Patterns of activity and travel by koalas in a disturbed urban landscape in Queensland
Philippa Kirsten Tacla A , Benjamin James Barth B , Sean Ian FitzGibbon B , Amber Kristen Gillett B and William Anthony Ellis A B *A
B
Abstract
Habitat loss and fragmentation threaten koala persistence in south-east Queensland, intensifying the challenges posed by climate change and disease to koala survival. Adaptation to modified landscapes may mediate species’ persistence, so we collected location and accelerometery data for 10 koalas in a changing landscape in the Moreton Bay region of Queensland, using remote global positioning system (GPS) collars and manual tracking. We investigated whether ambient temperature influenced how active koalas were and how far they moved, and compared area of use estimated by manual tracking and remote GPS uploads. Accelerometric data showed that koalas were more active at night, and GPS data showed that males moved further than females, but accelerometers measuring activity were not indicative of total distance travelled. Distance moved by koalas per day and night was greatest from May through August and least during March for both males and females. Remotely uploaded GPS locations described a not significantly larger total area of use by each koala compared to manual VHF tracking. There was no general trend in the relationship between day temperature and koala activity or distance moved.
Keywords: accelerometery, global positioning system, koala, Phascolarctos cinereus, radio tracking.
Introduction
Anthropogenic development invariably disturbs landscapes, forcing animals to travel and expend energy locating patchy resources, separated by structures, roads or treeless paddocks (Rhodes et al. 2008; Goldingay and Dobner 2014; Barth et al. 2020; Whisson et al. 2020). The resulting fragmented habitats put physiological pressure on koalas to find food and shelter during hot and dry conditions (Degabriele and Dawson 1979; Nagy and Martin 1985; Ellis et al. 1995, 2010), so nocturnal feeding and activity patterns are likely to be part of a thermoregulatory strategy and may provide information on adaptation to changes in landscape features and temperature regimes (Logan and Sanson 2002; Crowther et al. 2014; Adam et al. 2021). Resource distribution and koala activity patterns are key factors that influence the risk posed by roads, determining the propensity for vehicle strikes (Dique et al. 2003; Goldingay and Dobner 2014; Ellis et al. 2016; Dissanayake et al. 2023; Dexter et al. 2024) so gathering information on koala responses to pressures imposed by habitat fragmentation is important (Adams-Hosking et al. 2012; Narayan 2019). There is an ongoing need for improved monitoring technologies, to better understand the energetic costs and impacts of both land disturbance and climate change on free-ranging koalas.Mit
Global positioning system (GPS) use in wildlife tracking projects offers the benefit of recording data at regular intervals for free-ranging animals, across inaccessible locations, without the risk of observer artefacts such as disturbing the subjects (Tomkiewicz et al. 2010; Murgatroyd et al. 2023). Collars equipped with GPS have been used to monitor koala behaviour and habitat use for some time (Ellis et al. 2010; Matthews et al. 2013; Crowther et al. 2014; Mitchell et al. 2023), demonstrating that koalas travel further to find resources in fragmented habitats and are reliant on scattered trees in open farmland (Barth et al. 2020; Rus et al. 2021). Although GPS is useful to estimate home range and to identify habitat quality, it does not measure the energetic cost of utilising different habitats. Because energy expenditure is likely to be a limiting factor in koala distribution, it is an important component of habitat suitability in changing landscapes.
Accelerometery is a measure of the relative intensity of physical movement, usually monitored through a wearable device (Chen and Bassett 2005), so when GPS collars are paired with accelerometers, detailed inferences can be made about physiology and behaviour. Although the energetic advantages of non-food trees to koalas have been investigated (Briscoe et al. 2014), general patterns of movement or activity and critical environmental drivers of this activity (such as the benefit of shifting from food to non-food tree) are only postulated to date (Adam et al. 2020). If we can predict what habitat features are critical to koala persistence under specific environmental conditions, the ecological value of those features can be determined within a landscape. One way to do this is to monitor landscape use in the context of environmental data. The current project examined the potential for accelerometer and GPS outputs from commercially available LX K-trackers (LX Industries, NSW, Australia) to study koala ecology and inform habitat management. Our testable hypotheses were that: (1) accelerometric activity would be directly related to distance travelled and enable a more accurate estimate of energy expended than the distance between two GPS locations; and (2) there would be a relationship between behaviour of koalas (movement and/or activity) and daytime temperature. Finally, we aimed to correlate koala activity with environmental factors so that we could predict the energetic cost of koala habitat choices.
