Vegetation phenology as a key driver for fire occurrence in the UK and comparable humid temperate regions
Tadas Nikonovas
A
B
C
D
E
F
Abstract
Fire activity in the UK and comparable regions of northwest Europe is generally out of phase with peak fire weather conditions.
Here, we assess the potential effect of phenology on fire occurrence patterns for the UK.
We examined fire occurrence and vegetation phenology in the UK for 2012–2023, mapped onto the main fire-affected vegetation cover types within distinct precipitation regions, allowing the fire occurrence for fuels in different phenological phases to be explored across distinct ‘fuel’ types and regions.
The UK’s fire regime is characterised by burning in semi-natural grasslands and evergreen dwarf shrub ecosystems in early spring when vegetation is still dormant. During the high-greenness phase in late spring and summer, fire activity is reduced by a factor of 5–6 despite typically elevated fire weather conditions within that period.
Semi-natural vegetation in the UK is very resistant to burning during the high-greenness phase. However, this ‘fire barrier’ is diminished during severe drought episodes, which are predicted to become more extreme in the coming decades. Incorporating phenology information into models therefore has great potential for improving future fire danger and behaviour predictions in the UK and comparable humid temperate regions.
Keywords: active fire detections, flammability, humid temperate regions, land cover, phenology, Suomi-NPP, vegetation fuels, VIIRS, wildfire regimes.
Introduction
Vegetation fires are a growing concern in many regions of our changing planet (Jones et al. 2022; Santín et al. 2023), with temperate north-west Europe, including the United Kingdom (UK) being no exception (Arnell et al. 2021; Perry et al. 2022). The occurrence and spread of fires within the semi-natural temperate grasslands, heathlands and moorlands of this region are governed by the interplay among human-induced ignitions, prevailing weather conditions and fuel characteristics. The same vegetation fuels can amplify or dampen fire ignition risk and spread depending on the seasonally variable state of the ecosystems (Wotton and Beverly 2007; Davies et al. 2010; Cruz et al. 2015; Kidnie et al. 2015; Jolly et al. 2016; Sjöström and Granström 2023). The state of vegetation structure and physiology can be the primary determinants of fire occurrence and behaviour, especially when fire weather – that is, the meteorological conditions conducive to fire – is not extreme (Cruz et al. 2022). Research focused on relationships between fire weather and fire occurrence in temperate grassland and shrub fuels shows that the critical weather thresholds associated with elevated fire activity may vary depending on type of fuel and time of year (Beverly and Wotton 2007; Davies and Legg 2016; de Jong et al. 2016). These findings underscore the necessity of incorporating vegetation physiology into fire prediction systems (Dickman et al. 2023).
Weather conditions impact the likelihood of ignition, fire spread and intensity of wildfires once initiated. Antecedent air temperature, relative humidity, wind speed and precipitation control how much water vegetation fuels contain, thereby influencing their flammability, i.e. the ease with which vegetation fuels ignite and support combustion at any given time. Weather conditions during a fire, particularly wind speed, also directly determine fire behaviour, such as flame length and rate of spread. Fire danger prediction systems commonly integrate these meteorological parameters into fire weather indices to determine whether conditions are conductive for fire occurrence. In the UK, the Met Office Fire Severity Index forecasting system for England and Wales (MOFSI, Met Office 2023) is derived from the Canadian Forest Fire Weather Index System (FWI) (Van Wagner 1987). The FWI System processes meteorological inputs into six components: three codes representing moisture content across different depths of forest ground fuels, and three indices indicative of potential fire behaviour. Although fire weather is inextricably linked to both fire occurrence (Abatzoglou et al. 2018; Grillakis et al. 2022) and fire behaviour (Sedano and Randerson 2014; Kidnie and Wotton 2015), predictions solely based on fire weather, without consideration of information about vegetation physiology, do not perform well for temperate fuels (Beverly and Wotton 2007; Davies and Legg 2016; de Jong et al. 2016).
Vegetation in temperate regions undergoes substantial structural and physiological changes across seasons in response to changing environmental drivers (Jolly and Johnson 2018). This rhythmic annual change, also known as the phenological cycle, encompasses the spring-time emergence of foliage (‘green-up’), more vigorous growth of vegetation into the summer months, followed by die-back or senescence in autumn, and dormancy state throughout the coldest months. During the cycle, both deciduous and evergreen plants experience changes in water (Van Wagner 1977, Macias Fauria et al. 2011) and carbon (Jolly et al. 2016) content in live fuels and in the relative proportions of live and dead vegetation (i.e. degree of curing in grass fuels) (Cruz et al. 2015; Sjöström and Granström 2023), which significantly affects flammability of the host ecosystems (Dickman et al. 2023).
In the UK, most wildfires in semi-natural temperate grassland and heather-dominated ecosystems occur during March and April (de Jong et al. 2016; Forestry Commission 2023). This early spring fire activity window coincides with the onset of fire-favourable weather and when vegetation is still in its dormant phase and is flammable. During this time, an abundance of the previous season’s dead leaf litter in grasslands (Sjöström and Granström 2023) and low moisture content in live shrub fuels after the cold months (Davies et al. 2010) render ignitions and fire spread more likely after a period of dry weather. However, ground fuels often remain water-saturated following winter precipitation, with fires spreading predominately through surface fine litter and/or shrub canopies (Davies et al. 2009). The transition from dormancy to green-up has a large effect on flammability in these ecosystems, and fire activity is often reduced, despite increasing fire weather forcing during the summer months. Flammability of decomposing dead leaf diminishes over time (Weir and Limb 2013), while the proportion of live fuels in grasslands and moisture content in live shrub fuels increases and often exceeds flaming ignition sustainability thresholds, effectively serving as an ‘on/off’ fire switch (Weise et al. 2005; Davies and Legg 2011; Pimont et al. 2019; Sjöström and Granström 2023). Live vegetation is also more resilient to weather stress and can maintain water content during dry spells (Viegas et al. 2001).
