Enhancing fire emissions inventories for acute health effects studies: integrating high spatial and temporal resolution data
Sam D. Faulstich
A
B
Abstract
Daily fire progression information is crucial for public health studies that examine the relationship between population-level smoke exposures and subsequent health events. Issues with remote sensing used in fire emissions inventories (FEI) lead to the possibility of missed exposures that impact the results of acute health effects studies.
This paper provides a method for improving an FEI dataset with readily available information to create a more robust dataset with daily fire progression.
High temporal and spatial resolution burned area information from two FEI products are combined into a single dataset, and a linear regression model fills gaps in daily fire progression.
The combined dataset provides up to 71% more PM2.5 emissions, 69% more burned area, and 367% more fire days per year than using a single source of burned area information.
The FEI combination method results in improved FEI information with no gaps in daily fire emissions estimates.
The combined dataset provides a functional improvement to FEI data that can be achieved with currently available data.
Keywords: acute health effects studies, burned area, cloud cover, environmental epidemiology, exposure modelling, fire remote sensing, PM2.5, wildfire smoke exposure, wildfire smoke transport.
Introduction
Inhaling wildfire smoke can cause various health effects in humans (Yao et al. 2016; Borchers Arriagada et al. 2019; Liu et al. 2019). Wildfire smoke can be transported through the atmosphere, impacting communities far from the actual fire. As wildfire frequency, intensity, and duration are projected to increase under a changing climate (Liu et al. 2016; Aguilera et al. 2021), understanding the health effects of inhaling wildfire smoke is essential. Some of the most important health effects to understand are acute health effects, which occur shortly after exposure and are typically short-lived (Ye et al. 2022). To estimate the acute health effects of inhaling wildfire smoke, a public health study requires estimates of the concentration of pollutants from wildfire smoke on a daily time scale.
Information on fire behaviour or activity is needed to estimate the concentration of pollutants from wildfire smoke in a specific area. An FEI is a combination of fire behaviour information (i.e. data that accounts for burned area, the amount of vegetation burned (fuel loading)), and emissions factor (i.e. data that accounts for the compound of interest and fuel characteristics) to estimate the amount of emissions released by each individual fire (Urbanski et al. 2018). Single-fire information is useful for health effects studies because it allows for the linking of health effects to combustion type and fuel type. It can also be helpful to incorporate plume ageing and smoke transport factors when more than one smoke plume impacts a specific area. There is no definitive method to measure the amount of emissions released from a single fire (Hao and Larkin 2014; Black et al. 2017). FEIs offer a reasonable estimate of fire emissions for use in health effects studies.
While prior studies recognise the importance of understanding the health effects of inhaling wildfire smoke (Gao et al. 2023; Pan et al. 2023; Reid et al. 2023), existing literature reveals notable gaps in methods for estimating acute human exposure (Black et al. 2017). Challenges in estimating acute smoke exposure are related to limitations with FEIs, atmospheric models, and remote sensing technologies. While there have been recent advancements in geostationary satellite remote sensing capabilities, historically, satellite remote sensing fire and smoke products struggle to capture the high temporal resolution required for acute exposure estimates (Wooster et al. 2021). Satellite remote sensing also faces issues with cloud cover (Hawbaker et al. 2017), has difficulty sensing small fires (Laris 2005; Roteta et al. 2019), and lacks a conclusive method of direct measurement of fire emissions that would allow for validation (Giglio and Roy 2020). These challenges underscore the need for development of more robust methodologies for estimating acute exposure to wildfire smoke.
Currently, FEIs rely heavily on satellite remote sensing to determine the input variables for the emissions model, especially for near real-time fire detection. Fire ignition, fire progression, burned area, and fuel loading are often determined using remote sensing, but can also be supplemented with fire spread models or burn reports (i.e. bottom-up approaches). Remote sensing provides many advantages, including global coverage and the ability to provide information in near real-time (Wooster et al. 2021). However, many FEIs currently do not focus on providing real-time data; instead, they provide a consistent long-term dataset for understanding historical fire trends. The current state of the science for high temporal resolution remote sensing of fires uses geostationary satellites (Li et al. 2022), which constantly monitor the same region, providing a constant record of the fire. The state of the science for high spatial resolution remote sensing of burned area relies on changes in land reflectance over time (Eidenshink et al. 2007). Products with the shortest latency for remotely sensing fires can be accessed within hours (Soja et al. 2009).
Other issues with FEIs include emissions factors that are difficult to measure, and those emissions factors that can be measured may lack generalisability due to the complex nature of fire behaviour (Larkin et al. 2014). Because of the complexities of fire behaviour, there is no way to measure fire emissions directly to create a validation dataset (Black et al. 2017). Additionally, fuel load can be incredibly diverse in properties and vary highly in time and space, creating uncertainties in estimating fuel loading (Keane 2013). The complex nature of fire behaviour means many FEI datasets are created using several different methods for estimating the components of fire emissions (i.e. emissions factors and fuel loading), and the lack of validation data means we cannot determine which method is most accurate (Faulstich et al. 2022).
Although the fire science community understands the shortcomings of FEIs, many suggestions for improvement concentrate on enhancing input datasets or seeking validation through costly, in-depth field campaigns (Roy et al. 2007; Soja et al. 2009). While these in-depth studies improve our understanding of fire emissions, using current available information to explore alternative possibilities for enhancing existing datasets is crucial to make improvements in current studies that rely on FEIs. This study aims to improve existing FEIs by combining high spatial and temporal resolution data from two existing FEI products.
