Cross-landscape fuel moisture differences impact simulated fire behaviour
K. Little
A
B
C
D
E
F
G
Abstract
Predicting fire behaviour is an ongoing challenge in temperate peatlands and heathlands, where live fuels can form the dominant fuel load for wildfire spread, and where spatial heterogeneity in fuel moisture is important but not typically represented in fuel models.
We examine the impact of fuel moisture variation on simulated fire behaviour across a temperate peatland/heathland landscape.
We collected field measurements of fuel moisture content in Calluna vulgaris shrub from 36 sites across the North Yorkshire Moors, United Kingdom. We used these to define fuel moisture inputs within existing shrubland fuel models to simulate fire behaviour in BehavePlus.
Simulated rates of spread varied with fuel moisture content; average mean variance of 23–80% from the landscape average rate of spread. The driest sites had simulated rates of spread up to 135% above the landscape average and the wettest sites up to 86% below average. Fuel model selection dramatically impacted simulated rates of spread by a factor of five.
We need to constrain the role of live fuel moisture within temperate fuel models to develop accurate fire behaviour predictions.
Capturing cross-landscape heterogeneity in fire behaviour is important for safe and effective land and wildfire management decision-making.
Keywords: BehavePlus, Calluna vulgaris, fuel models, heathlands, live fuel moisture, peatlands, rate of spread, sensitivity analysis, shrub fuels, temperate ecosystems.
Introduction
Fire is integral to many ecosystems. Wildfires are a natural component of the landscape and there is a long history of human use of fire to manage landscapes (McLauchlan et al. 2020; Shuman et al. 2022). Global climate and land use changes are promoting increased interaction between humans and changing wildfire behaviour, including increasing burned area in many regions globally (Doerr and Santín 2016; Shuman et al. 2022).
One facet of this is the increasing wildfire risk in regions that have traditionally been considered to be less vulnerable to extreme wildfires, such as the temperate peatlands and heathlands of northwestern Europe (Belcher et al. 2021). Temperate peatlands and heathlands are ecosystems of high conservation importance that have an extensive history of landscape fire through both wild and managed fire that maintain biodiversity and reduce fuel loads (Davies et al. 2022). These ecosystems contain globally critical carbon stores that are vulnerable to smouldering combustion during severe wildfires and can result in significant carbon emissions (Mickler 2021). High intensity wildfires and their spatial patterns across peatlands and heathlands can have important ecological impacts in terms of post-fire vegetation and peat soil recovery, and potential water quality, infrastructure, and societal impacts (Doerr and Santín 2016). High rates of spread affect the controllability of fires and may result in managed fires ‘escaping’ their burn areas (Davies et al. 2009). Alignment of fuel and weather conditions conducive to wildfires in temperate peatlands and heathlands promote increased wildfire activity and their associated ecological consequences (Kirkland et al. 2023). Moreover, the seasonal window for conducting burning to meet management objectives is decreasing alongside changing policy and viewpoints on the use of fire to manage landscapes (Minsavage-Davis and Davies 2022; Pandey et al. 2023). Accurate fire behaviour predictions are therefore critical for safe, effective land and wildfire management decision-making. Understanding spatial variability in wild and managed fire behaviour is important for determining how fire risk may change across a landscape, including potential impacts on vegetation recovery and carbon stores. This has implications for determining suppression tactics and resource requirements within wildfire response operations, as well for determining suitable locations for burning to meet land management objectives while minimising the risk of out of control fires or failed ignitions (Minsavage-Davis and Davies 2022).
Modelling fire behaviour
Fire behaviour models use simplified inputs of fuel, weather, and topography to assess potential fire behaviour, including rate of spread (ROS), flame length, and fireline intensity. Rothermel’s surface fire spread model is a fundamental model used to predict fire behaviour within surface fuels (Rothermel 1972). BehavePlus is a fire behaviour modelling system that provides a user interface to Rothermel’s model (among others). BehavePlus is widely used to predict fire behaviour for different scenarios across fire management, prescribed fire planning, and research applications (Andrews 2014). It provides point-based fire behaviour metrics that can also be integrated with systems like FlamMap and FARSITE to model fire behaviour in space (Finney 1998, 2006). Wildfire Analyst operationalised fire spread models for real-time wildfire risk forecasting, wildfire spread predictions, wildland fire behaviour analysis, and risk mitigation planning in many regions (Monedero et al. 2019), including temperate regions such as The Netherlands (Cardil et al. 2021). BehavePlus has previously been used to predict surface fire behaviour in Calluna vulgaris (Davies et al. 2009) and was recently used by Minsavage-Davis and Davies (2022) to evaluate ROS models in heathlands.
Temperate peatland and heathland fuel models
Fuel models are used to describe fuel availability in fire behaviour models and exist for a range of fuel types, including shrubland fuels (Scott and Burgan 2005). These are designed for non-specific vegetation types and rely on user selection. The choice of fuel model is important in determining the proportion of live to dead fuels and therefore sensitivity to fuel moisture changes. Dead fuel moisture content is the water content of dead vegetation and is important for the initial ignition of fuels. Because dead fuels respond mainly to changing weather conditions, there are simple models that can be used for predicting dead fuel moisture content. For example, the Fosberg model uses air temperature and relative humidity measurements to predict 1-h fine fuel moisture, making adjustments for time of year, slope, aspect, and shading of the vegetation (Fosberg et al. 1971). Live fuel moisture content, the water content of living vegetation, is different as it can regulate drying through ecohydrological and plant physiological controls (Dickman et al. 2023).
In the dwarf shrub Calluna vulgaris L. Hull. (hereafter Calluna) that dominates peatland and heathland landscapes, both dead and live fuel moistures are important for wildfire behaviour (Davies et al. 2009, 2010). Despite increasing wildfire risk associated with climate and land use change, predicting fire behaviour continues to be challenging within these environments (Cardíl et al. 2023). Intense fire behaviour has been observed in temperate shrublands during fire weather conditions that are considered to be low risk in forested landscapes (Davies et al. 2019; Pepin and Wotton 2020). This questions whether existing fuel models are able to incorporate the role of live fuel moisture content that can drive spring-time wildfires in peatlands and heathlands (Jolly and Johnson 2018). This is an ongoing challenge for temperate landscapes even within traditionally fire prone countries (e.g. Canada (Pepin and Wotton 2020)) in addition to emerging fire prone temperate regions like northwestern Europe (Cardíl et al. 2023). We need to understand fuel moisture dynamics to constrain functioning fuel models for key fuel types in temperate peatlands and heathlands.
Some previous research has focused on the influence of live fuel moisture content of Calluna on flammability, fire ignition, and ROS (e.g. Davies and Legg 2008, 2011; Santana and Marrs 2014; Log 2020). Ecological research on the life cycle of Calluna (e.g. Kayll and Gimingham 1965; Gimingham 1988) provided the foundation for understanding differences in fire behaviour between pioneer, building, mature, and degenerate growth phases, leading to the development of empirical ROS models and later fireline intensity and flame length for these different growth phases of Calluna (Davies et al. 2009, 2019). Cardíl et al. (2023) used Visible Infrared Imaging Radiometer Suite (VIIRS) remotely sensed hotspots to derive the ROS for wildfires across northwestern Europe. Understanding the response of fire behaviour models to the range of live fuel moisture conditions across a landscape is critical in addressing scenarios where models may underestimate fire behaviour. Phenologically-driven declines in live fuel moisture at the end of winter and into spring are particularly important drivers of wildfire risk in Calluna-dominated landscapes, which are critical to capture in fire behaviour predictions (Davies et al. 2010; Log et al. 2017).
