mehlich 3 soil test extractant: a modification of Mehlich 2 extractant. Communications in Soil Science and Plant Analysis 15, 37-41.
| Crossref | Google Scholar |
Mendes LW, Tsai SM, Navarrete AA, de Hollander M, van Veen JA, Kuramae EE (2015) Soil-borne microbiome: linking diversity to function. Microbial Ecology 70, 255-265.
| Crossref | Google Scholar | PubMed |
Moonen A-C, Bàrberi P (2008) Functional biodiversity: an agroecosystem approach. Agriculture, Ecosystems & Environment 127, 7-21.
| Crossref | Google Scholar |
Morales SE, Cosart T, Holben WE (2010) Bacterial gene abundances as indicators of greenhouse gas emission in soils. The ISME Journal 4, 799-808.
| Crossref | Google Scholar | PubMed |
Musiani F, Broll V, Evangelisti E, Ciurli S (2020) The model structure of the copper-dependent ammonia monooxygenase. Journal of Biological Inorganic Chemistry 25, 995-1007.
| Crossref | Google Scholar | PubMed |
NandaKafle G, Seale T, Flint T, Nepal M, Venter SN, Brözel VS (2017) Distribution of diverse Escherichia coli between cattle and pasture. Microbes and Environments 32, 226-233.
| Crossref | Google Scholar |
Nota G, Ravetto Enri S, Pittarello M, Gorlier A, Lombardi G, Lonati M (2020) Sheep grazing and wildfire: disturbance effects on dry grassland vegetation in the western italian alps. Agronomy 11, 6.
| Crossref | Google Scholar |
Orellana LH, Rodriguez-R LM, Higgins S, Chee-Sanford JC, Sanford RA, Ritalahti KM, Löffler FE, Konstantinidis KT (2014) Detecting nitrous oxide reductase (nosZ) genes in soil metagenomes: method development and implications for the nitrogen cycle. mBio 5,.
| Crossref | Google Scholar |
Pauleta SR, Dell’Acqua S, Moura I (2013) Nitrous oxide reductase. Coordination Chemistry Reviews 257(2), 332-349.
| Crossref | Google Scholar |
Pereira e Silva MC, Schloter-Hai B, Schloter M, van Elsas JD, Salles JF (2013) Temporal dynamics of abundance and composition of nitrogen-fixing communities across agricultural soils. PLoS ONE 8(9), e74500.
| Crossref | Google Scholar |
Pereira P, Bogunovic I, Muñoz-Rojas M, Brevik EC (2018) Soil ecosystem services, sustainability, valuation and management. Current Opinion in Environmental Science & Health 5, 7-13.
| Crossref | Google Scholar |
Qu T-B, Du W-C, Yuan X, Yang Z-M, Liu D-B, Wang D-L, Yu L-J (2016) Impacts of grazing intensity and plant community composition on soil bacterial community diversity in a steppe grassland. PLoS ONE 11, e0159680.
| Crossref | Google Scholar | PubMed |
Raniolo S, Maretto L, Benedetti del Rio E, Cournut S, Cremilleux M, Nowak B, Michaud A, Lind V, Concheri G, Stevanato P, Squartini A, Ramanzin M, Sturaro E (2023) Soil pH dominance over livestock management in determining bacterial assemblages through a latitudinal gradient of European meadows and pastures. Ecological Indicators 155, 111063.
| Crossref | Google Scholar |
Regan K, Stempfhuber B, Schloter M, Rasche F, Prati D, Philippot L, Boeddinghaus RS, Kandeler E, Marhan S (2017) Spatial and temporal dynamics of nitrogen fixing, nitrifying and denitrifying microbes in an unfertilized grassland soil. Soil Biology and Biochemistry 109, 214-226.
| Crossref | Google Scholar |
Rhoades JD (1996) Salinity: electrical conductivity and total dissolved solids. In ‘Methods of soil analysis: Part 3 Chemical methods. Vol. 5’. (Eds RL Sparks, AL Page, PA Helmke, RH Loeppert, PN Soltanpour, MA Tabatabai, CT Johnston, ME Sumner) pp. 417–435. (Soil Science Society of America: Madison, WI, USA) doi:10.2136/sssabookser5.3.c14
Risch AC, Zimmermann S, Ochoa-Hueso R, Schütz M, Frey B, Firn JL, Fay PA, Hagedorn F, Borer ET, Seabloom EW, Harpole WS, Knops JMH, McCulley RL, Broadbent AAD, Stevens CJ, Silveira ML, Adler PB, Báez S, Biederman LA, Blair JM, Brown CS, Caldeira MC, Collins SL, Daleo P, di Virgilio A, Ebeling A, Eisenhauer N, Esch E, Eskelinen A, Hagenah N, Hautier Y, Kirkman KP, MacDougall AS, Moore JL, Power SA, Prober SM, Roscher C, Sankaran M, Siebert J, Speziale KL, Tognetti PM, Virtanen R, Yahdjian L, Moser B (2019) Soil net nitrogen mineralisation across global grasslands. Nature Communications 10, 4981.
