The impact of climate change and wildfire on decadal alpine vegetation dynamics
Brodie Verrall




A Centre of Planetary Health and Food Security, Griffith University, Gold Coast, Qld 4222, Australia.
B Griffith Climate Action Beacon, Griffith University, Gold Coast, Qld 4222, Australia.
C New South Wales National Parks and Wildlife Service, Jindabyne, NSW 2627, Australia.
Australian Journal of Botany 71(5) 231-251 https://doi.org/10.1071/BT23002
Submitted: 2 January 2023 Accepted: 26 May 2023 Published: 16 June 2023
© 2023 The Author(s) (or their employer(s)). Published by CSIRO Publishing. This is an open access article distributed under the Creative Commons Attribution-NonCommercial-NoDerivatives 4.0 International License (CC BY-NC-ND)
Abstract
Context: Climate-induced changes of alpine vegetation diversity, cover and composition have been recorded globally, but most insights have been gleaned from field studies over small spatiotemporal scales.
Aims: We assess dynamics in climate and vegetation of Australia’s highest and most biologically diverse alpine area that surrounds Mount Kosciuszko (~455 km2), as well as recovery following the 2003 wildfires.
Methods: Climatic changes were analysed using gridded climate data for mean annual temperature (1910–2019) and seasonal precipitation (1900–2019), and changes in snow cover were assessed from snow course records (1954–2021). A vegetation cover time series (1990, 2000, 2010, 2020) was modelled with an optimised random forest classification using recursive feature selection, and the LandTrendr algorithm was used to detect areas burnt during wildfires.
Key results: Over time, temperatures and summer precipitation increased, whereas snow cover and winter precipitation decreased. Subsequently, vegetation dynamics were dominated by the densification of subalpine woodlands at lower elevations, replacing dry and wet heathlands. There was treeline stasis but upslope advancement of dry and wet shrublines but grassland vegetation types were relatively stable. However, in burnt areas there was suppressed upslope advancement of shrublines, treeline recession and relatively less expansion of subalpine woodlands.
Conclusions: Alpine vegetation may be impacted by climate change incrementally through relatively gradual changes in climatic conditions, and transformatively through landscape-level disturbance from wildfires.
Implications: Higher temperatures and altered precipitation regimes increase the frequency and severity of wildfires, which may be amplified by increasing fuel loads and dryness from the proliferation and advance of woody vegetation in alpine areas.
Keywords: alpine vegetation cover classification, alpine wildfire regimes, Australian Alps, disturbance attribution, land-cover change, Landsat 5/7/8 time series, LandTrendr algorithm, multispectral remote sensing, optimised random forest modelling, plant–climate interactions.
Introduction
Alpine vegetation occurs above the bioclimatic treeline, and its taxonomic composition and vegetation structure are foremost governed by low temperatures and relatively short growing seasons (Körner 2003, 2012). However, complex topography, microclimatic heterogeneity, varying substrate and periglacial disturbance regimes result in treeline ecotones transitioning to a mosaic of low growing alpine grasslands, heathlands and fellfields (synonymous with ‘feldmark’) that support high biodiversity and endemism (Körner 2004; Elsen and Tingley 2015; Perrigo et al. 2020). Most alpine plants are cryophilic, slow growing and long-lived perennials that are resilient to fluctuations in weather, but respond to both extreme climatic events (Williams et al. 2008; Alatalo et al. 2016; De Boeck et al. 2016; Venn et al. 2017) and sustained climatic shifts (Nagy and Grabherr 2009; de Witte and Stöcklin 2010; De Boeck et al. 2018). However, anthropogenic climate change has caused rapidly rising temperatures, declining snow cover and lengthening growing seasons (Huss et al. 2017; Hock et al. 2019), and there is evidence of elevation-dependent amplification of these changes in climate (Pepin et al. 2015). Therefore, as the climate continues to warm, the stability of alpine ecosystems and vegetation is particularly vulnerable (Grabherr et al. 2010; Guisan et al. 2019; Verrall and Pickering 2020).
With disproportionate exposure to climate change impacts, along with particularly climate-sensitive plants (Dirnböck et al. 2011), climate-induced vegetation dynamics within alpine areas have been broadly reported and are expected to continue with climate change. Although there are numerous other factors that may influence alpine vegetation dynamics including the availability of water, nutrients and light as well as biotic interactions, climate change is widely attributed to be the primary factor causing declines in snow cover, variable precipitation regimes, longer growing seasons and warmer temperatures (Körner 2003; Hock et al. 2019). Treeline dynamics, woody encroachment and increasing competition from thermophilic taxa have been found to occur (Myers-Smith et al. 2015; Lamprecht et al. 2018; Hansson et al. 2021), as the amount of thermal energy available to alpine plants has changed considerably over recent decades (Grabherr et al. 2010). Climate change has also seemingly resulted in the loss of highly specialised, cryophilic vegetation maintained by periglacial process, such as snowpatch communities, via encroachment of thermophilic and generalist alpine species (Carbognani et al. 2014; Sandvik and Odland 2014; Verrall et al. 2023).
Climate-induced responses of alpine vegetation and subsequent implications for ecosystem processes are well documented, with an array of methods utilised to understand these changes (Grabherr et al. 2010; Hock et al. 2019; Verrall and Pickering 2020). Generally, responses of alpine vegetation to climate change are investigated by simulating environmental changes and space-for-time chronosequence approaches (e.g. Wieser et al. 2019), as well as investigating historical changes via dendrochronology (e.g. Danby et al. 2011) and palynology (e.g. Thomas et al. 2022). There has also been an increase in the use of long-term ecological monitoring techniques in alpine areas (Verrall and Pickering 2020), where permanent vegetation plots are resurveyed through time (Pauli et al. 2012). However, field sampling in large, remote and rugged alpine areas that experience extreme climatic conditions is not always possible, and these logistical barriers may lead to a biased understanding of these changing ecosystems. In response, alongside technological advances over the past few decades, remote sensing techniques have been increasingly used to assess alpine vegetation (Verrall and Pickering 2020). Remote sensing has a distinct spatial and accessibility advantage, leading to more efficient, effective and comprehensive monitoring of alpine areas (Peyre et al. 2021; Mienna et al. 2022; Zandler et al. 2022), yet most studies focused on the northern hemisphere (Chhetri and Thai 2019).
The northern hemisphere contains the majority of the world’s alpine ecosystems (Testolin et al. 2020) and has received disproportionate research attention (Verrall and Pickering 2020). However, relatively few studies have focused on the equally socially and ecologically important alpine ecosystems in the southern hemisphere (Körner 2003; Körner and Ohsawa 2005), including in the Australian Alps. With relatively small areas high-elevation mountain ranges and bioclimatic treelines only a few hundred metres below the highest peaks, alpine ecosystems are marginal and rare in Australia (Costin et al. 2000), covering less than 0.02% of the continent (Green and Stein 2015). Yet, they support high species richness and endemism as well as providing crucial ecosystem services including water provisioning to much of south-eastern Australia (Slattery and Worboys 2020). However, the lack of alpine weather stations and consistent climate data records have resulted in a poor understanding of recent climate changes above the treeline. Therefore, most studies only assess the few decades worth of available data (Verrall et al. 2021), but there is still clear evidence of warming and lengthening growing seasons (Fiddes et al. 2015).
Furthermore, Quaternary climate dynamics in the Australian Alps show that current temperatures are the warmest and snow cover is the lowest in 2000 years (McGowan et al. 2018), and wildfire activity has increased concurrently (Thomas et al. 2022). Generally, pollen-based vegetation reconstructions in the Australian Alps suggest that vegetation dynamics were responsive to past climatic changes, with the decline of alpine taxa coinciding with rising temperatures (Martin 1986; Thomas et al. 2022). In response to the rapid climate change over recent decades, there has been an array of field-based assessments aiming to understand alpine vegetation dynamics in the Australian Alps (Venn et al. 2017). Generally, there has been an increase in vegetation cover driven by thermophilic graminoids and shrubs (Verrall et al. 2021, 2023) whereas in burnt areas, most alpine vegetation communities are relatively resilient to a single wildfire event and have been found to recover rapidly (Camac et al. 2013; McDougall et al. 2015; Venn et al. 2016). Nonetheless, wildfire is a strong demographic filter on contemporary alpine and treeline dynamics in the Australian Alps (Naccarella et al. 2020), as pastoral burning practices have been linked to stand-densification of the monospecific Eucalptus niphophila treeline (Martin 1999).
Despite the importance and implications of alpine climate and vegetation dynamics in the Australian Alps, remote sensing has mostly been used to assess changes in snow cover (Bormann et al. 2012; Thompson 2016; Rasouli et al. 2022) and growing season phenology (Thompson and Paull 2017), and is yet to be utilised to model vegetation cover dynamics over large areas (McDougall 2003). Furthermore, given that the risk of wildfire has been found to be increasing in alpine areas around the world (Hock et al. 2019), the Australian Alps also presents an opportunity to assess the impact of landscape-level wildfire on alpine vegetation in the southern hemisphere. Over recent millennia, large wildfires have occurred every few hundred years in the Australian Alps but land use, fire practices and wildfire regimes changed dramatically post-colonisation (1788) (Dodson et al. 1994; Martin 1999; Williams et al. 2008). These altered regimes are now amplified by climate change leading to more severe and frequent impacts from wildfires (Clarke et al. 2013; Kirkpatrick and Bridle 2013; Zylstra 2018). Alpine vegetaion is experiencing higher temperatures and precipitation variability, and there is also evidence of woody encroachment and densification, which have increased flammability and biomass respectively, forming a positive feedback loop (Camac et al. 2017). Therefore, wildfire may present as a major threat that transforms alpine ecosystems in the future as the climate continues to warm (Williams et al. 2008; Fraser et al. 2016; Venn et al. 2017; Thomas et al. 2022).
By assessing recent climatic and associated vegetation dynamics in the highest alpine area in Australia, this study focused on three primary objectives. The first objective was to test dynamics in relevant climatic factors such as temperature, precipitation and snow cover over the last century, where we hypothesised increasing temperatures and precipitation variability along with declining snow cover. The second objective was to model the structural response of alpine vegetation over the past 30 years, where we hypothesised densification and in-filling of the subalpine woodlands at treeline as well as encroachment of dry heathlands, resulting in an advance of the shrubline over time. The third and final objective was to compare burnt and unburnt areas from the 2003 wildfires, where we hypothesised that the dry grasslands and dry heathlands recovered quickly in areas that burned, and there was also treeline and shrubline suppression in burnt areas.
Materials and methods
Study area
The largest and most diverse contiguous alpine vegetation complex in Australia is located in the Snowy Mountains section of the Australian Alps, surrounding Mount Kosciuszko/Kunama Namadgi (2228 m above sea level, a.s.l.), which is the highest mountain on the land mass (Costin et al. 2000) (Fig. 1). The study area experiences a marginal snowpack that commonly forms in June and becomes discontinuous in September (Bormann et al. 2012; Thompson and Paull 2017; Schwartz et al. 2020b), with an annual mean daily temperature of 4.37°C and an average annual precipitation (rain and snow) of 2165 mm (1976–2005 reference period – Xu and Hutchinson 2011). Although wildfire is an agent of disturbance in this area (Thomas et al. 2022), particularly in periods of extended drought, large and severe wildfires burning over 100 km2 of alpine vegetation are rare but were recorded in 1939 and 2003 across the Australian Alps (Williams et al. 2008). There have been several other smaller wildfires across the Australian Alps, with alpine vegetation in burning multiple times in recent decades (Naccarella et al. 2020). Although large swathes of the surrounding landscape burnt in the 2019/20 black summer bushfires, the study area remained largely unburnt.
Location and characteristics of the ~455 km2 study area (a–c) including reference photos of vegetation types (d–i) for the proposed classification. Map locations are shown in eastings and northings in plot (a).