Impact of fuel moisture spatial complexity on fire behaviour
Fuel loadings and fuel moisture are represented by single values within fuel models yet are highly spatially variable in reality (Jolly 2007). We recently conducted an intensive fuel moisture measurement campaign to capture the magnitude of cross-landscape fuel moisture differences in a temperate peatland/heathland landscape. We found that this variability created spatial discontinuity in the availability of fuel for sustained ignition of live fuels and in the potential for smouldering combustion in organic soils Little et al. (2024). However, the influence of cross-landscape fuel moisture differences on simulated fire behaviour, particularly live fuel moisture, is still largely unknown.
Fire behaviour within emerging fire prone environments like the temperate peatlands and heathlands of northwestern Europe can be affected by small-scale heterogeneity in landscape and fuel characteristics. Some previous research has examined the sensitivity of fire behaviour models using hypothetical live fuel moisture scenarios (Jolly 2007; Minsavage-Davis and Davies 2022). Jolly (2007) systematically varied live fuel moisture content across existing standard fuel models, finding high sensitivities to live fuel moisture depending on the fuel model used. Minsavage-Davis and Davies (2022) evaluated different ROS models against observed ROS from experimental burns in Calluna heathlands. They found that various implementations of the Rothermel surface spread model could adequately predict ROS observed in Calluna heathlands. Both studies reported a lack of sensitivity in existing fire behaviour models to live fuel moisture content (Jolly 2007; Minsavage-Davis and Davies 2022). However, there are no studies that have evaluated fire behaviour predictions using a cross-landscape range of direct live and dead fuel moisture content measurements. These field-based fuel measurement campaigns are needed to develop robust fuel models for temperate fuel types that inform fire behaviour predictions.
Research questions
We examine the impact of cross-landscape fuel moisture variation on simulated rate of spread across a temperate peatland and heathland landscape utilising direct fuel moisture content measurements in BehavePlus. We address the following specific research questions: (1) To what extent does landscape-scale fuel moisture variability impact simulated fire behaviour? (2) How is cross-landscape simulated fire behaviour influenced by existing fuel models used for temperate shrubby fuels? (3) Does cross-landscape simulated fire behaviour change when different live and dead Calluna fuel layers are used? (4) Can existing operational dead fuel moisture models adequately capture cross-landscape predicted fire behaviour?
Methods
Study region
We measured cross-landscape variability in the fuel moisture content of Calluna within the North Yorkshire Moors National Park, United Kingdom (Fig. 1a). The North Yorkshire Moors are dominated by extensively managed Calluna-dominated temperate peatland and wet heathland ecosystems (Simmons 1990). The landscape has been traditionally managed largely by rotational burning to create a mosaic of mixed-age homogenous Calluna plots for grouse habitat and fuel management (Glaves et al. 2020; Davies et al. 2022). Between the different life stages of Calluna, this mosaic creates spatial differences in potential wildfire behaviour and impacts. As such, the plots enabled a clear factorial experimental design to be constructed across the diversity of landscape characteristics within the regional oceanic climate.
Study region of the North Yorkshire Moors, UK reproduced from Little et al. (2024) (a). Live and dead Calluna fuel samples were collected from a total of 36 sites across the landscape on 5 days in spring and summer 2021. Source: Esri, Maxar, Earthstar Geographics, and the GIS User Community. © Natural England copyright. Contains Ordnance Survey data © Crown copyright and database right 2022. Image of Calluna at one of the sites visualising the dominant live fuel load compared to fine dead fuel load (b). Image credit: Kerryn Little.
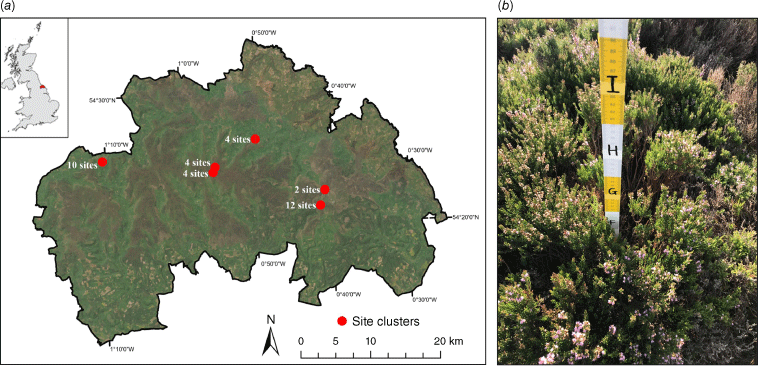
Sampling design
The following sections outline the field sampling design and fuel moisture measurements collected. We recently used these data to examine the landscape and micrometeorological controls on cross-landscape fuel moisture variation, and full details on the field experimental design can be found in Little et al. (2024). Briefly, we established 36 plots of 20 × 20 m homogenous Calluna across the landscape, with each plot comprising a unique combination of soil textures, slope, positions, aspects, and canopy ages that make up the diversity of the North Yorkshire Moors. We distinguished canopy age using time-since-last-burn records from land managers. ‘Building canopy’ plots were burned in the last 5–10 years and averaged 30 cm height with an accumulated moss/litter layer depth of 2.5 cm. ‘Mature canopy’ plots burned 15–20 years ago and averaged 60 cm in height with an accumulated moss/litter layer depth of 5 cm.
Field measurements
We collected fuel samples during hot, dry fire weather conditions between 11:00 and 17:00 local time, randomising the order of plots visited as much as logistically possible (see Little et al. 2024 for full details). Briefly, we collected three sets of samples during a 1-week period of drying in April, and two further sets in June and July to capture the peak spring and summer wildfire seasons of 2021. We sampled seven fuel layers in each plot to capture the vertical variation in fuel moisture: Calluna live canopy, live stems (<2 mm diameter), dead canopy, dead stems (<2 mm diameter), surface moss (top 2 cm), litter (top 2 cm), and the organic layer beneath the Calluna (top 5 cm of organic material above mineral soil). Of these seven fuel layers, BehavePlus only uses the live and dead fine fuel moisture inputs to model fire behaviour, so only the live and dead Calluna canopy and stem fuel layers are described further in this manuscript. We collected sprigs of live and dead Calluna following the protocol of Little et al. 2023. Briefly, ~10 sprigs with stem diameter <2 mm were collected from different plants along a 25-m transect to ensure fuel samples were representative of the entire plot area. We separated the leafy canopy material from the woody lower stems into separate 250 mL aluminium tins filled 3/4 full. We measured gravimetric fuel moisture content (mass of water per mass of dried sample, %, Eqn 1) by recording the wet weight of tinned samples, drying the samples for at least 48 h at 80°C, and reweighing the dried samples.
The 36 plots were spread across the landscape in pairs of building and mature canopy Calluna. At each pair of plots, we housed HOBO U23-001A PRO V2 (Onset Computer Corporation, Bourne, MA, USA) sensors in radiation shields to monitor 1.25 m air temperature and relative humidity at 15-min intervals.
Fuel models
We opted to use the shrub fuel models available within BehavePlus without any additional parameter customisation. We recognise that customised fuel models such as those developed by Davies et al. (2009) that tailor specific fuel physical and chemical parameters for Calluna are also available; however, we opted not to use these as they contain a high live herbaceous fuel load that was not representative of our study region. Of the preset shrub fuel models, we selected Scott and Burgan’s (2005) SH3 and SH6 shrub fuel models as these best represented the range of shrub fuel load and fire behaviour scenarios for Calluna-dominated heathlands and are therefore suitable for examining cross-landscape differences due to fuel moisture content variation. The SH3 and SH6 models have both been used in temperate peatlands and heathlands: the SH3 fuel model in Northern Ireland and SH6 in the Netherlands (Stoof et al. 2020) and Nova Scotia, Canada (Pepin and Wotton 2020). The SH3 fuel model describes a moderate fuel load with a high live (1.39 kg/m²) compared to fine dead (0.10 kg/m²) fuel load and fuel height of 0.73 m. The SH6 fuel model describes dense shrublands where there is a higher fine dead fuel load (0.65 kg/m²) compared to fine live fuels (0.31 kg/m²) and fuel height of 0.61 m.