Summer wildfires, although less frequent than those in spring across much of the UK and other temperate, humid Atlantic regions, possess the capacity to evolve into severe incidents, inflicting prolonged detrimental effects on the ecosystems (Maltby et al. 1990; Albertson et al. 2009; Davies et al. 2013). This heightened risk is attributed to the elevated fire weather conditions prevalent at that time of year, coupled with a reduced water content in ground fuels, including carbon-rich peat, which may smoulder for weeks or months once ignited (Rein et al. 2008). Climate model projections indicate a rise in the occurrence of extreme fire weather conditions across the UK, particularly during the summer months in the south and east of the country (Arnell et al. 2021; Jones et al. 2022; Perry et al. 2022). Consequently, a comprehensive understanding of phenological fire controls and their thresholds, which are anticipated to be increasingly surpassed in forthcoming decades, is imperative. Previous studies focusing on relationships between fire weather indicators and fire activity in the UK have found that specific components of the FWI System, notably the short-term indicators such as the fine fuel moisture code (FFMC) and initial spread index (ISI), have potential for predicting fire occurrence (Davies and Legg 2016; de Jong et al. 2016). Nevertheless, these studies also suggest that (i) the predictive skill of the FWI System components and the critical fire weather thresholds for fire occurrence are subject to variability according to season, land cover and geographic location within the UK; and (ii) predictions based solely on weather and climate data could benefit from the incorporation of phenology information. Despite growing knowledge of the dynamic nature of live fuels and the effect that vegetation physiology has on flammability (Davies et al. 2010; Cruz et al. 2015; Parisien et al. 2023), current fire severity prediction systems in the UK, such as MOFSI, do not integrate vegetation phenology. Efforts to implement phenology-driven fire danger assessments have demonstrated potential value of such an approach for temperate grass fuels (Sjöström and Granström 2023). However, it remains unclear whether similar phenological fire controls are applicable across a wider range of temperate fuels. Presently, there is a paucity of empirical evidence regarding the timing of phenological shifts and their impact on fire occurrence rates across the UK. This study addresses these gaps by (i) presenting an overview of phenological transition dates for land covers that are responsible for the majority of wildfires in the UK; and (ii) providing estimates of daily satellite fire detection rates for different vegetation phenological phases across the UK during the past decade (2012–2023).
Methods and data
Our fire occurrence and vegetation phenology analyses are based on a decade-long record from the Visible Infrared Imaging Radiometer Suite (VIIRS) sensor onboard the Suomi National Polar-orbiting Partnership (Suomi-NPP) satellite (2012–2023). We initially determined phenological transition dates and estimated the periods when UK vegetation was in different phenological phases. Then, we integrated our phenological phase estimates with active fire detections data to determine daily fire occurrence rates for the fuels during different phenological phases. The estimates target eight land cover types as defined by the UK Centre for Ecology & Hydrology (UKCEH) classification (Morton et al. 2020a, 2020b), segregated into nine distinct regions (Fig. 1) representative of the Met Office Hadley Centre coherent UK precipitation zones (Gregory et al. 1991; Alexander and Jones 2000). Finally, we generated seasonal profiles of fire weather indices and fuel moisture codes of the Canadian Forest Fire System to compare and contrast them with the fire activity record. Detailed information regarding the datasets and processing steps is provided in the sections bellow.
Distribution of UKCEH land covers in the UK and meteo-geographical regions of the study. Area plots show monthly VIIRS active fire detection counts for the regions and land cover types during the 2012–2023 period. The bottom right panel shows estimates combined for all regions.
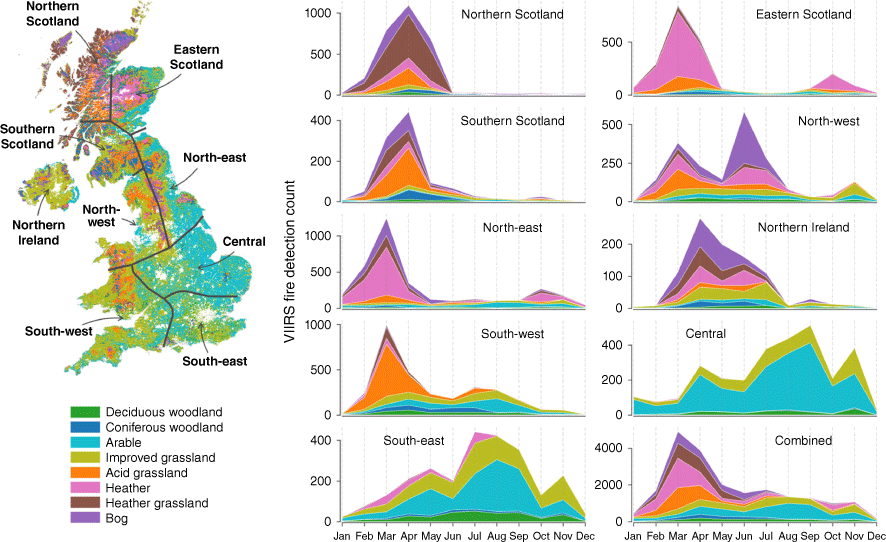
Vegetation fire activity record
The active fire detections dataset (VPN14MGML) (Schroeder et al. 2014) is derived from imagery collected by the VIIRS sensor and provides the central coordinates of sensor pixels (375 m at nadir, 450 m average size across the swath) flagged as containing an active fire. The satellite passes over at least twice every 24 h at approximately 1:30 am and 1:30 pm local solar time. At UK latitudes, some areas are observed more frequently owing to orbit overlap. The minimum detectable fire size depends on apparent fire temperature and background conditions. For instance, the algorithm has an estimated 50% probability of detecting ~700°C temperature fires as small as ~40 and <10 m2 during the daytime and night-time overpasses, respectively (Schroeder et al. 2014). Such detection thresholds indicate that the within-pixel area covered by active combustion may be many times smaller than the ~200,000 m2 pixel area, and as a result exact active fire location is uncertain within the pixel.
