Detection of rabbit and wombat warrens in broad-scale satellite imagery
Natarsha McPherson

A
Abstract
The ability to accurately assess the spatial pattern of wild animal distributions is essential for conservation management. Warrens constructed by burrowing species offer proxies for estimating geographic occupation. We tested the feasibility of open-access satellite-based detection for two semi-fossorial mammals, the southern hairy-nosed wombat (Lasiorhinus latifrons) and European rabbit (Oryctolagus cuniculus), across the Nullarbor Plain, South Australia. Along two 100 km road sections, we collected GPS locations of warrens from ground-walked transects (~1 km) at 22 sites. Wombat and rabbit warrens were identified and digitised using Google Earth and Microsoft Bing imagery (<1.5 m resolution) for each transect. We found a significant correlation between satellite and field estimates with R2 values of 0.98 (P < 1 × 10−15) and 0.56 (P < 1 × 10−4) for wombat and rabbit warrens, respectively. User accuracy was high for both wombat (91%) and rabbit (81%) warrens. Omission in the satellite imagery was low for wombats (14%) but high for rabbit warrens (44%). However, small warrens less than 10 m in diameter accounted for 79% of rabbit warren omissions. This demonstrates that the geospatial pattern of warrens constructed by two semi-fossorial mammals can be detected and distinguished in broad-scale satellite imagery across Australia’s semi-arid landscape.
Keywords: burrowing, rabbits, remote detection, satellite imagery, semi-fossorial mammals, species distribution, wildlife monitoring, wombats.
Introduction
The capacity to accurately assess the geospatial pattern of wild animal distributions is essential to conservation planning and natural resource management, providing a foundation for evidence-based decision making and targeted intervention (Burgess et al. 2020; Delplanque et al. 2024). Spatial distribution patterns can provide valuable insights into how animals utilise their habitat, interact with the environment, and respond to altered landscapes (Otis 1998; Estevo et al. 2017; Wagner et al. 2021). However, attaining accurate and representative datasets that reliably portray geographic occupancy is restricted by cost, labour, and accessibility (Witmer 2005; Ostendorf 2011; Bachman et al. 2019; Taggart et al. 2020; Johnston et al. 2023). Wildlife survey data has historically been collected via field surveys and in situ sensors (e.g. camera traps) (Borchers and Marques 2017; Nuttall et al. 2022; Bassing et al. 2023). However, these methods are often insufficient for monitoring remote regions due to limited site accessibility, low population densities, and widespread movement of species (Camino et al. 2020; Southwell et al. 2023). Additionally, these methods incur high investment into resources and maintenance (Witmer 2005; Seidlitz et al. 2021; Tuia et al. 2022) that can only provide limited coverage (Duckworth 1998; Steinbeiser et al. 2019; Cappelle et al. 2021). This introduces the risk of sampling bias that can skew inferences about distributions (Phillips et al. 2009; Molyneux et al. 2017; Cosentino and Maiorano 2021).
Remote wildlife detection using satellite imagery offers a non-invasive alternative to identify individual animals and monitor movement across large spatial extents (Hollings et al. 2018; Attard et al. 2024). The approach allows wildlife detection for different scales and conservation objectives, ranging from the management of local colonies to the assessment of broad-scale animal distribution patterns (Lynch and Schwaller 2014; Ainley et al. 2015). Recent applications have included the manual digitisation and training of deep learning models, targeting the detection of a wide selection of terrestrial and marine species (Rivas et al. 2018; Bolyn et al. 2022), including penguin colonies (Fretwell and Trathan 2009; Fretwell et al. 2021), polar bears (Stapleton et al. 2014; Chabot et al. 2022), and elephants (Duporge et al. 2021). Within the past decade, the increasing application of remote wildlife detection methods has been facilitated by advances in accessibility, resolution, temporal frequency, and the reduction of cost for aerial (uncrewed aerial vehicles, helicopter, fixed wing) and satellite imagery (Wang et al. 2010; Hollings et al. 2018; Tuia et al. 2022; Attard et al. 2024).
Despite these advances, accurately detecting cryptic species and those that inhabit heterogeneous landscapes remains challenging (Hollings et al. 2018). Remote detection is possible using thermal imaging in some cases (Chrétien et al. 2016; Psiroukis et al. 2021), but the high resolution required with this approach limits spatial extent. These species are also difficult to survey individually with traditional field-based approaches, such as spotlighting and capture–recapture methods, which are dependent on scale and the detection probability of individuals (Ingram et al. 2019; Matthias et al. 2021). However, burrows and burrow clusters (warrens) can provide effective proxies for estimating distribution and density (Matthews et al. 2010; Jansen et al. 2023; Knoblauch et al. 2023; Norris et al. 2024). Modifying the landscape, burrowing species are ecosystem engineers that construct and rely upon burrows for refugia; preventing excessive energy expenditure and water loss in regions of harsh environmental exposure (e.g. solar radiation) and minimal canopy cover (Steele and Temple-Smith 1998; Finlayson et al. 2005; Whittington-Jones et al. 2011; Pike and Mitchell 2013; Ostendorf et al. 2016). Subsequently, warrens are often shared systems for a range of burrowing and non-burrowing occupants (Read et al. 2008; Thornett et al. 2017; Dean et al. 2023). Due to the strong biophysical associations developed between fossorial species, their warrens, and landscape (Finlayson et al. 2005), these ground features can present a spatially accurate proxy of presence and distribution (Löffler and Margules 1980; Swinbourne et al. 2021).
Detecting wildlife or proxies from satellite imagery is critical for understanding spatial distributions of species with high conservation priority and the trends of invasive species. Remote sensing technologies enable broad-scale, non-invasive monitoring of animal populations and their habitats (Delplanque et al. 2024). However, few studies have explored the capacity of remote sensing approaches to directly compare the distribution of multiple species. Such comparative analyses are essential for addressing complex conservation challenges that involve interspecific competition, predator–prey relationships, or the impacts of invasive species on ecosystems (Pedler et al. 2016). Understanding how invasive species overlap with native species distributions can reveal patterns of resource competition and habitat partitioning that influence population viability and ecosystem health (Vidal‐García and Keogh 2017; Shalom et al. 2024). This information can then be applied to the prioritisation of areas for control efforts or habitat restoration (Allen et al. 2006; Elith et al. 2017). The development of robust remote sensing frameworks, therefore, provides the foundation for addressing broader management questions and conservation strategies.