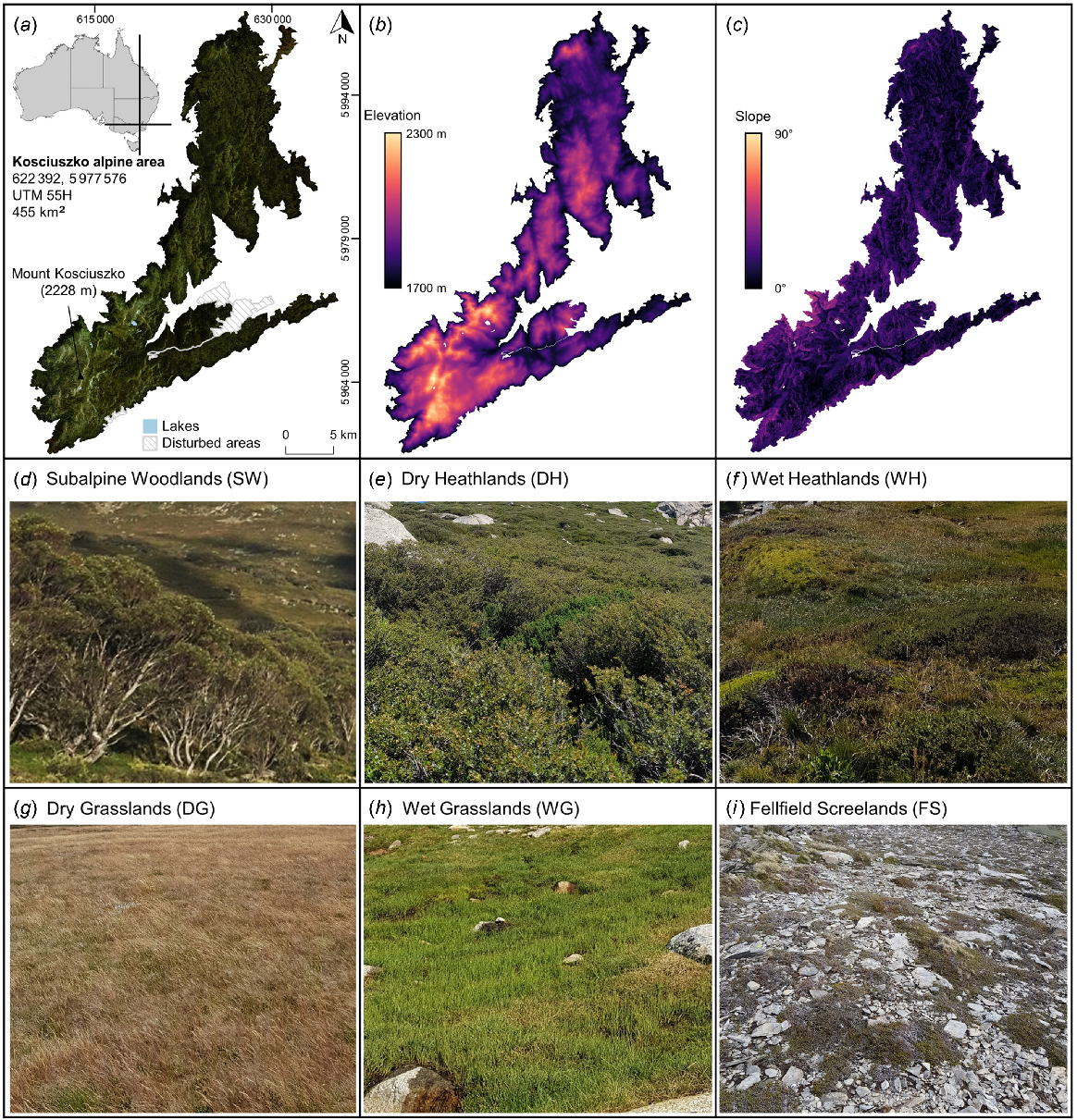
The complex topography, microclimatic heterogeneity and geographic isolation of the study area has given rise to a species rich flora with high endemism (Costin et al. 2000). The alpine treeline ecotone is dominated by snow gums (E. niphophila), forming an irregular and fractured demarcation between the alpine and subalpine zones where it ranges from 1700–1900 m a.s.l. depending on latitude, aspect, topography and soils (Green 2009; Green and Venn 2012). For the purposes of this investigation, the study area covers the continuous ~455.07 km2 above 1700 m that surrounds Mount Kosciuszko (Kosciuszko Alpine Area), but with permanent alpine lakes and areas disturbed by human activities (including ski resort infrastructure, cleared ski runs and bitumen roads) masked and excluded from analysis (Fig. 1). Previously, there have been several relevant plant community classifications conducted (CSIRO 1972; McDougall and Walsh 2007; Mackey et al. 2015). These plant community classifications can further be simplified to broad vegetation types including broad vegetation types that formed the classification framework for this investigation: subalpine woodlands (SW), dry heathlands (DH), wet heathlands (WH), dry grasslands (DG), wet grasslands (WG), fellfield screelands (FS) (Walsh and McDougall 2004) (Fig. 1, Supplementary Table S1); and one scarcely vegetated landcover type, rocky outcrops (RO) (Fig. S1).
Methods workflow
An overview of the methods used in this study to assess climatic and vegetation dynamics in a section of the Australian Alps is shown in Fig. 2. We used data from the Australian Gridded Climate Data project (Jones et al. 2009), snow course records (Snowy Hydro 2022) and Landsat satellite imagery sourced from the Google Earth Engine data catalogue. By filtering Landsat collections temporally by growing season dates (January 1st to May 31st) and geographically by the study area (091/085), growing season composites were constructed using a median reducer at decadal intervals (1990, 2000, 2010, 2020). The 2020 composite, along with geolocated training data, were used to optimise a random forest classification model using recursive feature elimination and optimal model parameters to improve classification accuracy. This model with optimised parameters and features was then used to hindcast vegetation cover types from growing season composites for 1990, 2000 and 2010. Temporal dynamics in cover and mean elevation weighted by area (zonation) of vegetation types were then calculated across the study area, as well as in unburnt and burnt areas that were identified using the normalised burn ratio (NBR) through the disturbance detection LandTrendr algorithm. Vegetation type cover and zonation dynamics were then assessed and attributed to dynamics in relevant climatic metrics including temperature, precipitation, snow cover and burn status.
Workflow of the methods used to assess climate and vegetation dynamics in the Australian Alps, which can be split into three broad steps with the platform used denoted for each step (Google Earth Engine and R). The first step overviews the Landsat data acquisition and filtering to obtain growing season collections at decadal intervals, and demonstrates how growing season composites were generated. The second step overviews the procedure to obtain an optimised random forest classification model, which was used to classify decadal growing season composites. The third and final step overviews the temporal analysis of dynamics in vegetation types and climate data.
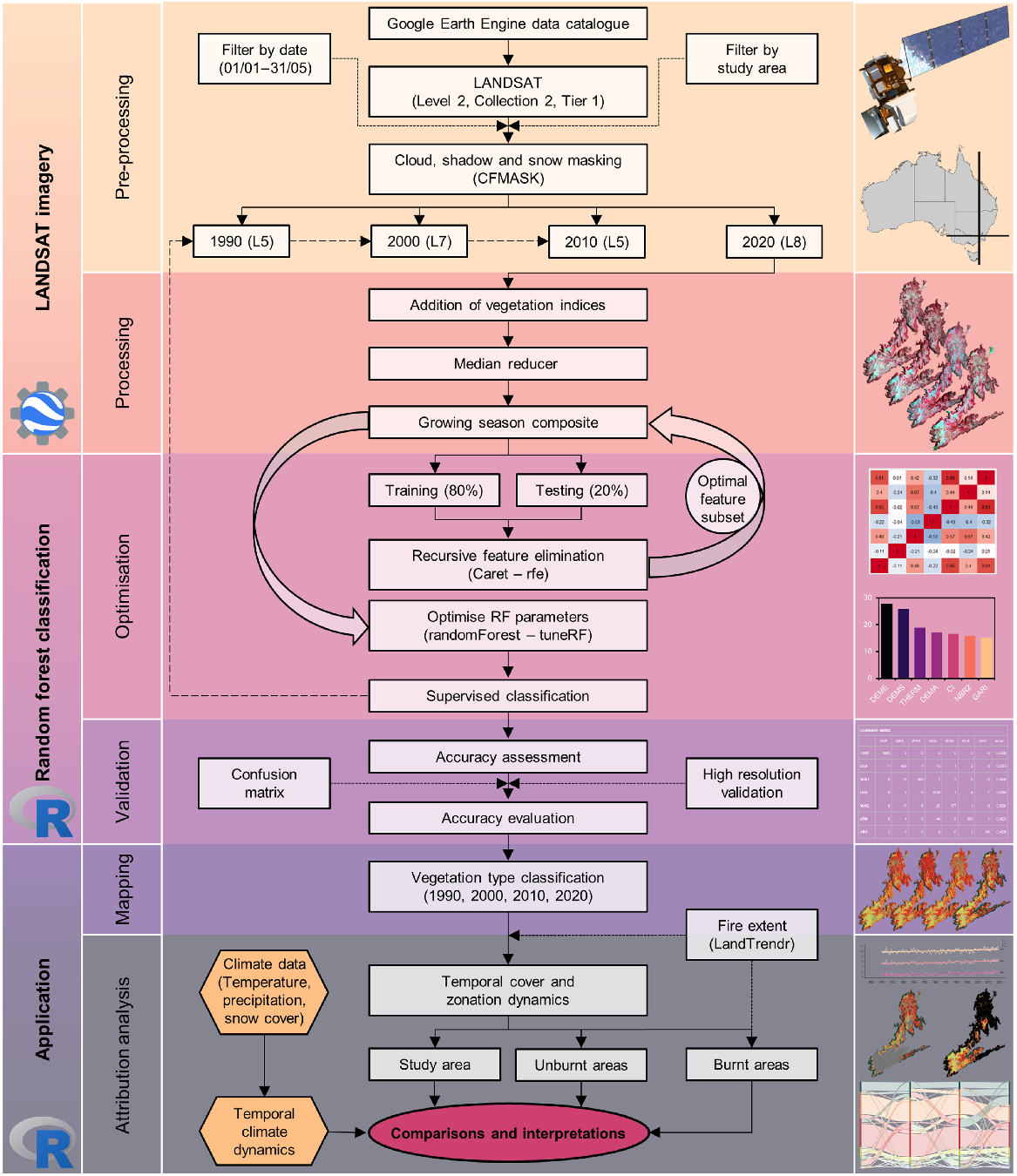
Climate and remote sensing data
Temperature (1910–2020) and precipitation (1900–2020) data were sourced from the Australian Gridded Climate Data (AGCD v1.0.0) (Jones et al. 2009). Data were downloaded as monthly two-dimensional raster arrays at 0.05° (~5 km) spatial resolution, and were clipped to 16 grids (~400 km2) that were located within the study area. Annual mean temperature was calculated by averaging over monthly mean temperatures. Seasonal precipitation was calculated as the sum of the three-monthly totals for summer (December from previous year, January and February) autumn (March, April and May), winter (June, July and August), and spring (September, October and November). Snow cover (1954–2021) data were sourced from the Snowy Hydro Project Spencers Creek Snow Course, which is located at 1829 m a.s.l. in the south-east of the study area (−36.4257° S, 148.3470° E). To reflect both depth and duration of snow cover, snow-metre days (md) per year were calculated by summing the snow depth multiplied by the number of days at that depth (Green and Pickering 2009; Sánchez-Bayo and Green 2013). Annual metrics for temperature, precipitation and snow cover used to assess temporal climatic dynamics, and linear regressions were fit via the base package ‘Stats’ v4.1.3 (R Core Team 2022). All climatic variables met the assumptions for linear regression except snow cover, which was log transformed.
Imagery data were sourced from the United States Geological Survey (USGS) Landsat Program via the Google Earth Engine data catalogue using atmospherically corrected surface reflectance and land surface temperature collections (Collection 2, Level 2, Tier 1) derived from Landsat 5 Enhanced Thematic Mapper (ETM), Landsat 7 Enhanced Thematic Mapper+ (ETM+) and Landsat 8 Operational Land Imager (OLI). Several studies posit that time series extracted from different Landsat sensors can provide an accurate and comparable representation of temporal vegetation dynamics (Banskota et al. 2014; Vogelmann et al. 2016). Thus, Landsat collections (Path: 091, Row: 085) were filtered consistently by date (01/01 to 31/05) to represent the snow-free growing season and the CFMASK algorithm was applied for cloud, cloud shadow and snow masking. Google Earth Engine was then used to generate composite images that were clipped to the extent of the study area at decadal intervals (1990, 2000, 2010, 2020) using a median reducer. Landsat 5 ETM was used to generate growing season composites in 1990 and 2010 (striping present in 2010 composites generated with Landsat 7 imagery due to the scan line corrector malfunction), and Landsat 7 ETM+ and Landsat 8 OLI were used to generate composites for 2000 and 2020, respectively. Digital elevation model (DEM) data at 2 m spatial resolution were downloaded for the study area, and mean elevation, slope and aspect were resampled to 30 m spatial resolution to align with Landsat pixels.
When classifying target objects such as vegetation cover types, raw multispectral data on their own are not always sufficient (Gislason et al. 2006; Guan et al. 2013). Therefore, an array of 69 features, made up of vegetation and geographic indices, were then added to growing season composites (Table S2). Previous classifications of vegetation cover in mountainous regions have highlighted the importance of particular features to improve model performance and classification accuracy, including specific vegetation indices and ancillary geographic data derived from DEM (McCaffrey and Hopkinson 2020; Lin et al. 2021). Since elevation, slope and aspect are key determinants of alpine vegetation types in the study area (Costin et al. 2000; McDougall and Walsh 2007; Mackey et al. 2015), they were added as features to growing season composites. Additionally, a range of vegetation indices (VIs) were calculated and added to growing season composites, including VIs previously used to classify alpine vegetation (Lin et al. 2021) and sensor-specific VIs (Henrich et al. 2009). Landsat bands were also added as features, with the three visible (Blue, Green and Red), one near-infrared (NIR), two short-wave infra-red (SWIR1, SWIR2) bands processed to orthorectified surface reflectance, and one thermal infra-red (TIR) band processed to orthorectified surface temperature (Table 1).
Bands | Spatial resolution | Spectral range of sensor (μm) | ||
---|---|---|---|---|
Landsat 5 ETM | Landsat 7 EMT+ | Landsat 8 OLI | ||
B | 30 | 0.45–0.52 | 0.45–0.52 | 0.452–0.512 |
G | 30 | 0.52–0.60 | 0.52–0.60 | 0.533–0.590 |
R | 30 | 0.63–0.69 | 0.63–0.69 | 0.636–0.673 |
NIR | 30 | 0.77–0.90 | 0.77–0.90 | 0.851–0.879 |
SWIR1 | 30 | 1.55–1.75 | 1.55–1.75 | 1.566–1.651 |
SWIR2 | 30 | 2.08–2.35 | 2.08–2.35 | 2.107–2.294 |
TIR | 30 | 10.40–12.50 | 10.40–12.50 | 10.60–11.19 |
Optimisation of random forest classification
Supervised classification of the study area into the six vegetation cover types (subalpine woodlands, dry heathlands, wet heathlands, dry grasslands, wet grasslands, fellfield screelands) and one landcover type (rocky outcrops) was executed using an optimised random forest (RF) algorithm on the 2020 growing season composite. As proposed by Breiman (2001), RF is a nonparametric, tree-based ensemble classifier that generates multiple decision trees using randomly selected features and training samples. Classification of unlabelled data is then determined by an unweighted voting scheme where each pixel is assigned the class with the majority of votes. RF is also highly effective in generalisation, reducing overfitting as well as handling dimensionality and collinearity bias, and has been shown to be suitable for classifying multispectral data (Belgiu and Drăguţ 2016). It also has been shown to yield greater accuracy in comparison to other classification algorithms, and is capable of dealing with data variability in both training samples and unclassified data (Ma et al. 2017; Talukdar et al. 2020). RF classification has become a popular method to accurately determine vegetation and land cover (Rodriguez-Galiano et al. 2012; Khatami et al. 2016; Ma et al. 2017), including in alpine environments (Zhang et al. 2019; Liu et al. 2021; Filippa et al. 2022; Meng et al. 2022).