We used the live and dead fuel moisture categories in BehavePlus, which describe the fine (<2 mm diameter) live and dead shrub fuel moisture contents, to input our measured fuel moisture content values. We used different combinations of measured live and dead Calluna fuel moisture content as the fuel moisture inputs in simulations to explore the response of the models to fuel moisture content (Tables 1, 2). In addition to using the canopy and stem fuel moisture inputs individually in simulations, we also used the plot-average combined fuel moisture content (the average of the canopy and stem fuel moisture contents for the plot). We calculated the landscape average fuel moisture content as the average of all the plot-average combined fuel moisture contents.
Fuel moisture/ROS scenario name | Fuel moisture description | ROS dead fuel moisture input | ROS live fuel moisture input | |
---|---|---|---|---|
Live canopy | Observed live canopy FMC | Average dead | Live canopy | |
Live stems | Observed live stem FMC | Average dead | Live stems | |
Live combined | Plot average of observed live canopy and live stem FMC | Average dead | Live combined | |
Average live | Landscape average of live combined FMC across all plots | Average dead | Average live | |
Dead canopy | Observed dead canopy FMC | Dead canopy | Average live | |
Dead stems | Observed dead stem FMC | Dead stems | Average live | |
Dead combined | Plot average of observed dead canopy and dead stem FMC | Dead combined | Average live | |
Average dead | Landscape average of dead combined FMC across all plots | Average dead | Average live | |
Fosberg dead unshaded | Predicted dead FMC from the unshaded Fosberg model using observed temperature and relative humidity measurements at time of sampling | Fosberg dead unshaded | Average live | |
Fosberg dead shaded | Predicted dead FMC from the shaded Fosberg model using observed temperature and relative humidity measurements at time of sampling | Fosberg dead shaded | Average live |
Fuel moisture and consequent ROS scenarios are named by the fuel layer of the fuel moisture content that is varying in the ROS predictions. For example, the live canopy scenario utilises field measured variable live canopy fuel moisture content and landscape average dead fuel moisture content in BehavePlus to predict ROS. Each plot has field collected fuel moisture contents for the following layers: live canopy, live stems, dead canopy, and dead stems. The combined scenarios refer to the average of the canopy and stem fuel moisture content for each of the 36 plots, calculated separately for live and dead Calluna. The average scenarios are calculated as the overall landscape average of the 36 plots combined fuel moisture contents for live and dead Calluna.
Date | Live combined range of FMC | Average live FMC | Dead combined range of FMC | Average dead FMC | |
---|---|---|---|---|---|
Mean (live canopy + live stem FMC) across the 36 plots | Landscape mean (live combined FMC) | Mean (dead canopy + dead stem FMC) across the 36 plots | Landscape mean (dead combined FMC) | ||
15/4/2021 | 51.8–76.3% | 67.9% (s.d. = 5.1) | 9.0–30.7% | 14.8% (s.d. = 4.5) | |
18/4/2021 | 52.4–73.1% | 63.3% (s.d. = 4.9) | 6.7–35.5% | 13.3% (s.d. = 6.5) | |
23/4/2021 | 42.0–71.2% | 60.3% (s.d. = 6.5) | 5.1–28.3% | 11.0% (s.d. = 6.3) | |
13/6/2021 | 66.1–109.1% | 88.9% (s.d. = 12.2) | 9.3–20.1% | 11.9% (s.d. = 2.0) | |
22/7/2021 | 66.5–123.4% | 100.0% (s.d. = 14.6) | 7.4–17.7% | 12.3% (s.d. = 2.6) |
The combined (plot average of canopy and stem FMC) range of FMC% and the landscape average FMC (calculated by averaging the combined FMCs) and standard deviations (s.d.) are given for both live and dead Calluna for each sampling date.
We also calculated dead fuel moisture content using the Fosberg model that uses plot relative humidity and temperature measurements at time of sampling for one case study each in spring (23 April 2021) and summer (22 July 2021). The Fosberg model is used to estimate fine dead fuel moisture content from relative humidity and temperature measurements and can be adjusted for canopy shading, elevation, aspect, slope, and season (Fosberg et al. 1971). The model has been incorporated into operational tools because of its simplicity and accessible data inputs, including look-up tables for predicting dead fine fuel moisture content and probability of ignition that are used within the National Wildfire Coordinating Group Incident Response Pocket Guide (NWCG 2022) and fire weather instruments, such as the Kestrel Fire Weather Meters (Kestrel Instruments, Boothwyn, PA). The Fosberg model was also integrated into Rothermel’s surface fire spread model in the USA and associated fire prediction modelling systems (NWCG 2023).
Fire behaviour simulations
We used the Rothermel surface ROS model in BehavePlus to simulate surface ROS at each of the 36 plots across the landscape on each of the five sampling dates, using the plot measured fuel moisture contents as fuel moisture inputs. We ran a series of different fuel simulations for each sampling date to address each of our research questions (Table 3). We kept all factors except fuel moisture constant, using 10 km/h midflame wind speed and 10% slope steepness to isolate the influence of fuel moisture content on ROS conditions. This scenario represented a moderate wind speed and slope that could reasonably occur within the landscape without impacting the simulated fire behaviour by using high wind speeds and slopes. We used the SH3 model as the default fuel model as its higher live compared to dead fuel load is most representative of the high proportion of live to dead Calluna at the sample sites across the North Yorkshire Moors (Fig. 1b).
Simulation description | Fuel model | Dead fuel moisture content input | Live fuel moisture content input | Number of runs | |
---|---|---|---|---|---|
1: What is the predicted RoS for the landscape using only average FMC? | SH3 | Overall average dead for each date (5) | Overall average live for each date (5) | 5 | |
2: How variable is simulated RoS across a landscape? | SH3 | Dead combined FMC per plot (36) and date (5) | Live combined FMC per plot (36) and date (5) | 180 | |
3: How is predicted RoS impacted by the fuel model selected? | SH6 | Dead combined FMC per plot (36) and date (5) | Live combined FMC per plot (36) and date (5) | 180 | |
4: What live Calluna fuel layers are important to collect? | SH3 | Overall average dead FMC for each date (5) | A. Live combined FMC per plot (36) and date (5) | 540 | |
B. Live canopy FMC per plot (36) and date (5) | |||||
C. Live stem FMC per plot (36) and date (5) | |||||
5: What dead Calluna layers are important to collect? | SH3 | A. Dead combined FMC per plot (36) and date (5) | Overall average live FMC for each date (5) | 540 | |
B. Dead canopy FMC per plot (36) and date (5) | |||||
C. Dead stem FMC per plot (36) and date (5) | |||||
6: Do existing operational tools perform just as well? | SH3 | A. Dead combined FMC per plot (36) and date (2) | Overall average live FMC for each date (2) | 144 | |
B. Fosberg dead unshaded predicted FMC for each plot (36) and date (2) | |||||
C. Fosberg dead shaded predicted FMC for each plot (36) and date (2) |
Total number of unique runs = 1589 (simulation 6.A is a repeat of 5.A so does not contribute to the overall number of unique runs). The simulation description summarises the research question and the fuel model, dead and live fuel moisture content (FMC) inputs describe the set up of the BehavePlus simulation. Each simulation is run for every sampling date (5 runs) using either the landscape averaged FMC or individual plot FMC (36 runs). Simulation 1 is used to generate the null model of predicted rate of spread (ROS) using landscape averaged fuel moisture content.