The focus of this paper is to present a method to create a combined FEI and discuss the advantages of the method, with a focus on using the combined FEI for public health research. Combining two sources of burned area information into one FEI allows models to use both high spatial and high temporal resolution satellite products. High spatial resolution data is essential to capture the most reliable burned area estimates, often used to approximate the fire size and intensity. Capturing the daily emissions profile is vital to understanding the acute health effects of inhaling wildfire smoke, making high temporal resolution data crucial. Combining these two sources of fire information can mitigate some of the disadvantages associated with each individual method for determining burned area. Adding a cloud cover interpolation eliminates gaps in daily fire emissions information. These two improvements allow the utilisation of the combined dataset as input data into a smoke exposure model for an acute health effects study.
Methods
Each FEI uses a unique method to estimate overall fire emissions, meaning combining data from one FEI with data from another FEI is very difficult. However, one FEI, the Wildland Fire Emissions Information System (WFEIS), has separate emissions estimates products that use the high-resolution spatial product from Landsat Monitoring Trends in Burn Severity (MTBS) and the high-resolution temporal burned area product from the Moderate Resolution Imaging Spectrometer (MODIS) individually (French et al. 2014). WFEIS uses estimates of fuel loading, burned area, and fire characteristics to estimate total emissions from a fire. Fuel loading is multiplied by burned area to determine the amount of fuel burned, and this is scaled by an emissions factor to determine how much of a specific compound was released by that burned fuel. Further discussion of how WFEIS uses this information to estimate fire emissions can be found in French et al. (2014). Since the two emissions estimates are both from WFEIS, they have a consistent method to determine overall fire emissions and thus they can be combined into a single product with the advantages of both burned area products. Combining these two WFEIS emissions estimates products retains the high-quality spatial resolution information from MTBS while leveraging MODIS to include a daily temporal profile.
High spatial resolution (30 m) fire information comes from the LandSat Monitoring Trends in Burn Severity (MTBS) product (Eidenshink et al. 2007). MTBS provides burned area information using the LandSat remote sensing of land cover reflectance changes resulting from fires. The high spatial resolution LandSat data used in the MTBS product provides the most reliable burned area estimate for an individual fire. Because burned area plays a significant role in the emissions calculations, this value can have a large impact on the FEI emissions estimates. While the spatial resolution from the MTBS product is not retained in our FEI combination, the high spatial resolution burned area provides better emissions estimates than other inventories (Faulstich et al. 2022). This is, in part, because the MTBS product is less affected by cloud cover and provides better detection for small fires. The LandSat satellite overpass frequency is every 8 days, meaning it only collects data from a specific location once every 8 days. Because of this frequency, MTBS does not include high temporal resolution data that can provide information on daily fire progression.
The Moderate Resolution Imaging Spectrometer (MODIS) active fire detection product (Giglio et al. 2016) provides high temporal resolution (i.e. daily) fire information. The MODIS active fire detection product comes from MODIS retrievals on two polar orbiting satellites that provide fire information for a specific location every 24 hours (Giglio et al. 2016). The two satellites overpass each location at different times, providing two measurements of the location per day, meaning that the MODIS active fire product can give information on daily fire progression. However, the spatial resolution is 500 m × 500 m, meaning small fires and prescribed burns may not be detected.
We combined the two WFEIS burned area products to create a single FEI that uses high spatial and temporal resolution data. WFEIS was selected due to the complex fuel characteristics, the inclusion of both flaming and smouldering combustion, and the two different burned area products available (Faulstich et al. 2022). This dataset was created for a domain within the western United States (Fig. 1) from 2007 to 2019 to support retrospective health studies of smoke exposure in Nevada. In this paper, results from 3 years with several large wildfires (2013, 2016, 2018) are presented. Because of the heavy reliance on remote sensing data, even after combing both burned area products, there are still missing days due to cloud cover. To address this, we fit a linear regression model to gap-fill the missing days resulting from cloud cover issues in the remote sensing products. The combination and interpolation methods are outlined below and shown in Fig. 2, and a step-by-step list of instructions can be found in Supplementary Material S1.
Flowchart of the FEI combination method using WFEIS MTBS and MODIS. WFEIS MTBS data are input in the first step, and the WFEIS MODIS data are input in the third step.
