Reflections on genetic improvement
J. H. J. van der Werf
A School of Environmental & Rural Science, University of New England, Armidale, NSW 2351, Australia.
Animal Production Science 63(11) 925-930 https://doi.org/10.1071/AN23214
Submitted: 7 June 2023 Accepted: 7 June 2023 Published: 6 July 2023
© 2023 The Author(s) (or their employer(s)). Published by CSIRO Publishing. This is an open access article distributed under the Creative Commons Attribution-NonCommercial-NoDerivatives 4.0 International License (CC BY-NC-ND)
Abstract
Dr Helen Newton-Turner introduced a powerful model of bringing science into practice by working closely with breeders and the wider industry. This collaboration model has led to considerable achievements in genetic improvement in the Australian animal industries, with efficient genetic evaluation systems being implemented, providing a platform to achieve well defined breeding objectives and the introduction of new technologies such as genomic selection. The same model can be used to foster future development to achieve sustainable further improvements, allowing further technologies such as functional genomics to be used where they add value.
Keywords: animal breeding, breeding objectives, genetic improvement, genomic selection, Helen Newton-Turner, Quantitative Trait Loci, sheep CRC, University of New England.
Introduction
When informed that I was the awardee of the Helen Newton-Turner Medal, I started to look into the history of the award including the career of Helen Newton-Turner, and the achievements of previous recipients of this award. I was increasingly impressed by the achievements of past awardees and at the same time I felt quite humbled and incredibly honoured to see my name added to the list of awardees. I might be the first awardee that has never met Dr Helen Newton-Turner as I came to Australia 25 years ago, 1 year after she died. Much has been written about Helen Newton-Turner (see Allen 1992; Moyal 1994), who after graduating in architecture worked as a secretary in the CSIR (now CSIRO) McMaster Laboratory on the campus of the University of Sydney. Her boss was the famous CSIRO chief and scientist, (Sir) Ian Clunies Ross who recognised her great talent for mathematics and statistics and encouraged her to study. In 1938, he arranged for her to spend a year in the UK to receive further training in the application of statistics to agriculture with (Sir) Ronald Fisher at University College, London, and Frank Yates at Rothamsted. On her return trip to Australia, she spent 10 weeks in the USA visiting sheep research laboratories. During her subsequent career, she was instrumental in the introduction of quantitative genetics to sheep breeding in Australia. When reading comments by previous medal recipients, who worked with her, it became apparent that she was inspirational and influential. Her strong message was to ‘breed by numbers’. Reading some of these past orations makes one aware how well scientists worked together with ‘the industry’, with individual breeders being very important in driving progress.
The Association for the Advancement of Animal Breeding and Genetics (AAABG) is an exemplary embodiment of this relationship among farmers, industry, and scientists. At the first AAABG meeting in 1979, Professor Stuart Barker presented ‘the birth of the AAABG, as a result of a multiple sire mating with Dr Keith Hammond as the dam carrying the baby, with possibly multiple sires’ (Barker 1979). He also pointed out the importance of scientists, breeders, and industry organisations working together to foster ‘the tremendous potential for genetic improvement of productivity and reduction in cost of production’. I will continue that theme and share some of the history of animal breeding in Australia with the new generation, as I think there are valuable lessons we can learn, and then look forward.
Dutch connection
I might be the first Dutch Helen Newton-Turner Medallist, but I am certainly not the first Dutchman to play a role in Australian Genetics. Barker (1979) suggested that an important influence in Australian animal breeding was provided by the visits of Dr J. L. Lush from Iowa, and Dr A. L. Hagedoorn from Holland (Fig. 1). Most of us know Dr Lush as ‘the father of animal breeding’, but few have heard of Dr Hagedoorn. He was here for only 4 months, but he was involved in 80 meetings with both breeders and scientists. This mysterious Dutchman, Hagedoorn, was an outstanding geneticist. However, in a biography, Theunissen (2014) pointed out that he was not well remembered by historians ‘mainly due to the fact that he was an expert in animal breeding’ (p. 55). As early as 1941, at a conference in New Zealand, McMahon referred to Hagendoorn’s ideas about nucleus breeding systems ‘as an ideal method of exploiting the progeny test’ (McMahon 1941).