Method
Study site and data collection
Kinma Valley (Stocklands Pty Ltd, −27.167124, 152.911216) is a 234-ha, developing residential project site in the Moreton Bay Regional Council of Queensland, comprised of regrowth eucalyptus and acacia woodland (across a previous pine plantation), riparian remnant forest and open cleared pasture. Some 2500 house lots are planned for the site, so the disruption to existing koala habitat is significant and occurs under the control of a Commonwealth Approval (EPBC 2018/8359).
Adult koalas (five male, five female) were monitored between 2021 and 2024, as part of a koala management plan (28_South 2022) for the site (Table 1). Koalas were captured using the flagging-only method (Ellis et al. 1995; Madani et al. 2020) and received veterinary health assessment on site. Diseased koalas were transported to Australia Zoo Wildlife Hospital (AZWH) for care. Healthy koalas were collared (LX K-tracker: Fig. 1) and released into their original capture tree. Four koalas were treated at AZWH and returned to the site during this project. Two male and three female koalas generated insufficient manual tracking data for analyses (<30 locations) and one male generated insufficient accelerometery data (from only 48 uploads) to be included in comparisons. Results for accelerometery and distance are hence presented for five females and four males and comparative home range analysis is presented for two females and three males. Complete tracking datasets, containing data for every month of the study, were only collected for five individuals across the 2 years of the study (three male, two female), and these koalas provide data for the comparison of Lx locations with manual tracking locations. Data from all koalas contributed to average monthly distance moved per koala and activity analyses.
Koala | Body | Sex | Body | Tooth | Head | Tracking | Lx | Manual | MCP | MCP | AKDE | AKDE | |
---|---|---|---|---|---|---|---|---|---|---|---|---|---|
Number | Mass | Score | Wear | Length (mm) | Period (days) | Fixes (used) | Tracking (events) | Tracking (ha) | Lx (ha) | Tracking (ha) | Lx (ha) | ||
13271 | 7.3 | Male | 9 | PM flat | 144.9 | 153 | 48 | 11 | Invalid | 4.6 | Invalid | 8.45 | |
13340 | 4.9 | Female | 7.5 | Tips | 128.3 | 365 | 289 | 18 | Invalid | 3.9 | Invalid | 4.83 | |
13341 | 6.6 | Female | 8 | 2 lines | 127.1 | 609 | 148 | 44 | 6.04 | 10.6 | 18.9 | 14.1 | |
13327 | 5.7 | Female | 7 | PM flat | 130 | 273 | 127 | 20 | Invalid | 4.07 | Invalid | 3.1 | |
13363 | 7.3 | Male | 7 | 2 lines | 79.7 | 668 | 271 | 21 | Invalid | 65.2 | Invalid | 98.7 | |
13364 | 7.3 | Male | 8.5 | 1 line | 141.8 | 365 | 241 | 26 | 20.2 | 38.3 | 37.9 | 33.5 | |
13369 | 6.4 | Female | 7 | 1 line | 131.9 | 151 | 135 | 11 | Invalid | 23.4 | Invalid | 25.2 | |
13379 | 7.1 | Male | 6.5 | 2 lines | 142.7 | 427 | 138 | 31 | 8.5 | 10.5 | 17.1 | 11.8 | |
13380 | 4.3 | Female | 7 | Nil | 111.5 | 519 | 169 | 37 | 10.5 | 20.8 | 19.7 | 19.2 | |
13387 | 6.7 | Male | 7 | 1 line | 145 | 122 | 159 | 9 | 23.6 | 64.9 | Invalid | 82.9 |
Lx tracking collar formerly monitoring M2, found on the ground with a broken ‘weak link’, designed to protect koalas from misadventure due to entanglement of collar.