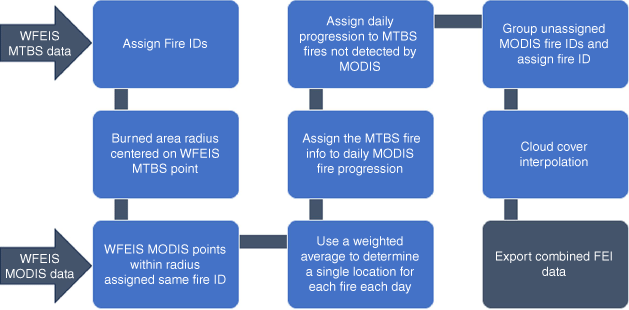
Assigning daily fire progression to MTBS data
To assign daily fire progression information to the high spatial resolution MTBS data, we assign unique fire IDs to each fire in the MTBS dataset. Pairing the MTBS fire information with daily MODIS fire progression data involves utilising the location centroid and burned area information provided in the MTBS dataset. We use the fire burned area to calculate a search radius around the fire centroid used only for pairing the fires from MTBS and MODIS. Since fires are not a perfect circle, the calculated radius is multiplied by 1.5 to ensure that this radius captures all MODIS fire points. When checked using a geographical map, we found this method did not produce overlapping radii or assign multiple fire IDs to a single grid cell. After assigning a fire ID to these MODIS fire points, we further refine the data corresponding to each fire to ensure there is only one point per fire, per day. We use a single point per fire that includes daily burned area (i.e. a new fire location each day based on MODIS data) to input fire information into the atmospheric dispersion model. We aggregate data points with the same fire ID to accomplish this, determining a single daily location using a weighted spatial average based on the daily MODIS emissions. Utilising the amount of PM2.5 emissions, the weighted average emphasises points with substantial PM2.5 emissions on a given day when determining fire location.
After assigning the daily fire progression, the MTBS burned area and PM2.5 are allocated to the corresponding daily MODIS points. The fire emissions or burned area total from MODIS is calculated based on the fire ID and compared with the totals per fire from MTBS. A daily percentage of the total per-fire emissions or burned area is assigned to each MODIS day. This MODIS daily percentage is then multiplied by the total fire emissions or burned area from MTBS to allocate the MTBS burned area and emissions information daily. Now, the daily fire information from MODIS represents the complete fire information reported by MTBS. After completing this process, MTBS burned area and emissions reflects the daily fire progression from MODIS.
Resolving discrepancies
Through this daily fire progression method, discrepancies between the datasets emerge, including cases where MODIS fails to identify fires that MTBS sensed, leading to an absence of daily fire progression. With its higher spatial resolution, MTBS detects some small fires that MODIS does not. In such instances, the fire duration needs to be determined to allocate daily emissions. To address the lack of fire duration provided by MTBS, we established a linear correlation between burned area and fire length for the entire spatial domain using data from other fires in the same year. Because the burned area for all unassigned MTBS fires was smaller than 25 km2, any fires larger than this were excluded from the linear correlation. The linear regression between the MODIS burned area and the fire length is used to infer the fire length for the unassigned MTBS fires based on the burned area. Additionally, some MODIS fires do not correspond to MTBS fires due to the differences in methods between the two burned area datasets. For example, MTBS may miss fires that have low burn severity (Hawbaker et al. 2017). Since these fires already have daily progression, they are grouped by location and date and assigned a fire ID. The burned area and emissions estimates from MODIS are used to provide fire information for these fires that are not captured by MTBS.
Cloud cover
After determining the daily fire progression, we can identify any missing days. Nearly all fires are missing at least 1 day, with many missing seven or more consecutive days. Given that some of these gaps span weeks or months, we split any fires with more than seven consecutive days missing between sensed points into two fires. Because we primarily use the fire IDs to determine fuel type, inadvertently breaking one fire into two poses no downsides. Once the long fires are separated, a linear interpolation can be applied to provide information on missing fire days. The linear interpolation looks for a sequential gap in the fire progression and then uses the two nearest sensed points to determine the slope of the line for both PM2.5 and burned area. The slope of this line determines what the value of PM2.5 or burned area should be for the missing day. The location of each interpolated point is the average of the location of the two nearest sensed points.
After completing these steps, the combined FEI dataset now incorporates data from both high spatial (MTBS) and high temporal (MODIS) resolution data sources, including gap-filled data to provide emissions estimates when remote sensing does not detect a fire. This combination provides daily information on fire characteristics that can be input into an atmospheric transport model and then used to estimate daily smoke exposures.
Results
Combining two sources of burned area information in a single FEI provides more information on fire emissions. To understand the additional information gained through the combination, we present comparisons of PM2.5, burned area, and number of fire days as estimated by each WFEIS product, the combined FEI that uses both burned area products, and the combined FEI with and without cloud cover interpolation.
Assigning daily fire progression to MTBS data
Using MODIS data, daily fire progression is assigned to MTBS fire perimeters. An example of this process is shown in Fig. 3 for the Happy Camp fire in 2014 (Kalamath National Forest, California, USA; 12 August–30 October 2014, 543 km2 in burned area (National Interagency Coordination Center 2014)). WFEIS MTBS provides only a single centroid and burned area for the fire, whereas WFEIS MODIS provides a daily fire progression. This figure shows the advantage of the WFEIS MODIS daily fire progression, though the WFEIS MODIS product has a lower spatial (1 km) resolution than WFEIS MTBS (30 m). The combination inventory has an average increase of 7% in the number of fire days per year over WFEIS MODIS and an average increase of 269% in the number of fire days over WFEIS MTBS over the 3 years investigated in depth (2013, 2016, 2018). Because MTBS only provides one date for each fire, the increase in number of fire days represents the daily fire progression assigned to each MTBS fire. The increase in the number of fire days for the combination inventory compared to WFEIS MODIS is because MTBS senses small fires that are not captured by the MODIS dataset. LandSat MTBS provides highly detailed burned area data at low temporal resolutions. The fine spatial resolution allows MTBS to achieve the most reliable burned area assessments, particularly for small fires. Consequently, inventories exclusively relying on MODIS data may overlook these smaller fires.
