Mapping wildfires in Canada with Landsat MSS to extend the National Burned Area Composite (NBAC) time series back to 1972
Rob Skakun A * , Guillermo Castilla A and Piyush Jain AA
Abstract
Satellite imaging has improved burned area mapping; however, few studies have taken advantage of the Multi-Spectral Scanner (MSS) in early Landsat satellites, which started acquiring data 10 years earlier than Thematic Mapper (TM).
To expand Canada’s National Burned Area Composite (NBAC) annual time series back to 1972 using MSS data and report annual statistics and national trends for 1972–2022.
Pre- and post-fire image composites were created using an improved collection of MSS data available from the Google Earth Engine. A Normalized Difference Vegetation Index (NDVI) difference image was adaptively thresholded to extract burned areas, which were then vectorised. To assess accuracy, MSS fire polygons were compared with TM in a year of overlap.
Compared with TM, MSS polygons overestimated burned area by 5.6% when the relativised differenced NDVI was used, with significant upward trends for number of fires > 200 ha, fire season length and mean duration of fires.
MSS is a valuable data source for retrospective mapping of boreal and temperate forest fires where data from finer-resolution sensors are lacking.
After the addition of MSS-mapped fires, NBAC is the longest satellite-based time series of annual burned area from individually mapped fires in the world.
Keywords: burned area, Canada, fire perimeters, Google Earth Engine, Landsat, Landsat Multi-Spectral Scanner, Landsat Thematic Mapper, MSS, NBAC, NDVI, trend analysis, wildfire mapping.
Introduction
Wildfires are a major natural disturbance that alter the composition, structure, succession patterns and relative health of forest ecosystems (McLauchlan et al. 2020). The level of fire activity in a year is strongly influenced by fuels, weather, and natural and human-induced ignitions (Flannigan et al. 2005). In Canada, increased fire activity from warmer and drier conditions linked to climate change are influencing fire regimes (Gillett et al. 2004; Wotton et al. 2010; Kirchmeier-Young et al. 2017). The size, frequency, duration and severity of fires have increased over recent decades, particularly in western regions (Hanes et al. 2019; Whitman et al. 2022; Parisien et al. 2023). The effects of repeated fires or high-intensity burning can have lasting impacts on landscape processes and ecosystem functions such as carbon storage (van Bellen et al. 2010; Walker et al. 2019). Understanding current trends of landscape fire dynamics requires an accurate account of the size and spatial distribution of past fires.
Fire records in Canada are collected by the provincial and territorial fire management agencies and by Parks Canada. The 1950s are often cited as the starting period of spatially explicit fire records (Stocks et al. 2003). Most fires perimeters in these early years were sketched on a map by a surveyor as they flew over the affected area, which led to broad delineations that often overestimated burned area by including unburned patches of forest and water (Kolden et al. 2015; Skakun et al. 2021). In addition, owing to a lack of surveillance in remote, northern regions, many fire events remained undocumented. Fire monitoring and data capture improved globally with the advent of satellite imaging (Chuvieco et al. 2019), especially since the launch of Landsat 4 in 1982, which carried the Thematic Mapper (TM), a multispectral sensor with higher spatial and spectral resolution than the Multi-Spectral Scanner (MSS) sensor in earlier Landsat satellites. The MSS instrument on board Landsat 1–5 provided irregular global coverage from 1972 to 1992, and then again in 2012 when it was brought back to operation following the failure of TM a few months before Landsat 5 was decommissioned after 28 years of service. In the Canadian National Fire Database (CNFDB, originally known as the Canadian Large Fire Database representing fires ≥200 ha) (Stocks et al. 2003), fire perimeters derived from TM imagery first appear in 1984. However, there are no fires mapped in the CNFDB utilising MSS, which was also part of the mission payload in Landsat 4 and 5 to provide data continuity (Mika 1997).
The first peer-reviewed study that used MSS data for burned area mapping considered a 1977 tundra fire in Alaska (Hall et al. 1980), where the authors used a discrete colour ramp on the digital numbers of the MSS near-infrared channel to identify areas burned with various levels of severity. To map a 1000 ha fire in Japan, Tanaka et al. (1983) used image clustering into 10 classes, including three classes of burned areas, in a feature space made of the principal components of the MSS channels. In Canada, only a few studies have investigated MSS data for retrospective fire mapping at regional scales (Boothman and Cardille 2022; Remmel et al. 2023). The scarcity of literature on burned area mapping with MSS is likely due to its limited spatial, temporal and spectral resolutions in comparison with TM (White and Wulder 2014). Coarser pixels in MSS (60 vs 30 m in TM) are more likely to contain both burned and unburned vegetation that complicate the mapping of fire perimeters. MSS data are also known to have lower image georegistration accuracy (i.e. positional accuracy on the ground), and have fewer image acquisitions owing to the experimental nature and lower limit of daily acquisitions of the first Landsat satellites, as well as malfunctions in Landsat 4 and 5 (Goward et al. 2006). Lastly, the MSS sensor lacked shortwave infrared bands to create the Normalized Burn Ratio (NBR), which was originally developed as a burned area mapping index (López García and Caselles 1991). NBR is sensitive to decreased vegetation cover and moisture, and increased presence of ash, char and bare soil (White et al. 1996). Despite the above limitations, MSS allows calculation of the Normalized Difference Vegetation Index (NDVI), which has also been used in burned area mapping (Chen et al. 2016; Hislop et al. 2018). NDVI is a ratio of the red and near-infrared bands, which are available across the MSS collection.
Satellite images for burned area mapping may contain atmospheric artifacts, which can be removed using multi-scene compositing (Whitman et al. 2020; Holsinger et al. 2022; Howe et al. 2022). In this process, a time series of valid observations (i.e. clear-sky image pixels not affected by clouds, haze, or cloud shadows) are extracted across a date range of interest and a summary statistic (e.g. mean, median, or percentile) is calculated for each pixel location and used as a pixel value, resulting in a composited image (Parks et al. 2018). This approach allows flexibility in producing clear-sky imagery that can be tailored to specific dates before and after a fire. For example, specifying a post-fire date range starting after the fire is extinguished will incorporate the immediate effects of fire, such as charring, scorching and mortality (Hudak et al. 2007) and avoid green-up from graminoids or forbs in the following year that can reduce the burn signal.
The goal of this study was to reconstruct a national time series of burned area maps from 1972 to 1983 by applying current image compositing methods and a proven bi-temporal change detection method (Hall et al. 2020) to Landsat MSS data. We present descriptive statistics in annual burned area, including a trend analysis over a 51-year span of fire history by integrating the time series into the National Burned Area Composite database (Skakun et al. 2022). The new fire data provide valuable insights into where and when forests burned, contributing to a deeper understanding of fire regime changes in Canada.
Materials and methods
Study area
The study area includes the forest regions of Canada (Rowe 1972). The boreal is the largest forest region, characterised by continuous and discontinuous tracts of trees interspersed with intact wetlands that stretch from the Yukon Territory in the northwest to the coast of Newfoundland in the northeast (Brandt et al. 2013). Coniferous trees are dominant in the boreal region, often occurring as extensive areas of even-aged stands with a few tree species regenerated from large, stand-replacing wildfires. Other regions include temperate coniferous forests along the west coast of British Columbia, and temperate broadleaf and mixed forests in the east coast and St Lawrence–Great Lakes area.