Existing fire behaviour models used operationally generally use a single live and dead fuel moisture content estimate to represent the average fuel moisture content for the region. We therefore calculated the landscape-average simulated fire behaviour as a null model to compare to the variability in simulated fire behaviour using the range of observed fuel moisture contents (Table 3, simulation 1). This simulation used the landscape average live and dead fuel moisture content inputs to produce a single average simulated ROS for the landscape. The main simulation of cross-landscape ROS variability uses the plot average canopy and stem fuel moisture content as the fuel moisture inputs to characterise the extent of landscape fuel moisture variability and its influence on simulated ROS (Table 3, simulation 2) as summarised in Table 2. We repeated the main simulation using the SH6 fuel model for comparison (Table 3, simulation 3). To assess the differences in simulated ROS using different fuel layer inputs, we ran the model using plot live canopy fuel moisture inputs while keeping dead fuel moisture constant as the landscape average dead fuel moisture content. We repeated this simulation for live stem fuel moisture and then plot average combined live fuel moisture (Table 3, simulation 4). This simulation setup was reversed, varying dead canopy, dead stem, and plot average dead fuel moisture content while keeping live fuel moisture constant as the landscape average (Table 3, simulation 5). Finally, we simulated ROS for the Fosberg modelled dead fuel moisture contents (Table 3, simulation 6).
Statistical analyses
For each plot, we calculated the percentage difference in the simulated ROS (Table 3, simulation 2) from the overall average ROS (Table 3, simulation 1) for each date. This metric was used to show how much simulated fire behaviour varied across the landscape (rather than using absolute values that vary day to day). We used Kruskal–Wallis non-parametric tests to test for statistically significant differences between the predicted ROS values calculated using canopy, stem, and combined fuel moisture content for both live and dead Calluna for each date (Table 3, simulation 4 and 5). We performed pairwise Wilcoxon tests where Kruskal–Wallis tests were significant to determine which fuel layers were different. We followed the same approach to compare predicted rates of spread between the observed and Fosberg modelled fuel moisture inputs (Table 3, simulation 6). We used Spearman correlations to compare fuel moisture predictions using the Fosberg model with observed dead fuel moisture content. We conducted all statistical analyses in R version 4.0.3 (R Core Team 2022), using packages stats (inbuilt), car (Fox and Weisberg 2019), and Hmisc (Harrell 2022).
Results
Cross-landscape fuel moisture variability
We observed high spatial fuel moisture variability across the 36 plots in the North Yorkshire Moors (Fig. 2, Table 2). Live combined fuel moisture (the plot average of live canopy fuel moisture and live stem fuel moisture) was least variable in April (overall variability from 42.0 to 76.3% across sampling dates). Live combined fuel moisture content increased in variability through June (66.1–109.1%) and July (66.5–123.4%). Conversely, dead combined fuel moisture (the plot average of dead canopy and dead stem fuel moisture) was least variable in June and July and most variable in April. The landscape average dead fuel moisture content showed little variation between the April (11.0–14.8%), June (11.9%), and July (12.3%) sampling dates.
Figure showing the range of cross-landscape live (left) and dead (right) fuel moisture variability for the 36 plots across the North Yorkshire Moors on each of the sampling dates. Green points depict plot combined fuel moisture content (average of canopy and stem fuel moisture content). Dark purple points show the mean fuel moisture content for the landscape overall (average of plot combined fuel moisture content), which is variable across each sampling date.
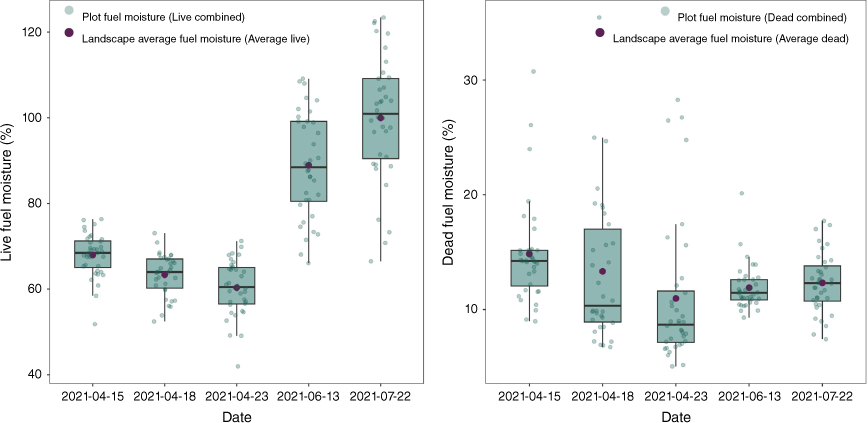
Cross-landscape predicted ROS variability
Cross-landscape fuel moisture differences resulted in a 23–80% mean variation from average ROS predicted using single fuel moisture inputs (Fig. 3, Table 4). The driest plots predicted rates of spread up to 135% above the landscape average. The range of variation from average ROS between the driest and wettest plots was between 46 and 160%. Predicted ROS variance and absolute ROS was highest in spring (Supplementary Fig. S1).
Box- and half violin-plots showing the percentage variation from average rate of spread (ROS) across the 36 plots in the North Yorkshire Moors for each sampling date. The landscape average ROS is specific to each sampling date: 1.7 m/min (15/4/2021); 1.9 m/min (18/4/2021); 2.2 m/min (23/4/2021); 1.5 m/min (13/6/2021); and 1.4 m/min (22/7/2021).
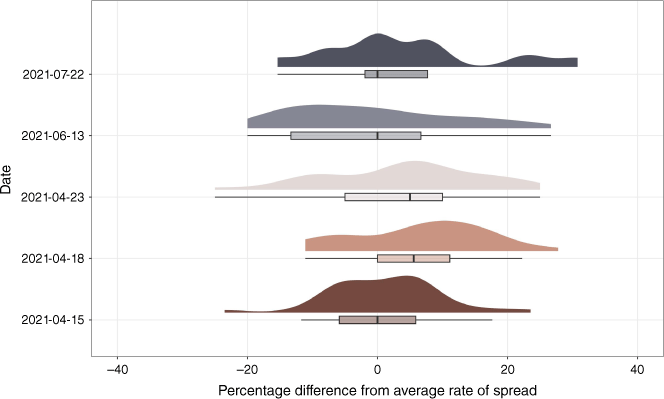
Date | Fuel model | Max ROS (m/min) | Min ROS (m/min) | Average ROS (m/min) | Max variation from average | Min variation from average | Mean variation from average | |
---|---|---|---|---|---|---|---|---|
15/4/21 | SH3 | 2.1 | 1.3 | 1.7 | 23.5 | −23.5 | 23.5 | |
18/4/21 | SH3 | 2.3 | 0.9 | 1.9 | 27.8 | −50.0 | 38.9 | |
23/4/21 | SH3 | 4.7 | 1.5 | 2.2 | 135.0 | −25.0 | 80 | |
13/6/21 | SH3 | 1.9 | 1.2 | 1.5 | 26.7 | −20.0 | 23.3 | |
22/7/21 | SH3 | 1.7 | 1.1 | 1.4 | 30.8 | −15.4 | 23.1 | |
15/4/21 | SH6 | 12.3 | 7.2 | 10.4 | 57.7 | −7.7 | 32.7 | |
18/4/21 | SH6 | 10.5 | 6.5 | 8.4 | 22.1 | −24.4 | 23.3 | |
23/4/21 | SH6 | 15.1 | 1.6 | 11.4 | 31.3 | −86.1 | 58.7 | |
13/6/21 | SH6 | 10.9 | 6.9 | 8.7 | 2.8 | −34.9 | 18.9 | |
22/7/21 | SH6 | 10.1 | 6.2 | 8.0 | 3.1 | −36.7 | 19.9 |
Absolute (m/min) and percentage variation from average ROS (%) results are shown, where average ROS is predicted from landscape averaged combined fuel moisture content under both the SH3 and SH6 fuel models (Table 2, simulation 1).
Simulated absolute ROS was significantly higher when the SH6 fuel model was used compared to the SH3 fuel model (Fig. 4). Average ROS was more than 5-fold higher overall when the SH6 fuel model was used to simulate ROS using the same fuel moisture content inputs. However, the mean ROS variance was comparable between the SH3 and SH6 simulations.