Fire detections for a single, large fire (the Happy Camp fire in 2014) from WFEIS MTBS and the combination FEI, which gets daily fire detections from WFEIS MODIS. The combo FEI is shown as circles, colour-coded by date. The darker colours were sensed in earlier weeks of the fire, and the lighter colours were sensed in later weeks of the fire. Week 1 represents the first week the fire was sensed and week 7 represents the last week the fire was sensed. WFEIS MTBS is represented as a single triangle. WFEIS MTBS only provides a single centroid and burned area for the fire, whereas WFEIS MODIS provides a daily fire progression for the combination FEI.
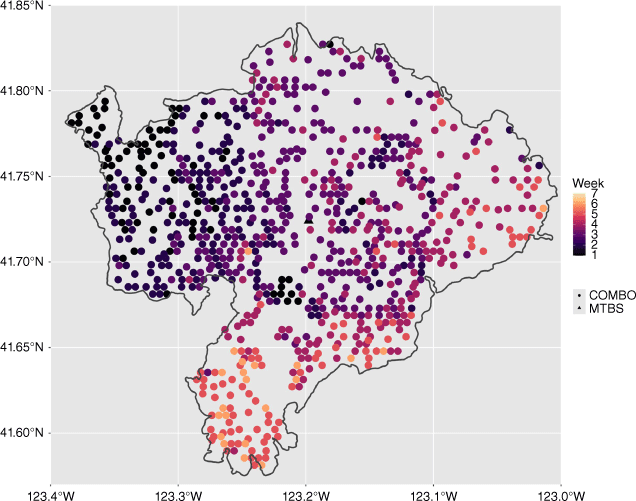
Assigning a daily fire progression to MTBS data to create a combined FEI provides more information on emissions and burned area. Fig. 4 provides a visual representation of the new information included in the combined FEI. The low fire intensity days at the beginning and end of the fire needed the most interpolation, likely because the smaller fire intensity days are more susceptible to cloud cover and other remote sensing issues. Table 1 provides the emissions, fire days, and burned area information comparison for each FEI, including the percent increase. The combined FEI provides larger increases for WFEIS MODIS than WFEIS MTBS for both PM2.5 and burned area due to the high spatial resolution of MTBS providing more fire information (e.g. on small fires) than the lower spatial resolution of MODIS. This combination enables the incorporation of daily fire progression captured by MODIS and the detection of small fires captured by MTBS into a single FEI.
Annual sum of PM2.5 emissions (kg), burned area (km2), and active fire days (days) for WFEIS MTBS (green), WFEIS MODIS (blue), and the combined FEI product (purple).
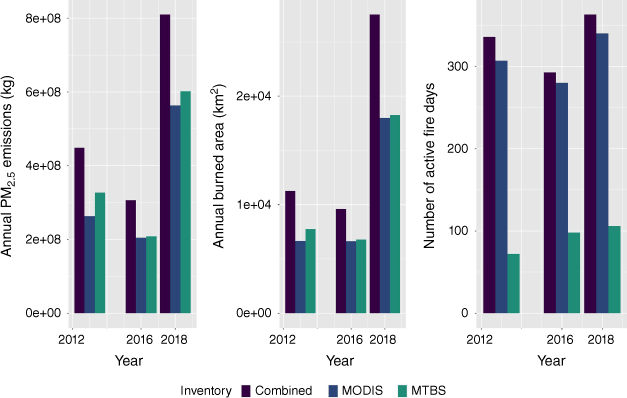
Combined FEI | WFEIS MODIS | Combined FEI percent increase (MODIS) (%) | WFEIS MTBS | Combined FEI percent increase (MTBS) (%) | ||
---|---|---|---|---|---|---|
PM2.5 (kg) | ||||||
2013 | 4.49 × 105 | 2.63 × 105 | 71 | 3.28 × 1017 | 37 | |
2016 | 3.06 × 105 | 2.05 × 105 | 49 | 2.09 × 1017 | 47 | |
2018 | 8.10 × 105 | 5.63 × 105 | 44 | 6.02 × 1017 | 34 | |
Burned area (km2) | ||||||
2013 | 1.13 × 104 | 6.65 × 103 | 69 | 7.74 × 109 | 45 | |
2016 | 9.60 × 103 | 6.63 × 103 | 45 | 6.78 × 109 | 42 | |
2018 | 2.75 × 104 | 1.80 × 104 | 53 | 1.83 × 1010 | 51 | |
Fire days (days) | ||||||
2013 | 336 | 307 | 9 | 72 | 367 | |
2016 | 293 | 280 | 5 | 98 | 199 | |
2018 | 362 | 340 | 6 | 106 | 242 |
The percentages represent the percent increase in the combined FEI as compared to the individual FEIs.
Resolving discrepancies
For the 3 years investigated in this study, 9–14% of the reported MTBS data had no MODIS data assigned. This accounted for a small percentage (3–4%) of the annual burned area as well as a small percentage (1–6%) of PM2.5 emissions. This indicates that these unassigned points are small fires. Though the total emissions impact over the course of a year may be relatively low, it is imperative to include the daily emissions from small fires in models to accurately assess acute smoke exposure. Capturing these daily emissions from small fires can also help with identifying the health effects of prescribed burns, which are primarily small fires. Using linear regression to assign fire length and evenly distribute PM2.5 and the burned area may not perfectly reflect real-life scenarios, but it plays a vital role in providing daily information on small fires that the MODIS dataset might overlook.