The VNP14IMGML record, available since 2012, determines the temporal span of our study (2012–2023). Although alternative satellite-based fire-detection products, such as those based on MODIS (Moderate Resolution Imaging Spectroradiometer) sensor data, offer longer historical records, the VIIRS dataset is better suited for characterising fire dynamics in the UK owing to its higher spatial resolution and enhanced ability to detect smaller fires.
To distinguish vegetation fires from other, non-vegetation heat sources, we clustered individual VIIRS fire detections into fire events by grouping any fire detections that were located within a 3-km radius and occurring within 5-day window into single objects. These spatial and temporal proximity thresholds were selected empirically after trying a range of combinations. Given that fire ignitions in the UK are fairly rare, the clustering algorithm was not sensitive to chosen threshold values, and the result event sizes were stable across a range of proximity values.
In the subsequent analysis, we selected only fire events in vegetated areas, thus filtering out fires that occurred primarily in non-vegetated land covers. To do this, we first identified land cover type, as described in the next section, for each of the active fire detections within a given fire event. Only fire events with more than half of fire detections characterised as vegetation fire detections were included for further analysis. This step reduced the total count of 2012–2023 UK fire detections from 87,000 to 23,000, eliminating detections that were part of fire events that were predominantly active over built-up areas (frequent detections attributable to industrial activity) and other non-vegetation surfaces such as bare land and water. Throughout this work, we use the term ‘vegetation fire detections’ to refer to VIIRS active fire pixels that were identified as vegetation fires by the method outlined above.
Land cover estimates
Vegetation fuel types were classified based on the UKCEH Land Cover Map raster dataset for the year 2018 (Morton et al. 2020a, 2020b). The product is based on Sentinel-2 sensor data and has a spatial resolution of 20 m. An equivalent dataset was not available for all years within the study’s timeframe and so we used the 2018 dataset to provide a best estimate of the land cover at the midpoint of the study period. The UKCEH land cover classes are based on the UK Biodiversity Action Plan (BAP) Broad Habitats classification system (Jackson 2000). Although not specifically fuels-orientated, the UKCEH land covers include categories representative of the main fire-bearing vegetation types in the UK.
This study focuses on eight key UKCEH land covers that collectively account for over 95% of all vegetation fire detections. Four of the categories represent semi-natural vegetation often found in remote areas, particularly in uplands. The semi-natural land covers are dominated by deciduous grasses on acid soils (acid grasslands), a combination of evergreen heath family shrubs mixed with gorse (heather land cover), a mixture of grasses and dwarf shrub species on well-drained soils (heather grasslands) or mixed grass- and dwarf shrub-dominated vegetation on deep, carbon-rich and waterlogged soils (bog). Two categories represent agricultural land, primarily featuring various crops (arable land) and grasslands that are intensively grazed and/or mowed (improved grasslands). The remaining two categories are characterised by primarily plantations of Sitka spruce, Scots pine, larch and other conifer trees (coniferous woodlands) or predominantly semi-natural stands of oak, birch, beech and other broadleaved tree species (deciduous woodlands) (see Supplementary Table S1 for further detail).
To generate land cover and region-specific seasonal profiles of satellite-based vegetation greenness and phenological phase changes, we selected land areas representing homogeneous land covers from the UKCEH dataset. This was achieved by performing binary erosion using a five-pixel window for each of the land cover classes in the UKCEH dataset. Binary erosion is a morphological operation that shrinks objects (pixels of a given land cover in this case) inwards from the edges and makes holes larger in proportion to size of the chosen eroding structure. After this processing step, only pixels that were located at least 100 m inside a homogeneous land cover patch were used in further analysis. This was done to adjust for the significant difference in spatial resolutions between the Sentinel-based UKCEH dataset (20 m) and the VIIRS sensor-based vegetation dataset (~450 m). From the remaining homogeneous areas, we randomly sampled 10,000 pixels to represent each land cover within each region of this study.
Fire weather indices and fuel moisture codes
We used daily historical fire weather indicators from the Canadian Forest FWI System (Van Wagner 1987) to derive seasonal fire weather variability in the study regions during the 2012–2023 period. Individual FWI System components were obtained from the Copernicus Emergency Management Service Fire danger indices dataset (Vitolo et al. 2020) generated by the Global European Centre for Medium-range Weather Forecasting (ECMWF) Fire Forecast (GEFF) model. The GEFF FWI indices are based on meteorological parameters (air temperature, precipitation, wind speed and relative humidity) taken ECMWF’s historical global climate reanalysis (ERA5) dataset, produced by the Copernicus Climate Change Service (C3S), and provided with a daily frequency at a 25-km spatial resolution.
The FWI System includes three fuel moisture codes and three fire behaviour indices. We focused on two fuel moisture codes, the FFMC and Duff Moisture Code (DMC), and two fire behaviour indices, the ISI and Buildup Index (BUI). The FFMC represents dead fuel moisture content in fast-drying fine fuels (i.e. cured grass, leaves and small twigs), whereas the DMC was designed to gauge moisture content in organic material under the forest floor litter, but can also offer insights into moisture stress in live fuels (Ruffault et al. 2018). The ISI represents potential rate of fire spread. It is a function of FFMC and wind speed. Consequently, ISI fluctuates rapidly with weather. The BUI is designed to represent the potential energy release from larger fuels, serving as an indicator of potential drought-driven fire intensity (and thus, severity) during the growing season (Van Wagner 1987).