For the RF classification of this study area, training data polygons were generated from recent field surveys and high resolution SPOT-7 imagery (1.5 m spatial resolution). Specifically, recent vegetation surveys of permanent, geolocated plots (Verrall and Pickering 2019; Verrall et al. 2021; Verrall et al. 2023) were used to assign the cover of vegetation types to polygons across the study area, which then guided the collection of additional training data from SPOT-7 imagery. As the study area is known to contain varying cover of each vegetation type (Costin et al. 2000; Mackey et al. 2015), training data reflects the in situ proportions of data in each class. To improve the accuracy of classification, a minimum of 25 polygons were generated across the study area with a minimum cumulative extent of 15 hectares (0.03% of study area) for each of the six vegetation cover types (subalpine woodlands, dry heathlands, wet heathlands, dry grasslands, wet grasslands, fellfield screelands), and 5 hectares for the one landcover type (rocky outcrops) (Millard and Richardson 2015). Training data were then randomly split, with 80% of the dataset used for training and 20% for validation. However, the 2020 growing season composite contained a large set of features (69), which can increase computational complexity and lead to dimensionality problems as features are commonly highly correlated and redundant (Gregorutti et al. 2017).
Deciding which features to select in RF classification is a crucial but difficult, subjective and often error prone task (Belgiu and Drăguţ 2016; Georganos et al. 2018). Hence, classification performance can be greatly improved by optimising feature selection by using Recursive Feature Elimination (RFE) (Lin et al. 2021). This optimised feature selection algorithm is broadly utilised to derive discriminant feature rankings and determine the best performing subset of features. At each iteration, the least useful feature is eliminated, and the model is refit on the reduced feature subset until all features have been evaluated (Georganos et al. 2018; Kuhn et al. 2022). Here, RF-RFE with 10-fold cross-validation repeated 10 times was used to select the optimal feature subset from the 69 contained within the 2020 growing season composite via the ‘caret’ package v6.0-92 (Kuhn et al. 2022) (Fig. S2). Features were ranked (Figs. S3 and S4) and an optimised subset of seven features were selected (Table S3): Digital Elevation Model Elevation (DEME); Digital Elevation Model Slope (DEMS); Thermal Infrared (TIR); Digital Elevation Model Aspect (DEMA); Colouration Index (CI); Normalised Burn Ratio 2 (NBR2); and Green Atmospherically Resistance Index (GARI). Following RFE, the parameters of the RF model were then optimised via the ‘tuneRF’ function in the ‘randomForest’ package v4.7-1.1 (Breiman et al. 2022) (Table S4). These same optimised features and model parameters were then used to classify the growing season composites for 1990, 2000 and 2010.
Validation and accuracy assessments of classification
After classification of the 2020 growing season composite, the overall cross-validation accuracy of the optimised RF-RFE model was 97.98%, and the class-specific accuracies were all above 80% (Table S4). To build upon cross-validation and further improve the confidence of classification, manual validation of classifications where pixels are assigned relevant landcover categories using higher resolution imagery is commonly used (Hansen et al. 2013; Mu et al. 2015; Blatchford et al. 2021). Here, manual validation was conducted using SPOT-7 imagery (1.5 m resolution) captured in 2020 for 500 randomly selected pixels. To avoid bias, pixels that underwent manual validation were independent of training data (Hammond and Verbyla 1996), and were distributed throughout the diversity of slopes, aspects and elevations present in the study area (Strahler et al. 2006; Millard and Richardson 2015). Each of the 500 testing pixels was assigned one of the seven cover types based on the dominant vegetation present within the bounds of that pixel and was then compared to the 2020 RF-RFE classification. Overall, manual classification accuracy was 88.2%, with a 2% over-estimation of subalpine woodlands and wet heathlands being the greatest classification error. Therefore, based on these results, we considered the optimised RF-RFE classifier to have recognised the major vegetation cover types across the study area with sufficient accuracy for the purposes of this study.
Pixel-based disturbance detection using the LandTrendr algorithm
LandTrendr (Landsat-based detection of trends in disturbance and recovery) is a time series segmentation algorithm to detect disturbance via identifying gradual and abrupt fluctuations in the trajectory of a given spectral index. First, this algorithm calculates the years of change by identifying the beginning and end of each temporal segment in the spectral trajectory, also known as vertices, and second, it calculates the spectral index values at each of the vertices to generate the trajectory of interconnected temporal segments that elucidate pixel-based disturbance (Kennedy et al. 2007; Kennedy et al. 2010). As the study area is known to have experienced wildfires between 1990 and 2020 (Williams et al. 2008; Mackey et al. 2021), the LandTrendr algorithm was used in Google Earth Engine to quantify the extent of fire-type disturbance using the normalised burn ratio (NBR) spectral index (Kennedy et al. 2018), which has been similarly applied in other mountainous regions (Li et al. 2021; Rodman et al. 2021). The NBR spectral index was selected due to its sensitivity to chlorophyll, charcoal ash and water content in leaves, enhancing the ability to detect fire-type disturbance of vegetation (Kennedy et al. 2010). To obtain the LandTrendr derived extent of burnt areas, the NBR recovery threshold was set at 0.25 and temporal segments in pixels with a magnitude of greater than this threshold in wildfire years (2003 and 2020) were identified as burnt areas.
Cover and zonation of vegetation types
Assessments of the temporal dynamics of vegetation types were pixel-based with each of the 514 214 Landsat pixels covering a planimetric area of 900 m2 (30 m × 30 m resolution) (Knoflach et al. 2021). Accounting for the topographical variation and associated bioclimatic zonation of vegetation across the study area (Costin et al. 2000; McDougall and Walsh 2007; Mackey et al. 2015), mean elevation was calculated from each of the pixel from a LiDAR-derived 2 metre DEM. Pixel centroids were generated and was used to spatially join mean elevation and the modelled vegetation type at each decadal interval. By calculating the culumative area and mean elevation weighted by area of each vegetation type, vegetation cover and zonation dynamics could be assessed at decadal intervals. Dynamics in vegetation cover were assessed using using Sankey diagrams via the R ‘networkD3’ package v0.4 (Allaire et al. 2017). The cumulative area of each vegetation type within 10 metre intervals throughout the elevation gradient was represented using an area plot via the ‘ggplot2’ package v0.4 (Wickham et al. 2022). Changes in cover and mean elevation weighted by area of vegetation types are assessed as annual change (m/year or km2/year) and as percentage change. By assessing changes in the cover of vegetation types along an elevational gradient, densification is inferred by a temporal increase of cover at lower elevations whereas advancement is inferred by an increase of cover at higher elevations. These analyses were conducted for the entire study area, and then for burnt and unburnt areas to better understand the impacts of wildfire.
Results
Climate dynamics
Climatic changes have been observed in the study area over the past century with mean annual temperatures increasing by 0.1°C per decade (R2 = 0.359, P < 0.001) (Table S5), with an overall increase of +0.75°C since the beginning of the records (1910–1939 in comparison to 1990–2019; a 30-year period) (Fig. 3a). There has also been an increase in minimum (R2 = 0.335, P < 0.001) and maximum (R2 = 0.245, P < 0.001) annual temperatures, also warming by 0.1°C per decade. Accumulative annual precipitation in the study area was variable, but there were trends in seasonal precipitation with increasing totals in summer by 6.51 mm per decade (R2 = 0.035, P = 0.041) but declining totals in winter by 9.68 mm per decade (R2 = 0.038, P = 0.032) (Fig. 3b). Snow course records from the only long-term plot (Spencers Creek) in the study area demonstrate declining snow cover by 12.74 md per decade (R2 = 0.058, P = 0.046), which is a reduction of 24.3% since the beginning of records (1954–1983 in comparison to 1992–2021; 30-year period) (Fig. 3c).
Vegetation cover dynamics from 1990 to 2020
There were considerable changes in the cover of vegetation types in the four observed growing seasons, with only the cover of rocky outcrops having remained relatively constant over time (+0.01 km2 p.a. (per annum)) (Fig. 4). The cover of subalpine woodlands fluctuated through time, with an overall net increase of 70.58 km2 (+134.8%; +2.35 km2 p.a.) from 1990 to 2020 (Table S6). In 2020, subalpine woodlands cover an additional 15.5% of the study area compared to 1990, mostly replacing heathlands with the net loss of 50.45 km2 by dry heathlands (−35.1%; −1.68 km2 p.a.) and 20.64 km2 of wet heathlands (−13.4%; −0.69 km2 p.a.). However, there was minimal loss in cover of all three woody vegetation types (−0.1%; −0.52 km2) when grouped together from 1990 to 2020. The cover of grasslands was variable, with a marginal loss in cover over time (−5.9%; −0.19 km2 p.a.) mostly due to the loss of 11.01 km2 of wet grasslands (−23.3%; −0.37 km2 p.a.). Furthermore, the cover of dry grasslands increased by 1.2% of the study area, expanding from 47.88 km2 in 1990 to 53.25 km2 in 2020 (+11.2%; +0.18 km2 p.a.). Areas of sparse vegetation cover (fellfield screelands) including screes, granitic boulder fields and feldmark communities were in decline from 7.97 km2 in 1990 to 6.90 km2 in 2000 (−13.4%; −0.11 km2 p.a.) and then rate of loss slowed, with 6.72 km2 present in 2010 (−2.6%; −0.02 km2 p.a.). However, the cover of fellfield screelands more than doubled to 13.82 km2 in 2020.
Decadal vegetation dynamics across the Kosciuszko Alpine Area with (a) the spatial distribution of vegetation types and (b) the flow of area covered by vegetation types through time. Vegetation and landcover types include subalpine woodlands (SW), dry heathlands (DH), wet heathlands (WH), dry grasslands (DG), wet grasslands (WG), fellfield screelands (FS) and rocky outcrops (RO).
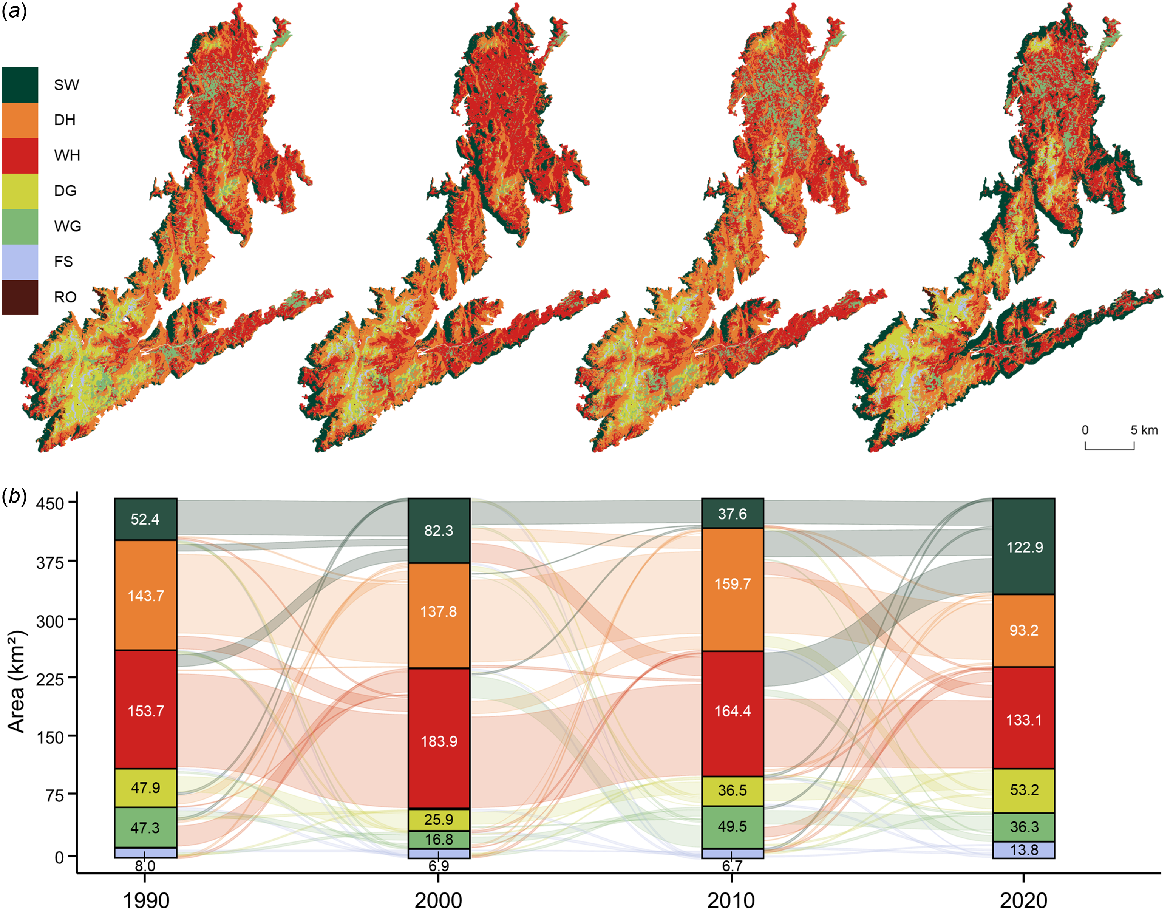
Burnt vegetation cover dynamics
The study area experienced two wildfires between 1990 and 2020, with large tracts of alpine vegetation burnt in 2003 and a smaller area burnt in 2020 (0.2%; 0.90 km2). In 2003, 58.9% of the study area burnt (267.83 km2) as the wildfire travelled up the steep western escarpment and across the relatively flat and low-elevation northern plateau (see Fig. 1). Based on the vegetation types present in 2000, mostly woody vegetation was burnt in 2003 including 130.31 km2 of wet heathlands, 65.84 km2 of subalpine woodlands and 65.63 km2 of dry heathlands, with only 6.05 km2 of grasslands impacted (Fig. 5, Table S6). Although the cover of woody vegetation had increased by 22.52 km2 (+9.4%; +2.25 km2 p.a.) in the decade to 2000, after the wildfire there was a considerable reduction in the cover of subalpine woodlands (−62.0%; −40.83 km2) over the following decade to 2010. Similarly, there was a reduction in the cover of wet heathlands (−12.2%) in the decade to 2010. However, there was an increase in dry heathlands by 20.39 km2 (+31.1%) as well as considerable expansions of wet grasslands by 27.31 km2 (+566.6%) and dry grasslands by 8.29 km2 (+674.0%). In 2020, the cover of subalpine woodlands had increased considerably from 2010 by 66.95 km2 (+227.7%) with losses mostly from wet heathlands by 41.09 km2 and dry heathlands by 26.47 km2. Similarly, the cover of dry grasslands increased by 5.89 km2 (+61.9%) from 2010 to 2020, mostly through the loss of 5.93 km2 of dry heathlands; and 13.87 km2 of wet grasslands transitioned to wet heathlands. Overall, when comparing vegetation types in 2000 (3 years pre-wildfire) and in 2020, those vegetation types that have increased in cover include subalpine woodlands (+39.7%; +1.31 km2 p.a.), wet grasslands (+410.4%; +0.99 km2 p.a.), dry grasslands (+1152.9%; +0.71 km2 p.a.) and fellfield screelands (+0.11 km2 p.a.). However, vegetation types that have decreased in cover after burning include wet heathlands (−31.5%; −2.05 km2 p.a.) and dry heathlands (−33.2%; −1.09 km2 p.a.).