The Nullarbor Plain is an expansive, remote, and semi-arid region spanning from the south-west of South Australia to the south-eastern corner of Western Australia. The region comprises the largest sub-population of the southern hairy-nosed wombat (Lasiorhinus latifrons) and a widespread population of the invasive European rabbit (Oryctolagus cuniculus) (Gillieson et al. 1996; Swinbourne et al. 2021). Residing across a combination of nature reserves, Indigenous land, and pastoral property, the wombat is a species of high conservation priority. However, their construction of warrens also conflicts with agriculture and pastoralism (O’Brien et al. 2021). The rabbit is a widespread invasive pest that increases soil erosion, weed abundance, and competition for resources (Cooke 2012; Finlayson et al. 2022). For both species, the knowledge of distribution and activity is limited by the region’s vast scale and restricted accessibility (Cox et al. 2013). This lack of information reduces the capacity to effectively determine conservation risk and the scale/distribution of control methods required for pest management. Because of the vast extent of the region, satellite remote sensing of burrowing is the only practical approach to obtain baseline distribution information of these two species (Delplanque et al. 2024). With minimal canopy cover and impact from anthropogenic disturbance, the largely open landscape of the Nullarbor Plain is an ideal homogeneous study site for comparing and assessing the remote detection capacity of these species (Gillieson et al. 1996; Swinbourne et al. 2018; Webb and James 2023).
In this study, we investigated the efficacy of open-source satellite imagery in distinguishing and mapping wombat and rabbit warrens in the Nullarbor region. We used field validation surveys to identify the capacity and constraints associated with this approach. Ascertaining this information is essential to building foundational distribution knowledge of cryptic, fossorial species in remote landscapes.
Methods
Field surveys were carried out in March and September of 2023 across the Nullarbor Plain in South Australia. Two road transects of 100 km length along Ooldea Road and Cook Road (Fig. 1) were surveyed with sites selected at 10 km intervals (n = 22). At each site, observers walked looping transects in three different directions for approximately 1 km (Fig. 2). An agile approach was implemented to allow observers to slightly adjust walking direction, ensuring maximum warren coverage along the loops. We recorded GPS positions of all warrens that were within approximately 25 m of the transect line, noting wombat, rabbit, and shared warren use. The number of active and inactive burrows (entrances), along with an estimate of warren diameter, were assessed. The GPS tracks and waypoint data were collected using Garmin eTrex 10 devices, with resultant spatial data processed in ArcGIS Pro software (version 3.1.2).
Field survey sites for the southern hairy-nosed wombat and European rabbit warren sampling along two road transects, Cook (n = 10) and Ooldea (n = 12) Road, that are located along the Nullarbor Plain, South Australia. Photographs by N. McPherson.
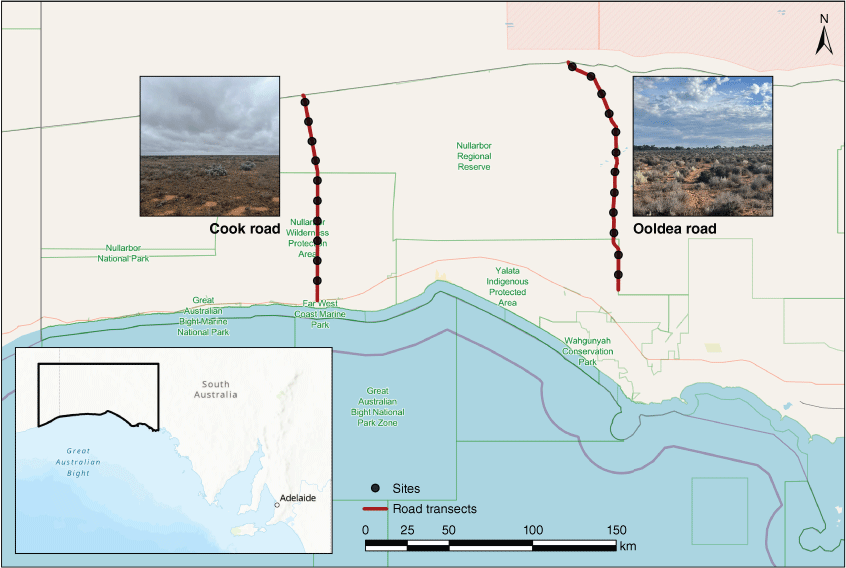
Schematic of field data collection at a survey site and comparison with satellite image interpretations. We identified GPS locations of rabbit and wombat warrens in the field along sampling tracks and in a 25 m buffer on satellite imagery in ArcGIS Pro. Agreed observations, omission, and commission errors were identified for rabbit and wombat warrens at each site.
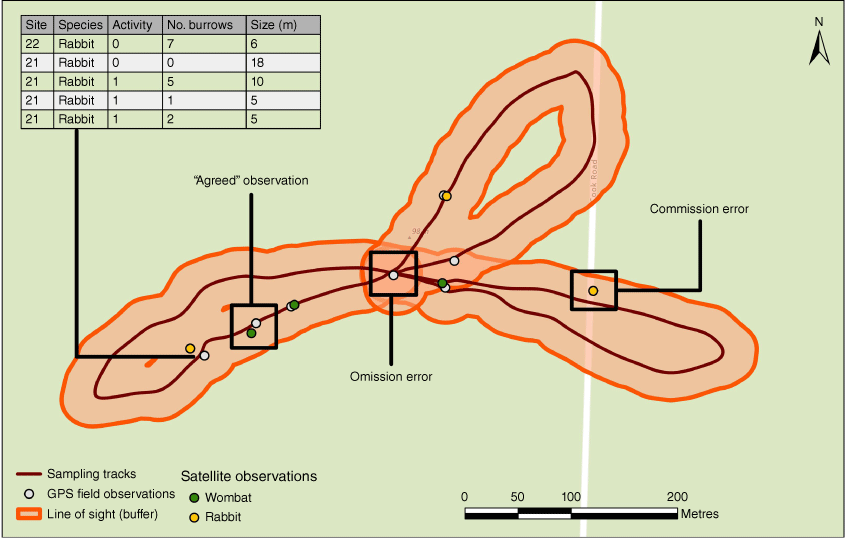
A combination of Bing maps and Google Earth imagery (<1.5 m resolution) was interpreted using a buffer with a 25 m distance from recorded survey tracks. Warrens of the southern hairy-nosed wombat exhibit distinctive grazing patterns, tracks, and spoil mounds that have allowed highly representative estimates of spatial distribution and abundance across an array of landscapes (Löffler and Margules 1980; Swinbourne et al. 2021). In contrast, rabbit warrens do not have tracks and demonstrate less distinct spoil mounds. Satellite imagery surveys yielded an average site area of 15 ha and a total 330 ha of area that was examined for the presence of warrens. Interpretation of imagery buffers was performed without visualisation of field-surveyed GPS waypoints, and all wombat and rabbit warrens were digitised for quantitative analysis.
We overlayed field-based and satellite-derived locations and manually examined the 22 site buffers for agreement (true positive), the presence of omission (false negative) in both methods, and commission (false positive) in the satellite interpretation (Fig. 2).