Jay Lush from Iowa State University (left) and Dr A.L. Hagedoorn (left in the right picture, with Ted Lefroy from Western Australia) were two world leading livestock to visit Australia. Ted referred to Hagedoorn’s book ‘Animal Breeding’ as ‘…the best there is as a guide for practical breeders.’ Photo: WA Newspapers (https://www.moffittsfarm.com.au/2020/11/19/unique-insight-into-merino-breeding-and-pastoral-history/ website).
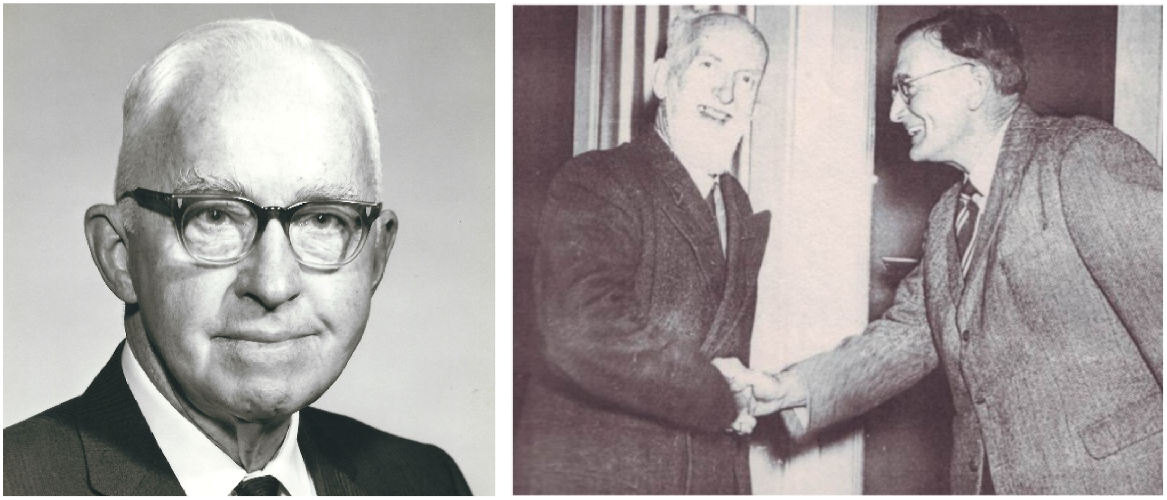
Before coming to Armidale, I was a lecturer in Wageningen, a University well known for agricultural research and education. The Dutch are very pragmatic, and they excel in quickly applying innovations. They generally have a practical approach and breeding program design is a strong element of their education in animal breeding. I was pleased to see that this was a good fit with the Australian approach. As a Masters student I spent a year at Cornell University. Initially, I was put behind the desk of Dr Henderson, who was away on sabbatical. Dr Henderson was the godfather of linear models and the one that invented best linear unbiased prediction (BLUP). I sat in the ‘baby-BLUP’ course delivered by Dr Dick Quaas, an amazing thinker who sadly passed away recently. Dick Quaas invented the ‘animal model’ as well as clever rules about the numerator relationship matrix, both are now extensively used in routine genetic evaluation. At that time, animal breeding in the USA was dominated by statistics and linear models. When Dr Henderson presented his Animal Model at a European conference in 1987, Professor Alan Robertson from Edinburgh, who was the godfather of genetic theory applied to animal breeding, asked whether we should expect more inbreeding when selecting on BLUP breeding values, Henderson had no idea what he was referring to! The person who was a master in understanding and teaching the coalescence between the theories of linear models and quantitative genetics was Dr Brian Kennedy from the University of Guelph, and I had the pleasure to work with him a lot while working on my PhD thesis.
Move to Armidale
Armidale and the University of New England (UNE) was internationally known for animal genetics and it was not difficult to decide to move to the other side of the world when offered a position and arriving in 1996. I worked briefly with Mike Goddard, FRS, and enjoyed many lunches with Mike during my first year. He was pragmatic, like the Dutch. Brian Kinghorn was, of course, one of my main mentors. I took over much of Brian’s teaching when I arrived, and still use many of his clever concepts, for example, when explaining BLUP to the un-initiated undergraduate. With Brian, I co-taught various courses, several of them overseas. This was always great fun and quite productive, with lots of ideas to talk about. During a long drive in New Zealand about 20 years ago, we made a single slide summarising inbreeding, involving spanners and nuts and bolts, on the backseat of the car. We are still using this slide in our undergraduate course (Fig. 2). Brian is a pure out of the box thinker. This is best illustrated by his slides about rules-based and tactical decision-making in breeding programs (Fig. 3). Once the ultimate goal is defined, he uses artificial intelligence, in the form of genetic algorithms, to find a solution to achieve it, just like nature does via evolution. This philosophy is at the heart of how scientists can work with breeders to find unorthodox but efficient ways of designing their breeding programs and it explains the attractiveness and success of Matesel (Kinghorn et al. 2022).