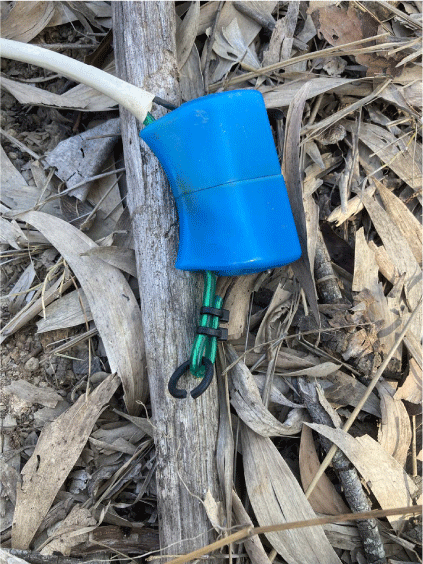
The LX collars reported accelerometric activity every 30 min and GPS location data approximately every 3 h each day. Accelerometric activity was reported as a number (0–100,000) corresponding to the intensity of movement during the 30-min recording period. No indicator of the plane or direction of movement was provided, so we were unable to correlate specific activity with the accelerometric signature, as has been done in other studies (Ryan et al. 2013), but we generated an individual index of general activity for each koala over time. Data were accessed through the INCYT platform (https://dashboard.incyt.io) and downloaded as .csv spreadsheets.
Daily maximum and minimum temperature readings recorded at the Beerburrum Forest Station were downloaded from the Australian Bureau of Meteorology climate database (Australian Bureau of Meteorology 2024).
Cleaning data
Only those GPS readings with positional dilution of precision (PDOP) less than 5 (D’Eon and Delparte 2005) were retained and many zero (or very low) accelerometery readings that coincided with (and hence conflicted with) consecutive days of koala movement (according to GPS location data) were removed. Accuracy testing of the Lx system GPS units has revealed errors of some 34 m for stationary units (no PDOP provided) (Jiang 2022), so our data may lack sufficient precision to detect individual tree use (Kavanagh and Stanton 2012). However, the Lx units were found to reliably predict the location of koalas during tracking activity, and only accepting a location with a lower PDOP (e.g. 3) would have reduced the usable locations for our analysis. The Lx units are solar powered and store data onboard when no connectivity with the base station is available. For our cycle of 3-hourly location fixes, these Lx units retained battery charge throughout the study and did not need replacing.
Sorting data
To compare day and night activity, the GPS and accelerometric movement data were sorted into temporal categories for ‘night’ (22:00 hours day 1 to 10:00 hours day 2) and ‘day’ (the alternative period). Koalas are known to use more trees overnight than by day, and to move from non-food into food trees prior to midnight (Ellis et al. 1998; Pfeiffer et al. 2005). The timing of Lx devices’ GPS locations was variable and inconsistent, and it was not uncommon for reporting schedules to shift by several hours, or for sequential days to be absent of data. Distance moved was measured between two timestamps; hence, to ensure both the initial and final GPS locations that were used to calculate the movement were in daylight (or darkness for night), and to account for the inevitable drift that we encountered in timestamp, this selection of 10:00 hours (and 22:00 hours) was made. While arbitrary, the designation of ‘day’ beginning at the 10:00 timestamp excluded that period after dawn (during which koalas are known to move, potentially from food to rest trees (Goldingay pers. comm.; Melzer 1994; Melzer et al. 2011) because the measured period may include an initial GPS location that was recorded in darkness and, hence, movement may have been made in darkness.
Calculating distance travelled
Distance (in metres) between GPS coordinates was calculated with a version of the Haversine formula (Robusto 1957) adapted for use in Microsoft Excel. Unfeasibly large distances (e.g. indicating transcription errors) and any journeys less than 10 m were removed, as distances less than the average canopy diameter of a tree could be the result of a koala moving around in the same tree or simply reflect GPS position error (which may be as large as 34 m; Jiang 2022).
Calculating activity
To compare distance travelled to accelerometric activity per day or night, accelerometric readings were summed for the same time categories used for distance calculations. Removing time periods with numerous zero values (that did not correspond to periods of stationary GPS records), and only retaining data for koalas with more than 30 matching activity and distance of travel records, resulted in a minimum accepted dataset for analysis of distance moved, temperature and summed accelerometric measures.
Calculating home range
To compare the area used by each koala, and to compare Lx-generated data with manual tracking, we calculated minimum convex polygon (95%) in QGIS (QGIS Development Team, 2018. QGIS Geographic Information System. Open Source Geospatial Foundation. URL http://qgis.org). For reference with other work, we also calculated autocorrelated kernel density estimate (95%) home ranges for all koalas (Table 1).
Results
Activity and environmental data
For koalas with sufficient sequential activity data, there was no significant correlation between daily activity (accelerometery) and daily maximum temperature. Accelerometric data were generally bi-modal, with summed daily recordings across an order of magnitude (e.g. 1–100,000), so we examined log10-transformed data for activity against maximum daily temperature (Fig. 2). There was no relationship between temperature and activity.