Decadal vegetation dynamics in burnt areas of the Kosciuszko Alpine Area from the 2003 wildfire with (a) the spatial distribution of vegetation types and (b) the flow of area covered by vegetation types through time. Vegetation and landcover types include subalpine woodlands (SW), dry heathlands (DH), wet heathlands (WH), dry grasslands (DG), wet grasslands (WG), fellfield screelands (FS) and rocky outcrops (RO). Burnt and unburnt areas detected using LandTrendr.
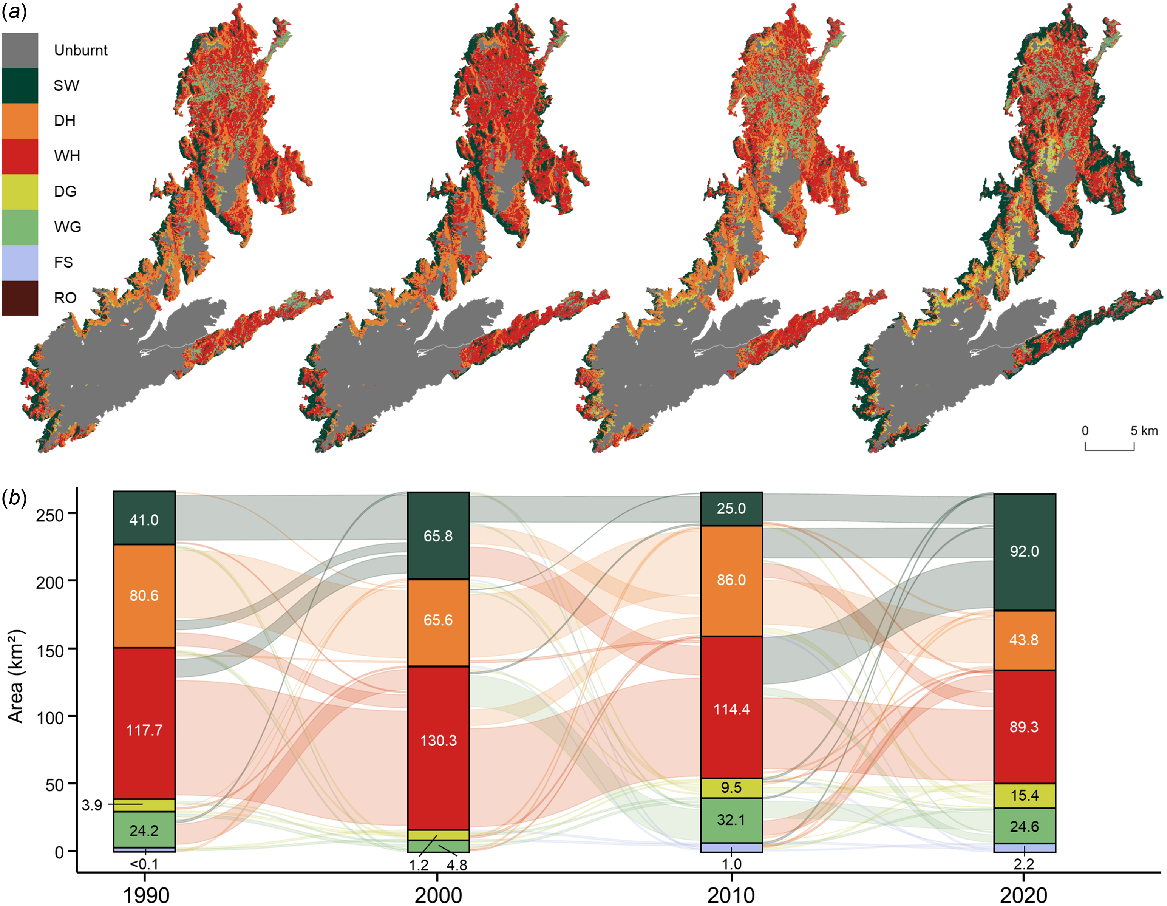
Unburnt vegetation cover dynamics
Of the 41.1% of the study area that remained unburnt in 2003, most of this area is centred around the high-elevation southern plateau (Fig. 6). Based on the vegetation types present in 2000, this unburnt area contained the majority of dry heathlands (52.4%; 72.14 km2), dry grasslands (95.2%; 26.63 km2), wet grasslands (71.3%; 12.00 km2) and fellfield screelands (100.0%; 6.90 km2). Similar to burnt areas, the cover of woody vegetation had increased between 1990 and 2000 by 31.64 km2, but at 1.4 times the rate (+28.6%; +3.16 km2 p.a.) (Table S6). During the decade to 2000, woody encroachment was largely driven by an increase in wet heathlands (+48.9%; +1.76 km2 p.a.) with smaller increases in subalpine woodlands (+44.0%; +0.50 km2 p.a.) and dry heathlands (+14.3%; +0.90 km2 p.a.). From 2000 to 2010, vegetation composition was relatively stable but there was a slight expansion of grasslands (+21.1%; 0.77 km2 p.a.) and a subsequent reduction in woody vegetation (−4.2%; −0.59 km2 p.a.). However, considerable differences emerge when comparing vegetation dynamics in burnt and unburnt areas between 2000 and 2020. The cover of subalpine woodlands increased by 14.57 km2 (+88.7%; +0.73 km2 p.a.) in unburnt areas during these two decades, which was considerably less than the rate of change observed in burnt areas (+39.7%; +1.31 km2 p.a.). Whereas the loss of dry heathlands was comparable between unburnt (−31.5%; −1.14 km2 p.a.) and burnt (−33.2%; −1.09 km2) areas, the loss of wet heathlands in unburnt areas (−18.3%; −0.49 km2) was considerably less than the rate of change observed in burnt areas (−31.5%; −2.05 km2). As for grasslands, there was a slight loss of wet grasslands in unburnt areas (−2.4%; −0.01 km2) but dry grasslands cover expanded by 13.2 km2 (+53.6%; 0.66 km2 p.a.), which is less than the rate of change for both wet grasslands (+410.4%; +0.99 km2 p.a.) and dry grasslands (+1152.9%; +0.71 km2 p.a.) observed in burnt areas.
Decadal vegetation dynamics in areas of the Kosciuszko Alpine Area that remained unburnt during the 2003 wildfire with (a) the spatial distribution of vegetation types and (b) the flow of area covered by vegetation types through time. Vegetation and landcover types include subalpine woodlands (SW), dry heathlands (DH), wet heathlands (WH), dry grasslands (DG), wet grasslands (WG), fellfield screelands (FS) and rocky outcrops (RO). Burnt and unburnt areas detected using LandTrendr.
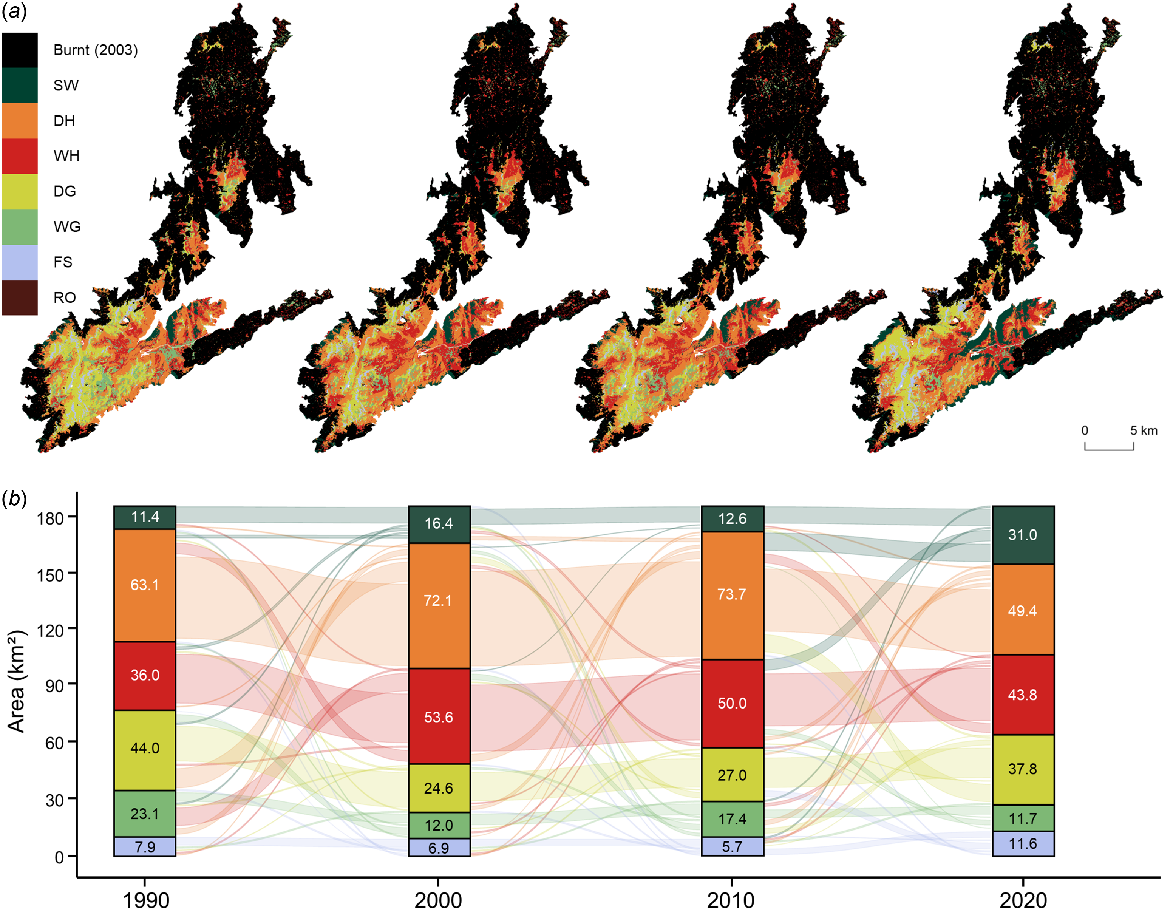
Vegetation zonation and dynamics from 1990 to 2020
Vegetation types displayed differences in the extent of cover along the elevation gradient (Fig. 7). Across the four observed growing seasons, the mean elevation weighted by area for subalpine woodlands was 1781.8 m a.s.l. (Table S7), with a consistent decline in area with increasing elevation. Above subalpine woodlands, there were less consistent elevation to area relationships aside from wet vegetation types covering lower elevations, including wet heathlands at 1819.5 m a.s.l and wet grasslands at 1862.7 m a.s.l. Dry vegetation types covered higher elevations with dry heathlands at 1869.7 m a.s.l and dry grasslands at 2017.3 m a.s.l. The vegetation type with the greatest mean elevation weighted by area was fellfield screelands at 2037.1 m a.s.l. However, there were also temporal dynamics in the area occupied by each vegetation type throughout the elevation gradient across the study area. Although the mean elevation weighted by area for subalpine woodlands decreased marginally from 1782.1 m in 1990 to 1779.6 m in 2020 (−0.1 m p.a.), largely due to densification via increasing cover at lower elevations, there was evidence of treeline advance between 1990 and 2000 (+0.4 m p.a.). There is also evidence of shrubline advance through time across the study area, with mean elevation weight by area of dry heathlands increasing from 1851.5 m in 1990 to 1885.0 m in 2020 (+1.1 m p.a.), with wet heathlands also increasing by 0.4 m p.a. during this period. Contrastingly, there has been a shift in the mean elevation weighted by area of dry grasslands (−0.4 m p.a.) and wet grasslands (−0.7 m p.a.) from 1990 to 2020.
Decadal vegetation type cover dynamics of the Kosciuszko Alpine Area throughout the elevation gradient, and the influence of wildfire on the spatiotemporal zonation of vegetation types. Area of each vegetation type was rounded to nearest 10 m interval to reduce noise and improve comprehensibility.