Cloud cover
Table 2 shows the amount of information the cloud cover interpolation added for 2013, 2016, and 2018. For 2013, 2016, and 2018, the interpolation had an average increase of 1% for PM2.5 concentration, 5% for burned area, and 4% for fire days per year compared to the combined FEI without the cloud cover interpolation. While these increases may seem slight, it is crucial to note that these fire days were not previously captured. The cloud cover interpolation adds a relatively small overall percentage of information, confirming that it predominantly accounts for mostly low-intensity fire days. Including low-intensity fire days in the analysis is crucial to ensure that the daily progression needed to understand acute health effects is as accurate as possible.
Combined FEI | No interpolation | Cloud cover interpolation | Cloud cover percent increase (%) | |
---|---|---|---|---|
PM2.5 (kg) | ||||
2013 | 4.46 × 105 | 4.49 × 105 | 1 | |
2016 | 3.02 × 105 | 3.0 × 1055 | 2 | |
2018 | 8.03 × 105 | 8.10 × 105 | 1 | |
Burned area (km2) | ||||
2013 | 7.13 × 103 | 7.49 × 103 | 5 | |
2016 | 6.89 × 103 | 7.37 × 103 | 7 | |
2018 | 1.80 × 104 | 1.87 × 104 | 4 | |
Fire days (days) | ||||
2013 | 317 | 336 | 6 | |
2016 | 281 | 293 | 4 | |
2018 | 359 | 362 | 1 |
Fig. 5 shows the daily fire progression for the 2014 Happy Camp fire, indicating both the original FEI and interpolated points. The low fire intensity days at the beginning and end of the fire required the most interpolation, likely because the smaller fire intensity days are more susceptible to cloud cover and other remote sensing issues. A NASA visible satellite image in Fig. 6 shows a day that did not need cloud cover interpolation, Fig. 7 shows a cloudy day during the peak of the fire that did not need cloud cover interpolation, and Fig. 8 shows a cloudy day near the end of the fire that did need cloud cover interpolation. The cloudy day (Fig. 8) has fewer thermal anomaly detections than the clear day (Fig. 6), highlighting the remote sensing issues due to heavy cloud cover. The cloudy day at the end of the fire (Fig. 8) was not captured in the fire emissions inventory and required interpolation. A comparison between fire radiative power (FRP) and MODIS detection confidence level (Fig. 9) reinforces the remote sensing issues shown in the satellite visible images. MODIS detects more thermal anomalies on a clear day than on a cloudy day, and several of these thermal anomalies have a high confidence level despite relatively low FRP. The cloudy day thermal anomaly detections show a higher correlation between FRP and confidence level, indicating that on a cloudy day, MODIS is more confident in detecting larger fires than smaller fires.
The 2014 Happy Camp fire in California after cloud cover interpolation. Interpolated points show the daily PM2.5 emitted in teragrams (1012 g), estimated by linear regression between the nearest FEI points.
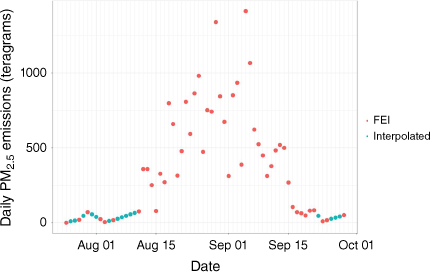
Visual satellite image of the Happy Camp fire on 29 August 2014, from NASA WorldView. Orange circles represent MODIS thermal anomalies, showing the location of the fire (the area of interest is denoted by a larger red circle). This is an example of a clear day with no satellite remote sensing issues in the FEI.
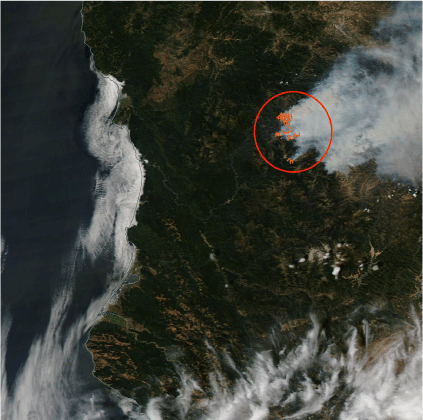
Visual satellite imagery of the Happy Camp fire on 30 August 2014, from NASA WorldView. Orange circles represent MODIS thermal anomalies, showing the location of the fire (the area of interest is denoted by the larger red circle). This represents a day with clouds where the FEI still captured thermal anomalies, likely because this was a high intensity fire day when the fire was at its largest.
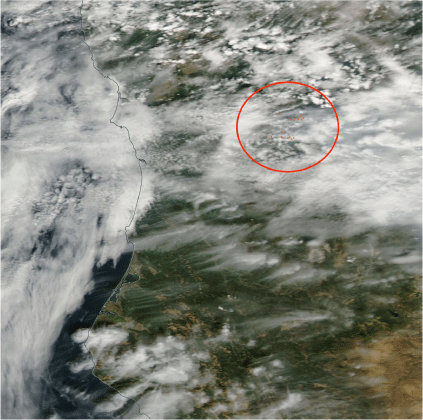
Visual satellite image of the Happy Camp fire on 22 September 2014, from NASA WorldView. Orange circles represent thermal anomalies sensed by the MODIS satellite, showing the location of the fire (the area of interest is denoted by a larger red circle). There are fewer thermal anomaly detections on this day compared to the previous day, which was clear. This day was not captured in the FEI and required interpolation. This day was near the end of the fire and was a lower intensity fire day.