Log10 total daily activity plotted against maximum daily temperature at Kinma Valley for male koala M1 (y = −0.1158x + 7.7388 R2 = 0.1466). Daily temperature data from Australian Bureau of Meteorology (2024).
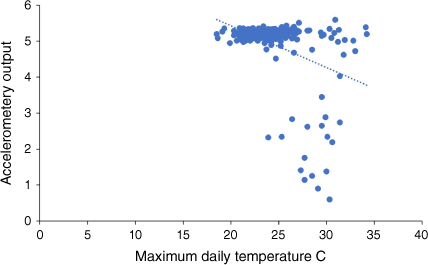
We also compared daily summed accelerometric data (log10) with daily minimum temperature, but there was no significant relationship (y = 0.0034x + 5.0213, R2 = 0.010. No relationship between the incidence or volume of rainfall and reported accelerometric activity or distance of travel was detected.
Daytime and nighttime activity and distance moved
Accelerometric activity and distance moved were not significantly correlated for koalas; however, koalas tended to move greater distances and record more accelerometric activity at night. Long-distance movement was generally accompanied by higher accelerometric activity, and some koalas were observed undertaking relatively long daytime journeys, but such events were uncommon. Some koalas had periods of very low or no movement for significant parts of the study, resulting in isolated clusters at the lower end of the activity axis. It is unclear whether these represent periods of prolonged inactivity (resting) or are the result of technical errors.
Despite this, the difference between the straight-line distance moved by day and by night was strongly significant and the variance in travel distance by night was approximately twice that by day. For example: M1: x̅day = 33 m, s.e. = 2.43, x̅night = 143 m, s.e. = 7.2, t241 = 14.6, P < 0.001; F1: x̅day = 37 m, s.e. = 2.6, x̅night = 49 m, s.e. = 3.5, t128 = 3.11, P = 0.001). Mean distance of nighttime travel events was between 102 and 143 m for males and between 29 and 54 m for females.
Seasonal variation in distance moved
Distance moved by both day and night for koalas increased between May and August, plateauing through the warmer months of the year. There was variation between individual koalas: M1 moved 474 m in total by day in June, while two other males travelled totals of 3000 and 1400 m for the same period. Similarly for females, F5 rarely moved by day in March (total 25 m) but travelled over 400 m by day in June. By night, across all koalas, the average total distance moved increased steadily after April, from a low that month of 791 m (s.e. = 252 m), to a peak of 2657 m (s.e. = 1023 m) in August (Fig. 3).
Home range
Males tended to have larger home ranges than females and one male (M1) had a range overlapping completely the range of two females while also appearing to exclude other males (Fig. 4). Based on the Lx data, male AKDE range averaged 47.1 ha (range 8.4–98.7 ha) and male MCP range averaged 36.7 ha (range 4.6–65.2 ha) compared to female AKDE range average of 13.3 ha (range 3.1–25.2 ha) and MCP range average of 12.5 ha (range 3.9–23.4 ha; Table 1).
Comparison of Lx-generated and manual tracking ranges
When compared for the same time period, the Lx data resulted in not significantly larger MCP range estimates compared to VHF-tracking data (Fig. 5: t4 = 2.16, P = 0.097 MCP), detecting areas of use that were not identified using the manual tracking method. Using the AKDE method, not significantly larger areas of use were predicted from the manual tracking data.
Discussion
The Lx tracking devices we deployed provided data on koala locations and activity, particularly alerting the research team to low activity, which could indicate sickness in koalas. However, the demands of our first hypothesis, that the activity reporting of the accelerometers would convey more detailed information on behaviour (and its energetic costs) for koalas were not met: we found no correlation between accelerometric data and movement data, temperature or rainfall. Our analysis of the relationship between environmental temperature (recorded ~30 km away) and koala activity and movement was inconclusive but leads us to propose that a more focussed approach that targets known periods of movement during temperature extremes could highlight the environmental drivers of activity, such as resting and travel.
There was a propensity for more zero or very low activity reports among female koalas compared to males, hindering analysis of relationships between environmental temperature and female koala activity. The data for male koalas also showed no relationship across temperature ranges of 18–37°C, perhaps attributable to the more variable activity reports at higher temperatures. The units report low activity through an alert system to the research team, meaning that any koalas that are stationary for long periods can be investigated, and it is probable that the equipment is not sensitive enough to differentiate across numerous activity levels, but rather to detect inactivity: this would explain the bi-modal data we recorded in this study for daily activity.