Observations were considered in agreement if satellite interpretations were recorded within 5 m of field-derived locations to account for field positioning and the margin of error in GPS accuracy. Omission errors occurred in both field and satellite methods. In the field, surveyors may have missed warrens within a 25 m buffer distance due to obscuration from vegetation or terrain. In satellite imagery, warrens were likely to be omitted if they were too small relative to the image resolution or obscured by vegetation. If landscape features were misinterpreted as warrens, commission errors could have occurred. However, commission errors could not occur in the field as warrens of both species could be distinctly identified and discriminated. Thus, we evaluated the total number of agreements per site and all omission and commission errors. While field observations of warrens were categorised as wombat, rabbit, or shared, satellite interpretation could only differentiate features as wombat or rabbit warrens. Subsequently, waypoints labelled as shared warren structures were attributed to the majority count of burrow attribution and counted as an omission error for the minority. In most cases, this resulted in an omission for rabbit warrens.
We further established that the best estimate of the true field warren count within the buffer area comprised the sum of field observations and field omissions. Linear regression was conducted to identify general trends of the remote methodology, assessing how the satellite estimates corresponded to the best estimate.
Results
During ground surveys, we recorded 85 wombat, 125 rabbit, and 29 shared warrens (for a total of 114 wombat and 154 rabbit warrens). Of the 29 shared warrens, 23 were attributed by burrow counts to wombats, three to rabbit, and three were omitted for both species during satellite interpretation. From satellite imagery, we interpreted 107 wombat and 100 rabbit warrens. By comparison between sampling methods, 17 wombat and 73 rabbit warrens were omitted in the satellite imagery, while five wombat and 13 rabbit warrens were omitted in the field (Table 1). Subsequently, we derived the best estimate for the wombat and rabbit with 119 (114 + 5) and 167 (154 + 13) warrens, respectively.
Species | GPS recordsA | Satellite detections | Agreed | Omission | Commission | Best estimateB | ||
---|---|---|---|---|---|---|---|---|
Satellite | Field | Satellite | ||||||
Wombat | 114 (85 + 29) | 107 | 97 | 17 | 5 | 5 | 119 (114 + 5) | |
Rabbit | 154 (125 + 29) | 100 | 81 | 73 | 13 | 6 | 167 (154 + 13) |
The study covered 22 sites along two road transects wherein both field and satellite imagery (<1.5 m resolution) were used to count wombat and rabbit warrens. The table summarises the comparison between field and satellite estimates for each species. A detailed table with records per road transect is also available as Supplementary Table S2.
Wombat warrens were recorded at 10 out of the 22 sites assessed, while rabbits were identified at 21. Shared warrens were identified in the field for 10 sites but could not be distinguished from satellite interpretation. Out of the 10 sites with shared warrens, eight had additional records for both individual wombat and rabbit warrens. A detailed table of field observations is available as Supplementary Table S1.
Comparison of the number of warrens detected on satellite imagery with the best estimate of the number of warrens at each site yielded highly significant relationships with coefficient of determination (R2) values of 0.98 (P < 1 × 10−15) and 0.56 (P < 1 × 10−4) for wombat and rabbit warrens, respectively. Both relationships showed a positive regression slope close to the one-to-one line (Fig. 3).
Linear regression between the best estimate of wombat and rabbit warren counts and satellite interpretation. The best estimate is calculated as the sum of field observations and omissions. For comparison, the one-to-one line is presented as a dashed line. The results demonstrate a strong positive relationship between satellite observations and ground conditions, highlighting that satellite interpretation is a valid method to distinguish wombat and rabbit warrens.
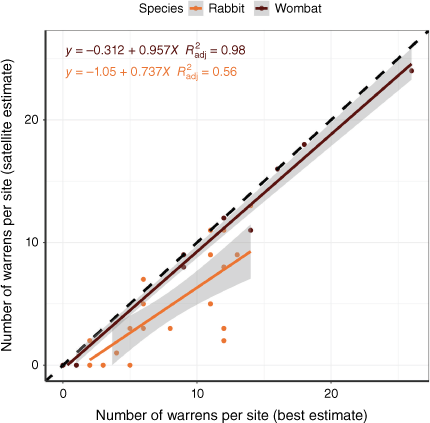
For both species, warrens that were detected in the satellite imagery corresponded well with field records. User accuracy for wombats and rabbits was 91% (97/107) and 81%, (81/100), respectively. However, overall agreement between satellite interpretation and field observations varied, with an 82% (97/119) agreement for wombat and 49% (81/167) agreement for rabbit warrens (Table 1). Omission rates for warrens in the satellite imagery were 14% (17/119) for wombat and 44% (73/167) for rabbit. The low agreement for rabbit warrens was largely due to omission rates of small warrens; 79% of omissions were attributed to warrens less than 10 m in diameter and 21% for the larger warrens (Fig. 4).
Proportion of rabbit satellite omissions (omissions/total omissions) by size class. The number of warrens (field observations) and omissions (on satellite) within size classes are shown on top of the columns. Most of the identified errors appear to occur for small warrens. Size classes less than 10 m account for 79% of the rabbit satellite omissions. The omission rate of larger rabbit warrens was 10/48 (21%).
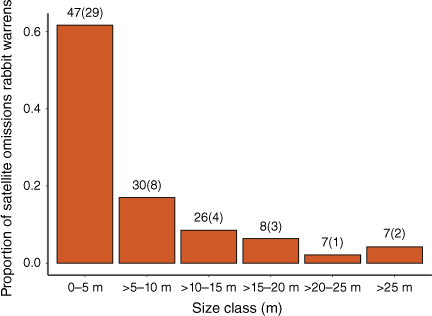
Discussion
Warrens of fossorial species can be identified and distinguished in open-source satellite imagery. This has been shown previously for several species (Koshkina et al. 2019; Bentze et al. 2023), but no prior work has assessed validation efforts, limiting factors, or shown that a systematic approach is possible for two interacting species. Our analysis subsequently shows that remote warren detection in the Nullarbor Plain can provide accurate records of broad-scale geospatial pattern. For both wombat and rabbit warrens, we found a significant positive relationship between satellite and field estimates. Regression results for both species suggest that these relationships produced a reliable fit between observed and predicted values, which was further supported by the high user accuracy for wombat (91%) and rabbit (81%) warrens. In this study, we also observed low commission errors during satellite interpretation, but high omission errors for the detection of rabbit warrens. In this discussion, we highlight and assess the successes and limitations of this approach, and identify the key potential factors contributing to detection errors.