Boxplots showing the range of variability in predicted rate of spread (ROS) due to variation in plot combined fuel moisture content (average of canopy and stem fuel moisture content for each of the 36 plots) between the SH3 and SH6 fuel models for each sampling date. The SH3 fuel model is the main model used in the simulations of Table 3, which has a moderate fuel load with a high live (1.39 kg/m²) compared to fine dead (0.10 kg/m²) fuel load. We compare these results to the SH6 fuel model that has a higher fine dead (0.65 kg/m²) to live (0.31 kg/m²) fuel load (Table 3; simulation 3) and may represent scenarios where there is a high proportion of dead fuel.
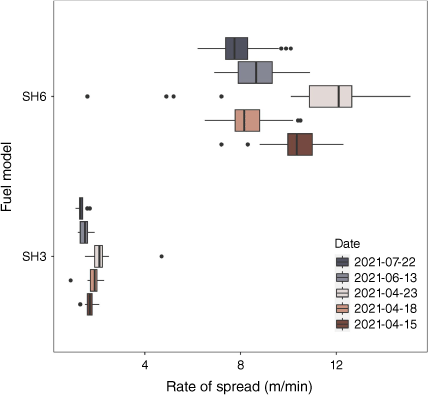
There were no statistically significant differences in predicted ROS when using dead canopy, dead stem, or combined fuel moisture contents. All live fuel moisture combinations led to significantly different ROS predictions, except for the June 13 field measurements (Tables 5, 6). Cross-landscape ROS predictions were more variable when live canopy Calluna fuel moisture values were used, leading to higher predicted rates of spread in spring and lower in summer (Fig. 5).
Fuel layer | Date | KW chi-squared | P-value | |
---|---|---|---|---|
Live | 15/4/2021 | 38.134 | 0.000 | |
Live | 18/4/2021 | 25.827 | 0.000 | |
Live | 23/4/2021 | 18.928 | 0.000 | |
Live | 13/6/2021 | 5.127 | 0.077 | |
Live | 22/7/2021 | 37.089 | 0.000 | |
Dead | 15/4/2021 | 0.269 | 0.874 | |
Dead | 18/4/2021 | 0.629 | 0.730 | |
Dead | 23/4/2021 | 0.015 | 0.993 | |
Dead | 13/6/2021 | 0.063 | 0.969 | |
Dead | 22/7/2021 | 1.908 | 0.385 | |
Predicted dead | 23/4/2021 | 6.546 | 0.038 | |
Predicted dead | 22/7/2021 | 0.756 | 0.685 |
Live fuel layers compare predicted ROS between live canopy, live stem, and combined live (average of canopy + stem) fuel moisture content measurement scenarios. Dead fuel layers compare predicted ROS in the same way but for measured dead fuel moisture contents. Predicted dead fuel layers use the Fosberg predicted shaded and unshaded dead fuel moisture contents as inputs. P < 0.05 differences are indicated in bold. Table 6 examines specific fuel layer differences for significant Kruskal–Wallis outcomes.
Pairwise fuel layers | 15/4/2021 | 18/4/2021 | 23/4/2021 | 13/6/2021 | 22/7/2021 | |
---|---|---|---|---|---|---|
Live combined × live canopy | 0.001 | 0.013 | 0.016 | 0.357 | 0.0025 | |
Live combined × live stems | 0.000 | 0.000 | 0.006 | 0.289 | 0.000 | |
Live stems × live canopy | 0.000 | 0.000 | 0.000 | 0.089 | 0.000 | |
Dead combined × Fosberg dead shaded | – | – | 0.270 | – | 0.570 | |
Dead combined × Fosberg dead unshaded | – | – | 0.000 | – | 0.000 | |
Fosberg dead unshaded × Fosberg dead shaded | – | – | 0.000 | – | 0.000 |
Statistically significant differences (P < 0.05) are indicated in bold.
Boxplots showing cross-landscape differences in predicted rate of spread (ROS) for each sampling date due to varying plot live fuel moisture content inputs. Live canopy and live stem scenarios describe predicted ROS due to plot live canopy and live stem fuel moisture content measurements, respectively. The live canopy + stem average scenario describes predicted ROS due to combined average live fuel moisture content for each plot. Dead fuel moisture content is kept as a single input of landscape-averaged combined dead fuel moisture content.
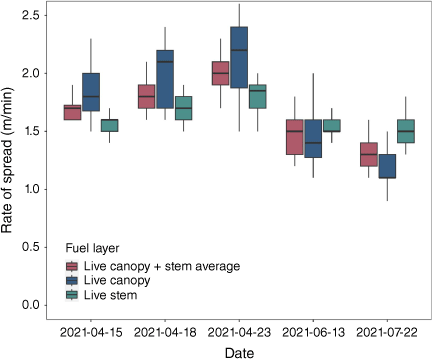
The Fosberg models predicted lower dead fuel moisture content using temperature and relative humidity measurements (Fig. 6a). The predictions using the shaded position Fosberg model were closer to observed dead fuel moisture despite the plots technically being unshaded. Predicted shaded (correlation coefficient = 0.48) and unshaded (0.46) fuel moisture content was, however, positively correlated with observed fuel moisture for July 22 (Supplementary Tables S1, S2). These differences did not translate to significantly different ROS predictions between the Fosberg shaded and observed combined dead fuel moisture simulations, but predicted ROS was significantly higher for the Fosberg dead unshaded simulations (Tables 5, 6). Predicted ROS was higher in spring than summer (Fig. 6b).
Boxplots showing variation between observed combined dead fuel moisture content (average of dead canopy and stem fuel moisture measurements for each plot) and predicted dead fuel moisture content (DFMC) using the Fosberg model that calculates DFMC from air temperature and relative humidity recorded at each plot. We calculated the Fosberg DFMC for both shaded and unshaded scenarios for each plot (a). Boxplots showing the consequent variation in predicted rates of spread (ROS) due to observed and predicted DFMC variation for a spring (23/4/2021) and summer (22/7/2021) example (b).
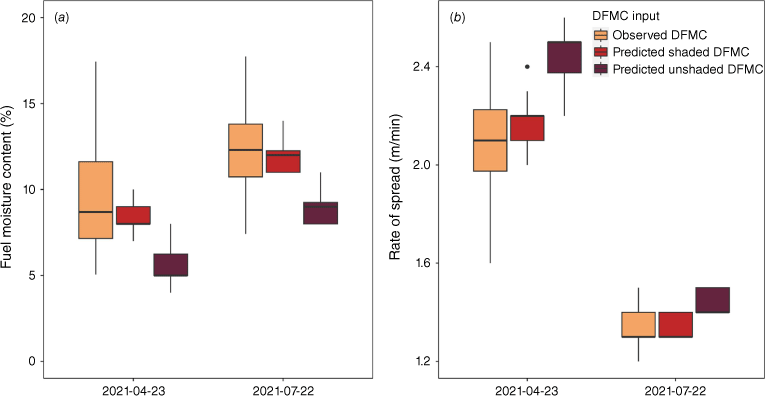
Discussion
Our research highlights the importance of including cross-landscape fuel moisture content differences in fire behaviour prediction models. This means it is also key that we collect fuel moisture content data and understand how its variability is controlled by landscape features. By comparing a null model with uniform fuel moisture content values to models that realistically represent the variability in fuel moisture across a landscape, our results show that the predicted fire behaviour differs significantly when incorporating natural variability in fuel moisture (Fig. 3, Table 4).