Diurnal patterns of activity and movement by koalas are well known (Martin and Handasyde 1999) and influence our understanding of various threats, such as roads in koala ecology (Dique et al. 2003; Ellis et al. 2016). The trend for greater accelerometric output and greater distances moved at night in this study are therefore unsurprising, and the lack of significant correlations between accelerometric data and movement data is further evidence of the limitations of the technology we are using. We conclude that the primary and most valuable benefit of the Lx collar accelerometery is its alerting of the research team to periods of low or no activity, which has, in other studies, allowed accurate determination of cause of death (Gentle et al. 2019; Robbins et al. 2019) and alerted teams to koalas in need of assistance (e.g. diseased: J. Hanger pers. comm).
The combination of accelerometery and GPS readings generated some contrasting data to describe relative activity between different koalas during the day and night, but analysis of this to determine the energetic cost of activity and travel was not possible. These observations confirmed that activity and distance travelled are greater at night for most koalas, which is not unexpected (Pfeiffer et al. 2005; Marsh et al. 2014; Ellis et al. 2016) and is probably an energy-saving strategy related to avoiding daytime heat (Ellis et al. 2010; Adam et al. 2021).
A key element of this study was the assessment of the effectiveness and convenience of the LX K-trackers. Overall, they yielded useful spatial and activity data which, when reported at regular intervals, was relatively easy to pair with environmental information, from which broader inferences and analyses may be made. Remote upload of data also increases the volume and regularity of data recorded, is cost-effective, reduces stress to wildlife and minimises biases resulting from observer proximity. The resolution and reporting schedule of the Lx units suggests that calculating daily energy budgets and real-time responses to environmental factors is possible. With this technology, it is possible to track very fine scale movements; for example, responses to disturbances such as light and noise.
The shortcomings of the technology include data errors and inconsistent reporting intervals which resulted in periods of missing data. To overcome this issue, time-consuming manual inspection and cleaning of data was necessary. In this study, there were instances of data loss for both accelerometery and GPS readings. Some degree of data loss in remote tracking is frequently reported and is often thought to be caused by signal interference from dense canopy (Cargnelutti et al. 2007; Frair et al. 2010; Matthews et al. 2013); but in our study, the increased MCP area identified as used by each koala based on Lx data, compared to manual tracking for the same time period, appears to provide a more comprehensive description of koala ranging area. In contrast, the AKDE range calculations, which provide a more accurate estimate of area used (as opposed to the total area between the data points we collected) did not return larger total areas of use using the Lx data compared to manual tracking and may better predict habitat use, for example. This outcome assumes that the GPS locations, of which there were many more than manual tracking fixes, were comparably accurate.
A pilot study using multi-axis accelerometers has demonstrated that information on activity type, as well as general activity, can be collected remotely for koalas (Ryan et al. 2013) and high-frequency GPS loggers have been used to detect distance and ground speed for koalas (Sparkes et al. 2023), so it is not unrealistic to predict that, in the future, artefact-free, remote observation of koala behaviour, constructed from GPS and accelerometery data, will be possible. For example, we have found that female travel is temporally correlated with male bellowing activity in the breeding season, and that bellowing activity is reduced during periods of high wind or extreme temperature (Ellis et al. 2011) and, using store-on-board GPS loggers, we found that koalas undertook longer distances of travel between 18:00 and 04:00 hours in southeast Queensland (Ellis et al. 2016).
However, ecological inferences that rely on the interpretation of accelerometric data are subject to limitations and errors that may be associated with sensor type and placement on the subject, and require precise calibration of sensors against subject activity (Garde et al. 2022). Our data describe the movement of koalas in a modified landscape but add information on relative activity in addition to distance moved. Coupled with environmental data, these observations can provide detail on koala behaviour to guide the construction of landscapes that meet the koalas’ physiological requirements, under predicted climate change scenarios and in disturbed landscapes. This will be an important element of any successful rehabilitation and revegetation work in climate change-affected and physically disturbed landscapes.
Acknowledgements
Koala capture and collaring was conducted under QLD Department of Agriculture and Fisheries Ethics permit CA 2021/07/1521 and QLD Scientific Purposes Permit WA0042976. Tanya Martin and Mitch Taylor assisted with access to the site.