Fire data
Fire data were obtained from two spatial databases available through the Canadian Wildland Fire Information System (CWFIS; Natural Resources Canada 2024). The first, the CNFDB consists of either fire locations (point data) or fire perimeters (polygon data), both provided by Canadian fire management agencies. We extracted all CNFDB records (point and polygons) from 1972 to 1983 with a burned area size >200 ha to identify candidate fires for retrospective mapping. Approximately 97% of the total burned area in the CNFDB consists of fires >200 ha, a size threshold employed in its precursor the Large Fire Database (Stocks et al. 2003); therefore, it was considered a suitable minimum mapping size for the present study. Some records in the CNFDB lacked a perimeter (polygon) and were only represented by a location (point). For this reason, we spatially intersected the points with a 1 km radius buffer to the polygon layer and set aside all non-intersecting points. Then, all non-intersecting points and fire perimeters were combined so as to retain a single record per fire event, including attribution of a fire start and/or end date if available. Non-intersecting points were converted to circular polygons with an area equal to the reported burned area of the point. This resulted in a harmonised CNFDB dataset consisting of buffered points and irregular polygons, of which those exceeding 200 ha were candidates for MSS mapping. The second spatial database used in this study is the National Burned Area Composite (NBAC; Skakun et al. 2022). NBAC is a time series (1984–present) of annual polygon layers consisting of the best-available fire perimeters from multiple sources, including a Landsat-based mapping procedure developed by Natural Resources Canada (a science-based federal department) to improve estimates of area burned for Canada’s forest carbon accounting program (Hall et al. 2020). NBAC fire data were used to evaluate MSS mapped fires and were later combined with data from this study to create a 51-year time series (1972–2022) to assess trends in annual burned area and other attributes. We note the NBAC layer for the extraordinary 2023 fire season (Jain et al. 2024) was not completed at the time of this study and therefore could not be included.
Landsat MSS data
The Google Earth Engine (GEE) cloud-computing platform contains a comprehensive catalogue of MSS data (Gorelick et al. 2017). Recently, the US Geological Survey released Collection 2, an update of the entire Landsat catalogue including all MSS data, which provides improved radiometric calibration, image registration and enhanced quality assessment bands (Crawford et al. 2023). Collection 2 has two tiers of different quality. Tier 1 includes terrain-corrected products satisfying an image-to-image georeferencing tolerance of ≤12 m horizontal Root Mean Square Error (RMSE); however, less than 1% of the global MSS archive is processed to Tier 1 (Crawford et al. 2023). Most MSS scenes are Tier 2, which does not meet the Tier 1 positional accuracy owing to significant cloud cover, less accurate orbital information and insufficient ground controls (Rengarajan et al. 2020). In the spectral domain, MSS images have green (0.5–0.6 μm), red (0.6–0.7 μm), near-infrared 1 (0.7–0.8 μm) and near-infrared 2 (0.8–1.1 μm) bands. Because there are no blue or shortwave infrared bands, MSS images are not atmospherically corrected in Collection 2 (Crawford et al. 2023). The images are re-sampled to a spatial resolution of 60 m (native resolution is 68 × 83 m), have a radiometric resolution of 6 bits and have a revisit period of 18 days (Landsat 1–3) or 16 days (Landsat 4 and 5). Despite the many advances in Collection 2, MSS images may still contain artifacts that limit the amount of usable data.
Image compositing
We implemented a compositing approach where each candidate fire from the harmonised CNFDB dataset is processed separately in GEE. The processing extent for each fire is the minimum bounding box of the fire plus a 5 km external buffer. In the rare case that the new perimeter from MSS mapping reaches the frame of this area, the processing extent is enlarged another 5 km in each direction and this process is repeated until the new delineation of the fire is fully inside the area. Within the processing extent, each pixel location is examined across a stack of MSS images acquired pre- and post-fire, where each of these two periods has a date range defined as follows. The post-fire image stack is created on a date range starting after the fire is extinguished, determined by the fire end date in the CNFDB attribution, and up to 60 consecutive days. For late-season fires, this date range is constrained to end of September, as snow can be present in northern latitudes as early as October. Whenever the post-fire dates are constrained this way, an image from the following year is inspected to ascertain no additional burning occurred after the cut-off date, otherwise the perimeter is modified accordingly. The pre-fire image stack is created using the same post-fire dates but 1 year earlier. When fire end dates were not available, an 1 August end date was set by default and then manually adjusted if required (by increasing or decreasing the date) based on visual inspection of the resulting post-fire composite for the presence of burned pixels. Tier 1 processed data were used in highest priority, and if none were available, we used Tier 2 data but only for images with a registration RMSE value less than 30 m (half the pixel size). Then, for each image in the stack, we used the image metadata to remove images with cloud coverage greater than 80% and used the quality assessment band to create exclusion masks to remove pixel values contaminated by cloud and cloud shadow. An exclusion mask for waterbodies was also created using the quality assessment band. Finally, image compositing was performed for each pre- and post-fire image stack using the median value of pixels of each spectral band. All candidate fires were processed this way, except for year 1972 when only a post-fire composite was created as there are no prior MSS data.
Burned area mapping
The NDVI was calculated using the near-infrared 2 and red bands of each image composite (Eqn 1). For mapping burned area, we evaluated three metrics of NDVI adapted from the NBR in Whitman et al. (2018), which include (a) the differenced NDVI (dNDVI, Eqn 2), (b) the relativised differenced NDVI (rdNDVI, Eqn 3) and (c) the relativised NDVI (rNDVI, Eqn 4):
The bi-temporal NDVI metrics produce a continuous measurement of change that effectively separates burned from unburned surfaces. Similarly to dNBR metrics, values greater than 0 are typically indicative of burned surfaces (Key and Benson 2006). We also included an offset term (dNDVIoffset) to account for phenology and vegetation moisture differences between pre- and post-fire composites so that the average dNDVI value in unburned forest is 0 (Key 2006). Following the method in Parks et al. (2018), the offset value was calculated as the difference between the pre- and post-fire mean NDVI within a three-pixel-thick frame around the processing extent (i.e. within a 180 m external buffer of the fire bounding box).
Burned area mapping was based on conventional image thresholding. To determine a suitable threshold for each fire, we initially select pixels with values greater than zero in the chosen NDVI metric. The threshold to differentiate burned from unburned pixels is set at 2 s.d. below the mean of the selected pixels, which is equivalent to the 2.3 percentile if the data are normally distributed. Similar methods have been used in Canada (Fraser et al. 2000; Kansas et al. 2016; Hall et al. 2020) and abroad (Kolden and Rogan 2013; Woźniak and Aleksandrowicz 2019; Sukmono et al. 2023). The approach creates an adaptive threshold because the mean and standard deviation statistics are unique for each processing extent from which burned pixels are identified. As such, each threshold accounts for differences in image conditions (e.g. solar elevation, atmospheric effects) and natural conditions (e.g. vegetation type, severity of burn, phenology) associated with each fire. Pixels classified as burned are then converted to vector polygons. Disjoint polygons less than 1 ha in size are removed, as they often are false positives. Finally, the result was quality controlled by a spatial analyst to verify the perimeter delineation against the post-fire composite. If necessary, the automated delineations of fire perimeters were manually adjusted by the analyst to the area they assessed as burned. For example, remnants of pixels contaminated by clouds after applying the exclusion masks hindered the full capture of burned area. Although all fire perimeters were visually checked during quality control, only a small fraction (<5% of fires) required manual editing.