Identification of wombat warrens in satellite imagery
Using high-resolution and open-access satellite imagery (i.e. Bing maps), we observed high discrimination (82%) of wombat warrens recorded in the field. This observed discrimination capacity is consistent with Swinbourne et al. (2018) who identified that the detection of wombat warrens is largely facilitated by the size of spoil mounds, the presence/absence of interconnected surface tracks, and the extent of grazing in the landscape. During the excavation process, high soil turnover creates elevated spoil mounds that are uniquely of lighter contrast and distinct from the underlying landscape (Shimmin et al. 2002; Haussmann 2017; Swinbourne et al. 2018). Social interactions produce extensive connectivity between warrens (Shimmin et al. 2002; Finlayson et al. 2005; Walker et al. 2007). Track patterns of these interactions are visually distinct from tracks associated with stock watering points and other grazers (Swinbourne et al. 2018), and are critical determinants for identification (Fig. 5). Previously, the detection of warren clusters from broad-scale satellite imagery was reliant on the distinctiveness and presence of grazing ‘halos’ (Löffler and Margules 1980). However, the broad availability of high-resolution satellite imagery now enables direct identification of individual wombat warrens.
Identification of rabbit warrens in satellite imagery
We found a high user accuracy of 81% for detecting rabbit warrens using broad-scale satellite imagery. Large rabbit warrens were identified in the satellite imagery through a change in surface colour arising from vegetation removal and the mixing of surface and subsurface soil. Rabbit warrens could also be distinguished by shape (generally circular or oval) with smooth edges that reflected a continuous activity gradient with distance from burrows. The Nullarbor landscape has little terrain variation and distinct surface features including claypans, dolines, and calcrete outcrops that facilitate object discrimination and detection (Webb and James 2023). This is in contrast to challenges identified in studies evaluating the utility of remote wildlife detection upon individuals in more densely vegetated and heterogeneous landscapes (Jachmann 2002; Chrétien et al. 2016; Hollings et al. 2018; Corcoran et al. 2021). Previously, rabbit burrows have been detected using thermal imaging (Cox et al. 2021), aerial surveys (Martin and Zickefoos 1976), and more recently high-resolution satellite imagery (Bentze et al. 2023). However, in regions with more heterogeneous terrain and vegetation, field-based surveys have been required to map distribution and density (Jansen et al. 2023). Furthermore, rabbit warrens in the study region can be very large, comprising a high number of clustered burrows that reflect the landscape’s extreme climate conditions and further enable remote identification.
However, the interpretation of small rabbit warrens on satellite imagery is limited by resolution. Compared to the warrens of the southern hairy-nosed wombat, the resolution of Google Earth and Bing maps satellite imagery was too low to show detail and detect small rabbit warrens. Moderate discrimination of field-recorded warrens from satellite imagery was observed (49%), with a 44% omission of warrens in the field. Rabbit warrens frequently demonstrated a lower relative height of spoil mounds, lacked prominent surface tracks, and could be confused with bare soil patches (Fig. 6). Shape and size of their warrens were also less consistent across the observed landscape. Thus, it was only possible to identify large warrens in areas with little vegetation obscuration and high edaphic contrast.
(a) High-resolution imagery (<1 m) of a detected rabbit warren from Bing maps with a comparison of detail captured by (b) drone imagery (<2 cm). Individual burrows of the rabbit are too small to be detected at the current resolution of available satellite imagery, yet can be detected in (c) drone imagery.
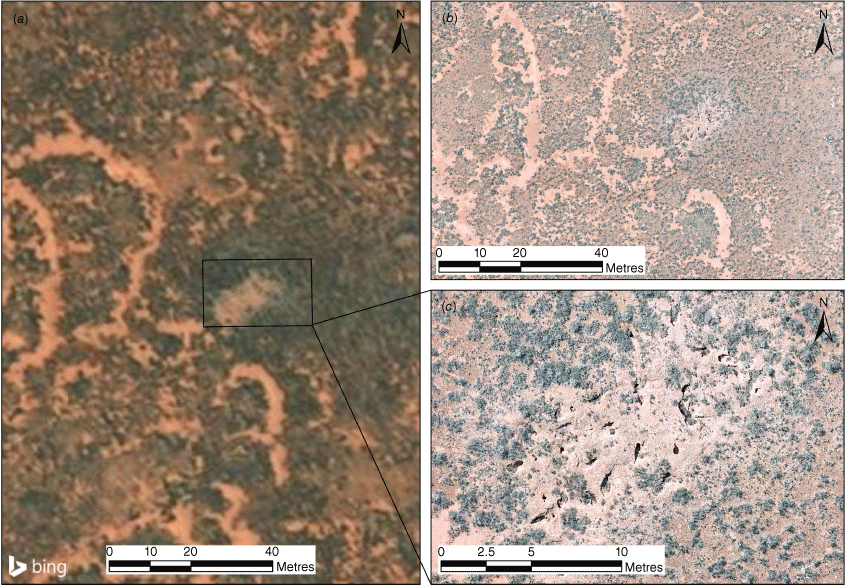
Analysis of field data demonstrated that for the rabbit, a warren size larger than 5–10 m was necessary for reliable detection. Rabbit warrens with an approximate diameter of less than 10 m comprised 79% of the observed rabbit warren satellite omissions. Although many contributing factors influence the detectability of warrens, the size-to-pixel ratio was likely a large contributing determinant of the study’s methodology. As the assessed satellite imagery was estimated to have a pixel size range between 0.5 and 1.5 m, the minimum detectable warren size was assumed to be approximately 10–20 times the pixel width. This may highlight the difference in omission frequency between the rabbit and wombat warren detection. Small wombat warrens were often at or above a minimum detectable warren size. This is consistent with the lower accuracies observed in older studies that used coarser resolution imagery (Löffler and Margules 1980). These results further indicate that finer image resolution produced by upcoming satellites, and the increased accessibility to high-resolution imagery, may allow the reliable mapping of smaller warrens.
The process of manual digitisation to identify warrens in open-source imagery is a labour-intensive task that is susceptible to user biases (Smith and Pinter‐Wollman 2021; Sudholz et al. 2022). In contrast, automated approaches, such as deep learning, have demonstrated success in tracking individuals and their proxies (Gedeon et al. 2022; Łopucki et al. 2022). This may provide increased efficacy for broad-scale data collection across Australia’s remote regions in the future, but high-resolution open-access imagery is currently unavailable and commercial imagery remains cost-prohibitive at broad spatial scales (Attard et al. 2024).