References
28_South (2022). Koala Management Plan. Available at https://communities.lendlease.com/siteassets/qld/kinma-valley/approved-management-plans/pine-valley-koala-management-plan.pdf
Adam, D., Johnston, S. D., Beard, L., Nicolson, V., Gaughan, J. B., Lisle, A. T., FitzGibbon, S., Barth, B. J., Gillett, A., Grigg, G., and Ellis, W. (2020). Body temperature of free-ranging koalas (Phascolarctos cinereus) in south-east Queensland. International Journal of Biometeorology 64(8), 1305-1318.
| Crossref | Google Scholar | PubMed |
Adam, D., Johnston, S., Beard, L., Nicolson, V., Lisle, A., Gaughan, J., Larkin, R., Theilemann, P., and Ellis, W. (2021). Temporal effect of feeding on the body temperature and behaviour of captive koalas (Phascolarctos cinereus). Australian Mammalogy 44(1), 16-23.
| Crossref | Google Scholar |
Adams-Hosking, C., McAlpine, C., Rhodes, J. R., Grantham, H. S., and Moss, P. T. (2012). Modelling changes in the distribution of the critical food resources of a specialist folivore in response to climate change. Diversity and Distributions 18(9), 847-860.
| Crossref | Google Scholar |
Australian Bureau of Meteorology (2024). Beerburrum Forest Station Daily Minimum Temperature Climate Data. http://www.bom.gov.au/climate/data/
Barth, B., FitzGibbon, S., Gillett, A., Wilson, R., Moffitt, B., Pye, G., Adam, D., Preece, H., and Ellis, W. (2020). Scattered paddock trees and road-side vegetation can provide important habitat for koalas (Phascolarctos cinereus) in an agricultural landscape. Australian Journal of Mammalogy 42(2), 194-203.
| Crossref | Google Scholar |
Briscoe, N. J., Handasyde, K. A., Griffiths, S. R., Porter, W. P., Krockenberger, A., and Kearney, M. R. (2014). Tree-hugging koalas demonstrate a novel thermoregulatory mechanism for arboreal mammals. Biology Letters 10(6), 20140235.
| Crossref | Google Scholar | PubMed |
Cargnelutti, B., Coulon, A., Hewison, A., Goulard, M., Angibault, J. M., and Morellet, N. (2007). Testing global positioning system performance for wildlife monitoring using mobile collars and known reference points. The Journal of Wildlife Management 71(4), 1380-1387.
| Crossref | Google Scholar |
Chen, K. Y., and Bassett, D. R., Jr (2005). The technology of accelerometry-based activity monitors: current and future. Medicine and Science in Sports and Exercise 37(11), S490-S500.
| Crossref | Google Scholar | PubMed |
Crowther, M. S., Lunney, D., Lemon, J., Stalenberg, E., Wheeler, R., Madani, G., Ross, K. A., and Ellis, M. (2014). Climate-mediated habitat selection in an arboreal folivore. Ecography 37(4), 336-343.
| Crossref | Google Scholar |
Degabriele, R., and Dawson, T. J. (1979). Metabolism and heat balance in an arboreal marsupial the koala (Phascolarctos cinereus). Journal of Comparative Physiology 134(4), 293-301.
| Crossref | Google Scholar |
D’Eon, R. G., and Delparte, D. (2005). Effects of radio‐collar position and orientation on GPS radio‐collar performance, and the implications of PDOP in data screening. Journal of Applied Ecology 42(2), 383-388.
| Crossref | Google Scholar |
Dexter, C., Scott, J., Blacker, A., Appleby, R., Kerlin, D., and Jones, D. (2024). Koalas in space and time: Lessons from 20 years of vehicle‐strike trends and hot spots in South East Queensland. Austral Ecology 49(2), e13465.
| Crossref | Google Scholar |
Dique, D. S., Thompson, J., Preece, H. J., Penfold, G. C., de Villiers, D. L., and Leslie, R. S. (2003). Koala mortality on roads in south-east Queensland: the koala speed-zone trial. Wildlife Research 30(4), 419-426.
| Crossref | Google Scholar |
Dissanayake, R. B., Stevenson, M., Astudillo, V. G., Allavena, R., and Henning, J. (2023). Anthropogenic and environmental factors associated with koala deaths due to dog attacks and vehicle collisions in South-East Queensland, Australia, 2009–2013. Scientific Reports 13(1), 14275.