MSS mapping evaluation
To assess which of the three bi-temporal NDVI metrics (Eqns 2–4) led to better results, and to report on the accuracy of MSS mapping, we selected a validation set consisting of fire events in 1986 that were mapped with the Multi-Acquisition Fire Mapping System (MAFiMS, Hall et al. 2020) in NBAC. We chose the year 1986 because it contains the largest number of MSS images in Canada since 1984 (White and Wulder 2014) from which to create fire perimeters for evaluation. MAFiMS was considered a suitable validation reference for three reasons. First, it produces fire perimeters derived from Landsat 5 TM pre- and post-fire imagery simultaneously acquired with that from the MSS instrument on board the same satellite, thereby facilitating pairwise comparison over the same fire season. Second, MAFiMS perimeters also exclude water and unburned forest refugia from the mapped area. Third, the area-based overall accuracy of MAFiMS perimeters was assessed to be 96% when compared with manually delineated polygons from aerial photography (Hall et al. 2020). In 1986, there were 40 fire events in NBAC mapped with MAFiMS, scattered across Canada and within both boreal and temperate biomes. We applied the aforementioned image compositing and mapping methods to pre- and post-fire MSS imagery for these 1986 fire events. For each version (Eqns 2–4) of MSS perimeters, we assessed (1) differences in polygon area, both signed (mean error or ME, i.e. mean under- or over-estimation relative to MAFiMS across the 40 fires) and absolute (mean absolute error or MAE, i.e. mean difference disregarding the sign in each fire); and (2) the proportion of spatial overlap between the MSS perimeters and their MAFiMS counterparts (i.e. area of intersection divided by the area of the MAFiMS polygon). ME and MAE were computed in hectares and as percentages of the area of the corresponding MAFiMS polygon (those percentages were in turn were averaged over the 40 polygons as to obtain %ME and %MAE values).
MSS mapping and time series compilation
All MSS perimeters were created in GEE using image compositing, thresholding and vectorisation. The threshold applied was based on the NDVI metric that yielded the best results in the aforementioned MSS mapping evaluation. An exception is 1972, where a single post-fire NDVI composite was used because there are no prior MSS data. In this case, image thresholding was performed manually for each fire by trial and error, trying to find a threshold value that balanced both commission and omission errors. A national fire dataset was then created by combining the MSS and CNFDB perimeters, in which CNFDB data were used when a particular fire could not be mapped by MSS owing to unusable imagery (e.g. lack of clear-sky pixels, poor cloud masking, sensor artifacts) (Fig. 1). For fires ≤200 ha, the CNFDB data were directly integrated into the time series. The resulting compilation forms a new segment (1972–1983) of the NBAC time series.
Two examples illustrating when (a) a Canadian National Fire database (CNFDB) perimeter could be replaced with a MSS automated delineation, and when (b) the MSS data did not allow automated mapping. The fire perimeter in the yellow outline in (a) is created by MSS mapping in GEE. The orange outline in (a) is the CNFDB perimeter, which was used to locate the fire for retroactive mapping. In (b), MSS imagery could not be used for mapping a different fire because of poor cloud removal and sensor artifacts. The post-fire image composites are displayed using the MSS near-infrared 2, red and green bands as Red, Green and Blue (RGB) channels, respectively. The dark grey pixels are burned area, red is healthy forest, white is unremoved clouds from the exclusion mask, and the faint horizontal stripes are sensor artifacts in the red band.
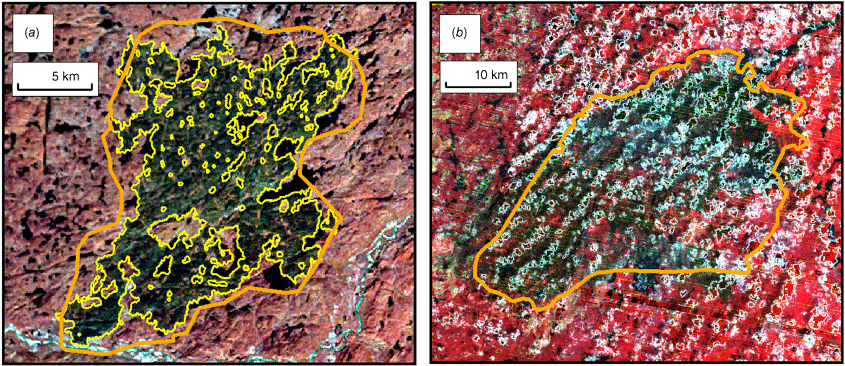
Results are summarised over 1972–1983 by the annual burned area from MSS and CNFDB perimeters and the total proportion mapped by MSS. We also examined the number and area of burns captured by MSS that lack a record in the CNFDB polygon version (i.e. that only appear in the CNFDB point version or do not appear at all), herein referred to as MSS-only perimeters. Last, the MSS perimeters were used as the more accurate reference from which to assess the CNFDB perimeters being replaced.
Trend analysis
The extended 1972–2022 NBAC time series was then used for assessing long-term trends in forest fires in Canada at the national level. Trend analyses were performed for nationally aggregated annual values of: area burned (AAB); number of fires greater than 200 ha (NoF200); number of fires greater than 10,000 ha (NoF10k); mean size of fires greater than 200 ha (meanFS200); mean size of fires greater than 10,000 ha (meanFS10k); length of fire season (FSLength, defined below); mean duration of fires greater than 200 ha (meanLFD200, defined as the mean number of days between start and end date of those fires); and mean duration of fires greater than 10,000 ha (meanLFD10k). Fire season length (FSLength) was computed by disaggregating the area burned uniformly between the start and end dates of each fire in a given year (i.e. assuming the daily burned area for any given fire event was constant for its duration), summing the total daily area burned across all fires in the season and taking the difference between the day corresponding to the 5th and the 95th percentile, as in Hanes et al. (2019). Given the widespread lack of agency end dates, fire season length and mean fire duration could only be computed using hotspots (bright pixels in thermal infrared satellite imagery) and therefore their trend analysis is constrained to 1989–2022 – the first satellite hotspot dataset started in 1989 with the 1.1 km pixel Advanced Very High-Resolution Radiometer (AVHRR, Pu et al. 2007).
For all variables considered, trends were estimated using Sen’s slope and its significance tested with the Mann–Kendall test. Sen’s slope method, which is the median slope of all possible segments connecting pairs of data points (Sen 1968), was computed using the sens.slope function in the R trend package (Pohlert 2023). The Mann–Kendall (MK) test is a non-parametric rank-based test for detecting monotonic trends and was computed using the mk.test function, also available in the trend package. Before performing the MK test, time series were tested for temporal autocorrelation, and when significant correlation was present at 1-year lag (which was the case for meanFS200 and meanFS10k), the modifiedmk package (Patakamuri and O’Brien 2021), a version of the MK test that accounts for autocorrelation, was instead employed, based on the moving block bootstrap (n = 10,000 simulated samples) as proposed by Khaliq et al. (2009). In all cases, statistical significance is indicated for P-values less than either 0.05 or 0.1 using a two-sided test.