Landscape fuel moisture variability impacts simulated fire behaviour
Cross-landscape fuel moisture differences impacted predicted ROS by 23–80% on average, compared to predictions based on a single fuel moisture estimate for the entire landscape (Table 4). From an ecological perspective, the magnitude of variability in predicted fire behaviour will create spatial differences in the potential intensity and severity of a fire on the landscape and the consequent recovery of areas of the landscape to managed or wild fire. Understanding these differences may help to inform land management decision-making to minimise the potential impacts of managed or wild fire. Furthermore, while the absolute ROS predicted was low on the days we collected fuel samples and so cross-landscape variation would not likely cause concern, the magnitude of variation in expected fire behaviour may provide useful information for fire managers during more extreme scenarios, particularly where fire behaviour crosses thresholds for the safe use of suppression tools and tactics. Similarly, points on the landscape where ROS is slower may provide Fire and Rescue Services with opportunities to focus suppression activities or create fire breaks.
This study contributes to existing model sensitivity studies as it utilises the measured magnitude of fuel moisture variation across a landscape on the same day and thus represents the range of differences in fire behaviour that could be expected in such a landscape. Fuel moisture content was more variable in our field campaigns than the observed fuel moisture ranges in previous experimental burns (Davies et al. 2009; Minsavage-Davis and Davies 2022). Spatial fuel moisture differences are dependent on preceding and concurrent weather conditions, which may explain some of the variation in field-measured fuel moisture content differences (Little et al. 2024). Absolute ROS tended to be lower than previous research has observed from experimental burns (Davies et al. 2009). This is likely due to differences in the specific parameters of the fuel models used that may not be fully representative of the fuel characteristics of Calluna. In particular, the SH3 and SH6 models both have a lower heat of combustion of 18.622 kJ/kg compared to customised models for Calluna based on field and laboratory measurements (Davies et al. 2009; Belcher 2023). Heat of combustion is known to be important for ROS (Madrigal et al. 2010) and the lower values used in the SH3 and SH6 models may have led to underpredicted ROS. Understanding the ecological and phenological variability of Calluna and its impact on fuel moisture dynamics, fuel loads, and flammability will allow for the calibration of fuel models, such as those developed by Davies et al. (2009), for more accurate predictions of fire behaviour.
Simulated fire behaviour is impacted by choice of fuel layer and fuel model
Absolute ROS was significantly higher when the SH6 fuel family was used in place of the SH3 (Fig. 4). There are a range of fuel models that are used for temperate shrubby fuels (Scott and Burgan 2005), and we demonstrate that the choice of fuel model can dramatically affect predictions. Fine dead fuel load in the SH6 fuel model (0.65 kg/m²) was six times higher than that of the SH3 fuel model (0.10 kg/m²). We selected the SH3 model for the main simulations as our field measurements were collected in a high live fuel load landscape; however, under future climate change the proportion of live to dead fuel may change, and it is not unrealistic to think the higher dead fuel load SH6 fuel model may become more representative. A number of studies using climate projections point to future increased fire risk in the UK and northwest Europe due to increases in fire weather (Arnell et al. 2021; Perry et al. 2022). Moreover, the SH3 model contained a 0.67 kg/m² 10-h dead fuel load (representing 6.35–25.4 mm diameter dead fuels), which is unrealistic within many Calluna-dominated peatland and heathlands. As such, ROS is likely underpredicted in our simulations. In reality, a scenario somewhere between the SH3 and SH6 models would be most appropriate and highlights the importance of tailoring fuel models for robust predictions of fire behaviour.
Previous research has demonstrated fuel moisture differences between the different fuel layers of Calluna (i.e. canopy and stem fuel moisture differs for both live and dead Calluna) (Davies et al. 2010; Davies and Legg 2011). Predicted rates of spread were not significantly different when either dead canopy, dead stem, or a combined average was used. For simplicity, either material could be used from a fire behaviour modelling perspective. Predicted rates of spread were more variable for live Calluna canopy than stems or combined and predicted higher rates of spread in spring and lower in summer. Fire behaviour has proven challenging to model in temperate peatland and heathland fuels like Calluna where live fuel can form the dominant fuel load for fire spread during spring (Belcher et al. 2021). Moreover, previous experimental research found a strong relationship between Calluna canopy fuel moisture and variation in observed fire behaviour (Davies et al. 2009). In lieu of customised fuel models that specifically account for phenology, using Calluna canopy live fuel moisture for fire behaviour model inputs would help capture the role of phenology in influencing wildfire behaviour. Previous research has described fire behaviour in Calluna as akin to mini crown fires as sustained spread can occur through the canopy without consumption of the surface material (Fernandes et al. 2000; Davies and Legg 2016). As such, capturing Calluna canopy fuel moisture variation may provide the most realistic fire behaviour scenarios. These findings suggest that the underlying relationships that apportion the importance of dead and live fuel loads within the Rothermel family of fire spread models may need to be adjusted to better account for the importance of live fuel moisture in these fuel types.
Direct fuel moisture measurements are generally too time consuming to collect to be utilised in a wildfire response fire behaviour modelling capacity. In these scenarios we need to rely on modelled fuel moisture. The Fosberg model uses relative humidity and temperature to predict fine dead fuel moisture content. The ability to use a look-up table to generate quick predictions of fire behaviour is incredibly beneficial for response decision-making needs. These forms of fuel moisture models, like the Fosberg model, likely need to be customised for shrub fuel types that may not fully cure in the way grasses or litter do. Field-based measurements of fuel moisture in shrub fuels would allow for a correction factor to adapt existing models to temperate shrub ecosystems.
The Fosberg model was unable to capture the range of variability in fuel moisture content because factors beyond these meteorological controls also influence fuel moisture. While the unshaded model predicted lower fuel moisture contents and consequently higher ROS, the shaded model fuel moisture content was closer aligned to the observed dead fuel moisture despite a lack of shading effect from overstory forest canopy. The shaded Fosberg model simulated ROS that were not significantly different to those simulated using observed fuel moisture content (Fig. 6). This suggests that the density of Calluna canopy may shade understory fuels and make shaded fuel moisture predictions more appropriate to use, adding further weight to the narrative of Calluna behaving akin to a mini forest canopy.
Fire behaviour models are insensitive to cross-landscape live fuel moisture content
There is a broader issue of whether fire behaviour models are sufficiently sensitive to fuel moisture changes. While we measured high cross-landscape spatial fuel moisture variability (Fig. 2), the resulting variability in predicted ROS was much smaller (Supplementary Fig. S1). This is a reflection of the sensitivity of the Rothermel model to live fuel moisture. Previous experimental research has suggested that ROS is likely more sensitive to live fuel moisture changes than existing models predict, and this sensitivity increases with fuel aridity (Jolly 2007; Nolan et al. 2016; Pimont et al. 2019). This creates high risk scenarios in temperate regions where ROS may be underpredicted when live fuels are dry, such as at the end of winter and early spring when both managed burning and the main wildfire season are taking place in the UK’s peatland and heathland landscapes (Davies et al. 2010). There is a need to constrain the role of fuel moisture, particularly live fuel moisture, within models to develop accurate predictions of fire behaviour in temperate environments (Dickman et al. 2023). One such avenue to achieve this would be to develop live fuel moisture models that capture plant phenological and physiological processes and constrain the sensitivity of fire behaviour models to live fuel moisture of temperate fuels.
Implications for fire management
In emerging fire prone environments like the temperate peatlands and heathlands of northwestern Europe, spatial heterogeneity in fire behaviour as a result of landscape characteristics is relevant to consider. Cross-landscape fire behaviour is important for fire management decision making, particularly where this variability may require changes in suppression tools and tactics employed or provide opportunities for suppression. Capturing cross-landscape fire behaviour variation would allow strategic decision-making for conducting burns to safely and effectively achieve management outcomes. Existing fire behaviour prediction tools based on a single regional average fuel moisture input could underpredict fire behaviour and create dangerous situations for those suppressing wildfires or increase the risk of escaped managed burns. Likewise, overpredictions could limit opportunities for managed burning and increase suppression costs associated with wildfire event turnouts.