Inbreeding cartoon developed with Brian Kinghorn in the back of the car on our teaching road trip through New Zealand.
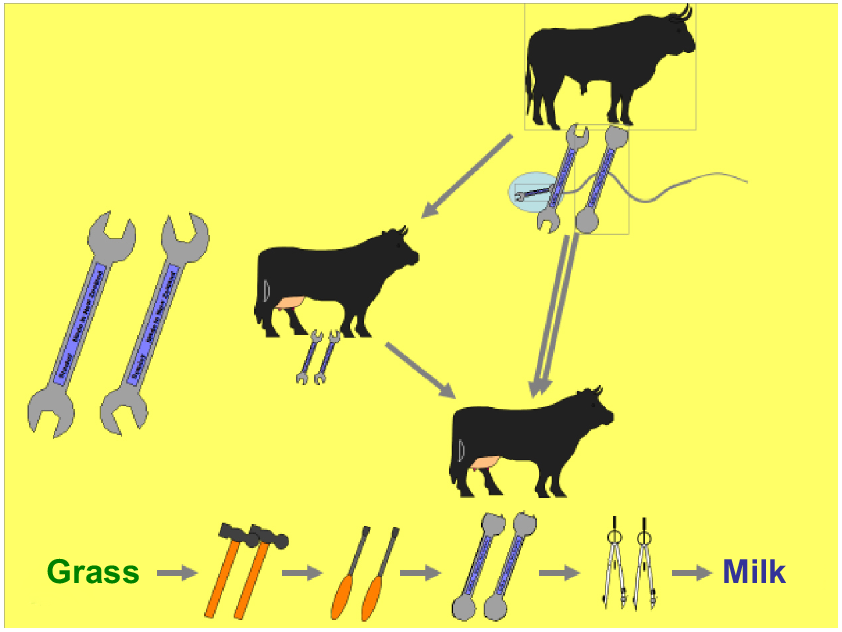
Brian Kinghorn’s slide on how scientists can interact with breeders in decision support. Rather than providing ‘rules-based’ recipes, the breeder provides options given the input parameters and constraints, and shows possible actions and outcomes, such that the end-user can make an informed decision about design and implementation of breeding programs.
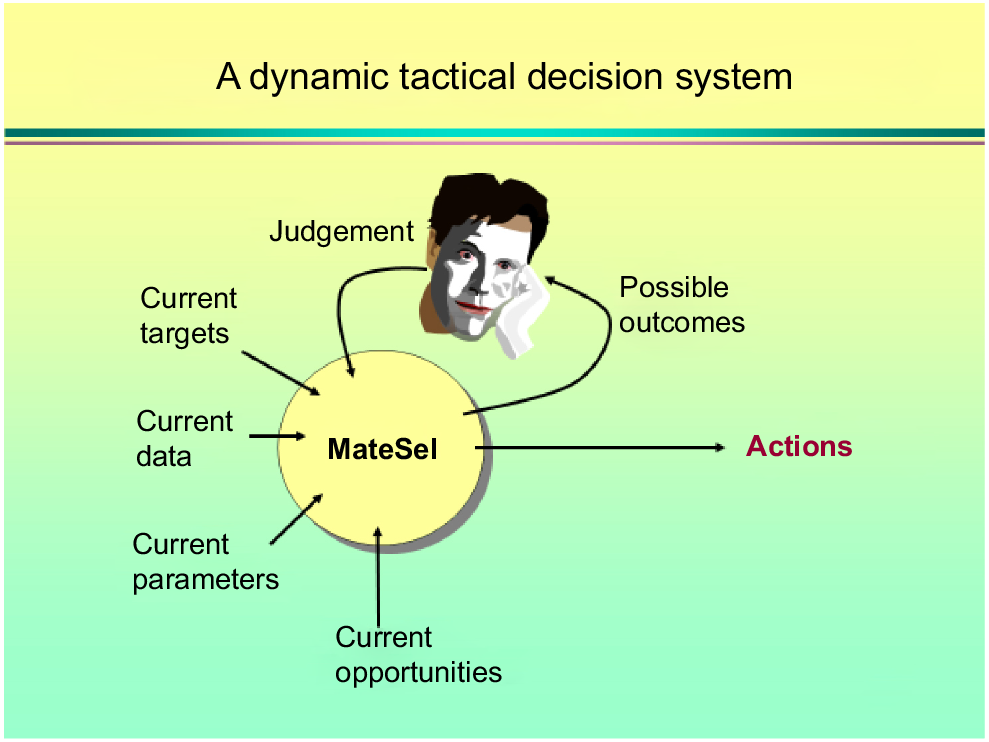
Another important influence was Rob Banks. Rob is a creative thinker and a passionate geneticist who strongly believes in the power of genetic improvement. With his office being just down the hallway, we had an ongoing dialogue, where he would come up with good ideas which I then turned into a spreadsheet. I often use his slide about the progress of the lamb industry, showing a five-fold value adding in 23 years between 1995 and 2018 (Fig. 4). Rob can be credited much of this, with starting up LAMBPLAN in the early 1990s (Banks and Gilmour 1992). The Australian lamb industry achieved the highest rate of genetic gain in Australia, and, unlike many cattle programs, did that without importing genetic improvement.