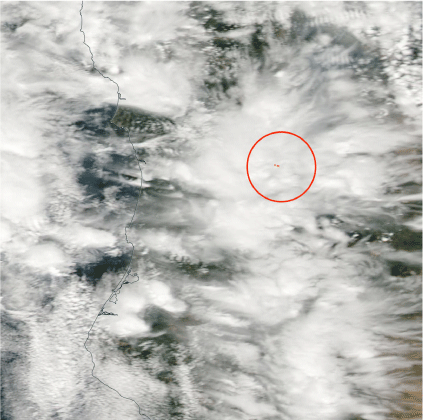
MODIS detection confidence level versus fire radiative power (FRP) in megawatts for each thermal anomaly detected for the Happy Camp Fire on 29 August (red) and 30 August (yellow) 2014. On the clear day (29 August, red), there is some correlation between FRP and the detection confidence level, meaning that the size and heat of the fire impact the confidence of the detection from MODIS.
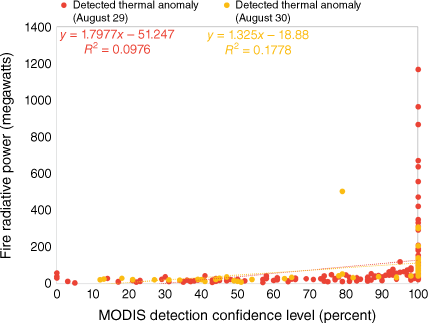
Conclusion
Combining two sources of burned area information to create a single FEI captures more information on fire emissions than relying on a single source of burned area information. Further, including a temporal interpolation method to gap-fill missing data addresses remote sensing issues in FEIs (i.e. missing data due to cloud cover). These updates are crucial to providing daily estimates of smoke exposure for acute health effects studies, where missing days of fire emissions data can pose a significant problem. This is important because many FEIs used for health effects studies have uncertainties due to remote sensing issues, and these remote sensing issues cause gaps in daily fire progression information, which may result in missing smoke exposure when used in an acute health effects study (Black et al. 2017; Fann et al. 2018). Many methods proposed to improve FEIs, like aircraft campaigns or updated remote sensing instruments, require significant resources (Larkin et al. 2014). The improvements described in this study to create the combination FEI can be implemented using currently available data. Our combined FEI dataset provides improved fire emissions inputs for models that estimate human exposure to wildfire smoke for acute health effects studies. While there are still uncertainties in the combined FEI, especially related to the fuel loading and emissions factors, for an acute health study, it is critical to have a daily timeseries of smoke exposures. Therefore, this combined FEI is a significant improvement over other approaches because it provides daily fire emissions over a 12-year period (2007–2019) using a consistent method (i.e. no time series differences due to FEI method changes). Previous research in this area reaffirms the importance of combining different data sources to better represent fire activity (Larkin et al. 2020; McClure et al. 2023).
The data presented on FRP versus MODIS thermal anomaly detection confidence levels show that MODIS struggles to sense low FRP fires on cloudy days, highlighting the solutions addressed by adding a high spatial resolution source of burned area information that is less affected by cloud cover issues and by adding the cloud cover interpolation. A comparison of results using each source of burned area information individually and the combined FEI shows that using the two sources provides more information than the FEI for each source of burned area individually. The cloud cover interpolation provides more information than the combined burned area inventory. It provides a consistent time series for use in acute health effects studies. This approach to addressing missing data is an important improvement for health effects studies. When daily smoke exposures are missing, public health researchers may be inclined to drop those days from their analyses, which results in a reduction in statistical power and a loss of information about smoke days. Alternatively, public health researchers may decide to implement an interpolation approach to compensate for missing data, and these researchers may not have sufficient expertise in fire science to appropriately adapt for the missing exposure days. The approach we have described for creating a combined FEI offers a practical solution for these challenges.
Assessing the quality of FEI data is crucial because FEIs serve as input for models that estimate the concentration of pollutants from wildfire smoke. Several studies (Roy et al. 2007; Soja et al. 2009) acknowledge the limitations of current FEIs and propose validation methods. However, many of the proposed validation efforts are often in-depth studies and resource-intensive field campaigns. While these provide invaluable knowledge, these methods also have limitations in terms of scalability, cost effectiveness, and timeliness of data availability. Another major hurdle when looking to validate FEIs is the absence of a method to directly measure the emissions from a single fire (Hao and Larkin 2014; Black et al. 2017). The complex nature of fire behaviour makes it impossible to establish a robust validation dataset, so FEIs will continue to face uncertainties. Future work to further understand the relationship between MODIS confidence levels and fire characteristics, as well as understanding the issues that may arise from satellite fire detections in dense canopy cover would be another useful step to improve FEIs.