As the risk from wildfires in these environments increases, availability of tools like BehavePlus to inform decision-making become increasingly important. These tools require user decisions such as choice of fuel model and fuel layer inputs, which can significantly impact predictions. Minsavage-Davis and Davies (2022) suggested fire behaviour modelling systems using Rothermel’s surface fire spread model can be applied within Calluna heathlands but implored caution in interpreting predictions. While spatial variation in dead fuel moisture between Calluna fuel layers is not likely to impact predicted rates of spread, care should be taken in defining live fuel moisture inputs and emphasis should be put on capturing Calluna canopy fuel moisture. Our findings highlight the need to validate existing fuel models or develop tailored models that capture live fuel moisture dynamics and fuel loads in temperate shrub fuels.
Data availability
The data and code that support this study are available in FigShare at https://figshare.com/s/3d25e596a05ce1c3bc37, doi:10.6084/m9.figshare.25043603.
Declaration of funding
This project has received funding from the European Union’s Horizon 2020 research and innovation programme under the Marie Skłodowska-Curie grant agreement No 860787 (PyroLife Innovative Training Network) and the NERC Highlight project NE/T003553/1. LJG was supported by NERC grant NE/T009373/1.
References
Andrews PL (2014) Current status and future needs of the BehavePlus Fire Modeling System. International Journal of Wildland Fire 23, 21-33.
| Crossref | Google Scholar |
Arnell NW, Freeman A, Gazzard R (2021) The effect of climate change on indicators of fire danger in the UK. Environmental Research Letters 16, 044027.
| Crossref | Google Scholar |
Belcher CM, Brown I, Clay GD, Doerr SH, Elliott A, Gazzard R, Kettridge N, Morison J, Perry M, Smith TEL (2021) UK Wildfires and their Climate Challenges. available at https://www.researchgate.net/profile/Iain-Brown-8/publication/352569211_UK_Wildfires_and_their_Climate_Challenges/links/60e315dd92851ca944aa8655/UK-Wildfires-and-their-Climate-Challenges.pdf
Cardil A, Monedero S, Schag G, de-Miguel S, Tapia M, Stoof CR, Silva CA, Mohan M, Cardil A, Ramirez J (2021) Fire behavior modeling for operational decision-making. Current Opinion in Environmental Science and Health 23, 100291.
| Crossref | Google Scholar |
Cardíl A, Tapia VM, Monedero S, Quiñones T, Little K, Stoof CR, Ramirez J, de-Miguel S (2023) Characterizing the rate of spread of large wildfires in emerging fire environments of northwestern Europe using Visible Infrared Imaging Radiometer Suite active fire data. Natural Hazards and Earth System Sciences 23, 361-373.
| Crossref | Google Scholar |
Davies GM, Legg CJ (2008) Developing a live fuel moisture model for moorland fire danger rating. WIT Transactions on Ecology and the Environment 119, 225-236.
| Crossref | Google Scholar |
Davies GM, Legg CJ (2011) Fuel moisture thresholds in the flammability of Calluna vulgaris. Fire Technology 47, 421-436.
| Crossref | Google Scholar |
Davies GM, Legg CJ (2016) Regional variation in fire weather controls the reported occurrence of Scottish wildfires. PeerJ 4, e2649.
| Crossref | Google Scholar | PubMed |
Davies GM, Legg CJ, Smith AA, McDonald AJ (2009) Rate of spread of fires in Calluna vulgaris-dominated moorlands. British Ecological Society 46, 1054-1063.
| Crossref | Google Scholar |
Davies GM, Legg CJ, O’Hara R, MacDonald AJ, Smith AA (2010) Winter desiccation and rapid changes in the live fuel moisture content of Calluna vulgaris. Plant Ecology and Diversity 3, 289-299.
| Crossref | Google Scholar |
Davies GM, Legg CJ, Smith AA, MacDonald A (2019) Development and participatory evaluation of fireline intensity and flame property models for managed burns on Calluna-dominated heathlands. Fire Ecology 15, 30.
| Crossref | Google Scholar |
Dickman LT, Jonko AK, Linn RR, Altintas I, Atchley AL, Bär A, Collins AD, Dupuy J-L, Gallagher MR, Hiers JK, Hoffman CM, Hood SM, Hurteau MD, Jolly WM, Josephson A, Loudermilk EL, Ma W, Michaletz ST, Nolan RH, O’Brien JJ, Parsons RA, Partelli-Feltrin R, Pimont F, Resco de Dios V, Restaino J, Robbins ZJ, Sartor KA, Schultz-Fellenz E, Serbin SP, Sevanto S, Shuman JK, Sieg CH, Skowronski NS, Weise DR, Wright M, Xu C, Yebra M, Younes N (2023) Integrating plant physiology into simulation of fire behavior and effects. New Phytologist 238, 952-970.
| Crossref | Google Scholar | PubMed |
Doerr SH, Santín C (2016) Global trends in wildfire and its impacts: perceptions versus realities in a changing world. Philosophical Transactions of the Royal Society. Series B, Biological Sciences 371, 20150345.
| Crossref | Google Scholar | PubMed |
Fernandes PM, Catchpole WR, Rego FC (2000) Shrubland fire behaviour modelling with microplot data. Canadian Journal of Forest Research 30, 889-899.
| Crossref | Google Scholar |
Finney MA (1998) FARSITE: Fire Area Simulator-model development and evaluation. Res Pap RMRS-RP-4, Revised 2004. p. 4. (US Department of Agriculture, Forest Service, Rocky Mountain Research Station 47, Ogden, UT) 10.2737/RMRS-RP-4
Finney MA (2006) An Overview of FlamMap Fire Modeling Capabilities. In ‘2006 Fuels Management - How to Measure Success: Conference Proceedings’, 28–30 March 2006, Portland, OR. Proceedings RMRS-P-41. (PL Andrews, BW Butler, comps) pp. 213–220. (US Department of Agriculture, Forest Service, Rocky Mountain Research Station, Fort Collins, CO) Available at https://www.fs.usda.gov/research/treesearch/25948
Fosberg MA, Deeming JE, Forest RM, Range Experiment Station (Fort Collins Colo) (1971) ‘Derivation of the 1- and 10-hour Timelag Fuel Moisture Calculations for Fire-danger Rating.’ (Rocky Mountain Forest and Range Experiment Station, Forest Service, US Department of Agriculture) Available at https://books.google.no/books?id=li3DwgEACAAJ
Fox J, Weisberg S (2019) An R Companion to Applied Regression. Available at https://socialsciences.mcmaster.ca/jfox/Books/Companion/
Gimingham CH (1988) A reappraisal of cyclical processes in Calluna heath. Vegetatio 77, 61-64.
| Crossref | Google Scholar |
Glaves DJ, Crowle AJ, Bruemmer C, Lenaghan SA (2020) ‘The causes and prevention of wildfire on heathlands and peatlands in England (NEER014).’ (Peterborough) Available at https://publications.naturalengland.org.uk/publication/4741162353295360
Harrell Jr FE (2022) Hmisc: Harrell Miscellaneous. Available at https://CRAN.R-project.org/package=Hmisc
Jolly WM (2007) Sensitivity of a surface fire spread model and associated fire behaviour fuel models to changes in live fuel moisture. International Journal of Wildland Fire 16, 503-509.
| Crossref | Google Scholar |
Jolly WM, Johnson DM (2018) Pyro-ecophysiology: shifting the paradigm of live wildland fuel research. Fire 1, 8.
| Crossref | Google Scholar |
Kayll AJ, Gimingham CH (1965) Vegetative regeneration of Calluna vulgaris after fire. Journal of Ecology 53, 729-734.
