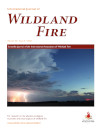
International Journal of Wildland Fire
Volume 32 Number 4 2023
This review paper summarises methodologies used to link field-based measures of severity, based on the Composite Burn Index (CBI), with remotely sensed data from optical sensors. It presents key analytical decisions and research gaps in the existing literature.
WF22050 Abstract | WF22050 Full Text | WF22050PDF (2.5 MB) | WF22050Supplementary Material (638 KB) Open Access Article
Research on the connections between atmospheric turbulence and wildland fires has a long history. In this paper, we summarise the key observational studies over the last 120 years that have contributed to our understanding of wildland fire effects on atmospheric turbulence and its feedback on fire behaviour and smoke dispersion.
WF22053 Abstract | WF22053 Full Text | WF22053PDF (4.2 MB) Open Access Article
A physics-based study of grassfire behaviour over flat and sloped (upslope and downslope) terrains at field scale with different driving wind velocities is presented. The results of RoS of fire, isochrones, firefront locations and intensities are presented and the RoS is compared with empirical studies found in the literature.
A physics-based study of grassfire behaviour over flat and sloped (upslope and downslope) terrain at field scale with varying wind velocities is presented. The flame dynamics, mode of fire propagation and surface radiative and convective heat fluxes are analysed.
WF21129The role of drought conditions on the recent increase in wildfire occurrence in the high Andean regions of Peru





Wildfire occurrence has increased sharply in the last two decades in the Peruvian Andes. Drought analysis using seasonal rainfall indicated fairly normal conditions during 2020, but dry-day frequency (DDF) analysis suggests that a dry period played an important role between September and November 2020, producing severe drought conditions.
WF21146Crown fire initiation of a thunderstorm
Bushfires can support the evolution of thunderstorms. Here, we combine a variety of observations of a bushfire thunderstorm with ensemble fire spread modelling and fire severity mapping. Results show the coupling between the intensity of the fire and its burning through forest canopy with thunderstorm updrafts.
This study evaluates the effect of different sampling strategies for unburned label selection and different ratios of burned and unburned labels in the datasets on the accuracy of machine learning modelling of wildfire occurrence probability.
Ground fraction is the key parameter affecting the ground pattern when combating wildfires. Based on the theoretical analysis and data fitting method, a quantitative ground fraction model is proposed, which reveals the induced effects of drop velocity, drop height, liquid viscosity and other factors on the ground fraction.
WF22055 Abstract | WF22055 Full Text | WF22055PDF (2.2 MB) Open Access Article
Combustion properties of dead herbaceous fuels were studied in no-wind conditions. The phases of fire development are characterised by anomalous diffusion and relaxation processes. The variation of flame height with burning rate exhibited a hysteresis cycle induced by memory effects. These newly discovered physical mechanisms seem to drive fire dynamics.
WF22088 Abstract | WF22088 Full Text | WF22088PDF (3.6 MB) Open Access Article
Wildland–urban interface (WUI) maps identify communities at risk from wildfires. However, WUI maps are often outdated. We evaluated a pre-trained convolutional neural network (CNN) model and CNN-based building dataset and found that they were too inaccurate to estimate building counts and destruction, but sufficient to map where WUI is.
This research explores USFS managers’ utilisation of the Wildland Fire Decision Support System (WFDSS) when evaluating risks and trade-offs related to wildfires. We found that managers use WFDSS to improve information sharing or to document decision rationale. However, users wish to maintain their decision latitude on fire strategy and organisation.
WF22206 Abstract | WF22206 Full Text | WF22206PDF (867 KB) Open Access Article
WF22216An artificial intelligence framework for predicting fire spread sustainability in semiarid shrublands



Models that predict wildfire behaviour in operational settings can support decision making across various fire management areas. Machine learning has the potential to develop predictive models of shrubland fire behaviour. This article tested the suitability of machine learning-based methods, which produced better results than logistic regression models.