Management implications
The feasibility of assessing spatial patterns of wombat and rabbit warrens has significant management implications. Our results suggest that spatial distribution patterns of multiple fossorial species can be reliably detected and distinguished. The Nullarbor bioregion encompasses approximately 200,000 km2 of area and on-ground actions can only cover small, prioritised regions (Finlayson et al. 2022). Although the data does not enable precise population estimates, warren distributions can potentially serve as indicators of relative population change when high-resolution imagery is used. This may be achieved through the repeated assessment of open-source imagery over time or by commissioning detailed imagery for smaller target extents. Spatially explicit population numbers may be obtained after calibration with representative field data (Swinbourne et al. 2021; Bentze et al. 2023). Data collected from satellite imagery may also be used to stratify landscapes for broad-scale survey efforts, allowing for more informed site selection and prioritisation of field surveys.
Species distribution models have also been useful for management, generating relative patterns of distribution without absolute population estimates (Sinclair et al. 2010; Sofaer et al. 2019). Generally, these methods utilise a combination of data derived from citizen science efforts or a collation of field survey observations and preserved specimens (Elith and Leathwick 2009; Feldman et al. 2021). Biases arising from a lack of sampling representation can produce models with overfitting (Kramer‐Schadt et al. 2013). Subsequent efforts to implement models into management require detailed and balanced occurrence data to more accurately characterise habitat suitability and distribution (Sofaer et al. 2019; Zurell et al. 2020). This study demonstrates that open-source satellite imagery offers a cost-effective, scalable solution to establish the spatial foundation for effective wildlife and habitat management.
The ability to correctly detect obvious and contrasting warrens in publicly available imagery offers high potential towards broad-scale sampling and monitoring. As financial and logistic constraints limit the capacity to determine conservation risk (Witmer 2005; Wintle et al. 2019; Woinarski et al. 2021), a non-invasive and cost-effective approach to monitoring and data acquisition can enhance the basic understanding of potential ecosystem responses and improve management in remote regions.
Conclusion
For species such as the southern hairy-nosed wombat and European rabbit, establishing the widespread distribution across the Nullarbor Plain is essential for determining future populations and the impact of environmental interactions. Our findings establish that discrimination of warrens from open-access satellite imagery is greatest for the wombat and influenced by a combination of feature size, connectivity, and image resolution. Satellite interpretation allows the description of broad-scale trends of geographic occupation; however, the detection of small warrens is limited by the pixel resolution of available satellite imagery. The detection of wombat and rabbit warrens from broad-scale satellite imagery is a valid approach in the production of spatially unbiased species distribution datasets and can increase the understanding of species interactions in remote arid and semi-arid landscapes.
Data availability
The data that support this study will be shared upon reasonable request to the corresponding author.
Declaration of funding
Funding was supplied to the first author through the University Research Grant provided by the Australian Wildlife Society.
Acknowledgements
We acknowledge and thank the Yalata Aboriginal Community, Far West Coast Aboriginal Corporation, and Department of Environment and Water for research management and local logistic support. Thank you to Gemma Sansom and Dr David Taggart from The University of Adelaide for their assistance during data collection and to the Unmanned Research Aircraft Facility (URAF) for drone support.
References
Ainley, D. G., Larue, M. A., Stirling, I., Stammerjohn, S., and Siniff, D. B. (2015). An apparent population decrease, or change in distribution, of Weddell seals along the Victoria Land coast. Marine Mammal Science 31(4), 1338-1361.
| Crossref | Google Scholar |
Allen, C. R., Johnson, A. R., and Parris, L. (2006). A Framework for Spatial Risk Assessments: Potential Impacts of Nonindigenous Invasive Species on Native Species. Ecology and Society 11(1), 39.
| Crossref | Google Scholar |
Attard, M. R. G., Phillips, R. A., Bowler, E., Clarke, P. J., Cubaynes, H., Johnston, D. W., and Fretwell, P. T. (2024). Review of satellite remote sensing and unoccupied aircraft systems for counting wildlife on land. Remote Sensing 16(4), 627.
| Crossref | Google Scholar |
Bachman, S. P., Field, R., Reader, T., Raimondo, D., Donaldson, J., Schatz, G. E., and Lughadha, E. N. (2019). Progress, challenges and opportunities for Red Listing. Biological Conservation 234, 45-55.
| Crossref | Google Scholar |
Bassing, S. B., DeVivo, M., Ganz, T. R., Kertson, B. N., Prugh, L. R., Roussin, T., Satterfield, L., Windell, R. M., Wirsing, A. J., and Gardner, B. (2023). Are we telling the same story? Comparing inferences made from camera trap and telemetry data for wildlife monitoring. Ecological Applications 33(1), e2745.
| Crossref | Google Scholar |
Bentze, C., Burningham, H., and Magee, E. (2023). Down the rabbit-hole: satellite-based analysis of spatiotemporal patterns in wild European rabbit burrows for better coastal dune management. Journal of Coastal Conservation 27(6), 61.
| Crossref | Google Scholar |
Bolyn, C., Lejeune, P., Michez, A., and Latte, N. (2022). Mapping tree species proportions from satellite imagery using spectral–spatial deep learning. Remote Sensing of Environment 280, 113205.
| Crossref | Google Scholar |
Borchers, D. L., and Marques, T. A. (2017). From distance sampling to spatial capture–recapture. AStA. Advances in Statistical Analysis 101(4), 475-494.
| Crossref | Google Scholar |
Burgess, M. D., Eaton, M. A., and Gregory, R. D. (2020). A review of spatial patterns across species ranges to aid the targeting of conservation interventions. Biological Conservation 251, 108755.
| Crossref | Google Scholar |
Camino, M., Thompson, J., Andrade, L., Cortez, S., Matteucci, S. D., and Altrichter, M. (2020). Using local ecological knowledge to improve large terrestrial mammal surveys, build local capacity and increase conservation opportunities. Biological Conservation 244, 108450.
| Crossref | Google Scholar |
Cappelle, N., Howe, E. J., Boesch, C., and Kühl, H. S. (2021). Estimating animal abundance and effort–precision relationship with camera trap distance sampling. Ecosphere 12, e03299.
| Crossref | Google Scholar |
Chabot, D., Stapleton, S., and Francis, C. M. (2022). Using Web images to train a deep neural network to detect sparsely distributed wildlife in large volumes of remotely sensed imagery: A case study of polar bears on sea ice. Ecological Informatics 68, 101547.
| Crossref | Google Scholar |
Chrétien, L.-P., Théau, J., and Ménard, P. (2016). Visible and thermal infrared remote sensing for the detection of white-tailed deer using an unmanned aerial system. Wildlife Society Bulletin 40, 181-191.
| Crossref | Google Scholar |
Cooke, B. D. (2012). Rabbits: manageable environmental pests or participants in new Australian ecosystems? Wildlife Research (East Melbourne) 39(4), 279-289.
| Crossref | Google Scholar |
Corcoran, E., Winsen, M., Sudholz, A., and Hamilton, G. (2021). Automated detection of wildlife using drones: Synthesis, opportunities and constraints. Methods in Ecology and Evolution 12(6), 1103-1114.