We generated seasonal profiles of the FWI System codes and indices spanning 2012 through to 2022 inclusive, by calculating percentiles of the values pooled per day of year and region. To emphasise seasonal trends, we removed day-to-day variability by smoothing the seasonal profiles of the FWI System components using a 7-day moving average window.
Enhanced vegetation index
The two-band Enhanced Vegetation Index 2 (EVI2) product VPN13A1 (Didan and Barreto 2018) from VIIRS sensor was employed as a proxy for assessing vegetation relative greenness and health. The product provides best available EVI2 estimates every 16 days at a 500-m spatial resolution. In contrast to the standard EVI, EVI2 is based on near-infrared and red bands, omitting the blue wavelengths used in EVI (Jiang et al. 2008). This allows the algorithm sensors that do not have blue bands for vegetation monitoring. EVI2 is expected to become the standard vegetation index for VIIRS and other sensors in the future (Didan and Barreto 2018). EVI2, as with other vegetation greenness-based indices, is sensitive to vegetation photosynthetic activity, and is widely used for tracking vegetation dynamics, including phenological phase transitions (Zhang et al. 2018).
The seasonal profiles of the vegetation in our study were derived from EVI2 data in two steps. First, we identified nearest neighbour pixels in the VPN13A1 product to those pixels previously selected as representing homogeneous land cover areas (described in the Land cover estimates section). Following this, we computed EVI2 percentile values for day-of-year pooling retrievals spanning all years between 2012 to 2022.
Phenological dates
The determination of phenological transition dates for the vegetation fuels and UK regions was based on the yearly global land surface phenology metrics dataset VNP22Q2, which is also generated from the VIIRS EVI2 retrievals. The dataset captures key phenological transition dates for up to two phenological cycles per year, although the vegetation land covers in this study exhibited only one cycle each year. The VNP22Q2 product has 500-m spatial and annual temporal resolutions and has been validated against ground-based and higher-resolution satellite-based phenology observations, showing strong agreement (Zhang et al. 2018).
Utilising the VNP22Q2 product estimates of dates for the onset of greenness increase, onset of greenness maximum, onset of greenness decrease and onset of greenness minimum phases, we were able to estimate the phenological phase of fuels at different times of year. We considered that the period between the dates of onset of greenness minimum and onset of greenness increase as the winter dormancy phase, whereas onset of greenness increase date marked the transition to the spring green-up phase (e.g. emergence of new foliage and stem growth). The onset of greenness maximum was indicative of plants reaching their maturity phase, while the onset of greenness decrease marked the onset of the plant senescence phase (e.g. discolouration and loss of deciduous foliage). The VNP22Q2 product also estimates the middle of greenness increase and the middle of greenness decrease dates, which we used to split green-up and senescence phases into early and late green-up or senescence phases, respectively. Transition dates for different land covers across the study regions were estimated by finding nearest pixels in the VNP22Q2 product to the location coordinates of the selected land cover pixels representing the study’s land covers and computing median transition date values.
Results and discussion
Fire activity in vegetated land cover types
The analysis of fire activity within vegetated land cover types in the UK reveals significant variability across different vegetation fuels and regions (Fig. 1). The eight land covers shown in Fig. 1 collectively covered ~86% of the entire UK area in 2018 and accounted for 95% of all vegetation fire detections during the study period, and thus the analysis is focused on these fuel types corresponding to these land cover classes.
Agricultural vegetation classes, specifically improved grasslands and arable land, covered the largest proportion of the UK’s land surface, accounting for 28 and 21% respectively. Heather, acid grasslands, heather grasslands and bog land covers constituted 4, 9, 6 and 4% respectively. Meanwhile, deciduous and coniferous woodlands covered 8 and 6% of the UK’s land area, respectively (Supplementary Fig. S1).
In terms of fire activity, semi-natural grassland and shrub land covers (heather, acid grasslands, heather grasslands and bogs) collectively accounted for more than half of the total fire detections, with respective contributions of 19, 13, 12 and 10%. Agricultural fires on arable land and improved grasslands accounted for an additional 21 and 15% of fire detections, respectively, whereas fire detections in deciduous and coniferous woodlands comprised only 6 and 4% of the total fire count. Semi-natural land covers also exhibited higher rates of fire detections per unit area compared with woodlands and agricultural land (Supplementary Fig. S2).
Fire detections in semi-natural land covers (acid grasslands, heather grasslands, heather and bog areas) exhibited a notable peak during March and April (Fig. 1). This pattern varied regionally; for example, fire activity in East Scotland, North-east and South-west regions peaked in March, whereas in Northern and Southern Scotland regions and Northern Ireland, the peak occurred in April.
Although fire activity in March was also high in the North-west region, the highest number of heather and grasslands fire detections here occurred in June. Similar increases in summertime fire activity were also observed in the South-west region and Northern Ireland; however, overall, such events were an exception rather than the norm (Fig. 1). In the Central and South-east regions, fire activity primarily occurred in agricultural land covers (arable land and improved grasslands) and deciduous woodlands. Such a distinct pattern reflects the different proportions of land covers between regions of the UK. Semi-natural grassland and heather-dominated land covers comprised <1% of the area in Central and South-east regions, whereas agricultural vegetation covered approximately 76 and 61% of their total area respectively (Supplementary Fig. S1). The temporal pattern of fire activity here was also different when compared with regions dominated by fires in semi-natural land covers, with peak burning observed from July through September and fire activity more evenly distributed across the seasons. The Central region recorded only a few fires recorded in non-agricultural land covers. The relatively small number of heather fires detected in South-east England displayed a peak in March and July, mirroring the temporal patterns observed in other dwarf shrub-dominated regions.