| Crossref | Google Scholar |
Rocca JD, Hall EK, Lennon JT, Evans SE, Waldrop MP, Cotner JB, Nemergut DR, Graham EB, Wallenstein MD (2015) Relationships between protein-encoding gene abundance and corresponding process are commonly assumed yet rarely observed. The ISME Journal 9, 1693-1699.
| Crossref | Google Scholar | PubMed |
Rodríguez-Ortega T, Oteros-Rozas E, Ripoll-Bosch R, Tichit M, Martín-López B, Bernués A (2014) Applying the ecosystem services framework to pasture-based livestock farming systems in Europe. Animal 8, 1361-1372.
| Crossref | Google Scholar | PubMed |
Rösch C, Mergel A, Bothe H (2002) Biodiversity of denitrifying and dinitrogen-fixing bacteria in an acid forest soil. Applied and Environmental Microbiology 68(8), 3818-3829.
| Crossref | Google Scholar |
Rotthauwe JH, Witzel KP, Liesack W (1997) The ammonia monooxygenase structural gene amoA as a functional marker: molecular fine-scale analysis of natural ammonia-oxidizing populations. Applied and Environmental Microbiology 63, 4704-4712.
| Crossref | Google Scholar | PubMed |
Rousseeuw PJ (1987) Silhouettes: a graphical aid to the interpretation and validation of cluster analysis. Journal of Computational and Applied Mathematics 20, 53-65.
| Crossref | Google Scholar |
Schirpke U, Kohler M, Leitinger G, Fontana V, Tasser E, Tappeiner U (2017) Future impacts of changing land-use and climate on ecosystem services of mountain grassland and their resilience. Ecosystem Services 26, 79-94.
| Crossref | Google Scholar | PubMed |
Song Z, Wang J, Liu G, Zhang C (2019) Changes in nitrogen functional genes in soil profiles of grassland under long-term grazing prohibition in a semiarid area. Science of The Total Environment 673, 92-101.
| Crossref | Google Scholar | PubMed |
Sturaro E, Marchiori E, Cocca G, Penasa M, Ramanzin M, Bittante G (2013) Dairy systems in mountainous areas: farm animal biodiversity, milk production and destination, and land use. Livestock Science 158, 157-168.
| Crossref | Google Scholar |
Tattoni C, Ciolli M, Ferretti F, Cantiani MG (2010) Monitoring spatial and temporal pattern of Paneveggio forest (northern Italy) from 1859 to 2006. iForest-Biogeosciences and Forestry 3, 72-80.
| Crossref | Google Scholar |
Uchida Y, Clough TJ, Kelliher FM, Sherlock RR (2008) Effects of aggregate size, soil compaction, and bovine urine on N2O emissions from a pasture soil. Soil Biology and Biochemistry 40(4), 924-931.
| Crossref | Google Scholar |
Unc A, Goss MJ (2004) Transport of bacteria from manure and protection of water resources. Applied Soil Ecology 25, 1-18.
| Crossref | Google Scholar |
Verhamme DT, Prosser JI, Nicol GW (2011) Ammonia concentration determines differential growth of ammonia-oxidising archaea and bacteria in soil microcosms. The ISME Journal 5, 1067-1071.
| Crossref | Google Scholar | PubMed |
Wakelin SA, Colloff MJ, Kookana RS (2008) Effect of wastewater treatment plant effluent on microbial function and community structure in the sediment of a freshwater stream with variable seasonal flow. Applied and Environmental Microbiology 74, 2659-2668.
| Crossref | Google Scholar | PubMed |
Wakelin SA, Gregg AL, Simpson RJ, Li GD, Riley IT, McKay AC (2009) Pasture management clearly affects soil microbial community structure and N-cycling bacteria. Pedobiologia 52, 237-251.
| Crossref | Google Scholar |
Wallenstein MD, Vilgalys RJ (2005) Quantitative analyses of nitrogen cycling genes in soils. Pedobiologia 49, 665-672.
| Crossref | Google Scholar |
Wang M, Hou F (2018) Correlation model analysis of nitrogen addition and tan sheep grazing effects on soil bacterial community in the Loess Plateau, China. In ‘IEEE International Conference on Bioinformatics and Biomedicine (BIBM)’. pp. 1846–1853. (IEEE) Available at doi:10.1109/BIBM.2018.8621343
Wang H, Deng N, Wu D, Hu S (2017) Quantitative response relationships between net nitrogen transformation rates and nitrogen functional genes during artificial vegetation restoration following agricultural abandonment. Scientific Reports 7(1), 7752.
| Crossref | Google Scholar | PubMed |
Wang F, Che R, Deng Y, Wu Y, Tang L, Xu Z, Wang W, Liu H, Cui X (2021) Air-drying and long time preservation of soil do not significantly impact microbial community composition and structure. Soil Biology and Biochemistry 157, 108238.