The combined dataset addresses specific issues with using FEIs to estimate human exposure to wildfire smoke. While it does not solve all problems, it represents a functional improvement achievable with existing datasets. When using FEIs to study acute health effects, it is crucial to have a daily time series of exposure estimates that are as complete as possible. We show that combining two sources of burned area information into a single FEI and using a cloud cover interpolation creates an FEI dataset with more information on fires and no gaps in daily fire progression.
Data availability
The data that support this study will be shared upon reasonable request to the corresponding author.
Declaration of funding
This work is supported in part by the National Institutes of Health under award number R01ES029528.
Acknowledgements
The support and resources from the Center for High Performance Computing at the University of Utah are gratefully acknowledged. We acknowledge the use of imagery from the NASA Worldview application (https://worldview.earthdata.nasa.gov), part of the NASA Earth Observing System Data and Information System (EOSDIS). The fire emissions information data that supports this study can be found on the WFEIS website at https://wfeis.mtri.org/home.
References
Aguilera R, Corringham T, Gershunov A, Benmarhnia T (2021) Wildfire smoke impacts respiratory health more than fine particles from other sources: observational evidence from Southern California. Nature Communications 12, 1493.
| Crossref | Google Scholar | PubMed |
Black C, Tesfaigzi Y, Bassein JA, Miller LA (2017) Wildfire smoke exposure and human health: significant gaps in research for a growing public health issue. Environmental Toxicology and Pharmacology 55, 186-195.
| Crossref | Google Scholar | PubMed |
Borchers Arriagada N, Horsley JA, Palmer AJ, Morgan GG, Tham R, Johnston FH (2019) Association between fire smoke fine particulate matter and asthma-related outcomes: systematic review and meta-analysis. Environmental Research 179, 108777.
| Crossref | Google Scholar | PubMed |
Eidenshink J, Schwind B, Brewer K, Zhu Z-L, Quayle B, Howard S (2007) A project for monitoring trends in burn severity. Fire Ecology 3, 3-21.
| Crossref | Google Scholar |
Fann N, Alman B, Broome RA, Morgan GG, Johnston FH, Pouliot G, Rappold AG (2018) The health impacts and economic value of wildland fire episodes in the U.S.: 2008–2012. Science of the Total Environment 610–611, 802-809.
| Crossref | Google Scholar |
Faulstich SD, Schissler AG, Strickland MJ, Holmes HA (2022) Statistical Comparison and Assessment of Four Fire Emissions Inventories for 2013 and a Large Wildfire in the Western United States. Fire 5, 27.
| Crossref | Google Scholar | PubMed |
French NHF, McKenzie D, Erickson T, Koziol B, Billmire M, Endsley KA, Yager Scheinerman NK, Jenkins L, Miller ME, Ottmar R, Prichard S (2014) Modeling Regional-Scale Wildland Fire Emissions with the Wildland Fire Emissions Information System. Earth Interactions 18, 1-26.
| Crossref | Google Scholar |
Gao Y, Huang W, Yu P, Xu R, Yang Z, Gasevic D, Ye T, Guo Y, Li S (2023) Long-term impacts of non-occupational wildfire exposure on human health: a systematic review. Environmental Pollution 320, 121041.
| Crossref | Google Scholar | PubMed |
Giglio L, Roy DP (2020) On the outstanding need for a long-term, multi-decadal, validated and quality assessed record of global burned area: caution in the use of Advanced Very High Resolution Radiometer data. Egyptian Journal of Remote Sensing and Space Sciences 2, 100007.
| Crossref | Google Scholar |
Giglio L, Schroeder W, Justice CO (2016) The collection 6 MODIS active fire detection algorithm and fire products. Remote Sensing of the Environment 178, 31-41.
| Crossref | Google Scholar | PubMed |
Hao WM, Larkin NK (2014) Wildland fire emissions, carbon, and climate: wildland fire detection and burned area in the United States. Forest Ecology and Management 317, 20-25.
| Crossref | Google Scholar |
Hawbaker TJ, Vanderhoof MK, Beal Y-J, Takacs JD, Schmidt GL, Falgout JT, Williams B, Fairaux NM, Caldwell MK, Picotte JJ, Howard SM, Stitt S, Dwyer JL (2017) Mapping burned areas using dense time-series of Landsat data. Remote Sensing of Environment 198, 504-522.
| Crossref | Google Scholar |
Keane RE (2013) Describing wildland surface fuel loading for fire management: a review of approaches, methods and systems. International Journal of Wildland Fire 22, 51-62.
| Crossref | Google Scholar |
Laris PS (2005) Spatiotemporal problems with detecting and mapping mosaic fire regimes with coarse-resolution satellite data in savanna environments. Remote Sensing of Environment 99, 412-424.
| Crossref | Google Scholar |
Larkin NK, Raffuse SM, Strand TM (2014) Wildland fire emissions, carbon, and climate: U.S. emissions inventories. Forest Ecology and Management 317, 61-69.
| Crossref | Google Scholar |
Larkin NK, Raffuse SM, Huang S, Pavlovic N, Lahm P, Rao V (2020) The Comprehensive Fire Information Reconciled Emissions (CFIRE) inventory: wildland fire emissions developed for the 2011 and 2014 U.S. National Emissions Inventory. Journal of the Air & Waste Management Association (1995) 70, 1165-1185.