Rob Banks’ slide on the impressive genetic improvement made by the meat sheep industry in Australia. Note that the currency in the figure is AU$.
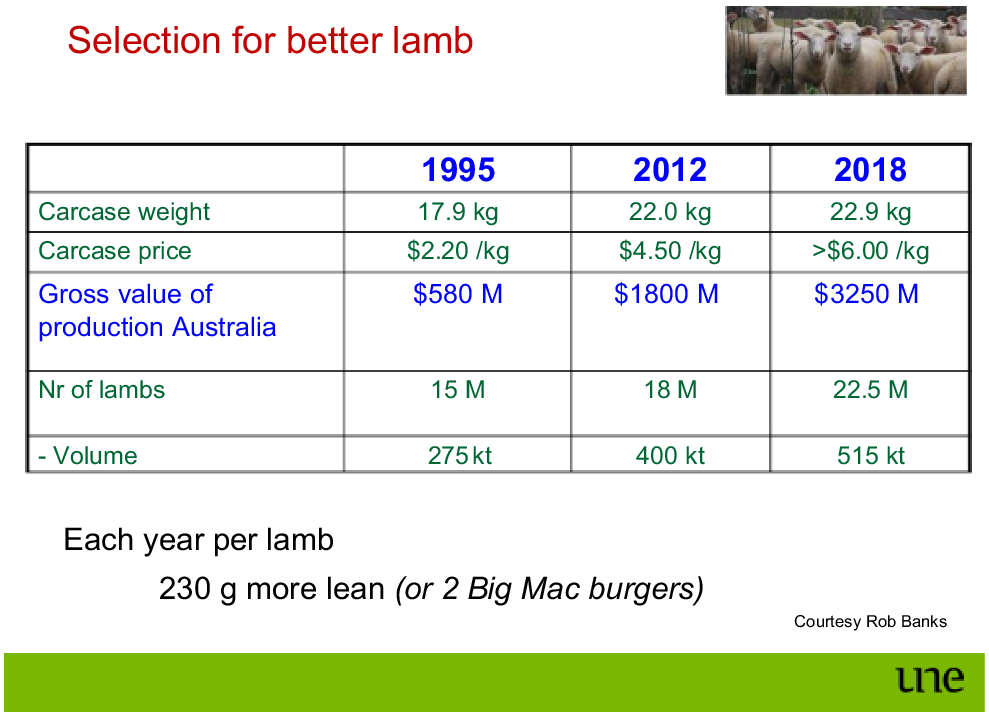
Some of the early work I undertook in Armidale was on quantitative train locus (QTL) mapping, similar to the work everyone else was doing in the world, but few in Armidale. We looked at meat sheep, with LAMBPLAN in conjunction with Jay Hetzel, who had just started his company Genetic Solutions. I remember this as spending more time on working out intellectual property (IP) issues than on analysing data or finding any QTL. It would be remiss not to mention the Golden Ram project. People in Armidale were convinced of this special ram that must have a major gene for parasite resistance. In 2001, we set up a project with Australian Wool Innovation (AWI) to genotype for 200 markers in 400 lambs in four half-sib families, costing AU$3 for each genotype. The cost of genotyping for each genetic marker is now 6000 times cheaper with a single-nucleotide polymorphism (SNP) chip and marker genotypes from whole genome sequence data are 180 000 times cheaper. We can now find QTL in datasets of 100 000 sheep by using 30 million markers. The remarkable fact is that although we do find some convincing QTL, very few of them have a large effect on phenotype, and even fewer of them are explicitly targeted in livestock breeding programs, as most traits appear to be quite polygenic (Dekkers 2012). Along this vein, the QTL search in the Golden Ram project did find some significant QTL, but not the major gene that was hypothesised (Marshall et al. 2009).