| Crossref | Google Scholar | PubMed |
Ellis, W., Melzer, A., Green, B., Newgrain, K., Hindell, M. A., and Carrick, F. N. (1995). Seasonal-variation in water flux, field metabolic-rate and food-consumption of free-ranging koalas (Phascolarctos cinereus). Australian Journal of Zoology 43(1), 59-68.
| Crossref | Google Scholar |
Ellis, W., Sullivan, B. J., Lisle, A. T., and Carrick, F. N. (1998). The spatial and temporal distribution of koala faecal pellets. Wildlife Research 25(6), 663-668.
| Crossref | Google Scholar |
Ellis, W., Melzer, A., Clifton, I., and Carrick, F. (2010). Climate change and the koala Phascolarctos cinereus: water and energy. Australian Zoologist 35(2), 369-377.
| Crossref | Google Scholar |
Ellis, W., Bercovitch, F., FitzGibbon, S., Roe, P., Wimmer, J., Melzer, A., and Wilson, R. (2011). Koala bellows and their association with the spatial dynamics of free-ranging koalas. Behavioral Ecology 22(2), 372-377.
| Crossref | Google Scholar |
Ellis, W. A., FitzGibbon, S. I., Barth, B. J., Niehaus, A. C., David, G. K., Taylor, B. D., Matsushige, H., Melzer, A., Bercovitch, F. B., Carrick, F., Jones, D. N., Dexter, C., Gillett, A., Predavec, M., Lunney, D., and Wilson, R. S. (2016). Daylight saving time can decrease the frequency of wildlife–vehicle collisions. Biology Letters 12, 20160632.
| Crossref | Google Scholar | PubMed |
Frair, J. L., Fieberg, J., Hebblewhite, M., Cagnacci, F., DeCesare, N. J., and Pedrotti, L. (2010). Resolving issues of imprecise and habitat-biased locations in ecological analyses using GPS telemetry data. Philosophical transactions of the Royal Society of London. Series B, Biological Sciences 365, 2187-2200.
| Crossref | Google Scholar | PubMed |
Garde, B., Wilson, R. P., Fell, A., Cole, N., Tatayah, V., Holton, M. D., Rose, K. A. R., Metcalfe, R. S., Robotka, H., Wikelski, M., Tremblay, F., Whelan, S., Elliott, K. H., and Shepard, E. L. C. (2022). Ecological inference using data from accelerometers needs careful protocols. Methods in Ecology and Evolution 13(4), 813-825.
| Crossref | Google Scholar | PubMed |
Gentle, M., Allen, B. L., Oakey, J., Speed, J., Harriott, L., Loader, J., Robbins, A., de Villiers, D., and Hanger, J. (2019). Genetic sampling identifies canid predators of koalas (Phascolarctos cinereus) in peri-urban areas. Landscape and Urban Planning 190, 103591.
| Crossref | Google Scholar |
Goldingay, R. L., and Dobner, B. (2014). Home range areas of koalas in an urban area of north-east New South Wales. Australian Mammalogy 36(1), 74-80.
| Crossref | Google Scholar |
Kavanagh, R., and Stanton, M. (2012). Koalas use young Eucalyptus plantations in an agricultural landscape on the Liverpool Plains, New South Wales. Ecological Management & Restoration 13, 297-305.
| Crossref | Google Scholar |
Logan, M., and Sanson, G. (2002). The effect of tooth wear on the feeding behaviour of free-ranging koalas (Phascolarctos cinereus, Goldfuss). Journal of Zoology (London) 256, 63-69.
| Crossref | Google Scholar |
Madani, G. F., Ashman, K. R., Mella, V. S. A., and Whisson, D. A. (2020). A review of the ‘noose and flag’ method to capture free-ranging koalas. Australian Mammalogy 42(3), 341-348.
| Crossref | Google Scholar |
Marsh, K. J., Moore, B. D., Wallis, I. R., and Foley, W. J. (2014). Continuous monitoring of feeding by koalas highlights diurnal differences in tree preferences. Wildlife Research 40(8), 639-646.