| Crossref | Google Scholar |
Wheeler RE, Torchiano M (2010) Permutation tests for linear models in R. The Comprehensive R Archive Network 1(2), 1-36.
| Google Scholar |
Wrage-Mönnig N, Horn MA, Well R, Müller C, Velthof G, Oenema O (2018) The role of nitrifier denitrification in the production of nitrous oxide revisited. Soil Biology and Biochemistry 123, A3-A16.
| Crossref | Google Scholar |
Wu Y, Gao X, Cao D, Li L, Li X, Zeng F (2021) Nitrous oxide emissions from an alpine grassland as affected by nitrogen addition. Atmosphere 12, 976.
| Crossref | Google Scholar |
Xu Y, Zhong Z, Zhang W, Han X, Yang G, Ren C, Feng Y, Ren G, Wang X (2019) Responses of soil nosZ-type denitrifying microbial communities to the various land-use types of the Loess Plateau, China. Soil and Tillage Research 195, 104378.
| Crossref | Google Scholar |
Yan L, Zhang W, Duan W, Zhang Y, Zheng W, Lai X (2021) Temporal bacterial community diversity in the nicotiana tabacum rhizosphere over years of continuous monocropping. Frontiers in Microbiology 12, 641643.
| Crossref | Google Scholar | PubMed |
Yang P, van Elsas JD (2018) Mechanisms and ecological implications of the movement of bacteria in soil. Applied Soil Ecology 129, 112-120.
| Crossref | Google Scholar |
Yang Y, Gao Y, Wang S, Xu D, Yu H, Wu L, Lin Q, Hu Y, Li X, He Z, Deng Y, Zhou J (2014) The microbial gene diversity along an elevation gradient of the Tibetan grassland. The ISME Journal 8, 430-440.
| Crossref | Google Scholar | PubMed |
Yang Y, Tilman D, Furey G, Lehman C (2019) Soil carbon sequestration accelerated by restoration of grassland biodiversity. Nature Communications 10, 718.
| Crossref | Google Scholar |
Yergeau E, Kang S, He Z, Zhou J, Kowalchuk GA (2007) Functional microarray analysis of nitrogen and carbon cycling genes across an Antarctic latitudinal transect. The ISME Journal 1, 163-179.
| Crossref | Google Scholar | PubMed |
Yin M, Gao X, Tenuta M, Li L, Gui D, Li X, Zeng F (2020) Enhancement of N2O emissions by grazing is related to soil physicochemical characteristics rather than nitrifier and denitrifier abundances in alpine grassland. Geoderma 375, 114511.
| Crossref | Google Scholar |
Yuan ZY, Jiao F, Li YH, Kallenbach RL (2016) Anthropogenic disturbances are key to maintaining the biodiversity of grasslands. Scientific Reports 6, 22132.
| Crossref | Google Scholar |
Zanella A, Tattoni C, Ciolli M (2010) Studio della variazione temporale della quantità e qualità del bestiame nel Parco di Paneveggio Pale di San Martino e influenza sui cambiamenti del paesaggio forestale. Dendronatura 1, 24-33.
| Google Scholar |
Zendri F, Ramanzin M, Bittante G, Sturaro E (2016) Transhumance of dairy cows to highland summer pastures interacts with breed to influence body condition, milk yield and quality. Italian Journal of Animal Science 15, 481-491.
| Crossref | Google Scholar |
Zhang L, Wang X, Wang J, Wan Q, Liao L, Liu G, Zhang C (2021) Grazing exclusion reduces soil N2O emissions by regulating nirK-and nosZ-type denitrifiers in alpine meadows. Journal of Soils and Sediments 21, 3753-3769.
| Crossref | Google Scholar |
Zhong L, Bowatte S, Newton PCD, Hoogendoorn CJ, Li FY, Wang Y, Luo D (2015) Soil N cycling processes in a pasture after the cessation of grazing and CO2 enrichment. Geoderma 259–260, 62-70.
| Crossref | Google Scholar |
Zhou J, Xue K, Xie J, Deng Y, Wu L, Cheng X, Fei S, Deng S, He Z, Van Nostrand JD, Luo Y (2012) Microbial mediation of carbon-cycle feedbacks to climate warming. Nature Climate Change 2, 106-110.
| Crossref | Google Scholar |
Zhou H, Ma A, Zhou X, Chen X, Zhang J, Gen P, Liu G, Wang S, Zhuang G (2022) Soil phosphorus accumulation in mountainous alpine grassland contributes to positive climate change feedback via nitrifier and denitrifier community. Science of The Total Environment 804, 150032.
| Crossref | Google Scholar | PubMed |
Zhu Y, Merbold L, Leitner S, Xia L, Pelster DE, Diaz-Pines E, Abwanda S, Mutuo PM, Butterbach-Bahl K (2020) Influence of soil properties on N2O and CO2 emissions from excreta deposited on tropical pastures in Kenya. Soil Biology and Biochemistry 140, 107636.
| Crossref | Google Scholar |