| Crossref | Google Scholar | PubMed |
Li F, Zhang X, Kondragunta S, Lu X, Csiszar I, Schmidt CC (2022) Hourly biomass burning emissions product from blended geostationary and polar-orbiting satellites for air quality forecasting applications. Remote Sensing of Environment 281, 113237.
| Crossref | Google Scholar |
Liu JC, Mickley LJ, Sulprizio MP, Dominici F, Yue X, Ebisu K, Anderson GB, Khan RFA, Bravo MA, Bell ML (2016) Particulate air pollution from wildfires in the Western US under climate change. Climatic Change 138, 655-666.
| Crossref | Google Scholar | PubMed |
Liu C, Chen R, Sera F, Vicedo-Cabrera AM, Guo Y, Tong S, Coelho MSZS, Saldiva PHN, Lavigne E, Matus P, Valdes Ortega N, Osorio Garcia S, Pascal M, Stafoggia M, Scortichini M, Hashizume M, Honda Y, Hurtado-Díaz M, Cruz J, Nunes B, Teixeira JP, Kim H, Tobias A, Íñiguez C, Forsberg B, Åström C, Ragettli MS, Guo Y-L, Chen B-Y, Bell ML, Wright CY, Scovronick N, Garland RM, Milojevic A, Kyselý J, Urban A, Orru H, Indermitte E, Jaakkola JJK, Ryti NRI, Katsouyanni K, Analitis A, Zanobetti A, Schwartz J, Chen J, Wu T, Cohen A, Gasparrini A, Kan H (2019) Ambient Particulate Air Pollution and Daily Mortality in 652 Cities. The New England Journal of Medicine 381, 705-715.
| Crossref | Google Scholar | PubMed |
McClure CD, Pavlovic NR, Huang S, Chaveste M, Wang N (2023) Consistent, high-accuracy mapping of daily and sub-daily wildfire growth with satellite observations. International Journal of Wildland Fire 32, 694-708.
| Crossref | Google Scholar |
National Interagency Coordination Center (2014) Wildland Fire Summary and Statistics Annual Report 2014. Available at https://www.nifc.gov/sites/default/files/NICC/2-Predictive%20Services/Intelligence/Annual%20Reports/2014/Annual_Report_2014_508.pdf
Pan S, Gan L, Jung J, Yu W, Roy A, Diao L, Jeon W, Souri AH, Gao HO, Choi Y (2023) Quantifying the premature mortality and economic loss from wildfire-induced PM2.5 in the contiguous U.S. Science of The Total Environment 875, 162614.
| Crossref | Google Scholar | PubMed |
Reid C, Considine E, Watson G, Telesca D, Pfister G, Jerrett M (2023) Effect modification of the association between fine particulate air pollution during a wildfire event and respiratory health by area-level measures of socio-economic status, race/ethnicity, and smoking prevalence. Environmental Research: Health 1, 025005.
| Crossref | Google Scholar | PubMed |
Roteta E, Bastarrika A, Padilla M, Storm T, Chuvieco E (2019) Development of a Sentinel-2 burned area algorithm: generation of a small fire database for sub-Saharan Africa. Remote Sensing of Environment 222, 1-17.
| Crossref | Google Scholar |
Roy B, Pouliot GA, Gilliland A, Pierce T, Howard S, Bhave PV, Benjey W (2007) Refining fire emissions for air quality modeling with remotely sensed fire counts: a wildfire case study. Atmospheric Environment 41, 655-665.
| Crossref | Google Scholar |
Soja AJ, Al-Saadi JA, Giglio L, Randall D, Kittaka C, Pouliot GA, Kordzi JJ, Raffuse SM, Pace TG, Pierce T, Moore T, Roy B, Pierce B, Szykman JJ (2009) Assessing satellite-based fire data for use in the National Emissions Inventory. Journal of Applied Remote Sensing 3, 031504.
| Crossref | Google Scholar |
Urbanski SP, Reeves MC, Corley RE, Silverstein RP, Hao WM (2018) Contiguous United States wildland fire emission estimates during 2003–2015. Earth System Science Data 10, 2241-2274.
| Crossref | Google Scholar |
Wooster MJ, Roberts GJ, Giglio L, Roy DP, Freeborn PH, Boschetti L, Justice C, Ichoku C, Schroeder W, Davies D, Smith AMS, Setzer A, Csiszar I, Strydom T, Frost P, Zhang T, Xu W, de Jong MC, Johnston JM, Ellison L, Vadrevu K, Sparks AM, Nguyen H, McCarty J, Tanpipat V, Schmidt C, San-Miguel-Ayanz J (2021) Satellite remote sensing of active fires: history and current status, applications and future requirements. Remote Sensing of Environment 267, 112694.
| Crossref | Google Scholar |
Yao J, Eyamie J, Henderson SB (2016) Evaluation of a spatially resolved forest fire smoke model for population-based epidemiologic exposure assessment. Journal of Exposure Science & Environmental Epidemiology 26, 233-240.
| Crossref | Google Scholar |
Ye T, Xu R, Yue X, Chen G, Yu P, Coêlho MSZS, Saldiva PHN, Abramson MJ, Guo Y, Li S (2022) Short-term exposure to wildfire-related PM2.5 increases mortality risks and burdens in Brazil. Nature Communications 13, 7651.
| Crossref | Google Scholar |