The timing of fire occurrence in the UK is governed by a range of factors. Natural ignitions by lightning are exceedingly rare. Instead, the vast majority of vegetation fires result from human actions – whether for land management purposes, through arson, or by accident (Gazzard et al. 2016). It is important to clarify that many early-season fires are associated with prescribed burns, which are part of regulated land management practices. Prescribed fires in the UK are primarily used to modify the structure and age of Calluna vulgaris stands on red grouse game shooting estates and to manage semi-natural pastures dominated by Molinia grass (Harper et al. 2018). Such fires are authorised from October or November until the end of March or April (depending on the region and site elevation) and do not generally pose a significant risk. This period of sanctioned burning very likely contributes substantially towards the early-season fire detection counts across most regions, and also may explain the observed spikes in fire activity within heather-dominated land covers during October in East Scotland and the North-east region (Fig. 1). The proportion of total fire detection counts of this study that are attributable to prescribed fires could not be determined from the datasets employed herein and should be the focus of more region-specific future studies. Wildfire statistics for England (Forestry Commission 2023) covering 2009–2021 show that area burned in semi-natural grasslands, mountain, heath and bog land covers peaks in April, rather than March as reported in this study. This temporal discrepancy is likely caused by prescribed fires that are recorded by VIIRS sensor (but are generally not reported by Fire and Rescue Services as wildfire incidents). However, fire incident records also indicate elevated burning activity in March across many regions of the UK (de Jong et al. 2016; Forestry Commission 2023), suggesting that the early-season fire activity peak reported in the present study cannot be solely attributed to managed burns. Accidental ignitions and arson fires also contribute to driving fire detection counts at this time of year.
The seasonal profiles of fuel moisture codes (Fig. 2) and fire behaviour indices (Supplementary Fig. S3) exhibit large inter- and intra-annual variability, highlighting considerable disparities in the timing of peak values among the codes themselves and between the different regions of the UK. The FFMC, which is sensitive to relative humidity, is more variable on a daily basis but changes less between seasons when compared with drought-driven DMC (Fig. 2). The ISI, driven by wind speed and FFMC also reaches maximum values much earlier in the year. In the Scottish regions, North-East and Northern Ireland, the FFMC is at or near peak values in April and May, whereas in the North-West, Central, South-West and South-East regions, it remains elevated from April through September. In contrast, the DMC typically peaks during the summer months across all regions and exhibits a much higher seasonal variability.
Seasonal variability of Fine Fuel Moisture Code (FFMC) and Duff Moisture Code (DMC) and daily fire detections in the study regions. Solid lines show upper quartile values (75th percentile), shaded regions envelope 50th and 99th percentiles of FFMC and DMC values for the 2012–2022 period. Grey bars show fire detection counts aggregated per day of year for the 2012–2023 period.
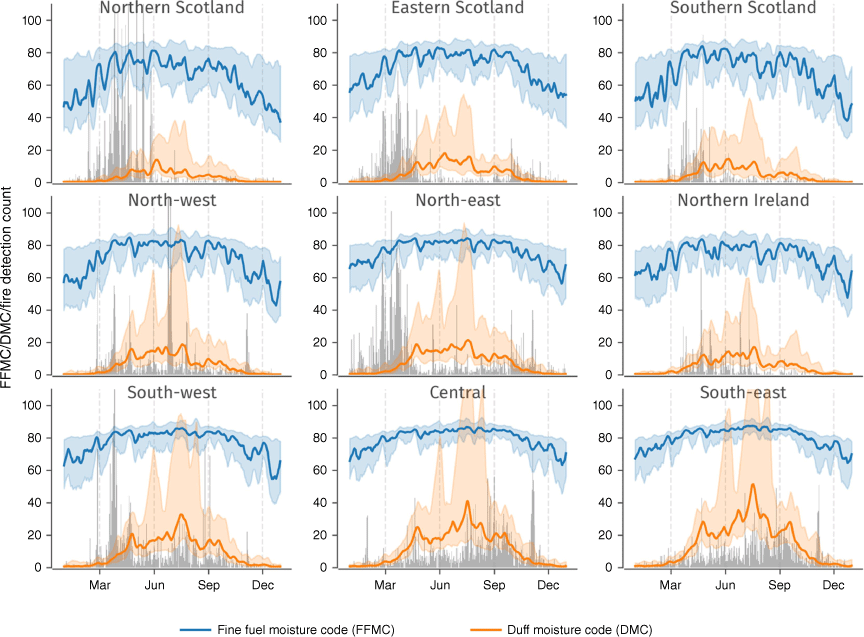
Fire weather is an important piece of the highly variable fire activity puzzle. However, the highest values of fuel moisture codes and fire weather indices are typically recorded in summer months (Fig. 2), when fire activity is generally low (Fig. 1). If fire weather was the dominant factor at this time of year, then it could be expected that favourable weather conditions coupled with accidental ignitions caused by increasing number of visitors to the UK’s open spaces in the summer months (Albertson et al. 2009) would be associated with significant increase in fire activity. The discrepancy between the seasonal patterns of peak fire activity and fire weather components illustrated in Figs 1 and 2 indicates that other factors, such as plant phenology, exert a profound effect on fire activity here.
Phenological climatology of UK fuels
The humid temperate ecosystems of the UK undergo significant changes in greenness over the course of a year. Although all land covers of this study experienced a single phenological cycle each year, the timing and amplitude of this cycle was distinct between the land covers and different regions (Figs 3 and 4). During the study’s period, the greatest variations in vegetation greenness according to EVI2 values were observed in agricultural land (arable and improved grasslands), acid grasslands and deciduous woodlands. In contrast, the phenological cycle’s amplitude was more subdued in heather land covers dominated by evergreen dwarf shrubs (i.e. Calluna vulgaris, Erica tetralix, Ulex minor) and conifer woodlands (Fig. 3). This finding aligns with expectations based on plant phenology. The annual shedding of leaves by deciduous trees and die-back in grasslands (Salim et al. 1988) were reflected by larger amplitude of EVI2 cycles when compared with land covers representing evergreen species. The seasonal profile of the vegetation index for heather grasslands, as expected, falls somewhere in between the curves recorded for acid grassland and heather land covers in terms of amplitude (Fig. 3), yet exhibits significant regional variability within the UK (Fig. 4). Bog land cover also represents mixed vegetation ecosystems, containing both evergreen dwarf shrub vegetation and deciduous purple moor-grass (Jackson 2000), and EVI2 seasonal amplitude of bog areas is more akin to heather than to heather grassland (Fig. 3).