| Crossref | Google Scholar |
Cosentino, F., and Maiorano, L. (2021). Is geographic sampling bias representative of environmental space? Ecological Informatics 64, 101369.
| Crossref | Google Scholar |
Cox, T. E., Matthews, R., Halverson, G., and Morris, S. (2021). Hot stuff in the bushes: Thermal imagers and the detection of burrows in vegetated sites. Ecology and Evolution 11(11), 6406-6414.
| Crossref | Google Scholar | PubMed |
Dean, A. T., Brandle, R., Barmuta, L. A., Jones, M. E., and Jansen, J. (2023). Rabbit warrens: an important resource for invasive alien species in semi-arid Australia. Wildlife Research 51, WR22154.
| Crossref | Google Scholar |
Delplanque, A., Théau, J., Foucher, S., Serati, G., Durand, S., and Lejeune, P. (2024). Wildlife detection, counting and survey using satellite imagery: are we there yet? GIScience and Remote Sensing 61(1), 2348863.
| Crossref | Google Scholar |
Duckworth, J. W. (1998). The difficulty of estimating population densities of nocturnal forest mammals from transect counts of animals. Journal of Zoology 246(4), 466-468.
| Crossref | Google Scholar |
Duporge, I., Isupova, O., Reece, S., Macdonald, D. W., Wang, T., Pettorelli, N., and Buchanan, G. (2021). Using very‐high‐resolution satellite imagery and deep learning to detect and count African elephants in heterogeneous landscapes. Remote Sensing in Ecology and Conservation 7(3), 369-381.
| Crossref | Google Scholar |
Elith, J., and Leathwick, J. R. (2009). Species Distribution Models: Ecological Explanation and Prediction Across Space and Time. Annual Review of Ecology, Evolution, and Systematics 40(1), 677-697.
| Crossref | Google Scholar |
Estevo, C., Nagy-Reis, M., and Nichols, J. (2017). When habitat matters: Habitat preferences can modulate co-occurrence patterns of similar sympatric species. PLoS One 12(7), e0179489.
| Crossref | Google Scholar | PubMed |
Feldman, M. J., Imbeau, L., Marchand, P., Mazerolle, M. J., Darveau, M., and Fenton, N. J. (2021). Trends and gaps in the use of citizen science derived data as input for species distribution models: A quantitative review. PLoS One 16(3), e0234587.
| Crossref | Google Scholar | PubMed |
Finlayson, G. R., Shimmin, G. A., Temple-Smith, P. D., Handasyde, K. A., and Taggart, D. A. (2005). Burrow use and ranging behaviour of the southern hairy-nosed wombat (Lasiorhinus latifrons) in the Murraylands, South Australia. Journal of Zoology 265(2), 189-200.
| Crossref | Google Scholar |
Finlayson, G., Taggart, P., and Cooke, B. (2022). Recovering Australia’s arid-zone ecosystems: learning from continental-scale rabbit control experiments. Restoration Ecology 30, e13552.
| Crossref | Google Scholar |
Fretwell, P. T., and Trathan, P. N. (2009). Penguins from space: faecal stains reveal the location of emperor penguin colonies. Global Ecology and Biogeography 18(5), 543-552.
| Crossref | Google Scholar |
Fretwell, P. T., Trathan, P. N., Scales, K., and Bouchet, P. (2021). Discovery of new colonies by Sentinel2 reveals good and bad news for emperor penguins. Remote Sensing in Ecology and Conservation 7(2), 139-153.
| Crossref | Google Scholar |
Gedeon, C. I., Árvai, M., Szatmári, G., Brevik, E. C., Takáts, T., Kovács, Z. A., and Mészáros, J. (2022). Identification and counting of European Souslik burrows from UAV images by pixel-based image analysis and random forest classification: a simple, semi-automated, yet accurate method for estimating population size. Remote Sensing 14(9), 2025.
| Crossref | Google Scholar |
Gillieson, D., Wallbrink, P., and Cochrane, A. (1996). Vegetation change, erosion risk and land management on the Nullarbor Plain, Australia. Environmental Geology 28(3), 145-153.
| Crossref | Google Scholar |
Haussmann, N. S. (2017). Soil movement by burrowing mammals: A review comparing excavation size and rate to body mass of excavators. Progress in Physical Geography 41, 29-45.
| Crossref | Google Scholar |
Hollings, T., Burgman, M., van Andel, M., Gilbert, M., Robinson, T., Robinson, A., and McPherson, J. (2018). How do you find the green sheep? A critical review of the use of remotely sensed imagery to detect and count animals. Methods in Ecology and Evolution 9(4), 881-892.
| Crossref | Google Scholar |
Ingram, D. J., Willcox, D., and Challender, D. W. S. (2019). Evaluation of the application of methods used to detect and monitor selected mammalian taxa to pangolin monitoring. Global Ecology and Conservation 18, e00632.
| Crossref | Google Scholar |
Jachmann, H. (2002). Comparison of aerial counts with ground counts for large African herbivores. Journal of Applied Ecology 39(5), 841-852.
| Crossref | Google Scholar |
Jansen, J., Jansen, J., Dean, A. T., Brandle, R., Peacock, D. E., and Jones, M. E. (2023). High-resolution mapping of rabbit (Oryctolagus cuniculus) densities for targeted conservation management. Journal of Applied Ecology 60(12), 2602-2612.
| Crossref | Google Scholar |
Johnston, A., Matechou, E., and Dennis, E. B. (2023). Outstanding challenges and future directions for biodiversity monitoring using citizen science data. Methods in Ecology and Evolution 14, 103-116.
| Crossref | Google Scholar |
Knoblauch, W., Carver, S., Driessen, M. M., Gales, R., and Richards, S. A. (2023). Abundance and population growth estimates for bare‐nosed wombats. Ecology and Evolution 13(9), e10465.
| Crossref | Google Scholar | PubMed |
Koshkina, A., Grigoryeva, I., Tokarsky, V., Urazaliyev, R., Kuemmerle, T., Hölzel, N., and Kamp, J. (2019). Marmots from space: assessing population size and habitat use of a burrowing mammal using publicly available satellite images. Remote Sensing in Ecology and Conservation 6(2), 153-167.
| Crossref | Google Scholar |
Kramer‐Schadt, S., Niedballa, J., Pilgrim, J. D., Schröder, B., Lindenborn, J., Reinfelder, V., Stillfried, M., Heckmann, I., Scharf, A. K., Augeri, D. M., Cheyne, S. M., Hearn, A. J., Ross, J., Macdonald, D. W., Mathai, J., Eaton, J., Marshall, A. J., Semiadi, G., Rustam, R., Bernard, H., Alfred, R., Samejima, H., Duckworth, J. W., Breitenmoser‐Wuersten, C., Belant, J. L., Hofer, H., Wilting, A., Robertson, M., Robertson, M., and Robertson, M. (2013). Importance of correcting for sampling bias in MaxEnt species distribution models. Diversity & Distributions 19(11), 1366-1379.