| Crossref | Google Scholar |
Kirkland M, Atkinson PW, Pearce-Higgins JW, de Jong MC, Dowling TPF, Grummo D, Critchley M, Ashton-Butt A (2023) Landscape fires disproportionally affect high conservation value temperate peatlands, meadows, and deciduous forests, but only under low moisture conditions. Science of The Total Environment 884, 163849.
| Crossref | Google Scholar | PubMed |
Little K, Graham LJ, Kettridge N (2023) Accounting for among-sampler variability improves confidence in fuel moisture content field measurements. International Journal of Wildland Fire 33, WF23078.
| Crossref | Google Scholar |
Little K, Graham LJ, Flannigan M, Belcher CM, Kettridge N (2024) Landscape controls on fuel moisture variability in fire-prone heathland and peatland landscapes. Fire Ecology 20, 14.
| Crossref | Google Scholar |
Log T (2020) Modeling drying of degenerated Calluna vulgaris for wildfire and prescribed burning risk assessment. Forests 11, 759.
| Crossref | Google Scholar |
Log T, Thuestad G, Velle LG, Khattri SK, Kleppe G (2017) Unmanaged heathland – A fire risk in subzero temperatures? Fire Safety Journal 90, 62-71.
| Crossref | Google Scholar |
Madrigal J, Guijarro M, Hernando C, Díez C, Marino E (2010) Effective Heat of Combustion for Flaming Combustion of Mediterranean Forest Fuels. Fire Technology 47(2), 461-474.
| Crossref | Google Scholar |
McLauchlan KK, Higuera PE, Miesel J, Rogers BM, Schweitzer J, Shuman JK, Tepley AJ, Varner JM, Veblen TT, Adalsteinsson SA, Balch JK, Baker P, Batllori E, Bigio E, Brando P, Cattau M, Chipman ML, Coen J, Crandall R, Daniels L, Enright N, Gross WS, Harvey BJ, Hatten JA, Hermann S, Hewitt RE, Kobziar LN, Landesmann JB, Loranty MM, Maezumi SY, Mearns L, Moritz M, Myers JA, Pausas JG, Pellegrini AFA, Platt WJ, Roozeboom J, Safford H, Santos F, Scheller RM, Sherriff RL, Smith KG, Smith MD, Watts AC (2020) Fire as a fundamental ecological process: research advances and frontiers. Journal of Ecology 108, 2047-2069.
| Crossref | Google Scholar |
Mickler RA (2021) Carbon emissions from a temperate coastal peatland wildfire: contributions from natural plant communities and organic soils. Carbon Balance and Management 16, 26.
| Crossref | Google Scholar | PubMed |
Minsavage-Davis CD, Davies GM (2022) Evaluating the performance of fire rate of spread models in northern-European Calluna vulgaris heathlands. Fire 5, 46.
| Crossref | Google Scholar |
Monedero S, Ramirez J, Cardil A (2019) Predicting fire spread and behaviour on the fireline. Wildfire analyst pocket: a mobile app for wildland fire prediction. Ecological Modelling 392, 103-107.
| Crossref | Google Scholar |
Nolan RH, Boer MM, Resco De Dios V, Caccamo G, Bradstock RA (2016) Large-scale, dynamic transformations in fuel moisture drive wildfire activity across southeastern Australia. Geophysical Research Letters 43, 4229-4238.
| Crossref | Google Scholar |
NWCG (2022) NWCG Incident Response Pocket Guide (IRPG) | NWCG. Available at https://www.nwcg.gov/publications/461
NWCG (2023) Dead Fuel Moisture Content. Available at https://www.nwcg.gov/publications/pms437/fuel-moisture/dead-fuel-moisture-content
Pandey P, Huidobro G, Lopes LF, Ganteaume A, Ascoli D, Colaco C, Xanthopoulos G, Giannaropoulos T, Gazzard R, Boustras G, Steelman T, Charlton V, Ferguson E, Kirschner J, Little K, Stoof C, Nikolakis W, Fernández-Blanco CR, Ribotta C, Lambrechts H, Fernandez M, Dossi S (2023) A global outlook on increasing wildfire risk: current policy situation and future pathways. Trees, Forests and People 100431.
| Crossref | Google Scholar |
Pepin A-C, Wotton M (2020) Fire behaviour observation in shrublands in Nova Scotia, Canada and assessment of aids to operational fire behaviour prediction. Fire 3, 34.
| Crossref | Google Scholar |
Perry MC, Vanvyve E, Betts RA, Palin EJ (2022) Past and future trends in fire weather for the UK. Natural Hazards and Earth System Sciences 22, 559-575.
| Crossref | Google Scholar |
Pimont F, Ruffault J, Martin-StPaul NK, Dupuy J-L (2019) Why is the effect of live fuel moisture content on fire rate of spread underestimated in field experiments in shrublands? International Journal of Wildland Fire 28, 127-137.
| Crossref | Google Scholar |
R Core Team (2022) ‘R: A Language and Environment for Statistical Computing.’ (R Foundation for Statistical Computing: Vienna, Austria) Available at https://www.R-project.org/
Rothermel RC (1972) A mathematical model for predicting fire spread in wildland fuels. Res Pap INT-115. p. 115. (US Department of Agriculture, Intermountain Forest and Range Experiment Station 40, Ogden, UT) Available at http://www.fs.usda.gov/treesearch/pubs/32533
Santana VM, Marrs RH (2014) Flammability properties of British heathland and moorland vegetation: models for predicting fire ignition. Journal of Environmental Management 139, 88-96.
| Crossref | Google Scholar | PubMed |
Scott JH, Burgan RE (2005) Standard fire behavior fuel models: A comprehensive set for use with Rothermel’s surface fire spread model. USDA Forest Service - General Technical Report RMRS-GTR 1–76. 10.2737/RMRS-GTR-153
Shuman JK, Balch JK, Barnes RT, Higuera PE, Roos CI, Schwilk DW, Stavros EN, Banerjee T, Bela MM, Bendix J, Bertolino S, Bililign S, Bladon KD, Brando P, Breidenthal RE, Buma B, Calhoun D, Carvalho LMV, Cattau ME, Cawley KM, Chandra S, Chipman ML, Cobian-Iñiguez J, Conlisk E, Coop JD, Cullen A, Davis KT, Dayalu A, De Sales F, Dolman M, Ellsworth LM, Franklin S, Guiterman CH, Hamilton M, Hanan EJ, Hansen WD, Hantson S, Harvey BJ, Holz A, Huang T, Hurteau MD, Ilangakoon NT, Jennings M, Jones C, Klimaszewski-Patterson A, Kobziar LN, Kominoski J, Kosovic B, Krawchuk MA, Laris P, Leonard J, Loria-Salazar SM, Lucash M, Mahmoud H, Margolis E, Maxwell T, McCarty JL, McWethy DB, Meyer RS, Miesel JR, Moser WK, Nagy RC, Niyogi D, Palmer HM, Pellegrini A, Poulter B, Robertson K, Rocha AV, Sadegh M, Santos F, Scordo F, Sexton JO, Sharma AS, Smith AMS, Soja AJ, Still C, Swetnam T, Syphard AD, Tingley MW, Tohidi A, Trugman AT, Turetsky M, Varner JM, Wang Y, Whitman T, Yelenik S, Zhang X (2022) Reimagine fire science for the anthropocene. PNAS Nexus 1, pgac115.
| Crossref | Google Scholar | PubMed |
Simmons IG (1990) The mid-Holocene ecological history of the moorlands of England and Wales and its relevance for conservation. Environmental Conservation 17, 61-69.
| Crossref | Google Scholar |
Stoof CR, Tapia VM, Marcotte AL, Stoorvogel JJ, Ribau MC (2020) Relatie tussen natuurbeheer en brandveiligheid in de Deurnese Peel: onderzoek naar aanleiding van de brand in de Deurnese Peel van 20 april 2020. PE&RC, Soil Geography and Landscape. 10.18174/533574