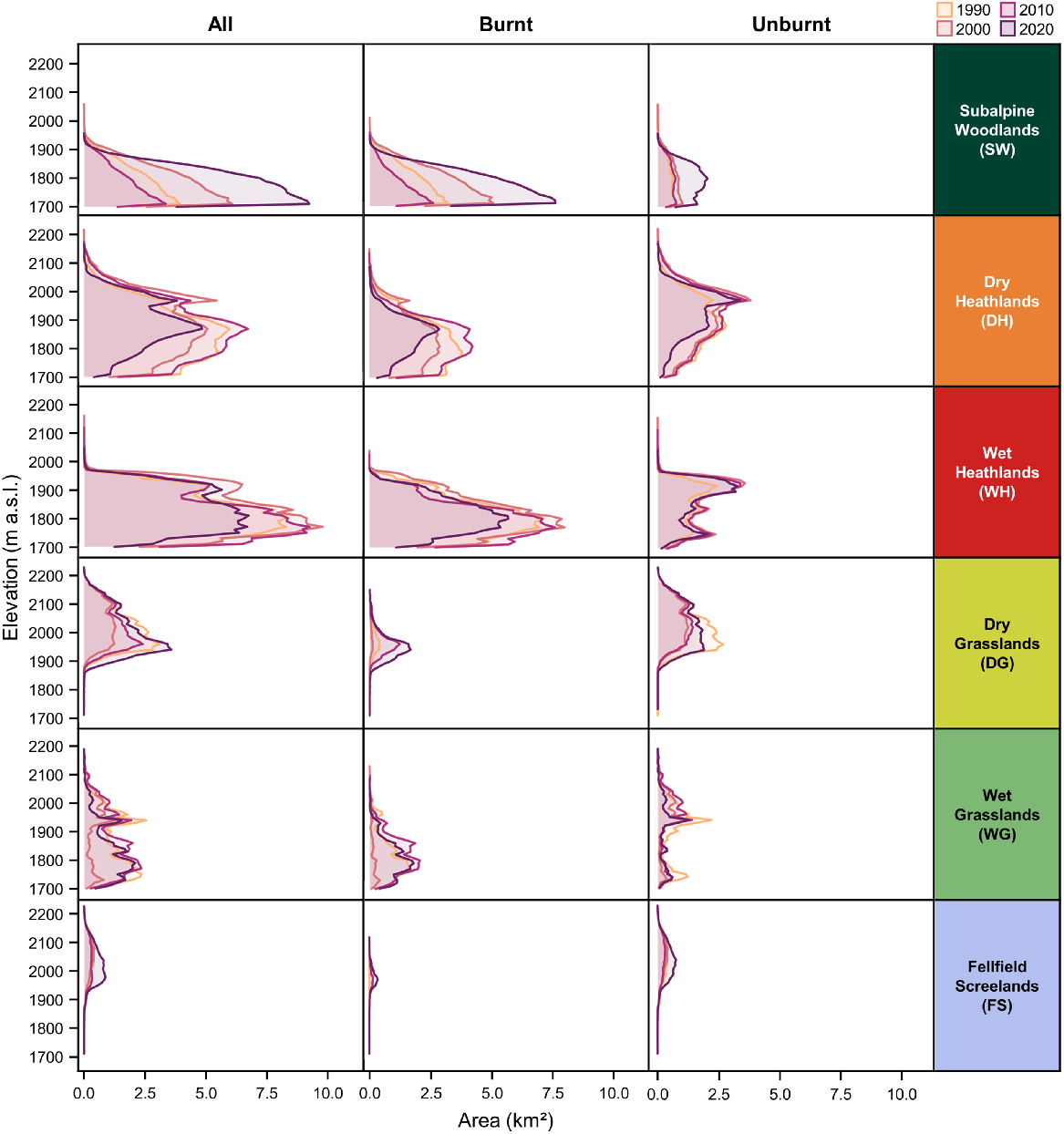
Burnt and unburnt vegetation zonation dynamics
Similar to varying vegetation cover dynamics in burnt and unburnt areas, the vegetation zonation dynamics differed over time (Fig. 7; Table S6). Based on the vegetation types present in 2000 and 2020, the mean elevation weighted by area of subalpine woodlands decreased in burnt (−0.4 m p.a.) and unburnt (−0.2 m p.a.) areas through increasing cover and densification at lower elevations. Dry heathlands in burnt areas have advanced through the elevation gradient from 2000 to 2020 at a rate of 0.2 m p.a., whereas this advance has been much faster in unburnt areas at 0.4 m p.a. during the same period. However, wet heathlands in burnt areas have remained relatively stable from 2000 to 2020 (−0.01 m p.a.) while advancing in unburnt areas (+0.2 m p.a.). As for grassland vegetation types, the mean elevation weighted by area for dry grasslands (+2.1 m p.a.) and wet grasslands (+5.9 m p.a.) increased between 1990 and 2000. However, there has been a consistent decline in elevation of dry grasslands (−1.81 m p.a.) and wet grasslands (−1.2 m p.a.) in burnt areas from 2000 to 2020 as the cover of these vegetation types increased at lower elevations after the 2003 wildfire. Similarly, fellfield screelands advanced upslope from 1990 to 2000 (+0.8 m p.a.) but there was an overall decline between 1990 and 2020 (−0.5 m p.a.), with greater declines in burnt areas (−2.3 m p.a.) in comparison to unburnt areas (−0.2 m p.a.).
Discussion
Linking past, present and future climate change
Rapidly increasing temperatures have been reported in numerous alpine areas around the world (Beniston et al. 1997; Hock et al. 2019), and are often compounded by elevation (Pepin et al. 2015). Over the past century, the study area has warmed by 1.10°C, which is in line with the broader climatic warming rate in south-eastern Australia of 1.11°C during the same period (Jones et al. 2009; Wahren et al. 2013; Bureau of Meteorology (BoM) 2022), and a rate of 1.2°C in the Eastern Italian Alps from 1836–2015 (Knoflach et al. 2021). Subsequently, there has been a rapid increase in soil temperatures in the study area over the past two decades (Verrall et al. 2021, 2023). Soil temperatures are often used as a surrogate for the microclimate experienced by alpine plants and are correlated to surface air temperatures. Similarly, rates of increasing temperatures have also been recorded over recent decades on the nearby Bogong High Plains (Wahren et al. 2013). The current rate of warming occurring in the Australian Alps is unprecedented in the past 2000 years, with temperatures observed from 1970 increasing 2.6 times faster than the previous fastest rate of warming during Medieval Climate Anomaly (950–1400 AD) (McGowan et al. 2018). This warming has also coincided with marked decline in snow cover since 1970, which is highlighted in the snow cover records presented in this study, to the lowest during the last 2000 years (McGowan et al. 2018). As temperatures have increased and snow cover has subsequently declined, growing seasons are becoming longer in the study area (Thompson 2016; Thompson and Paull 2017). Globally, similar declines in the snow cover have also been widely reported (Huss et al. 2017; Notarnicola 2020), with south-eastern Australia identified as a hotspot (Li et al. 2018). Although snow cover is known to be highly variable in the Australian Alps, there has been a marked decline in spring snow cover (Nicholls 2005; Bormann et al. 2012), and an overall decline in years with above average snow cover since 2000 (Rasouli et al. 2022). The recorded decline in snow cover since 1954 is primarily driven by increasing temperatures and declining winter precipitation, with higher temperatures accelerating snow melt and light snowfall events, and declining winter precipitation limiting snow accumulation (Fiddes et al. 2015; Pepler et al. 2015). Snow cover is also related to fluctuations in pertinent large-scaled climate drivers especially the El Niño-Southern Oscillation (ENSO), Southern Annular Mode (SAM) and Indian Ocean Dipole (IOD), which considerably influence temperatures and precipitation in the study area (Pepler et al. 2015; Rasouli et al. 2022).
In addition to the observed climate in the study over the past century, these climatic trends are projected to continue into the future (IPCC 2022). Similarly, alpine areas in the northern hemisphere are projected to experience comparable climatic changes in coming decades (Engler et al. 2011; Ernakovich et al. 2014). As the Australian Alps contain relatively low-elevation alpine areas at mid-latitudes and experience a mild, maritime mountain climate, they are likely to be among the most sensitive and vulnerable to changes in climate (Brown and Mote 2009; Di Luca et al. 2018). Downscaled climate models, as part of the New South Wales and Australian Capital Territory Regional Climate Modelling project (NARCliM), project a further warming of mean annual surface temperatures (~1.5–2°C) as well as declining winter (~15–30 mm) and spring (~45–60 mm) precipitation in the Australian Alps by 2070 from present day levels (1990–2009) (Olson et al. 2016). Concurrently, NARCliM modelling projects further increases of mean annual surface temperatures (~1.7–2.1°C), a 7% decline in annual precipitation and a further decline in snow cover in the Australian Alps by 15% and 60% by 2030 and 2070, respectively (Di Luca et al. 2018). Most of these projected changes are strongly linked to a strengthening subtropical ridge (Olson et al. 2016; Di Luca et al. 2018), in addition to a positive trend in SAM under high emission scenarios, leading to drier conditions in winter (Lim et al. 2016). These climatic changes are in line with global patterns, as snow, glaciers and permafrost are expected to decline in most regions throughout the 21st century (Hock et al. 2019).
Climate change is also likely to result in increasing frequency, severity and magnitude of extreme climatic events such as heatwaves and dangerous wildfire weather conditions (IPCC 2021). The warming and drying trend identified from 1975 to 2009 in the Australian Alps has led to considerable increases in the forest fire danger index (Bradstock et al. 2014; Dowdy 2018), and wildfire regimes are predicted to intensify in coming decades (Clarke and Evans 2019). An increase in the frequency of pyrocumulonimbus wildfires within the mountainous areas of south-eastern Australia is also expected as the climate continues to change (Di Virgilio et al. 2019), further increasing the risk of wildfires within the Australian Alps. As winter and spring precipitation, and consequently, snow cover declines and the growing seasons lengthen with warming temperatures, there is a clear trend toward increasing vegetation flammability and increasingly dangerous wildfire conditions during spring and summer in addition to an earlier start to the wildfire season in the Australian Alps (Clarke et al. 2013; Dowdy 2018; Zylstra 2018).
Climate- and fire-induced vegetation dynamics
Over the past 30 years, it is evident that alpine plants are on the move alongside rapid climatic changes that are occurring in the study area. The observed dynamics in cover and zonation of vegetation types correlated with wildfire impacts, particularly for the alpine treeline ecotone, with varying responses when comparing burnt and unburnt areas from the 2003 wildfire. As the risk and incidence of wildfire in high-elevation areas have increased with climate change, there has been growing interest in how wildfire may impact alpine treelines globally (Bader et al. 2008; Stine and Butler 2015; Cansler et al. 2016; Cansler et al. 2018; Wang et al. 2019), and in Australia (Green 2009; Naccarella et al. 2020). In the study area, there has been a considerable expansion of subalpine woodlands over the study period with cover expanding rapidly in burnt areas. Although there was little change in the zonation of the treeline in the study area, with an overall decline of −0.08 m per year, a single wildfire has apparently caused a decline in the treeline by a factor of 7.5 when compared to unburnt treeline. As the alpine treeline ecotone is primarily governed by thermal growth limitation (Körner 2012), it is widely accepted that climate change has caused the observed widespread advance in treelines globally (Harsch et al. 2009; Hansson et al. 2021). However, treeline dynamics are also dependent on seedling establishment and microclimatic factors dictated by snow cover, as well as solar and wind and frost exposure, which limit seedling survival above the current treeline (Smith et al. 2009; Harsch and Bader 2011; Hansson et al. 2021). These change dramatically in a post-wildfire landscape.
In the Australian Alps, treeline stasis amidst recent climate change has been attributed to the lack of an effective seed dispersal mechanism and low stature of the dominant E. niphophila, which relies primary on gravity and less commonly on windblown branches containing viable seed (Green 2009; Green and Venn 2012; Naccarella et al. 2020). In contrast, most treelines around the world are dominated by wind dispersed species that permit the establishment of seedlings above the current treeline, if climatic conditions permit (Körner 2012). However, a single wildfire seems to promote the establishment of E. niphophila seedlings at or below the treeline following the removal of competition from understory plants and loss of canopy (Green 2009; Green and Venn 2012; Naccarella et al. 2020). Wildfires can cause an abrupt change in microclimatic conditions that lead to accelerated snowmelt (Schwartz et al. 2020a, 2021), which may further promote seedling establishment (Green and Venn 2012). Although the 2003 wildfire caused the widespread loss of E. niphophila canopy, over 95% of trees survived and resprouted from lignotubers leading to lower-growing trees with a mallee form (Pickering and Barry 2005). Ultimately, a single wildfire has resulted in increasing cover of subalpine woodlands via densification, as E. niphophila established as the dominant vegetation over smaller stature vegetation types, with grasslands and heathlands cover faster to recover following wildfire (Camac et al. 2013; McDougall et al. 2015; Camac et al. 2017). Wildfire has also effectively resulted in the densification of subalpine woodlands at lower elevations, as E. niphophila regenerate from lignotubers to form mallee stands; and seedlings germinate at higher rates following wildfire. However E. niphophila lacks effective dispersal mechanisms to establish above the current treeline, even if climatic, abiotic and biotic conditions are favourable in a post-wildfire landscape (Green and Venn 2012).
There were also important dynamics of heathlands and grasslands in burnt areas. Generally, there was a reduction in cover of both dry and wet heathlands in areas that burnt in 2003. Dry heathlands recovered swiftly following wildfire at lower elevations, as heathlands in the Australian Alps are resilient to infrequent wildfire (Camac et al. 2013; McDougall et al. 2015), and it can promote increased seedling establishment of a dominant alpine shrub Grevillea australis (Camac et al. 2017). Surprisingly, the cover of fellfield screelands increased over time, but this may be attributed to the small tracts that burnt in the 2020 wildfires that left soils exposed. Furthermore, increasing cover of fellfield screelands could also be the result of drought impacts on dry grasslands including amplified senescence and dieback from phytophagous moth larvae (Scherrer and Pickering 2005; McDougall et al. 2018). The decline of wet heathlands may be attributed to their well-established vulnerability to wildfire, with considerable losses of important and defining structural species following the 2003 wildfire, as underlying peat burnt down to mineral soils (McDougall et al. 2015). However, large tracts of heathlands were replaced by grasslands in burnt areas at higher elevations in the study area, causing the suppression of shrubline advance by a factor of approximately 2.5 when compared to unburnt areas. Following the 2003 wildfire, dry and wet grasslands were the first to recover in terms of vegetative cover (McDougall et al. 2015), and were dominated by competitive, tussock-forming graminoids. Concurrently, the relatively high flammability of heathlands led to high temperature burns and subsequently, recovered much slower than surrounding grasslands (Williams et al. 2006; McDougall et al. 2015). Similarly, in high-elevation paramo grasslands of South America, fire has been shown to suppress shrub advance and encroachment (Matson and Bart 2013; Zomer and Ramsay 2021). However, most research in the Australian Alps suggests the encroachment of dry heathlands on grasslands following wildfire (Camac et al. 2013, 2015, 2017), but these studies were mostly conducted at lower elevations below or at treeline (~1750 m a.s.l.) and focus on less than a decade of recovery. Overall, alpine vegetation in the Australian Alps is resilient to large, infrequent wildfires (Williams et al. 2008; Kirkpatrick and Bridle 2013; Venn et al. 2016), apart from cryophilic periglacial communities (Verrall 2018), but frequent wildfires may lower this resilience (Fairman et al. 2017; Naccarella et al. 2020).