One good example of an important QTL is the Booroola gene (Mulsant et al. 2001). At UNE, we were involved in a project where this gene was successfully introgressed into a local sheep breed in India, with Chanda Nimbkar, then also a UNE PhD student. Chanda Nimbkar now plays a major role in animal breeding in India where she is Director of Animal Husbandry, Nimbkar Agricultural Research Institute, Phaltan, India. Similarly, Karen Marshall, my first PhD student in Armidale, moved to the International Livestock Research Institute, Nairobi, Kenya, and has worked tirelessly on implementing genetic improvement in the most challenging circumstances in Africa and Asia. We are proud to have them both as UNE alumni. Helen Newton-Turner would have been pleased had she known about the contributions of these two talented women because she spent the latter part of her career on genetic improvement of livestock in developing countries.
Genomic selection
The 2007, the AAABG conference was in Armidale and it signified a marked shift in using genetic markers, because this was the time that we moved from marker-assisted selection to genomic selection. At the conference, Curt van Tassel, a keynote speaker from the USDA, Washington, USA, who led a team that had almost completed the first 50 k Illumina SNP-chip for cattle, provided an overview of genetic selection by using genomics (Van Tassel et al. 2007). Another speaker was Ben Hayes who presented one of the first reports on genomic prediction accuracy in dairy bulls (Hayes et al. 2007). The dairy industry was first to embrace genomic selection, and has received large benefits from this initiative (Hayes et al. 2009). The sheep industry followed relatively quickly and, at the next AAABG conference in Adelaide, in 2009, we presented modelling that demonstrated the benefits of genomic selection to the Australian sheep industry (Banks and Van der Werf 2009; Van der Werf 2009).
The Sheep Cooperative Research Centre (CRC), headquartered in Armidale, was instrumental in the introduction of genomic selection to the sheep industry. The CRC started in 2002 and finished in 2019; a detailed overview of the many achievements of the CRC is available (Thompson and Rowe 2019). The CRC program was very successful, mainly in meat science and in genetics, with great progress made in introducing genomic selection and understanding the need for measuring eating quality of sheep meat. The timing was great. We followed the ambitious Sheep Genomics program from Meat & Livestock Australia and AWI, but the tack had changed from QTL to genomics.
The success of the Sheep CRC research was truly a team effort. From the design of the first ovine SNP chip, launched in 2009, for which we owe a lot to the input from John McEwan, to benefitting from the experience gained in the Dairy CRC through Ben Hayes, and the massive effort in data collection from the Information Nucleus Flock, and the farm and meat science teams associated with it. The Information Nucleus was a significant planned innovation, in fact one of the first designed reference populations for genomic selection, and at the same time a rich data resource for estimating parameters for traits that are hard to measure on farm (Fogarty et al. 2007; van der Werf et al. 2010). Scientists and breeders collaborated across Australia ensuring the cost-effectiveness of the program, via design; a nice example of the pragmatic innovation attitude that signifies the Australian animal breeding community. The implementation and use of genomic data in genetic evaluation could not have been achieved without the AGBU team of Daniel Brown and Andrew Swan and expertise in higher-end sequencing with Hans Daetwyler and Iona MacLeod from the team at AgBio in Melbourne. At the start in 2007, we budgeted AU$500 for a genomic test, and at the end of the CRC in 2019, a genomic test was down to AU$25. The genomic pilot program, the cost–benefit modelling and the early uptake of the technology by many breeders is an excellent example of how science and industry can work together to implement powerful new technology. I also want to acknowledge James Rowe, CEO of the CRC, for his leadership. He was always progressive, encouraged innovators, and as a non-geneticist he had a tremendous input in introducing new genetic products such as the Parentage test, the Horned-Polled test, RamSelect and Flock Profile. He was instrumental in getting Neogen onside to help develop better genotyping arrays for us. These products and tools are important for showing the importance of using improved genetics, because they drive genetic gains through the whole industry.