EVI2 (vegetation greenness) seasonal cycle (solid line indicates median values, shaded intervals interquartile and 95–5 percentile ranges) for the land covers for the 2012–2022 period. Vertical dashed lines indicate median dates of onset of greenness increase, onset of greenness maximum, onset of greenness decrease and onset of greenness minimum from left to right respectively. Shaded vertical intervals show interquartile ranges of the phenological transition dates. Red bars show VIIRS Suomi-NPP vegetation fire detections for the land cover.
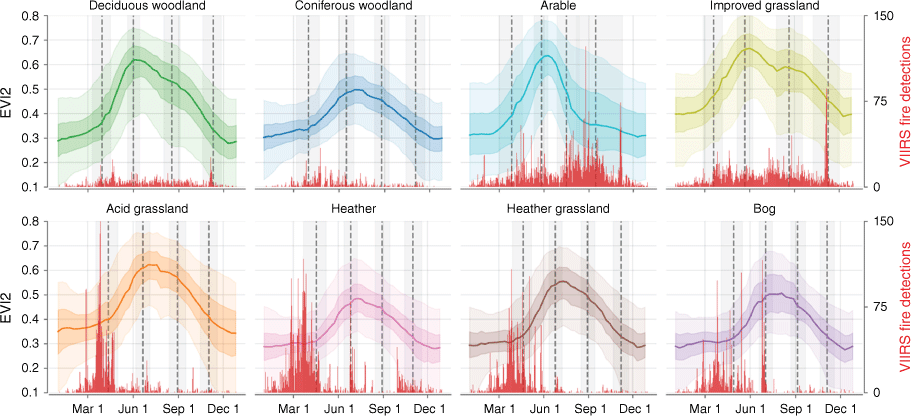
Middle day of year (DOY) of green-up phase, middle date of senescence phase, growing season length and maximum EVI2 value for different UK regions and land covers. Points indicate median values; error bars represent interquartile value ranges. The remaining land covers are shown in Supplementary Fig. S4.
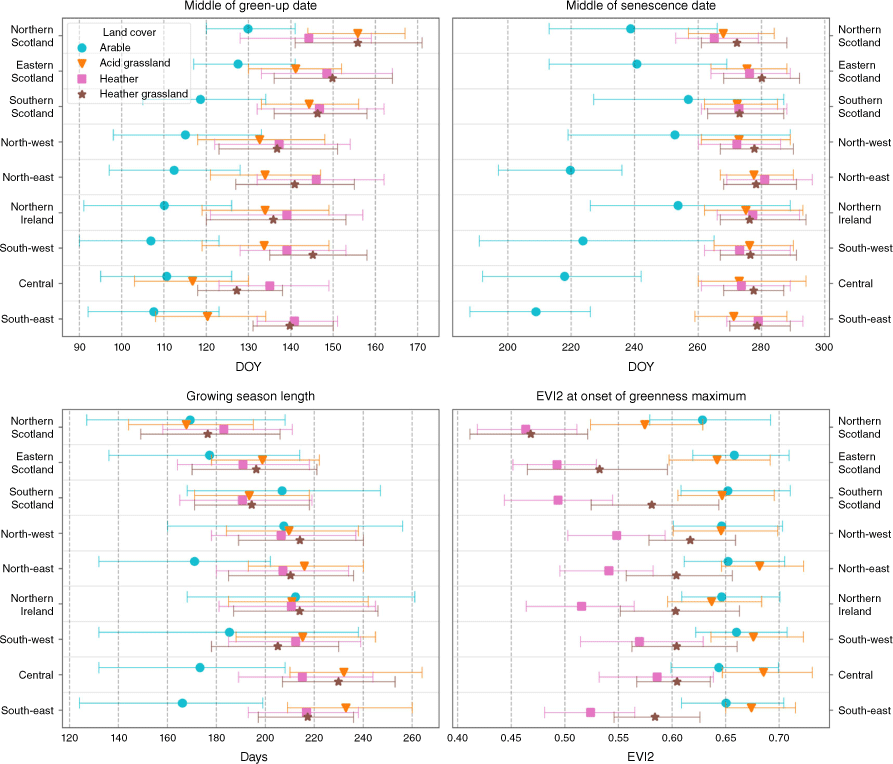
The estimated onset of the green-up phase differed between fuels and regions of the study (Fig. 4). Transition from dormancy to the growing phase occurred the earliest (by far) in agricultural land, whereas in semi-natural habitats, the greening was, on average, becoming apparent several weeks later. In Northern Scotland, heather land cover commenced green-up approximately 1–2 weeks after the South-west and South-east regions. The latitudinal disparity in green-up onset dates was most pronounced in acid grasslands, with up to a 1-month difference between Northern Scotland and South-east regions (Fig. 4). The north–south gradients in phenological timings and fire detections for acid grasslands of this study align well with fire activity studies of grass fuels in Sweden (Sjöström and Granström 2023). Notably, the phenological transition dates differ between the regions for the onset of greenness increase phase, but less so for the onset of the greenness decrease phase.