| Crossref | Google Scholar |
Matthews, A., Ruykys, L., Ellis, B., FitzGibbon, S., Lunney, D., Crowther, M., Glen, A., Purcell, B., Moseby, K., Stott, J., Fletcher, D., Wimpenny, C., Allen, B., Van Bommel, L., Roberts, M., Davies, N., Green, K., Newsome, T., Ballard, G., Flemming, P., Dickman, C., Eberhart, A., Troy, S., McMahon, C., and Wiggins, N. (2013). The success of GPS collar deployments on mammals in Australia. Australian Mammalogy 35(1), 65-83.
| Crossref | Google Scholar |
Melzer, A., Baudry, C., Kadiri, M., and Ellis, W. (2011). Tree use, feeding activity and diet of koalas on St Bees Island, Queensland. Australian Zoologist 35(3), 870-875.
| Crossref | Google Scholar |
Mitchell, DL, Soto-Berelov, M, and Jones, SD (2023). Remote sensing shows south-east Queensland koalas (Phascolarctos cinereus) prefer areas of higher tree canopy height within their home ranges. Wildlife Research 50(11), 939-953.
| Crossref | Google Scholar |
Murgatroyd, M., Tate, G., and Amar, A. (2023). Using GPS tracking to monitor the breeding performance of a low-density raptor improves accuracy, and reduces long-term financial and carbon costs. Royal Society Open Science 10(8), 221447.
| Crossref | Google Scholar | PubMed |
Nagy, K. A., and Martin, R. W. (1985). Field Metabolic Rate, Water Flux, Food Consumption and Time Budget of Koalas, Phascolarctos cinereus (Marsupialia: Phascolarctidae) in Victoria. Australian Journal of Zoology 33(5), 655-665.
| Crossref | Google Scholar |
Narayan, E. (2019). Physiological stress levels in wild koala sub-populations facing anthropogenic induced environmental trauma and disease. Scientific Reports 9(1), 6031.
| Crossref | Google Scholar | PubMed |
Pfeiffer, A., Melzer, A., Tucker, G., Clifton, D., and Ellis, W. (2005). Tree use by koalas (Phascolarctos cinereus) on St Bees Island, Queensland - Report of a pilot study. Proceedings of the Royal Society of Queensland 112, 47-51.
| Google Scholar |
Rhodes, J. R., Callaghan, J. G., McAlpine, C. A., de Jong, C., Bowen, M. E., Mitchell, D. L., Lunney, D., and Possingham, H. P. (2008). Regional variation in habitat-occupancy thresholds: a warning for conservation planning. Journal of Applied Ecology 45(2), 549-557.
| Crossref | Google Scholar |
Robbins, A., Loader, J., de Villiers, D., Beyer, H. L., and Hanger, J. (2019). Predation by carpet pythons (Morelia spilota) is an important cause of mortality in a free-living koala (Phascolarctos cinereus) population in South East Queensland. Australian Veterinary Journal 97(9), 351-356.
| Crossref | Google Scholar | PubMed |
Robusto, C. C. (1957). The cosine-haversine formula. The American Mathematical Monthly 64(1), 38-40.
| Crossref | Google Scholar |
Rus, A. I., McArthur, C., Mella, V. S., and Crowther, M. S. (2021). Habitat fragmentation affects movement and space use of a specialist folivore, the koala. Animal Conservation 24(1), 26-37.
| Crossref | Google Scholar |
Ryan, M. A., Whisson, D. A., Holland, G. J., and Arnould, J. P. (2013). Activity patterns of free-ranging koalas (Phascolarctos cinereus) revealed by accelerometry. PLoS One 8(11), e80366.
| Crossref | Google Scholar | PubMed |
Sparkes, G., Wilson, O., Del-Simone, K., Amir-Abdul-Nasir, A. F., Barth, B., FitzGibbon, S., Ellis, W., and Wilson, R. (2023). The secret life of koalas: using accelerometry to quantify fine-scale behaviours in the wild. Integrative and Comparative Biology 63, S435-S436.
| Google Scholar |
Tomkiewicz, S. M., Fuller, M. R., Kie, J. G., and Bates, K. K. (2010). Global positioning system and associated technologies in animal behaviour and ecological research. Philosophical Transactions of the Royal Society of London. Series B, Biological Sciences 365, 2163-2176.
| Crossref | Google Scholar | PubMed |
Whisson, D. A., Zylinski, S., Ferrari, A., Yokochi, K., and Ashman, K. R. (2020). Patchy resources and multiple threats: how do koalas navigate an urban landscape? Landscape and Urban Planning 201, 103854.
| Crossref | Google Scholar |