Broad vision built on core precepts
When teaching animal breeding to students I have found it always important to consider ‘the big picture’. Although estimating genetic parameters of new traits, and analysis of genomic data, can be really exciting, it is important to develop a framework that allows putting things in perspective in relation to an overall goal of genetic improvement. Defining a clear breeding objective and determining the relative value of trait improvement is the most important first step. Then, I believe, there are only a few formulas that capture the essence of making progress in a breeding program. These are
– the breeder’s equation: R = i.rIA.σA/L,
– the prediction equation for breeding value: ,
– and the optimal selection equation: .
where i is the selection intensity, rIA = the selection accuracy, σA is the genetic standard deviation, L is the generation interval, û is a vector with estimated breeding values, V is a variance-covariance matrix among the observed phenotypes, G is the covariance matrix between the observed phenotyes and the breeding values, x is a vector with genetic contributions of selection candidates to the next generation, λ is a penalty on co-ancenstry and A is the numerator relationships matrix among selection candidates.
The interesting thing about breeding programs is that the factors that determine genetic progress are interdependent, such as, for example, accuracy and generation interval. Genomics has now become a big part of our research, but we have to understand how they affect the genetic gain, i.e. higher accuracy and earlier selection. It is similar for using reproductive technologies.
It is important that students learn this big picture view, and have insight in how their efforts contribute to genetic gain. An important part of a breeding program is how we balance multiple-trait selection. How can we get what we want without negative responses? An economic as well as biological understanding of the production systems is needed here, and, subsequently, a weighting of traits as well as an optimal measurement regime is needed.
One factor that always receives the most attention is the prediction equation or the ‘BLUP’, namely, . Complexities can be added, such as correction for fixed effects, but prediction depends really on the phenotypic data you have available, across a range of traits and these phenotypes are related to the genotypes that you want to predict. This can be determined by pedigree or by genomic information, and the many predictors from genomic information can be modelled in a linear or a Bayesian manner. From a breeding program point of view, the genomic information is an extension of pedigree, it allows us to use phenotypic information from a much wider group of animals to predict merit of a DNA-tested animal. This will have a large impact on genomic prediction accuracy. In the near future, as we can expect to collect DNA information from a much larger part of the population, and, equally, technology will allow collection of phenotypes on a much larger scale, and, indeed, on new traits such as efficiency and enteric methane emissions. All such data can be used and we will need to be pragmatic about how to put it in our prediction machinery.
Future perspective
A lot of excellent genomic research is now functional (e.g. Prowse-Wilkins et al. 2022), and we have fantastic tools to learn about biology and genetics across and within species, the genetic model of variation, how it interacts with the environment, etc. But even if we knew a lot more of the so-called ‘functional variants’, we would still need to estimate the effects and these may well differ among populations, breeds, ages and environments. To estimate average effects, as relevant in breeding values, a whole-genome approach with more data is usually the simplest recipe to increase accuracy. However, while the accuracy of a breeding value is mainly important for genetic improvement, a better understanding of the genetic model underlying phenotypic information has more uses, such as, for example, for more precise management, similar to personalised medicine in humans. There are only few examples where specific functional variants are targeted in selection or gene editing. Whether the number of such applications will expand is yet to be seen.
The application of genomics to animal breeding programs has led to massive changes such as doubling the rate of genetic gain in dairy cattle and the ability to select for hard-to-measure traits in various species (Swan et al. 2018; Lassen and Difford 2020; Brito et al. 2021; Banks 2022). What are the main challenges as we look to the future? In 1979, Stuart Barker suggested not to take a parochial view, but to focus on the needs to feed the growing human population. This challenge has been exacerbated by the climate crisis. In fact, animal production per se is even questioned by some in society (Pethick et al. 2023). These challenges make it imperative to define clear goals, taking account as best we can of anticipated societal demands, develop a full understanding of the whole system, the role of animal production in the system, and how genetic improvement can contribute. The triple bottom line, i.e. considering profit, people and the planet, is a 25-year-old concept that is still highly relevant for sustainable goal setting. It is clear that genetic improvement can have a large impact on whatever we want to improve, for example, ‘methane efficiency’.
I will conclude by saying that there is a lot that can be done in animal genetic improvement to contribute to the global challenge of sustainable production of animal products. We need a wholesystem approach for clear setting of sustainable goals, such that the incredible tools that are being developed are utilised most efficiently and effectively. An open and transparent collaboration and innovation models that involve researchers, breeders and producers as well as the broader community have been and will be a powerful way forward that can use the full range of skills to address future challenges and opportunities.