Transitions between phenological phases do not coincide with calendar seasons. This is a critical factor that emphasises the importance of a phenology-based framing when defining seasonally variable fuels in the UK. For example, the onset of greenness increase occurs sometime during calendar spring in most fuels across the UK; however, there are substantial differences when comparing regions and land covers (Fig. 4). The rapid increase in EVI2 observed during the green-up phase indicates that fuels change dramatically between March and May, and hence they cannot be characterised by a single broad umbrella term of ‘spring fuels.’ At the same time, grasslands in their phenological dormancy phase are similar between late winter and early spring in terms of degree of curing, and any increases in fire occurrence in early spring are likely to be driven by more favourable fire weather, rather than changes in the fuels themselves.
Although the estimated phenological transition dates and amplitudes of greening for different regions of the study broadly correspond with the earlier arrival of spring in lower latitudes, there are some notable irregularities. These are likely due to the lack of altitudinal stratification in this study, and from uncertainties in the phenological dates in the VNP22Q2 product, which are particularly high in regions with frequent snow and cloud cover (Zhang et al. 2018), such as the Scottish Highlands. Further work, for example, is needed to investigate phenological climatology patterns for lowland versus highland ecosystems, as altitudinal gradients can be of similar magnitude to the latitudinal differences in phenological dates presented in this study.
Phenology and fire occurrence
The analysis integrating fire detection data with phenological transition dates reveals that the majority of fires in the UK occur when host ecosystems are in their dormant, early green-up, or senescent phases (Fig. 5). This trend is particularly pronounced in semi-natural land covers dominated by grasslands and dwarf shrubs, and, to a lesser extent, in conifer woodlands. Over arable lands, fire activity also showed small differences across phenological phases. In contrast, fire detection rates in deciduous woodlands and improved grasslands did not differ nearly as much between the phenological seasons when compared with the semi-natural land covers.
Median rates of active fire detections per day for different phenological phases and land covers pooled over all regions and study years. Early green-up phase represents the period between onset of green-up and middle green-up dates, while detection rates for late green-up phase include active fire pixels between middle of green-up and onset of greenness maximum dates. Senescence phase is also split the same way, using the middle of senescence date (see Methods). Note that the rates were not normalised by land cover size. As a result, the rates should not be used for comparing different fuels.
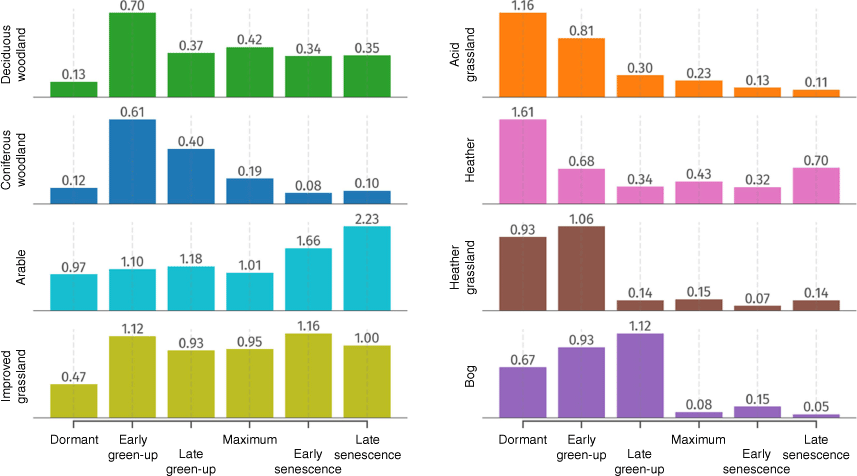
Fire activity in acid grasslands and heather grasslands exhibited large differences between the phenological phases during the study period, with fire detection rates five and six times higher during dormancy when compared with the phenological maximum phase respectively (Fig. 5). This is an expected result for deciduous vegetation with a low proportion of coarse fuels. A common example of these fuels is the deciduous perennial purple moor-grass (Molinia caerulea), which sheds leaves late in autumn and regrows during the spring months (Salim et al. 1988). During the dormant and early greening phases, grasslands contain a high load of dead blades and litter, which react rapidly to weather changes and are easily ignitable (Wotton 2009; Cruz et al. 2015; Kidnie and Wotton 2015). When grasslands transition to the growing phase and approach phenological maturity, these ecosystems become more resistant to ignition and fire spread (Sjöström and Granström 2023). While dead litter is still present, new growth live matter with high moisture content tends to supress ignition and fire spread.
Fire detection rates for the heather land cover areas were nearly four times higher in the dormant phase compared with the greenness maximum phase, whereas in bogs, this difference reached eightfold (Fig. 5). Notably, the highest fire detection rates for bog areas were observed during the late green-up phenological phase rather than dormancy or early green-up. Whereas the overall patterns resemble those of areas dominated by grass fuels, the underlying mechanisms are likely to be different. In contrast to acid grasslands, the ratio between live and dead fuels does not differ as much between dormant and high-greenness phases in evergreen dwarf shrub ecosystems that dominate heather and bog land covers. Although moisture content in dead fuels is an important factor controlling flammability in dwarf shrub ecosystems (Davies and Legg 2011; Santana and Marrs 2014), seasonal FFMC cycles shown in Fig. 2 indicate that variability in dead fuel moisture content does not explain the observed reduction in daily fire occurrence rates during the greenness maximum phase. Phenologically driven changes in moisture content in live fuels, however, offer a more plausible explanation for the large contrast in fire rates between the seasons (Davies et al. 2009, Davies and Legg 2011). In winter and early spring months, live heather fuels in the dormancy phase have a low moisture content when compared with the greenness maximum phase owing to cold weather-driven desiccation (Davies et al. 2010). Other changes in physiology and chemistry may play a role as well, and further field-based research is needed to understand factors affecting flammability and fire behaviour in dwarf shrub ecosystems (Vandvik et al. 2014).