In contrast, attribution of climate-induced dynamics without the influence of wildfire can be assessed in the unburnt areas. Over the study period, there was an increase in the cover subalpine woodlands by 0.65 km2 per year with treeline stasis. As the climate warms and growing seasons lengthen, the densification and in-filling processes at the alpine treeline ecotone without treeline advancement have been widely observed across the world (Shi et al. 2022). Globally, climate change has promoted treeline densification via increased seed germination, seedling establishment and tree growth (Körner 2012; Lett and Dorrepaal 2018). In the Australian Alps, there is some evidence of treeline densification in unburnt areas (Green 2009; Green and Venn 2012), with stands not recruitment-limited as there are several established saplings and young trees relative to older trees (Naccarella et al. 2020). There was also an advance of the dry shrubline by 1.24 m per year in unburnt areas over the study period. Although increasing shrub cover and densification has occurred in many alpine regions around the world (Wilson and Nilsson 2009; Brandt et al. 2013; Myers-Smith et al. 2015; Verrall et al. 2021), shrubline advance has also been recorded in the northern hemisphere (Hallinger et al. 2010; Dial et al. 2016; Myers-Smith and Hik 2018; Wang et al. 2021). Overall, the expansion of woody vegetation types in unburnt areas ultimately occurred as they encroached on dry and wet grasslands. A study of aerial photography of similar vegetation in Victoria between 1936 and 1980 also found an increase in the cover of woody vegetation types and subsequent decline in grasslands (McDougall 2003), which aligns with the more recent dynamics in this study area. Furthermore, the phenomena of woody encroachment and increasing competition from thermophilic growth forms occurring in the study area are in line with climate-induced responses of alpine vegetation in the northern hemisphere (Erschbamer et al. 2011; Lamprecht et al. 2018; Scharnagl et al. 2019; Malfasi and Cannone 2020). While alpine plants are on the move in response to climate change in the Australian Alps (Verrall et al. 2021; Auld et al. 2022), there may be dispersal lags and growing extinction debt for long-lived perennials that are unlikely to keep pace with the rapid rate of warming (Alexander et al. 2018; Zimmer et al. 2018).
Implications, benefits, limitations and future research
Globally, climate change has resulted in increasing vegetation biomass in alpine areas via densification of treelines and woody encroachment of grasslands. These responses in vegetation are a reflection of rising temperatures, declining snow cover and increased precipitation variability. The combination of these changing biotic and abiotic factors (especially decreasing winter rainfall and increasing spring temperatures) ultimately results in more severe wildfire weather conditions and increased landscape flammability (Zylstra 2013, 2018), which has considerable implications in the Australian Alps and other fire-prone high-elevation areas as wildfire may become a more frequent and influential agent of change (Hock et al. 2019; Thomas et al. 2022). Before 2003, the last landscape-level wildfire in the study area occurred 64 years prior in 1939 where ~34% of the study area burnt, in addition to a smaller tract (~4% of study area) of the western escarpment burning in 1973 (Williams et al. 2008; NSW Government 2022). However, there was potential for the 2020 black summer wildfires to cause widespread burning of alpine vegetation in the Australian Alps, shortening the interval between severe widespread burns to just 17 years following the 2003 wildfires. Furthermore, global warming has the potential to strengthen positive feedback loops between wildfire and woody encroachment (Camac et al. 2017). Post-wildfire landscapes have been shown to alter microclimate by amplifying soil and vegetation temperatures, leading to accelerated snowmelt, runoff and erosion (Schwartz et al. 2020a, 2021), which is likely to impact the establishment and recovery of alpine vegetation following wildfire into the future. Altered hydrology in alpine catchments because of increasing frequency, severity and magnitude of wildfires may have considerable ecological and socio-economic consequences as climate trends continue (Gallucci et al. 2011; IPCC 2021). Increasing dangerous wildfire weather conditions and the flammability of alpine ecosystem vegetation poses an immediate threat to cryophilic and periglacial communities and species that are vulnerable to wildfire (Venn et al. 2017; Verrall 2018), but increases in the frequency and intensity of wildfire regimes may result in changes in the resilience of previously-thought fire-tolerant vegetation leading to ecosystem transition or collapse (Fairman et al. 2017; Lindenmayer and Taylor 2020; Naccarella et al. 2020).
Limitations of this study include that analysis were focused on broad vegetation types and not the composite individual species, which can vary in their life history traits and responses to changes in climate conditions and wildfire regimes (McDougall et al. 2015). Although this study used the most sophisticated climate products available for this study area, the establishment and maintenance of multiple weather stations and snow courses in Australia’s largest alpine area is vitally important to understanding the impacts of climate change into the future (Fiddes et al. 2015). This study was the first to use remotely sensed times series data to study alpine vegetation dynamics in Australia and high classification accuracy was achieved. Future research would benefit from complementary field data measurements, not withstanding the logistical difficulties this presents. Furthermore, the 30-year period where vegetation dynamics were assessed spanned considerable year-to-year and decadal variability in climatic conditions including drought preceding the 2003 wildfire. Thus, further research is needed on the impacts of this intra-decadal climatic variability on vegetation dynamics. More detailed studies are also warranted on subalpine woodland mortality and establishment through surveying burnt and unburnt alpine areas along with comparisons of soil seedbanks and soil properties (Pickering and Barry 2005; Naccarella et al. 2020). Given the rapid rate of change, there is a need for continued monitoring of shifts in the extent, density and zonation of the major alpine vegetation types in Australia.
Conclusion
Examining recent changes in temperature, precipitation, and snow cover over the past century in conjunction with climate-induced alpine vegetation dynamics that were modelled using optimised random forest classification of Landsat time series, provided novel insights into how the major vegetation types in the highest and most diverse alpine area in Australia are responding to climate change. Furthermore, this study applied a robust and cost-effective methodology that makes use of widely available remotely sensed and ancillary data, which could be readily applied to monitor similar climate-induced changes in other alpine areas globally including trends in treeline densification, woody encroachment and advancement. Alpine ecosystems are particularly sensitive to warming temperatures, varying precipitation regimes and declining snow cover, demonstrating relatively incremental vegetation responses in addition to transformative changes that occur after large fire-type disturbance events. The interaction and feedbacks between climate change, densification woody encroachment, and landscape flammability may present as a crucial aspect in understanding the responses of alpine vegetation globally to climate change.
Data availability
Data sets utilised for this research are all publicly available and were retrieved from the Australian Gridded Climate Data (AGCD v1.0.0), the Snowy Hydro Project and the United States Geological Survey (USGS) Landsat Program via the Google Earth Engine data catalogue. Query details are described in the methods. Code and data utilised for this research are provided for peer review via a GitHub repository.
Acknowledgements
We acknowledge the Ngarigo, Walgalu, Djilamatang, Ngunnawal and Kombumerri as First Nations and Traditional Custodians of the land on which research for this study was focused and conducted. We pay our respect to Elders past and present, and recognise the continuing connection to land, sea and culture, and that sovereignty was never ceded.
References
Alatalo JM, Jagerbrand AK, Molau U (2016) Impacts of different climate change regimes and extreme climatic events on an alpine meadow community. Scientific Reports 6, 21720.
| Crossref | Google Scholar |
Alexander JM, Chalmandrier L, Lenoir J, Burgess TI, Essl F, Haider S, Kueffer C, McDougall K, Milbau A, Nuñez MA, Pauchard A, Rabitsch W, Rew LJ, Sanders NJ, Pellissier L (2018) Lags in the response of mountain plant communities to climate change. Global Change Biology 24, 563-579.
| Crossref | Google Scholar |
Allaire JJ, Ellis P, Gandrud C, Huo K, Lewis BW, Owen J, Russell K, Rogers J, Sese C, Yetman CJ (2017) networkD3: D3 JavaScript Network Graphs from R. R package version 0.4. Available at https://cran.r-project.org/web/packages/networkD3/networkD3.pdf
Auld J, Everingham SE, Hemmings FA, Moles AT (2022) Alpine plants are on the move: quantifying distribution shifts of Australian alpine plants through time. Diversity and Distributions 28, 943-955.
| Crossref | Google Scholar |
Bader MY, Rietkerk M, Bregt AK (2008) A simple spatial model exploring positive feedbacks at tropical alpine treelines. Arctic Antarctic and Alpine Research 40, 269-278.
| Crossref | Google Scholar |
Banskota A, Kayastha N, Falkowski MJ, Wulder MA, Froese RE, White JC (2014) Forest monitoring using Landsat time series data: a review. Canadian Journal of Remote Sensing 40, 362-384.
| Crossref | Google Scholar |
Belgiu M, Drăguţ L (2016) Random forest in remote sensing: a review of applications and future directions. ISPRS Journal of Photogrammetry and Remote Sensing 114, 24-31.
| Crossref | Google Scholar |
Beniston M, Diaz HF, Bradley RS (1997) Climatic change at high elevation sites: an overview. Climatic Change 36, 233-251.
| Crossref | Google Scholar |
Blatchford ML, Mannaerts CM, Zeng Y (2021) Determining representative sample size for validation of continuous, large continental remote sensing data. International Journal of Applied Earth Observation and Geoinformation 94, 102235.
| Crossref | Google Scholar |
Bormann KJ, McCabe MF, Evans JP (2012) Satellite based observations for seasonal snow cover detection and characterisation in Australia. Remote Sensing of Environment 123, 57-71.
| Crossref | Google Scholar |
Bradstock R, Penman T, Boer M, Price O, Clarke H (2014) Divergent responses of fire to recent warming and drying across south-eastern Australia. Global Change Biology 20, 1412-1428.
| Crossref | Google Scholar |
Brandt JS, Haynes MA, Kuemmerle T, Waller DM, Radeloff VC (2013) Regime shift on the roof of the world: Alpine meadows converting to shrublands in the southern Himalayas. Biological Conservation 158, 116-127.
| Crossref | Google Scholar |
Breiman L (2001) Random forests. Machine Learning 45, 5-32.
| Crossref | Google Scholar |
Breiman L, Cutler A, Liaw A, Wiener M (2022) randomForest: Breiman and Cutler’s Random Forests for Classification and Regression. R package version 4.7-1.1. Available at https://cran.r-project.org/web/packages/randomForest/randomForest.pdf
Brown RD, Mote PW (2009) The response of northern hemisphere snow cover to a changing climate. Journal of Climate 22, 2124-2145.
| Crossref | Google Scholar |
Bureau of Meteorology (BoM) (2022) Australian climate variability & change - Time series graphs - Southeastern Australia. (Australian Government) Available at http://www.bom.gov.au/climate/change/#tabs=Tracker&tracker=timeseries&tQ=graph%3Dtmean%26area%3Dsaus%26season%3D0112%26ave_yr%3D0
Camac JS, Williams RJ, Wahren C-H, Morris WK, Morgan JW (2013) Post-fire regeneration in alpine heathland: does fire severity matter? Austral Ecology 38, 199-207.
| Crossref | Google Scholar |
Camac JS, Williams RJ, Wahren C-H, Jarrad F, Hoffmann AA, Vesk PA (2015) Modeling rates of life form cover change in burned and unburned alpine heathland subject to experimental warming. Oecologia 178, 615-628.
| Crossref | Google Scholar |
Camac JS, Williams RJ, Wahren C-H, Hoffmann AA, Vesk PA (2017) Climatic warming strengthens a positive feedback between alpine shrubs and fire. Global Change Biology 23, 3249-3258.
| Crossref | Google Scholar |
Cansler CA, McKenzie D, Halpern CB (2016) Area burned in alpine treeline ecotones reflects region-wide trends. International Journal of Wildland Fire 25, 1209-1220.
| Crossref | Google Scholar |
Cansler CA, McKenzie D, Halpern CB (2018) Fire enhances the complexity of forest structure in alpine treeline ecotones. Ecosphere 9, e02091.
| Crossref | Google Scholar |
Carbognani M, Tomaselli M, Petraglia A (2014) Current vegetation changes in an alpine late snowbed community in the south-eastern Alps (N-Italy). Alpine Botany 124, 105-113.
| Crossref | Google Scholar |
Chhetri PK, Thai E (2019) Remote sensing and geographic information systems techniques in studies on treeline ecotone dynamics. Journal of Forestry Research 30, 1543-1553.
| Crossref | Google Scholar |
Clarke H, Evans JP (2019) Exploring the future change space for fire weather in southeast Australia. Theoretical and Applied Climatology 136, 513-527.
| Crossref | Google Scholar |
Clarke H, Lucas C, Smith P (2013) Changes in Australian fire weather between 1973 and 2010. International Journal of Climatology 33, 931-944.
| Crossref | Google Scholar |
Danby RK, Koh S, Hik DS, Price LW (2011) Four decades of plant community change in the alpine tundra of Southwest Yukon, Canada. Ambio 40, 660-671.
| Crossref | Google Scholar |
De Boeck HJ, Bassin S, Verlinden M, Zeiter M, Hiltbrunner E (2016) Simulated heat waves affected alpine grassland only in combination with drought. New Phytologist 209, 531-541.
| Crossref | Google Scholar |
De Boeck HJ, Hiltbrunner E, Verlinden M, Bassin S, Zeiter M (2018) Legacy effects of climate extremes in alpine grassland. Frontiers in Plant Science 9, 1586.
| Crossref | Google Scholar |
de Witte LC, Stöcklin J (2010) Longevity of clonal plants: why it matters and how to measure it. Annals of Botany 106, 859-870.
| Crossref | Google Scholar |
Di Luca A, Evans JP, Ji F (2018) Australian snowpack in the NARCliM ensemble: evaluation, bias correction and future projections. Climate Dynamics 51, 639-666.
| Crossref | Google Scholar |
Di Virgilio G, Evans JP, Blake SAP, Armstrong M, Dowd AJ, Sharples J, McRae R (2019) Climate change increases the potential for extreme wildfires. Geophysical Research Letters 46, 8517-8526.
| Crossref | Google Scholar |
Dial RJ, Smeltz TS, Sullivan PF, Rinas CL, Timm K, Geck JE, Tobin SC, Golden TS, Berg EC (2016) Shrubline but not treeline advance matches climate velocity in montane ecosystems of south-central Alaska. Global Change Biology 22, 1841-1856.
| Crossref | Google Scholar |
Dirnböck T, Essl F, Rabitsch W (2011) Disproportional risk for habitat loss of high-altitude endemic species under climate change. Global Change Biology 17, 990-996.