| Crossref | Google Scholar |
Löffler, E., and Margules, C. (1980). Wombats detected from space. Remote Sensing of Environment 9, 47-56.
| Crossref | Google Scholar |
Łopucki, R., Klich, D., and Kociuba, P. (2022). Detection of spatial avoidance between sousliks and moles by combining field observations, remote sensing and deep learning techniques. Scientific Reports 12, 8264.
| Crossref | Google Scholar | PubMed |
Lynch, H. J., and Schwaller, M. R. (2014). Mapping the abundance and distribution of Adélie penguins using landsat-7: First steps towards an integrated multi-sensor pipeline for tracking populations at the continental scale. PLoS One 9(11), e113301.
| Crossref | Google Scholar | PubMed |
Martin, J. T., and Zickefoos, E. (1976). The effectiveness of aerial survey for determining the distribution of rabbit warrens in a semiarid enviroment. Wildlife Research 3, 79-84.
| Crossref | Google Scholar |
Matthews, A., Spooner, P. G., Lunney, D., Green, K., and Klomp, N. I. (2010). Influences of snow cover, vegetation and topography on the upper range limit of common wombats Vombatus ursinus in the subalpine zone, Australia. Diversity & Distributions 16(2), 277-287.
| Crossref | Google Scholar |
Matthias, L., Allison, M. J., Maslovat, C. Y., Hobbs, J., and Helbing, C. C. (2021). Improving ecological surveys for the detection of cryptic, fossorial snakes using eDNA on and under artificial cover objects. Ecological Indicators 131, 108187.
| Crossref | Google Scholar |
Molyneux, J., Pavey, C. R., James, A. I., and Carthew, S. M. (2017). The efficacy of monitoring techniques for detecting small mammals and reptiles in arid environments. Wildlife Research (East Melbourne) 44(6–7), 534-545.
| Crossref | Google Scholar |
Norris, R., Koertner, G., Meek, P., Cairns, S. C., and Old, J. (2024). Digging for answers: defining the external architecture of the southern hairy-nosed wombats’ (Lasiorhinus latifrons) subterranean excavations. Australian Mammalogy 46(3), AM24027.
| Crossref | Google Scholar |
Nuttall, M. N., Griffin, O., Fewster, R. M., McGowan, P. J. K., Abernethy, K., O’Kelly, H., Nut, M., Sot, V., and Bunnefeld, N. (2022). Long‐term monitoring of wildlife populations for protected area management in Southeast Asia. Conservation Science and Practice 4(2), e614.
| Crossref | Google Scholar |
O’Brien, C., Sparrow, E., Dibben, R., Ostendorf, B., and Taggart, D. (2021). Evaluation of olfactory and visual cues to deter southern hairy-nosed wombats (Lasiorhinus latifrons) from their burrows. Australian Mammalogy 43(1), 110-119.
| Crossref | Google Scholar |
Ostendorf, B. (2011). Overview: Spatial information and indicators for sustainable management of natural resources. Ecological Indicators 11, 97-102.
| Crossref | Google Scholar |
Ostendorf, B., Boardman, W. S. J., and Taggart, D. A. (2016). Islands as refuges for threatened species: multispecies translocation and evidence of species interactions four decades on. Australian Mammalogy 38(2), 204-212.
| Crossref | Google Scholar |
Otis, D. (1998). Analysis of the Influence of Spatial Pattern in Habitat Selection Studies. Journal of Agricultural, Biological, and Environmental Statistics 3(3), 254-267.
| Crossref | Google Scholar |
Pedler, R. D., Brandle, R., Read, J. L., Southgate, R., Bird, P., and Moseby, K. E. (2016). Rabbit biocontrol and landscape-scale recovery of threatened desert mammals. Conservation Biology 30(4), 774-782.
| Crossref | Google Scholar | PubMed |
Phillips, S. J., Dudík, M., Elith, J., Graham, C. H., Lehmann, A., Leathwick, J., and Ferrier, S. (2009). Sample Selection Bias and Presence-Only Distribution Models: Implications for Background and Pseudo-Absence Data. Ecological Applications 19(1), 181-197.
| Crossref | Google Scholar | PubMed |
Pike, D. A., and Mitchell, J. C. (2013). Burrow-dwelling ecosystem engineers provide thermal refugia throughout the landscape. Animal Conservation 16(6), 694-703.
| Crossref | Google Scholar |
Psiroukis, V., Malounas, I., Mylonas, N., Grivakis, K.-E., Fountas, S., and Hadjigeorgiou, I. (2021). Monitoring of free-range rabbits using aerial thermal imaging. Smart Agricultural Technology 1, 100002.
| Crossref | Google Scholar |
Read, J. L., Carter, J., Moseby, K. M., and Greenville, A. (2008). Ecological roles of rabbit, bettong and bilby warrens in arid Australia. Journal of Arid Environments 72(11), 2124-2130.
| Crossref | Google Scholar |
Rivas, A., Chamoso, P., González-Briones, A., and Corchado, J. M. (2018). Detection of cattle using drones and convolutional neural networks. Sensors 18(7), 2048.
| Crossref | Google Scholar | PubMed |
Seidlitz, A., Bryant, K. A., Armstrong, N. J., Calver, M. C., and Wayne, A. F. (2021). Sign surveys can be more efficient and cost effective than driven transects and camera trapping: a comparison of detection methods for a small elusive mammal, the numbat (Myrmecobius fasciatus). Wildlife Research 48(6), 491-500.
| Crossref | Google Scholar |
Shalom, I., Calfayan, L. M., Rospide, M., Thornton, L., Burgos, E. F., and Gómez Villafañe, I. E. (2024). Do exotic invasive mammals disturb the native fauna? Spatiotemporal distribution and overlap between species in a national park of Argentina. Integrative Zoology 0, 1-17.
| Crossref | Google Scholar | PubMed |
Shimmin, G. A., Skinner, J., and Baudinette, R. V. (2002). The warren architecture and environment of the southern hairy-nosed wombat (Lasiorhinus latifrons). Journal of Zoology 258(4), 469-477.
| Crossref | Google Scholar |
Sinclair, S. J., White, M. D., and Newell, G. R. (2010). How Useful Are Species Distribution Models for Managing Biodiversity under Future Climates? Ecology and Society 15(1), 8.