Results
MSS mapping evaluation
The 40 MAFiMS fires in NBAC from 1986 used for evaluation ranged from 222 to 92,191 ha in size, with an average burned area of 12,556 ha (median = 3963 ha). The evaluation of the MSS perimeters compared with NBAC is shown in Table 1. For each NDVI metric applied to MSS (Eqns 2–4), the mean area differences (ME) in fire sizes to the NBAC data ranged from 267 ha when using rdNDVI to 667 ha for rNDVI (respectively, 4.8–6.0% in terms relative to the individual MAFiMS polygon size); hence, MSS-mapping tends to slightly overestimate fire size. Larger fire sizes were expected because MSS pixels are twice the size of TM, such that more mixed pixels with unburned vegetation are inevitable. The mean absolute difference (MAE) for all NDVI metrics was less than 1000 ha, which is reasonably low, with the caveat that the NBAC sample had more smaller fire sizes than larger ones. A subsample of the data for fires >50,000 ha (n = 4) produced an absolute error on average of 3000 ha. An example of a larger fire from the subsample is shown in Fig. 2. In NBAC, this fire measured 54,850 ha and was mapped to 57,605 ha using MSS, i.e. an area difference of 2755 ha, or ~5%. In fact, the %MAE for the best metric, rdNDVI, is 7.6%. The proportions of spatial overlap were also high for each metric, demonstrating good agreement in the shapes of mapped perimeters. Although the results of the different NDVI metrics are comparable, rdNDVI had the lowest bias (4.8% overestimation) and highest spatial overlap with NBAC (92%) and was therefore applied to the 1973–1983 time series.
ME (ha) | ME (%) | MAE (ha) | MAE (%) | Overlap (%) | ||
---|---|---|---|---|---|---|
dNDVI | 541 | 5.0 | 944 | 13.5 | 88 | |
rdNDVI | 267 | 4.8 | 639 | 7.6 | 92 | |
rNDVI | 667 | 6.0 | 726 | 9.4 | 90 |
Visual comparison of an MSS perimeter (a) derived from 60 m imagery shows good agreement with a validation NBAC perimeter, (b) created on 30 m TM imagery. Fire perimeters are shown as yellow outlines. The MSS and TM imagery are processed using the same pre- and post-fire acquisition dates, with the MSS perimeter created using rdNDVI and NBAC using dNBR (image backdrops). Areas of light-coloured pixels in both image displays are indicative of burned area. The insets illustrate a less refined delineation of burned area due to the coarse pixel resolution of MSS. The NBAC fire measured 54,850 ha and was mapped to 57,605 ha using MSS, which is an area difference of 2755 ha, or ~5%.
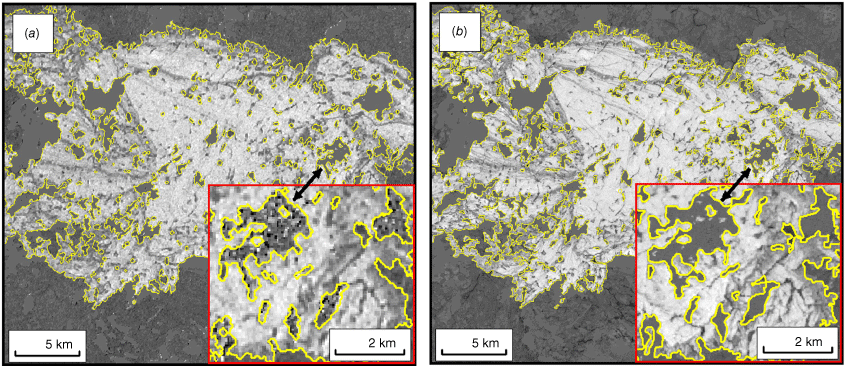
Burned area: 1972–1983
Across the 1972–1983 period, annual maps of burned area from the MSS/CNFDB composite ranged from peak highs of 4.9 and 4.8 Mha in 1980 and 1981, respectively, to 0.2 Mha in 1978 (Fig. 3). The high variability between consecutive years of burned areas is consistent with previous reports in Canada (Hanes et al. 2019; Skakun et al. 2022), as evidenced by an AR1 of 0.47 for the 1972–1983 time series (AR1 is the autocorrelation coefficient for 11 observations of the correlation between the burned area of 1 year and that of the following year). The average annual burned area was 1.9 Mha, compared with 2.1 Mha from the full CNFDB data. Only for the year 1973 was annual burned area greater than the CNFDB because of a large proportion of MSS-only perimeters (Fig. 3). Fires captured by MSS were distributed across the provinces and territories and contributed to 75% of the total burned area (Fig. 4). However, by total count, most fire perimeters in the annual maps were from CNFDB (76%) because most fires were ≤200 ha and no MSS mapping was attempted on them.
Annual burned area from MSS/CNFDB combined perimeters compared with the full CNFDB polygon database (grey). In the MSS/CNFDB composite, MSS-only burned area are mapped fires that lack a CNFDB polygon (dark red), MSS burned area are mapped fires replacing CNFDB (red), and CNFDB burned area is used when a particular fire could not be mapped by MSS (orange).
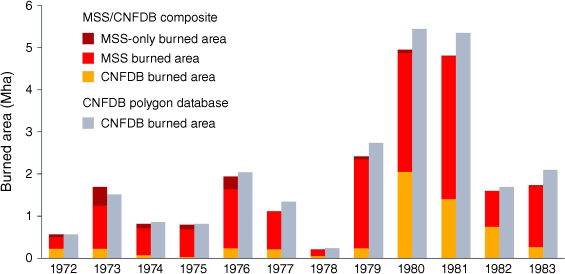
Historical wildfire maps created from Landsat MSS represent 75% of the total burned area from 1972 to 1983, of which 5.6% are MSS-only perimeters (fires that lacked a CNFDB perimeter). Note that Nunavut did not become a territory of Canada until 1999 and is therefore not represented on the map.
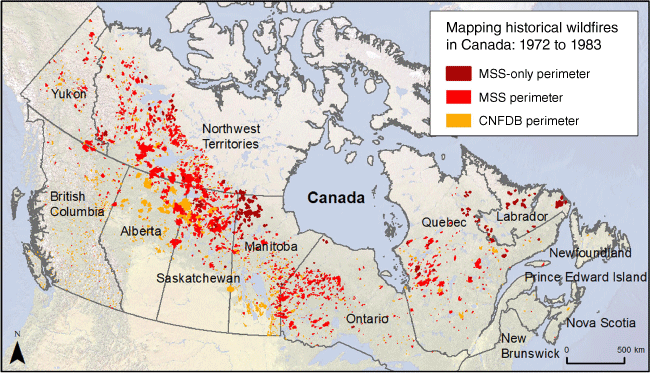
There were 214 MSS-only mapped fires that lacked a CNFDB perimeter. Most of these fires were fortuitously found while mapping a nearby fire, as only 39 of them appeared in the CNFDB point version. The MSS-only perimeters are present in every year of the time series, with 1973 having the largest count (51 fires) and burned area mapped (45,000 ha). The total area of all MSS-only perimeters is 1.26 Mha, which represents 5.6% of the total burned area in the 1972–1983 time series. Across the provinces and territories, a large proportion of MSS-only perimeters were in northwestern Manitoba (Fig. 4), a similar pattern to that found by Skakun et al. (2022) when they mapped missing fires in the polygon version of the CNFDB from 1986 to 2020.