| Crossref | Google Scholar |
Dodson JR, de Salis T, Myers CA, Sharp AJ (1994) A thousand years of environmental change and human impact in the alpine zone at Mt Kosciusko, New South Wales. Australian Geographer 25, 77-87.
| Crossref | Google Scholar |
Dowdy AJ (2018) Climatological variability of fire weather in Australia. Journal of Applied Meteorology and Climatology 57, 221-234.
| Crossref | Google Scholar |
Elsen PR, Tingley MW (2015) Global mountain topography and the fate of montane species under climate change. Nature Climate Change 5, 772-776.
| Crossref | Google Scholar |
Engler R, Randin CF, Thuiller W, et al. (2011) 21st century climate change threatens mountain flora unequally across Europe. Global Change Biology 17, 2330-2341.
| Crossref | Google Scholar |
Ernakovich JG, Hopping KA, Berdanier AB, Simpson RT, Kachergis EJ, Steltzer H, Wallenstein MD (2014) Predicted responses of arctic and alpine ecosystems to altered seasonality under climate change. Global Change Biology 20, 3256-3269.
| Crossref | Google Scholar |
Erschbamer B, Unterluggauer P, Winkler E, Mallaun M (2011) Changes in plant species diversity revealed by long-term monitoring on mountain summits in the Dolomites (northern Italy). Preslia 83, 387-401.
| Google Scholar |
Fairman TA, Bennett LT, Tupper S, Nitschke CR (2017) Frequent wildfires erode tree persistence and alter stand structure and initial composition of a fire-tolerant sub-alpine forest. Journal of Vegetation Science 28, 1151-1165.
| Crossref | Google Scholar |
Fiddes SL, Pezza AB, Barras V (2015) A new perspective on Australian snow. Atmospheric Science Letters 16, 246-252.
| Crossref | Google Scholar |
Filippa G, Cremonese E, Galvagno M, Bayle A, Choler P, Bassignana M, Piccot A, Poggio L, Oddi L, Gascoin S, Costafreda-Aumedes S, Argenti G, Dibari C (2022) On the distribution and productivity of mountain grasslands in the Gran Paradiso National Park, NW Italy: a remote sensing approach. International Journal of Applied Earth Observation and Geoinformation 108, 102718.
| Crossref | Google Scholar |
Fraser IP, Williams RJ, Murphy BP, Camac JS, Vesk PA (2016) Fuels and landscape flammability in an Australian alpine environment. Austral Ecology 41, 657-670.
| Crossref | Google Scholar |
Gallucci J, Tryhorn L, Lynch A, Parkyn K (2011) On the meteorological and hydrological mechanisms resulting in the 2003 post-fire flood event in Alpine Shire, Victoria. Australian Meteorological and Oceanographic Journal 61, 31-42.
| Crossref | Google Scholar |
Georganos S, Grippa T, Vanhuysse S, Lennert M, Shimoni M, Kalogirou S, Wolff E (2018) Less is more: optimizing classification performance through feature selection in a very-high-resolution remote sensing object-based urban application. GIScience & Remote Sensing 55, 221-242.
| Crossref | Google Scholar |
Gislason PO, Benediktsson JA, Sveinsson JR (2006) Random Forests for land cover classification. Pattern Recognition Letters 27, 294-300.
| Crossref | Google Scholar |
Grabherr G, Gottfried M, Pauli H (2010) Climate change impacts in alpine environments. Geography Compass 4, 1133-1153.
| Crossref | Google Scholar |
Green K (2009) Causes of stability in the alpine treeline in the Snowy Mountains of Australia – a natural experiment. Australian Journal of Botany 57, 171-179.
| Crossref | Google Scholar |
Green K, Pickering CM (2009) Vegetation, microclimate and soils associated with the latest-lying snowpatches in Australia. Plant Ecology and Diversity 2, 289-300.
| Crossref | Google Scholar |
Green K, Stein JA (2015) Modeling the thermal zones and biodiversity on the high mountains of meganesia: the importance of local differences. Arctic Antarctic and Alpine Research 47, 671-680.
| Crossref | Google Scholar |
Green K, Venn S (2012) Tree-limit ribbons in the Snowy Mountains, Australia: characterization and recent seedling establishment. Arctic Antarctic and Alpine Research 44, 180-187.
| Crossref | Google Scholar |
Gregorutti B, Michel B, Saint-Pierre P (2017) Correlation and variable importance in random forests. Statistics and Computing 27, 659-678.
| Crossref | Google Scholar |
Guan H, Li J, Chapman M, Deng F, Ji Z, Yang X (2013) Integration of orthoimagery and lidar data for object-based urban thematic mapping using random forests. International Journal of Remote Sensing 34, 5166-5186.
| Crossref | Google Scholar |
Guisan AA, Broennimann O, Buri A, Cianfrani C, Di Cola V, Fernandes R, Gray SM, Mateo RG, Pinto E, Praderv JN, Scherrer D, Vittoz P, Von Däniken I, Yashiro E (2019) Climate change impacts on mountain biodiversity. In ‘Biodiversity and climate change: transforming the biosphere’. (Eds TE Lovejoy, L Hannah) pp. 221–233. (Yale University Press: New Haven, CT, USA)
Hallinger M, Manthey M, Wilmking M (2010) Establishing a missing link: warm summers and winter snow cover promote shrub expansion into alpine tundra in Scandinavia. New Phytologist 186, 890-899.
| Crossref | Google Scholar |
Hammond TO, Verbyla DL (1996) Optimistic bias in classification accuracy assessment. International Journal of Remote Sensing 17, 1261-1266.
| Crossref | Google Scholar |
Hansen MC, Potapov PV, Moore R, Hancher M, Turubanova SA, Tyukavina A, Thau D, Stehman SV, Goetz SJ, Loveland TR, Kommareddy A, Egorov A, Chini L, Justice CO, Townshend JRG (2013) High-resolution global maps of 21st-century forest cover change. Science 342, 850-853.
| Crossref | Google Scholar |
Hansson A, Dargusch P, Shulmeister J (2021) A review of modern treeline migration, the factors controlling it and the implications for carbon storage. Journal of Mountain Science 18, 291-306.
| Crossref | Google Scholar |
Harsch MA, Bader MY (2011) Treeline form – a potential key to understanding treeline dynamics. Global Ecology and Biogeography 20, 582-596.
| Crossref | Google Scholar |
Harsch MA, Hulme PE, McGlone MS, Duncan RP (2009) Are treelines advancing? A global meta-analysis of treeline response to climate warming. Ecology Letters 12, 1040-1049.
| Crossref | Google Scholar |
Henrich V, Götze E, Jung A, Sandow C, Thürkow D, Gläßer C (2009) Development of an online indices database: motivation, concept and implementation. In ‘Proceedings of the 6th EARSeL imaging spectroscopy sig workshop innovative tool for scientific and commercial environment applications’, Tel Aviv, Israel, 16–18 March 2009. (European Association of Remote Sensing Laboratories: Münster, Germany)
Hock R, Rasul G, Adler C, Cáceres B, Gruber S, Hirabayashi Y, Jackson M, Kääb A, Kang S, Kutuzov S, Milner A, Molau U, Morin S, Orlove B, Steltzer H (2019) High mountain areas. In ‘IPCC special report on the ocean and cryosphere in a changing climate’. (Eds HO Pörtner, DC Roberts, V Masson-Delmotte, et al.) pp. 131–202. (Intergovernmental Panel on Climate Change [IPCC]: Geneva, Switzerland)
Huss M, Bookhagen B, Huggel C, Jacobsen D, Bradley RS, Clague JJ, Vuille M, Buytaert W, Cayan DR, Greenwood G, Mark BG, Milner AM, Weingartner R, Winder M (2017) Toward mountains without permanent snow and ice. Earth’s Future 5, 418-435.
| Crossref | Google Scholar |
Jones DA, Wang W, Fawcett R (2009) High-quality spatial climate data-sets for Australia. Australian Meteorological and Oceanographic Journal 58, 233-248.
| Crossref | Google Scholar |
Kennedy RE, Cohen WB, Schroeder TA (2007) Trajectory-based change detection for automated characterization of forest disturbance dynamics. Remote Sensing of Environment 110, 370-386.
| Crossref | Google Scholar |
Kennedy RE, Yang ZG, Cohen WB (2010) Detecting trends in forest disturbance and recovery using yearly Landsat time series: 1. LandTrendr – temporal segmentation algorithms. Remote Sensing of Environment 114, 2897-2910.
| Crossref | Google Scholar |
Kennedy RE, Yang Z, Gorelick N, Braaten J, Cavalcante L, Cohen WB, Healey S (2018) Implementation of the LandTrendr algorithm on Google Earth Engine. Remote Sensing 10, 691.
| Crossref | Google Scholar |
Khatami R, Mountrakis G, Stehman SV (2016) A meta-analysis of remote sensing research on supervised pixel-based land-cover image classification processes: general guidelines for practitioners and future research. Remote Sensing of Environment 177, 89-100.
| Crossref | Google Scholar |
Kirkpatrick JB, Bridle KL (2013) Natural and cultural histories of fire differ between Tasmanian and mainland Australian alpine vegetation. Australian Journal of Botany 61, 465-474.
| Crossref | Google Scholar |
Knoflach B, Ramskogler K, Talluto M, Hofmeister F, Haas F, Heckmann T, Pfeiffer M, Piermattei L, Ressl C, Wimmer MH, Geitner C, Erschbamer B, Stoetter J (2021) Modelling of vegetation dynamics from satellite time series to determine proglacial primary succession in the course of global warming – a case study in the Upper Martell Valley (Eastern Italian Alps). Remote Sensing 13, 4450.
| Crossref | Google Scholar |
Körner C (2004) Mountain biodiversity, its causes and function. Ambio 33, 11-17.
| Crossref | Google Scholar |
Kuhn M, Wing J, Weston S, Williams A, Keefer C, Engelhardt A, Cooper T, Mayer Z, Kenkel B, Benesty M, Lescarbeau R, Ziem A, Scrucca L, Tang Y, Candan C, Hunt T (2022) caret: Classification and regression training. R package version 6.0-92. Available at https://cran.r-project.org/web/packages/caret/caret.pdf
Lamprecht A, Semenchuk PR, Steinbauer K, Winkler M, Pauli H (2018) Climate change leads to accelerated transformation of high-elevation vegetation in the central Alps. New Phytologist 220, 447-459.
| Crossref | Google Scholar |
Lett S, Dorrepaal E (2018) Global drivers of tree seedling establishment at alpine treelines in a changing climate. Functional Ecology 32, 1666-1680.
| Crossref | Google Scholar |
Li Q, Ma M, Wu X, Yang H (2018) Snow cover and vegetation-induced decrease in global albedo from 2002 to 2016. Journal of Geophysical Research: Atmospheres 123, 124-138.
| Crossref | Google Scholar |
Li Y, Wu Z, Xu X, Fan H, Tong X, Liu J (2021) Forest disturbances and the attribution derived from yearly Landsat time series over 1990–2020 in the Hengduan Mountains Region of Southwest China. Forest Ecosystems 8, 73.
| Crossref | Google Scholar |
Lim E-P, Hendon HH, Arblaster JM, Delage F, Nguyen H, Min S-K, Wheeler MC (2016) The impact of the Southern Annular Mode on future changes in Southern Hemisphere rainfall. Geophysical Research Letters 43, 7160-7167.
| Crossref | Google Scholar |
Lin X, Chen J, Lou P, Yi S, Qin Y, You H, Han X (2021) Improving the estimation of alpine grassland fractional vegetation cover using optimized algorithms and multi-dimensional features. Plant Methods 17, 96.
| Crossref | Google Scholar |
Lindenmayer DB, Taylor C (2020) New spatial analyses of Australian wildfires highlight the need for new fire, resource, and conservation policies. Proceedings of the National Academy of Sciences 117, 12481-12485.
| Crossref | Google Scholar |
Liu X, Feng S, Liu H, Ji J (2021) Patterns and determinants of woody encroachment in the eastern Eurasian steppe. Land Degradation & Development 32, 3536-3549.
| Crossref | Google Scholar |
Ma L, Li M, Ma X, Cheng L, Du P, Liu Y (2017) A review of supervised object-based land-cover image classification. ISPRS Journal of Photogrammetry and Remote Sensing 130, 277-293.
| Crossref | Google Scholar |
Mackey B, Jacobs P, Hugh S (2015) Classifying and mapping the Australian Alps’ native vegetation. Cunninghamia 15, 185-199.
| Google Scholar |
Mackey B, Lindenmayer D, Norman P, Taylor C, Gould S (2021) Are fire refugia less predictable due to climate change? Environmental Research Letters 16, 114028.
| Crossref | Google Scholar |
Malfasi F, Cannone N (2020) Climate warming persistence triggered tree ingression after shrub encroachment in a high alpine tundra. Ecosystems 23, 1657-1675.
| Crossref | Google Scholar |
Martin ARH (1986) Late glacial and holocene alpine pollen diagrams from the Kosciusko National Park, New South Wales, Australia. Review of Palaeobotany and Palynology 47, 367-409.
| Crossref | Google Scholar |
Martin ARH (1999) Pollen analysis of Digger’s Creek Bog, Kosciuszko National Park: Vegetation history and tree-line change. Australian Journal of Botany 47, 725-744.
| Crossref | Google Scholar |
Matson E, Bart D (2013) Interactions among fire legacies, grazing and topography predict shrub encroachment in post-agricultural paramo. Landscape Ecology 28, 1829-1840.
| Crossref | Google Scholar |
McCaffrey DR, Hopkinson C (2020) Modeling watershed-scale historic change in the alpine treeline ecotone using random forest. Canadian Journal of Remote Sensing 46, 715-732.
| Crossref | Google Scholar |
McDougall KL (2003) Aerial photographic interpretation of vegetation changes on the Bogong High Plains, Victoria, between 1936 and 1980. Australian Journal of Botany 51, 251-256.
| Crossref | Google Scholar |
McDougall KL, Walsh NG (2007) Treeless vegetation of the Australian Alps. Cunninghamia 10, 1-57.
| Google Scholar |
McDougall KL, Walsh NG, Wright GT (2015) Recovery of treeless subalpine vegetation in Kosciuszko National Park after the landscape-scale fire of 2003. Australian Journal of Botany 63, 597-607.