| Crossref | Google Scholar |
Smith, J. E., and Pinter‐Wollman, N. (2021). Observing the unwatchable: Integrating automated sensing, naturalistic observations and animal social network analysis in the age of big data. Journal of Animal Ecology 90, 62-75.
| Crossref | Google Scholar | PubMed |
Sofaer, H. R., Jarnevich, C. S., Pearse, I. S., Smyth, R. L., Auer, S., Cook, G. L., Edwards, T. C., Guala, G. F., Howard, T. G., Morisette, J. T., and Hamilton, H. (2019). Development and Delivery of Species Distribution Models to Inform Decision-Making. BioScience 69(7), 544-557.
| Crossref | Google Scholar |
Southwell, D., Skroblin, A., Moseby, K., Southgate, R., Indigo, N., Backhouse, B., Bellchambers, K., Brandle, R., Brenton, P., Copley, P., Dziminski, M. A., Galindez‐Silva, C., Lynch, C., Newman, P., Pedler, R., Rogers, D., Roshier, D. A., Ryan‐Colton, E., Tuft, K., Ward, M., Zurell, D., and Legge, S. (2023). Designing a large‐scale track‐based monitoring program to detect changes in species distributions in arid Australia. Ecological Applications 33(2), e2762.
| Crossref | Google Scholar |
Stapleton, S., LaRue, M., Lecomte, N., Atkinson, S., Garshelis, D., Porter, C., and Atwood, T. (2014). Polar bears from space: Assessing satellite imagery as a tool to track arctic wildlife. PloS One 9(7), e101513.
| Crossref | Google Scholar | PubMed |
Steinbeiser, C. M., Kioko, J., Maresi, A., Kaitilia, R., and Kiffner, C. (2019). Relative abundance and activity patterns explain method-related differences in mammalian species richness estimates. Journal of Mammalogy 100, 192-201.
| Crossref | Google Scholar |
Sudholz, A., Denman, S., Pople, A., Brennan, M., Amos, M., and Hamilton, G. (2022). A comparison of manual and automated detection of rusa deer (Rusa timorensis) from RPAS-derived thermal imagery. Wildlife Research 49, 46-53.
| Crossref | Google Scholar |
Swinbourne, M. J., Taggart, D. A., Swinbourne, A. M., Lewis, M., and Ostendorf, B. (2018). Using satellite imagery to assess the distribution and abundance of southern hairy-nosed wombats (Lasiorhinus latifrons). Remote Sensing of Environment 211, 196-203.
| Crossref | Google Scholar |
Swinbourne, M., Taggart, D., and Ostendorf, B. (2021). The population status of southern hairy-nosed wombats (Lasiorhinus latifrons). I. Distribution and abundance. Australian Mammalogy 43, 40-53.
| Crossref | Google Scholar |
Taggart, D. A., Finlayson, G. R., Sparrow, E. E., Dibben, R. M., Dibben, J. A., Campbell, E. C., Peacock, D. E., Ostendorf, B., White, C. R., and Temple-Smith, P. D. (2020). Environmental factors influencing hairy-nosed wombat abundance in semi-arid rangelands. Journal of Wildlife Management 84(5), 921-929.
| Crossref | Google Scholar |
Thornett, E., Ostendorf, B., and Taggart, D. A. (2017). Interspecies co-use of southern hairy-nosed wombat (Lasiorhinus latifrons) burrows. Australian Mammalogy 39(2), 205-212.
| Crossref | Google Scholar |
Tuia, D., Kellenberger, B., Beery, S., Costelloe, B. R., Zuffi, S., Risse, B., Mathis, A., Mathis, M.W., van Langevelde, F., Burghardt, T., Kays, R., Klinck, H., Wikelski, M., Couzin, I. D., van Horn, G., Crofoot, M. C., Stewart, C.V., and Berger-Wolf, T. (2022). Perspectives in machine learning for wildlife conservation. Nature Communications 13, 792.
| Crossref | Google Scholar | PubMed |
Vidal‐García, M., and Keogh, J. S. (2017). Invasive cane toads are unique in shape but overlap in ecological niche compared to Australian native frogs. Ecology and Evolution 7(19), 7609-7619.
| Crossref | Google Scholar | PubMed |
Wagner, B., Baker, P., and Nitschke, C. (2021). The influence of spatial patterns in foraging habitat on the abundance and home range size of a vulnerable arboreal marsupial in southeast Australia. Conservation Science and Practice 3(12), e566.
| Crossref | Google Scholar |
Walker, F. M., Taylor, A. C., and Sunnucks, P. (2007). Does soil type drive social organization in southern hairy-nosed wombats. Molecular Ecology 16(1), 199-208.
| Crossref | Google Scholar | PubMed |
Wang, K., Franklin, S. E., Guo, X., and Cattet, M. (2010). Remote sensing of ecology, biodiversity and conservation: a review from the perspective of remote sensing specialists. Sensors 10(11), 9647-9667.
| Crossref | Google Scholar | PubMed |
Whittington-Jones, G. M., Bernard, R. T. F., and Parker, D. M. (2011). Aardvark burrows: a potential resource for animals in arid and semi-arid environments. African Zoology 46(2), 362-370.
| Crossref | Google Scholar |
Wintle, B. A., Cadenhead, N. C. R., Morgain, R. A., Legge, S. M., Bekessy, S. A., Cantele, M., Possingham, H. P., Watson, J. E. M., Maron, M., Keith, D. A., Garnett, S. T., Woinarski, J. C. Z., and Lindenmayer, D. B. (2019). Spending to save: What will it cost to halt Australia’s extinction crisis? Conservation Letters 12(6), e12682.
| Crossref | Google Scholar |
Witmer, G. W. (2005). Wildlife population monitoring: some practical considerations. Wildlife Research 32(3), 259-263.
| Crossref | Google Scholar |
Woinarski, J., Crase, B., Garnett, S., and Rumpff, L. (2021). Addressing issues relating to the conservation of data-poor species, and options for their resolution. NESP Threatened Species Recovery Hub Project 5.2 report, Brisbane. https://www.nespthreatenedspecies.edu.au/media/svgbxrcm/5-2-addressing-issues-relating-to-the-conservation-of-data-poor-species-report_v5.pdf
Zurell, D., Franklin, J., König, C., Bouchet, P. J., Dormann, C. F., Elith, J., Fandos, G., Feng, X., Guillera‐Arroita, G., Guisan, A., Lahoz‐Monfort, J. J., Leitão, P.J., Park, D. S., Peterson, A. T., Rapacciuolo, G., Schmatz, D. R., Schröder, B., Serra‐Diaz, J. M., Thuiller, W., Yates, K. L., Zimmermann, N. E., and Merow, C. (2020). A standard protocol for reporting species distribution models. Ecography 43(9), 1261-1277.
| Crossref | Google Scholar |