Comparison with CNFDB perimeters
Even if slightly overestimating burned area compared with MAFiMS, MSS-mapped fires did reduce overestimation of the burned area of the replaced CNFDB fire perimeters. Compared with MSS, the CNFDB perimeters reported a 16.6% greater area on average, although in 25.5% of these fire events (19.2% of burned area mapped with MSS), the area in MSS was greater than that in CNFDB. This is due to mapping omissions of the full area of the fire event in the CNFDB. The MAE was 1700 ha (16.6% relative to the mean size of MSS-mapped fires), and the overlap relative to the MSS area was 71.2%, much lower than was found between MAFiMS and MSS.
Trends
The time series and Sen’s slope estimator are shown for the national-level annual statistics in Fig. 5. All variables considered showed a positive trend over their respective time periods. Notably, over the period 1972–2022, annual area burned showed large interannual variability with a small positive (non-significant) trend of ~16,000 ha/year. Slightly increasing but non-significant trends were also found for number of fires >10,000 ha, mean size of those fires and mean size of fires >200 ha. In contrast, trends of the annual number of fires greater than 200 ha, the fire season length and the mean fire duration of fires >200 and >10,000 ha were all statistically significant (P-value < 0.05) according to the MK test, with increasing values per year of 2.69 fires, 1.34 days, 0.27 days and 0.65 days, respectively (Fig. 5).
Time series (points) and Sen’s slope estimator (with trend shown by red line) for annual (a) number of fires >200 ha; (b) number of fires >10,000 ha; (c) mean fire size for fires >200 ha; (d) mean fire size for fires >10,000 ha; (e) annual area burned; (f) fire season length; (g) mean fire duration for fires >200 ha; and (h) mean fire duration for fires >10,000 ha. Trends for (a–e) are shown over the entire NBAC time period (1972–2022) whereas seasonal variables (f–h) are shown over the shorter time period for which hotspot data were available (1989–2022). Statistical significance using the Mann–Kendall test is indicated at the P-value <0.05 level (**) or the <0.1 level (*).
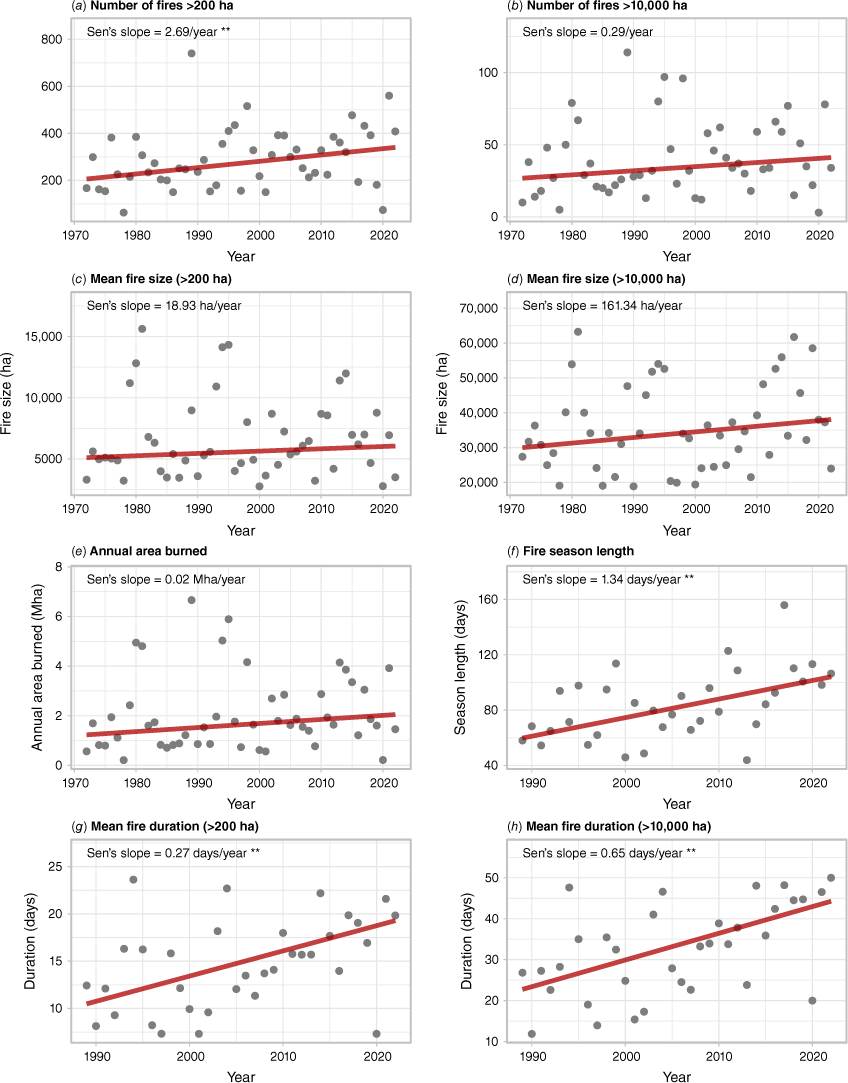
Discussion
This study reconstructed a Canadian annual time series of wildfire burned areas using MSS and CNFDB data from 1972 to 1983. To the best of our knowledge, the integration of MSS mapped fires from this study into the NBAC product makes NBAC the longest satellite-based time series of annual burned area from individually mapped fires in any country in the world (51 years at the time of this study). Other countries with Landsat-derived burned area data include the United States of America (Monitoring Trends in Burn Severity (MTBS); Eidenshink et al. 2007), Brazil (MapBiomas; Alencar et al. 2022) and Chile (The Landscape Fire Scars Database for Chile; Miranda et al. 2022), but these products all start in the 1980s or later. In Canada, other Landsat-based products of burned area exist (Composite to Change (C2C); Hermosilla et al. 2016; Canada Landsat Disturbance (CanLaD); Guindon et al. 2018), but are in raster format (hence no attribution other than the fire year) and start in 1985. An exception is the pre-1985 CanLaD time series, which goes back to 1965 but is based on a modelled disturbance year derived from the pattern of spectral recovery since 1985 of each old, visible disturbance (Correia et al. 2024). At the global level, a Landsat-based time series of burned area starts in 1990 (GABAM, Long et al. 2019).
Although creating the extended NBAC time series presented several challenges, in the end, 75% of the total burned area in the 1972–1983 period corresponds to MSS-mapped fires, a higher proportion than we expected before the study began. Some unique challenges we encountered were finding suitable, clear-sky imagery within the short time frame between fire extinguishment and snow fall (1–3 months) for some candidate fires, and mapping burned areas with inconsistent exclusion masks to remove all pixel values contaminated by cloud, cloud shadow, and water. Low-severity fires, where the tree crowns are singed but the leaves remain on the branches, leading to a weaker rdNDVI signal, required more manual verification of the automated fire perimeters than other fires. For this, we did not have available the additional information that satellite hotspot data provide (as mentioned in the Methods, they only began in 1989) to assist with identifying the full extent of burned areas and relied solely on visual interpretation. Despite these limitations, the MSS perimeters constitute a leap forward enabling accurate spatial analyses that are not possible using the aerial sketches available from CNFDB, such as identifying fire refugia (Downing et al. 2021), assessing short-interval reburns (Whitman et al. 2019) and a more general understanding of changes in fire regimes (Hanes et al. 2019).