Acknowledgements
I want to thank the AAABG for awarding me this great honour and the Committee for organising such a great conference under the difficult circumstances of ‘COVID’. They are too numerous to mention, but I sincerely thank all my colleagues and students, near and far, for the great interactions we have had, and their contribution to my success. I thank my family for their unconditional support and tolerating my ongoing obsession with genomic selection. Dr Robert Banks and an anonymous reviewer are acknowledged for useful comments on this written version of my oration.
References
Allen N (1992) Helen Newton Turner and the wool industry. Journal of Australian Studies 16(33), 56-62.
| Crossref | Google Scholar |
Banks R (2022) Evolution of genetics organisations’ strategies through the implementation of genomic selection: learnings and prospects. Agriculture 12, 1524.
| Crossref | Google Scholar |
Brito LF, Bedere N, Douhard F, Oliveira HR, Arnal M, Peñagaricano F, Schinckel AP, Baes CF, Miglior F (2021) Review: Genetic selection of high-yielding dairy cattle toward sustainable farming systems in a rapidly changing world. Animal 15, 100292.
| Crossref | Google Scholar |
Dekkers JCM (2012) Application of genomics tools to animal breeding. Current Genomics 13, 207-212.
| Crossref | Google Scholar |
Fogarty NM, Banks RG, Van der Werf JHJ, Ball AJ, Gibson JP (2007) The information nucleus – a new concept to enhance sheep industry genetic improvement. In ‘Proceedings of the seventeenth conference of the association for the advancement of animal breeding and genetics’, pp. 29–32. (Association for the Advancement of Animal Breeding and Genetics)
Hayes BJ, Bowman PJ, Chamberlain AJ, Goddard ME (2009) Invited review: genomic selection in dairy cattle: progress and challenges. Journal of Dairy Science 92, 433-443.
| Crossref | Google Scholar |
Lassen J, Difford GF (2020) Review: Genetic and genomic selection as a methane mitigation strategy in dairy cattle. Animal 14, s473-s483.
| Crossref | Google Scholar |
Marshall K, Maddox JF, Lee SH, Zhang Y, Kahn L, Graser H-U, Gondro C, Walkden-Brown SW, van der Werf JHJ (2009) Genetic mapping of quantitative trait loci for resistance to Haemonchus contortus in sheep. Animal Genetics 40, 262-272.
| Crossref | Google Scholar |
Mulsant P, Lecerf F, Fabre S, Schibler L, Monget P, Lanneluc I, Pisselet C, Riquet J, Monniaux D, Callebaut I, Cribiu E, Thimonier J, Teyssier J, Bodin L, Cognié Y, Chitour N, Elsen J-M (2001) Mutation in bone morphogenetic protein receptor-IB is associated with increased ovulation rate in Booroola Mérino ewes. Proceedings of the National Academy of Sciences 98, 5104-5109.
| Crossref | Google Scholar |
Pethick DW, Bryden WL, Mann NJ, Masters DG, Lean IJ (2023) The societal role of meat: the Dublin Declaration with an Australian perspective. Animal Production Science
| Crossref | Google Scholar |
Prowse-Wilkins CP, Lopdell TJ, Xiang R, Vander Jagt CJ, Littlejohn MD, Chamberlain AJ, Goddard ME (2022) Genetic variation in histone modifications and gene expression identifies regulatory variants in the mammary gland of cattle. BMC Genomics 23, 815.
| Crossref | Google Scholar |
Theunissen B (2014) Practical animal breeding as the key to an integrated view of genetics, eugenics and evolutionary theory: Arend L. Hagedoorn (1885–1953). Studies in History and Philosophy of Science Part C: Studies in History and Philosophy of Biological and Biomedical Sciences 46, 55-64.
| Crossref | Google Scholar |
van der Werf JHJ, Kinghorn BP, Banks RG (2010) Design and role of an information nucleus in sheep breeding programs. Animal Production Science 50, 998-1003.
| Crossref | Google Scholar |
Van Tassel CP, Sonstegard TS, Liu G, Matukumalli LK (2007) Current direction in cattle genomics research. In ‘Proceedings of the seventeenth conference of the association for the advancement of animal breeding and genetics’, pp. 461–470. (Association for the Advancement of Animal Breeding and Genetics)