| Crossref | Google Scholar |
McDougall KL, Wright GT, Burgess TI, Farrow R, Khaliq I, Laurence MH, Wallenius T, Liew ECY (2018) Plant, invertebrate and pathogen interactions in Kosciuszko National Park. Proceedings of the Linnean Society of New South Wales 140, 295-312.
| Google Scholar |
McGowan H, Callow JN, Soderholm J, McGrath G, Campbell M, Zhao JX (2018) Global warming in the context of 2000 years of Australian alpine temperature and snow cover. Scientific Reports 8, 4394.
| Crossref | Google Scholar |
Meng B, Zhang Y, Yang Z, Lv Y, Chen J, Li M, Sun Y, Zhang H, Yu H, Zhang J, Lian J, He M, Li J, Yu H, Chang L, Yi S (2022) Mapping grassland classes using unmanned aerial vehicle and MODIS NDVI data for temperate grassland in Inner Mongolia, China. Remote Sensing 14, 2094.
| Crossref | Google Scholar |
Mienna IM, Klanderud K, Ørka HO, Bryn A, Bollandsås OM (2022) Land cover classification of treeline ecotones along a 1100 km latitudinal transect using spectral- and three-dimensional information from UAV-based aerial imagery. Remote Sensing in Ecology and Conservation 8, 536-550.
| Crossref | Google Scholar |
Millard K, Richardson M (2015) On the importance of training data sample selection in random forest image classification: a case study in peatland ecosystem mapping. Remote Sensing 7, 8489-8515.
| Crossref | Google Scholar |
Mu X, Hu M, Song W, Ruan G, Ge Y, Wang J, Huang S, Yan G (2015) Evaluation of sampling methods for validation of remotely sensed fractional vegetation cover. Remote Sensing 7, 16164-16182.
| Crossref | Google Scholar |
Myers-Smith IH, Hik DS (2018) Climate warming as a driver of tundra shrubline advance. Journal of Ecology 106, 547-560.
| Crossref | Google Scholar |
Myers-Smith IH, Elmendorf SC, Beck PSA, et al. (2015) Climate sensitivity of shrub growth across the tundra biome. Nature Climate Change 5, 887-891.
| Crossref | Google Scholar |
Naccarella A, Morgan JW, Cutler SC, Venn SE (2020) Alpine treeline ecotone stasis in the face of recent climate change and disturbance by fire. PLoS ONE 15, e0231339.
| Crossref | Google Scholar |
Nicholls N (2005) Climate variability, climate change and the Australian snow season. Australian Meteorological Magazine 54, 177-185.
| Google Scholar |
Notarnicola C (2020) Hotspots of snow cover changes in global mountain regions over 2000–2018. Remote Sensing of Environment 243, 111781.
| Crossref | Google Scholar |
Olson R, Evans JP, Di Luca A, Argueso D (2016) The NARCliM project: model agreement and significance of climate projections. Climate Research 69, 209-227.
| Crossref | Google Scholar |
Pauli H, Gottfried M, Dullinger S, et al. (2012) Recent plant diversity changes on Europe’s mountain summits. Science 336, 353-355.
| Crossref | Google Scholar |
Pepin N, Bradley RS, Diaz HF, Baraer M, Caceres EB, Forsythe N, Fowler H, Greenwood G, Hashmi MZ, Liu XD, Miller JR, Ning L, Ohmura A, Palazzi E, Rangwala I, Schöner W, Severskiy I, Shahgedanova M, Wang MB, Williamson SN, Yang DQ (2015) Elevation-dependent warming in mountain regions of the world. Nature Climate Change 5, 424-430.
| Crossref | Google Scholar |
Pepler AS, Trewin B, Ganter C (2015) The influences of climate drivers on the Australian snow season. Australian Meteorological and Oceanographic Journal 65, 195-205.
| Crossref | Google Scholar |
Perrigo A, Hoorn C, Antonelli A (2020) Why mountains matter for biodiversity. Journal of Biogeography 47, 315-325.
| Crossref | Google Scholar |
Peyre G, Osorio D, François R, Anthelme F (2021) Mapping the páramo land-cover in the Northern Andes. International Journal of Remote Sensing 42, 7777-7797.
| Crossref | Google Scholar |
Pickering CM, Barry K (2005) Size/age distribution and vegetative recovery of Eucalyptus niphophila (snowgum, Myrtaceae) one year after fire in Kosciuszko National Park. Australian Journal of Botany 53, 517-527.
| Crossref | Google Scholar |
Rasouli AA, Cheung KKW, Mohammadzadeh Alajujeh K, Ji F (2022) On the detection of snow cover changes over the Australian Snowy Mountains using a dynamic OBIA approach. Atmosphere 13, 826.
| Crossref | Google Scholar |
Rodman KC, Andrus RA, Veblen TT, Hart SJ (2021) Disturbance detection in Landsat time series is influenced by tree mortality agent and severity, not by prior disturbance. Remote Sensing of Environment 254, 112244.
| Crossref | Google Scholar |
Rodriguez-Galiano VF, Ghimire B, Rogan J, Chica-Olmo M, Rigol-Sanchez JP (2012) An assessment of the effectiveness of a random forest classifier for land-cover classification. ISPRS Journal of Photogrammetry and Remote Sensing 67, 93-104.
| Crossref | Google Scholar |
Sánchez-Bayo F, Green K (2013) Australian snowpack disappearing under the influence of global warming and solar activity. Arctic, Antarctic, and Alpine Research 45, 107-118.
| Crossref | Google Scholar |
Sandvik SM, Odland A (2014) Changes in alpine snowbed-wetland vegetation over three decades in northern Norway. Nordic Journal of Botany 32, 377-384.
| Crossref | Google Scholar |
Scharnagl K, Johnson D, Ebert-May D (2019) Shrub expansion and alpine plant community change: 40-year record from Niwot Ridge, Colorado. Plant Ecology & Diversity 12, 407-416.
| Crossref | Google Scholar |
Scherrer P, Pickering CM (2005) Recovery of alpine vegetation from grazing and drought: Data from long-term photoquadrats in Kosciuszko National Park, Australia. Arctic, Antarctic and Alpine Research 37, 574-584.
| Crossref | Google Scholar |
Schwartz AJ, McGowan H, Callow N (2020a) Impact of fire on montane snowpack energy balance in Snow Gum forest stands. Agricultural and Forest Meteorology 294, 108164.
| Crossref | Google Scholar |
Schwartz AJ, McGowan HA, Theobald A, Callow N (2020b) Quantifying the impact of synoptic weather types and patterns on energy fluxes of a marginal snowpack. Cryosphere 14, 2755-2774.
| Crossref | Google Scholar |
Schwartz A, McGowan H, Callow N (2021) Snowpack thermal patterns in pre- and post-bushfire Snow Gum forests. Journal of Hydrology 602, 126789.
| Crossref | Google Scholar |
Shi H, Zhou Q, He R, Zhang Q, Dang H (2022) Climate warming will widen the lagging gap of global treeline shift relative to densification. Agricultural and Forest Meteorology 318, 108917.
| Crossref | Google Scholar |
Smith WK, Germino MJ, Johnson DM, Reinhardt K (2009) The altitude of alpine treeline: a bellwether of climate change effects. Botanical Review 75, 163-190.
| Crossref | Google Scholar |
Snowy Hydro (2022) Snowy live data – snow depths. (Snowy Hydro Limited: Cooma, NSW, Australia) Available at https://www.snowyhydro.com.au/generation/live-data/snow-depths/
Stine MB, Butler DR (2015) Effects of fire on geomorphic factors and seedling site conditions within the alpine treeline ecotone, Glacier National Park, MT. Catena 132, 37-44.
| Crossref | Google Scholar |
Strahler AH, Boschetti L, Foody GM, Friedl MA, Hansen MC, Herold M, Mayaux P, Morisette JT, Stehman SV, Woodcock CE (2006) Global land cover validation: recommendations for evaluation and accuracy assessment of global land cover maps. Report of Institute of Environmental Sustainability, Joint Research Centre, European Commission, Ispra, Italy.
Talukdar S, Singha P, Mahato S, Shahfahad, Pal S, Liou YA, Rahman A (2020) Land-use land-cover classification by machine learning classifiers for satellite observations – a review. Remote Sensing 12, 1135.
| Crossref | Google Scholar |
Testolin R, Attorre F, Jiménez-Alfaro B (2020) Global distribution and bioclimatic characterization of alpine biomes. Ecography 43, 779-788.
| Crossref | Google Scholar |
Thomas ZA, Mooney S, Cadd H, Baker A, Turney C, Schneider L, Hogg A, Haberle S, Green K, Weyrich LS, Pérez V, Moore NE, Zawadzki A, Kelloway SJ, Khan SJ (2022) Late Holocene climate anomaly concurrent with fire activity and ecosystem shifts in the eastern Australian Highlands. Science of The Total Environment 802, 149542.
| Crossref | Google Scholar |
Thompson JA (2016) A MODIS-derived snow climatology (2000–2014) for the Australian Alps. Climate Research 68, 25-38.
| Crossref | Google Scholar |
Thompson JA, Paull DJ (2017) Assessing spatial and temporal patterns in land surface phenology for the Australian Alps (2000–2014). Remote Sensing of Environment 199, 1-13.
| Crossref | Google Scholar |
Venn SE, Pickering CM, Butler SA, Letten AD (2016) Using a model based fourth-corner analysis to explain vegetation change following an extraordinary fire disturbance. Oecologia 182, 855-863.
| Crossref | Google Scholar |
Verrall B, Pickering CM (2019) Recovery of subalpine grasslands 15 years after landscape level fires. Australian Journal of Botany 67, 425-436.
| Crossref | Google Scholar |
Verrall B, Pickering CM (2020) Alpine vegetation in the context of climate change: a global review of past research and future directions. Science of The Total Environment 748, 141344.
| Crossref | Google Scholar |
Verrall B, Green K, Pickering CM (2021) Dynamics in plant diversity and composition on Australian alpine summits over time. Biodiversity and Conservation 30, 1855-1880.
| Crossref | Google Scholar |
Verrall B, Green K, Pickering CM (2023) Temporal dynamics in alpine snowpatch plants along a snowmelt gradient explained by functional traits and strategies. Oecologia 201, 155-171.
| Crossref | Google Scholar |
Vogelmann JE, Gallant AL, Shi H, Zhu Z (2016) Perspectives on monitoring gradual change across the continuity of Landsat sensors using time-series data. Remote Sensing of Environment 185, 258-270.
| Crossref | Google Scholar |
Wahren CH, Camac JS, Jarrad FC, Williams RJ, Papst WA, Hoffmann AA (2013) Experimental warming and long-term vegetation dynamics in an alpine heathland. Australian Journal of Botany 61, 36-51.
| Crossref | Google Scholar |
Walsh NG, McDougall KL (2004) Progress in the recovery of the flora of treeless subalpine vegetation in Kosciuszko National Park after the 2003 fires. Cunninghamia 8, 439-452.
| Google Scholar |
Wang YF, Case B, Lu XM, Ellison AM, Peñuelas J, Zhu HF, Liang EY, Camarero JJ (2019) Fire facilitates warming-induced upward shifts of alpine treelines by altering interspecific interactions. Trees 33, 1051-1061.
| Crossref | Google Scholar |
Wang YF, Liang EY, Lu XM, Camarero JJ, Babst F, Shen MG, Penuelas J (2021) Warming-induced shrubline advance stalled by moisture limitation on the Tibetan Plateau. Ecography 44, 1631-1641.
| Crossref | Google Scholar |
Wickham H, Chang W, Henry L, Pedersen T, Takashi K, Wilke C, Woo K, Yutani H, Dunnington D (2022) ggplot2: create elegant data visualisations using the grammar of graphics. R package version 3.3.6. Available at https://cran.r-project.org/package=ggplot2.
| Google Scholar |
Wieser G, Oberhuber W, Gruber A (2019) Effects of climate change at treeline: lessons from space-for-time studies, manipulative experiments, and long-term observational records in the Central Austrian Alps. Forests 10, 508.
| Crossref | Google Scholar |
Williams RJ, Wahren CH, Bradstock RA, Müller WJ (2006) Does alpine grazing reduce blazing? A landscape test of a widely-held hypothesis. Austral Ecology 31, 925-936.
| Crossref | Google Scholar |
Williams RJ, Wahren C-H, Tolsma AD, Sanecki GM, Papst WA, Myers BA, McDougall KL, Heinze DA, Green K (2008) Large fires in Australian alpine landscapes: their part in the historical fire regime and their impacts on alpine biodiversity. International Journal of Wildland Fire 17, 793-808.
| Crossref | Google Scholar |
Wilson SD, Nilsson C (2009) Arctic alpine vegetation change over 20 years. Global Change Biology 15, 1676-1684.
| Crossref | Google Scholar |
Zandler H, Faryabi SP, Ostrowski S (2022) Contributions to satellite-based land cover classification, vegetation quantification and grassland monitoring in Central Asian Highlands using Sentinel-2 and MODIS data. Frontiers in Environmental Science 10, 684589.
| Crossref | Google Scholar |
Zhang M, Du H, Zhou G, Li X, Mao F, Dong L, Zheng J, Liu H, Huang Z, He S (2019) Estimating forest aboveground carbon storage in Hang-Jia-Hu using Landsat TM/OLI data and random forest model. Forests 10, 1004.
| Crossref | Google Scholar |
Zimmer A, Meneses RI, Rabatel A, Soruco A, Dangles O, Anthelme F (2018) Time lag between glacial retreat and upward migration alters tropical alpine communities. Perspectives in Plant Ecology, Evolution and Systematics 30, 89-102.
| Crossref | Google Scholar |
Zomer MA, Ramsay PM (2021) Post-fire changes in plant growth form composition and diversity in Andean paramo grassland. Applied Vegetation Science 24, e12554.
| Crossref | Google Scholar |
Zylstra P (2013) The historical influence of fire on the flammability of subalpine Snowgum forest and woodland. Victorian Naturalist 130, 232-239.
| Google Scholar |
Zylstra PJ (2018) Flammability dynamics in the Australian Alps. Austral Ecology 43, 578-591.
| Crossref | Google Scholar |