This study presents a new method for mapping burned areas using Landsat MSS data. Our approach is similar to MAFiMS in Hall et al. (2020), but we implemented pre- and post-fire image compositing and bi-temporal NDVI metrics in GEE. The use of GEE greatly facilitated the automation of MSS mapping for candidate fires. Unlike methods that utilise region-specific models for MSS burn mapping (Boothman and Cardille 2022), our approach was adaptable across all forest regions and within various vegetation types (conifer, broadleaf and mixed forests), burn conditions (burn-over-burn) and phenology conditions (early and late season fires) without the use of training data. Overall, our study indicates that MSS is a valuable data source for retrospective mapping in boreal and temperate forests where data from finer resolution sensors like TM are lacking.
The extension of the NBAC dataset with MSS mapped fires facilitated a trend analysis of several annual fire metrics from 1972 to 2022 (51 years). The trend in fire season length (an increase of 1.34 days/year, or ~44 days over 33 years) is larger than the trend of 0.251 days/year reported by Hanes et al. (2019) over a similar time period (1980–2015). In that paper, fire season length was determined by the 5th and 95th percentiles of reported fire start, whereas here we used first and last detected hotspots for each fire and aggregated each fire to determine total daily area burned over the country. Apart from this difference in methodology, one possible source of bias in the trend we report is that the current method in NBAC to determine hotspot start and end date uses the earliest and latest hotspots inside each fire perimeter, which could lead to errors if those hotspots actually correspond to a smaller, earlier fire that was quickly extinguished but that was later engulfed by a larger fire, or in the case of the end date, a late ignition in an unburned island. Although future work will search for these possible errors and correct them, we did indirectly ascertain that their potential impact on the trend is likely negligible. To further check our result, we also estimated fire season length by aggregating daily area burned from daily fire maps available from the Canadian Fire Spread Dataset (CFSDS, Barber et al. 2024). We obtained a similar result of 1.1 days/year for the national trend in fire season length, albeit for a shorter time period (2002–2021). The CFSDS is a Canada-wide time series (2002–2021) of annual raster maps with the day of year (DoY) in which each 180 m pixel inside a fire >1000 ha burned. Given the higher trend found in CFSDS, where the burn DoY in a given pixel is interpolated using the dates of the nearest six hotspots, it seems unlikely that the aforementioned possible errors in NBAC are biasing the detected trend.
This study used the CNFDB polygons and buffered points to locate candidate areas for MSS mapping. Given that some incidental, previously unreported fires were found in the vicinity of these areas, it is likely that there are still some fire events from the 1972–1983 period that are unmapped, which can be verified through the pre-1985 CanLaD time series. Likewise, not all fires in the CNFDB could be mapped with MSS using automated methods owing to clouds, haze, artifacts, or lack of suitable dates, leading to some 25% of the burned area in 1972–1983 still mapped using aerial sketches. However, some of these sketches could be refined using MSS imagery guided by manual delineation. These two aspects may be the subject of future work.
Conclusions
In this study, we extended the NBAC database back to 1972 by taking advantage of modern image compositing of an enhanced collection of Landsat MSS data available from GEE that were subject to bi-temporal (pre- and post-fire) change detection. Although MSS data lack the shortwave infrared channel customarily used to derive NBR, they have near-infrared channels required for NDVI, which can also be used for burned area mapping. Indeed, using the MSS-derived rdNDVI led to similar results than when Landsat TM dNBR is used, with just a slight overestimation due to the coarser 60 m pixels in MSS. Even with this and the detection of 1.6 Mha of previously unreported fires, the NBAC time series reduces considerably overestimation of burned area for historic fires previously mapped using aerial sketches, thereby allowing a more precise trend and spatial analyses. The five-decades-long NBAC time series is freely available for download from the Canadian Wildland Fire Information System (https://cwfis.cfs.nrcan.gc.ca).
Data availability
The NBAC and CNFDB databases are openly available on the Canadian Wildland Fire Information System at the following URL/DOI: https://cwfis.cfs.nrcan.gc.ca.
Acknowledgements
Marc-André Parisien encouraged the first author to test Landsat MSS for fire mapping and did an internal review of an earlier version of this manuscript.
References
Alencar AAC, Arruda VLS, Silva WVd, Conciani DE, Costa DP, Crusco N, Duverger SG, Ferreira NC, Franca-Rocha W, Hasenack H, Martenexen LFM, Piontekowski VJ, Ribeiro NV, Rosa ER, Rosa MR, dos Santos SMB, Shimbo JZ, Vélez-Martin E (2022) Long-term Landsat-based monthly burned area dataset for the Brazilian biomes using deep learning. Remote Sensing 14, 2510.
| Crossref | Google Scholar |
Barber QE, Jain P, Whitman E, Thompson DK, Guindon L, Parks SA, Wang X, Hethcoat MG, Parisien MA (2024) The Canadian Fire Spread Dataset. Scientific Data 11(1), 764.
| Crossref | Google Scholar | PubMed |
Boothman R, Cardille JA (2022) New techniques for old fires: using deep learning to augment fire maps from the early satellite era. Frontiers in Environmental Science 10, 914493.
| Crossref | Google Scholar |
Brandt JP, Flannigan MD, Maynard DG, Thompson ID, Volney WJA (2013) An introduction to Canada’s boreal zone: ecosystem processes, health, sustainability, and environmental issues. Environmental Reviews 21(4), 207-226.
| Crossref | Google Scholar |
Chen W, Moriya K, Sakai T, Koyama L, Cao CX (2016) Mapping a burned forest area from Landsat TM data by multiple methods. Geomatics, Natural Hazards and Risk 7(1), 384-402.
| Crossref | Google Scholar |
Chuvieco E, Mouillot F, van der Werf GR, Miguel JS, Tanase M, Koutsias N, García M, Yebra M, Padilla M, Gitas I, Heil A, Hawbaker TJ, Giglio L (2019) Historical background and current developments for mapping burned area from satellite Earth observation. Remote Sensing of Environment 225, 45-64.
| Crossref | Google Scholar |
Correia DLP, Guindon L, Parisien MA (2024) Extending Canadian forest disturbance history maps prior to 1985. Ecosphere 15(8), e4956.
| Crossref | Google Scholar |
Crawford CJ, Roy DP, Arab S, Barnes C, Vermote E, Hulley G, Gerace A, Choate M, Engebretson C, Micijevic E, Schmidt G, Anderson C, Anderson M, Bouchard M, Cook B, Dittmeier R, Howard D, Jenkerson C, Kim M, Kleyians T, Zahn S (2023) The 50-year Landsat Collection 2 archive. Science of Remote Sensing 8, 100103.
| Crossref | Google Scholar |
Downing W, Meigs G, Gregory M, Krawchuk M (2021) Where and why do conifer forests persist in refugia through multiple fire events? Global Change Biology 27(15), 3642-3656.
| Crossref | Google Scholar | PubMed |
Eidenshink J, Schwind B, Brewer K, Zhu ZL, Quayle B, Howard S (2007) A project for monitoring trends in burn severity. Fire Ecology 3, 3-21.
| Crossref | Google Scholar |
Flannigan MD, Logan KA, Amiro BD, Skinner WR, Stocks BJ (2005) Future area burned in Canada. Climatic Change 72, 1-16.
| Crossref | Google Scholar |
Fraser R, Li Z, Cihlar J (2000) Hotspot and NDVI differencing synergy (HANDS): a new technique for burned area mapping over boreal forest. Remote Sensing of Environment 74, 362-376.
| Crossref | Google Scholar |
Gillett NP, Weaver AJ, Zwiers FW, Flannigan MD (2004) Detecting the effect of climate change on Canadian forest fires. Geophysical Research Letters 31, L18211.
| Crossref | Google Scholar |
Gorelick N, Hancher M, Dixon M, Ilyushchenko S, Thau D, Moore R (2017) Google Earth Engine: planetary-scale geospatial analysis for everyone. Remote Sensing of Environment 202, 18-27.
| Crossref | Google Scholar |
Goward S, Arvidson T, Williams D, Faundeen J, Irons J, Franks S (2006) Historical record of Landsat global coverage: mission operations, NSLRSDA, and international cooperator stations. Photogrammetric Engineering and Remote Sensing 72(10), 1155-1169.
| Crossref | Google Scholar |
Guindon L, Bernier PY, Gauthier S, Stinson G, Villemaire P, Beaudoin A (2018) Missing forest cover gains in boreal forests explained. Ecosphere 9(1), e02094.
| Crossref | Google Scholar |
Hall DK, Ormsby JP, Johnson L, Brown J (1980) Landsat digital analysis of the initial recovery of burned tundra at Kokolik River, Alaska. Remote Sensing of Environment 10(4), 263-272.
| Crossref | Google Scholar |
Hall RJ, Skakun RS, Metsaranta JM, Landry R, Fraser RH, Raymond D, Gartrell M, Decker V, Little J (2020) Generating annual estimates of forest fire disturbance in Canada: the National Burned Area Composite. International Journal of Wildland Fire 29, 878-891.
| Crossref | Google Scholar |
Hanes CC, Wang X, Jain P, Parisien MA, Little JM, Flannigan MD (2019) Fire-regime changes in Canada over the last half century. Canadian Journal of Forest Research 49, 256-269.
| Crossref | Google Scholar |
Hermosilla T, Wulder MA, White JC, Coops NC, Hobart GW, Campbell LB (2016) Mass data processing of time series Landsat imagery: pixels to data products for forest monitoring. International Journal of Digital Earth 9(11), 1035-1054.
| Crossref | Google Scholar |
Hislop S, Jones S, Soto-Berelov M, Skidmore A, Haywood A, Nguyen TH (2018) Using Landsat spectral indices in time-series to assess wildfire disturbance and recovery. Remote Sensing 10(3), 460.
| Crossref | Google Scholar |
Holsinger LM, Parks SA, Saperstein LB, Loehman RA, Whitman E, Barnes J, Parisien MA (2022) Improved fire severity mapping in the North American boreal forest using a hybrid composite method. Remote Sensing in Ecology and Conservation 8, 222-235.
| Crossref | Google Scholar |
Howe AA, Parks SA, Harvey BJ, Saberi SJ, Lutz JA, Yocom LL (2022) Comparing Sentinel-2 and Landsat 8 for burn severity mapping in western North America. Remote Sensing 14, 5249.
| Crossref | Google Scholar |
Hudak AT, Morgan P, Bobbitt M, Smith AMS, Lewis SA, Lentile LB, Robichaud PR, Clark JT, McKinley RA (2007) The relationship of multispectral satellite imagery to immediate fire effects. Fire Ecology 3, 64-90.
| Crossref | Google Scholar |
Jain P, Barber QE, Taylor S, Whitman E, Acuna DC, Boulanger Y, Chavardes R, Chen J, Englefield P, Flannigan M, Girardin M, Hanes C, Little J, Morrison K, Skakun RS, Thompson D, Wang X, Parisien MA (2024) Drivers and impacts of the record-breaking 2023 wildfire season in Canada. Nature Communications 15, 6764.
| Crossref | Google Scholar | PubMed |
Kansas J, Vargas J, Skatter HG, Balicki B, McCullum K (2016) Using Landsat imagery to backcast fire and post-fire residuals in the Boreal Shield of Saskatchewan: implications for woodland caribou management. International Journal of Wildland Fire 25, 597-607.
| Crossref | Google Scholar |
Key CH (2006) Ecological and sampling constraints on defining landscape fire severity. Fire Ecology 2, 34-59.
| Crossref | Google Scholar |
Key CH, Benson NC (2006) Landscape Assessment: Ground measure of severity, the Composite Burn Index, and remote sensing of severity, the Normalized Burn Ratio. In ‘FIREMON: Fire Effects Monitoring and Inventory System’. General Technical Report RMRS-GTR-164-CD: LA1-51. (Eds DC Lutes, RE Keane, JF Caratti, CH Key, NC Benson, S Sutherland, LJ Gangi) (USDA Forest Service, Rocky Mountain Research Station: Ogden, UT)
Khaliq MN, Ouarda TBMJ, Gachon P, Sushama L, St-Hilaire A (2009) Identification of hydrological trends in the presence of serial and cross correlations: a review of selected methods and their application to annual flow regimes of Canadian rivers. Journal of Hydrology 368, 117-130.
| Crossref | Google Scholar |
Kirchmeier-Young MC, Zwiers FW, Gillett NP, Cannon AJ (2017) Attributing extreme fire risk in western Canada to human emissions. Climate Change 144, 365-79.
| Crossref | Google Scholar | PubMed |
Kolden CA, Rogan J (2013) Mapping wildfire burn severity in the arctic tundra from downsampled MODIS data. Arctic, Antarctic, and Alpine Research 45(1), 64-76.
| Crossref | Google Scholar |
Kolden CA, Smith AM, Abatzoglou JT (2015) Limitations and utilisation of Monitoring Trends in Burn Severity products for assessing wildfire severity in the USA. International Journal of Wildland Fire 24(7), 1023-1028.
| Crossref | Google Scholar |
Long T, Zhang Z, He G, Jiao W, Tang C, Wu B, Zhang X, Wang G, Yin R (2019) 30m resolution global annual burned area mapping based on Landsat images and Google Earth Engine. Remote Sensing 11, 489.
| Crossref | Google Scholar |
López García JM, Caselles V (1991) Mapping burns and natural reforestation using Thematic Mapper data. Geocarto International 6, 31-37.
| Crossref | Google Scholar |
McLauchlan KK, Higuera PE, Miesel J, Rogers BM, Schweitzer J, Shuman JK, Tepley AJ, Morgan Varner J, Veblen TT, Adalsteinsson SA, Balch JK, Baker P, Batllori E, Bigio E, Brando P, Cattau M, Chipman ML, Coen J, Crandall R, Daniels L, Enright N, Gross WS, Harvey BJ, Hatten JA, Hermann S, Hewitt RE, Kobziar LN, Landesmann JB, Loranty MM, Maezumi SY, Mearns L, Moritz M, Myers JA, Pausas JG, Pellegrini AFA, Platt WJ, Roozeboom J, Safford H, Santos F, Scheller RM, Sherriff RL, Smith KG, Smith MD, Watts AC (2020) Fire as a fundamental ecological process: research advances and frontiers. Journal of Ecology 108, 2047-2069.
| Crossref | Google Scholar |
Mika AM (1997) Three decades of Landsat instruments. Photogrammetric Engineering and Remote Sensing 63(7), 839-852 https://www.asprs.org/wp-content/uploads/pers/1997journal/jul/1997_jul_839-852.pdf.
| Google Scholar |
Miranda A, Mentler R, Moletto-Lobos Í, Alfaro G, Aliaga L, Balbontín D, Barraza M, Baumbach S, Calderón P, Cárdenas F, Castillo I, Contreras G, de la Barra F, Galleguillos M, González ME, Hormazábal C, Lara A, Mancilla I, Muñoz F, Oyarce C, Pantoja F, Ramírez R, Urrutia V (2022) The Landscape Fire Scars Database: mapping historical burned area and fire severity in Chile. Earth System Science Data 14, 3599-3613.
| Crossref | Google Scholar |
Natural Resources Canada (2024) ‘CWFIS Datamart.’ (Natural Resources Canada, Canadian Forest Service: Ottawa, ON) Available at https://cwfis.cfs.nrcan.gc.ca [verified 7 June 2024]
Parisien MA, Barber QE, Bourbonnais ML, Daniels LD, Flannigan MD, Gray RW, Hoffman KM, Jain P, Stephens SL, Taylor SW, Whitman E (2023) Abrupt, climate-induced increase in wildfires in British Columbia since the mid-2000s. Communications Earth and Environment 4, 309.
| Crossref | Google Scholar |
Parks SA, Holsinger LM, Voss MA, Loehman RA, Robinson NP (2018) Mean composite fire severity metrics computed with Google Earth Engine offer improved accuracy and expanded mapping potential. Remote Sensing 10, 879.
| Crossref | Google Scholar |
Patakamuri S, O’Brien N (2021) modifiedmk: modified versions of Mann Kendall and Spearman’s rho trend tests. R package version 1.6. Available at https://CRAN.R-project.org/package=modifiedmk
Pohlert T (2023) trend: non-parametric trend tests and change-point detection. R package version 1.1.6. Available at https://CRAN.R-project.org/package=trend
Pu R, Li Z, Gong P, Csiszar I, Fraser R, Hao W, Kondragunta S, Weng F (2007) Development and analysis of a 12-year daily 1-km forest fire dataset across North America from NOAA/AVHRR data. Remote Sensing of Environment 108(2), 198-208.
| Crossref | Google Scholar |
Remmel TK, Ouellette M, Wu WJ (2023) A boreal wildfire and harvesting database with ensemble confidence attributes for Ontario (1972-2021+). International Journal of Applied Earth Observation and Geoinformation 117, 103199.
| Crossref | Google Scholar |
Rengarajan R, Choate M, Storey J, Franks S, Micijevic E (2020) Landsat Collection-2 geometric calibration updates. In ‘Proceedings Society of Photo-Optical Instrumentation Engineers 11501, Earth Observing Systems XXV’. p. 115010N. 10.1117/12.2570429
Sen PK (1968) Estimates of the regression coefficient based on Kendall’s tau. Journal of the American Statistical Association 63, 1379-1389.
| Crossref | Google Scholar |
Skakun R, Whitman E, Little JM, Parisien MA (2021) Area burned adjustments to historical wildland fires in Canada. Environmental Research Letters 16, 064014.
| Crossref | Google Scholar |
Skakun R, Castilla G, Metsaranta J, Whitman E, Rodrigue S, Little J, Groenewegen K, Coyle M (2022) Extending the National Burned Area Composite time series of wildfires in Canada. Remote Sensing 14(13), 3050.
| Crossref | Google Scholar |
Stocks BJ, Mason JA, Todd JB, Bosch EM, Wotton BM, Amiro BD, Flannigan MD, Hirsch KG, Logan KA, Martell DL, Skinner WR (2003) Large forest fires in Canada, 1959–1997. Journal of Geophysical Research 108, 8149.
| Crossref | Google Scholar |
Sukmono A, Hadi F, Widayanti E, Nugraha AL, Bashit N (2023) Identifying burnt areas in forests and land fire using multitemporal Normalized Burn Ratio (NBR) index on Sentinel-2 Satellite imagery. International Journal of Safety and Security Engineering 13(3), 469-477.
| Crossref | Google Scholar |
Tanaka S, Kimura H, Suga Y (1983) Preparation of a 1:25000 Landsat map for assessment of burnt area on Etajima Island. International Journal of Remote Sensing 4(1), 17-31.
| Crossref | Google Scholar |
van Bellen S, Garneau M, Bergeron Y (2010) Impact of climate change on forest fire severity and consequences for carbon stocks in boreal forest stands of Quebec, Canada: a synthesis. Fire Ecology 6, 16-44.
| Crossref | Google Scholar |
Walker XJ, Baltzer JL, Cumming SG, Day NJ, Ebert C, Goetz S, Johnstone JF, Potter S, Rogers BM, Schuur EAG, Turetsky MR, Mack MC (2019) Increasing wildfires threaten historic carbon sink of boreal forest soils. Nature 572, 520-523.
| Crossref | Google Scholar | PubMed |
White JC, Wulder MA (2014) The Landsat observation record of Canada: 1972–2012. Canadian Journal of Remote Sensing 39(6), 455-467.
| Crossref | Google Scholar |
White JD, Ryan KC, Key CH, Running SW (1996) Remote sensing of forest fire severity and vegetation recovery. International Journal of Wildland Fire 6(3), 125-136.
| Crossref | Google Scholar |
Whitman E, Parisien MA, Thompson DK, Hall RJ, Skakun RS, Flannigan MD (2018) Variability and drivers of burn severity in the northwestern Canadian boreal forest. Ecosphere 9, e02128.
| Crossref | Google Scholar |
Whitman E, Parisien MA, Thompson DK, Flannigan M (2019) Short-interval wildfire and drought overwhelm boreal forest resilience. Scientific Reports 9(1), 18796.
| Crossref | Google Scholar | PubMed |
Whitman E, Parisien MA, Holsinger LM, Park J, Parks SA (2020) A method for creating a burn severity atlas: an example from Alberta, Canada. International Journal of Wildland Fire 29, 995-1008.
| Crossref | Google Scholar |
Whitman E, Parks SA, Holsinger LM, Parisien MA (2022) Climate-induced fire regime amplification in Alberta, Canada. Environmental Research Letters 17, 055003.
| Crossref | Google Scholar |
Wotton BM, Nock CA, Flannigan MD (2010) Forest fire occurrence and climate change in Canada. International Journal of Wildland Fire 19(3), 253-271.
| Crossref | Google Scholar |
Woźniak E, Aleksandrowicz S (2019) Self-adjusting thresholding for burnt area detection based on optical images. Remote Sensing 11(22), 2669.
| Crossref | Google Scholar |