In woodland and agricultural land covers, the contrast between fire detection rates observed during different phenological phases was more subtle when compared with grassland and dwarf shrub-dominated habitats. In coniferous and deciduous woodlands, fire activity peaked during the early green-up phase, when it was higher by a factor of three and 40% compared with estimates for greenness maximum phase respectively. In contrast to other vegetation fuels, daily fire detection rates over arable land peaked later in the season and were twice as high during the late senescence phase compared with other phases. Over improved grasslands, the differences between the phases were very small. We note that the absence of substantial differences in fire occurrence rates between the phases does not necessarily indicate an absence of phenological fire controls. If fire weather was the only factor governing fire occurrence, then we would expect to see elevated fire activity during the late increase in greenness and greenness maximum phenological phases when fire weather indicators are generally at their highest values (Fig. 2). However, this is not the case. The agricultural land covers of arable land and improved grasslands are intensively managed through crop rotation, harvesting and grazing, and thus fire activity is likely to be more strongly driven by human activity rather than climatic and phenology factors. In addition, Fig. 3 indicates that the phenological phase transition dates of the VNP22Q2 product may perform less well over agricultural land when compared with semi-natural grassland and areas dominated by dwarf shrubs. This is particularly noticeable over improved grasslands. Thus, fire detection rate estimates for different phenological phases may be less reliable here.
Overall, the date for the middle of green-up phase aligned best with the reduction in the rate of fire detections for both grassland- and dwarf shrub-dominated landscapes (Figs 5 and 6), indicating that this phenological transition could be utilised in fire risk prediction and for formulating seasonal fuel models. The high rates in bog land cover type observed during the late green-up phase do not contradict this pattern but require further scrutiny to explain.
Yearly VIIRS SNP active fire detection counts for high greenness phase (between mid-increase in greenness and mid-decrease in greenness dates) top bars, and low greenness phase (between mid-decrease in greenness and mid increase in greenness dates) bottom bars. Hatched area shows detections attributed to Saddleworth Moor and Winter Hill wildfires.
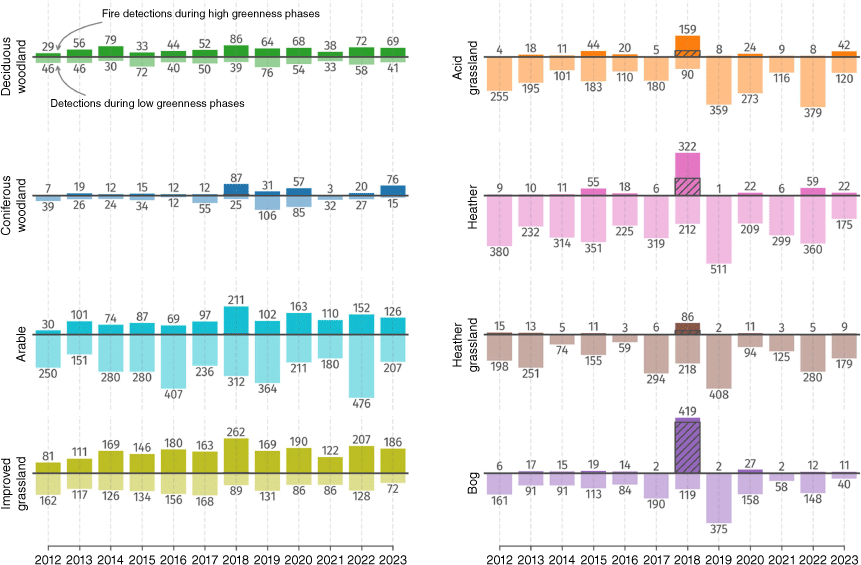
Drought and fire weather-driven fire activity
During the 2012–2023 period, there were several seasons characterised by unusually dry conditions and/or extreme fire weather. Fire detection counts for high and low vegetation greenness phases aggregated by year (Fig. 6) illustrate the stand-out nature of fire activity during the high-greenness phenological phase of 2018 in semi-natural land covers. The very dry summer of 2018 was associated with extreme fire activity in the UK (Forestry Commission 2023) and across north-west Europe (Kelly et al. 2021). Detections for this year comprised between approximately half and three quarters of total high-greenness phase active fire observations during the study period in grassland- and dwarf shrub-dominated ecosystems. Critically, this indicates that the differences in fire detection rate estimates between the phenological phases shown in Fig. 5 were, in fact, much greater during years when weather conditions were less than extreme.
A severe drought in 2018 caused widespread drying in peatlands across the UK and elsewhere in north-west Europe (Lees et al. 2021), and low water table depths (Lees et al. 2021), increasing the likelihood of combustion in deep peat soils. Indeed, a substantial fraction of the high greenness detections in 2018 were attributable to a few very large individual fires over peatlands. The Saddleworth Moor and Winter Hill wildfires were two such events that started in late June 2018 and smouldering fires in peat were active for several weeks. They were the largest and fourth largest UK events respectively in terms of VIIRS fire detection counts in the record at the time of writing, and collectively accounted for over 90% of total bog and over 30% of total heather fire detections during the high-greenness phase of that year (Fig. 6).
Although fire observation counts for the high-greenness phase in 2018 were also elevated over agricultural land and woodlands, the differences were smaller, when compared with estimates for the same phenological phase in other years, and interannual variability in fire detection counts was lower when compared with semi-natural ecosystems. Such a pattern suggests a higher and relatively constant number of ignitions in agricultural landscapes across the study years when compared with semi-natural land covers. Although fires on arable land and improved grasslands did not develop into very large events in terms of satellite detection counts even during the extreme fire weather seasons, some of the events that occurred during the high-greenness phase were nonetheless damaging and difficult to suppress. For example, multiple grassland fire events broke out on 19 July 2022, during the peak of an unprecedented heatwave in the UK (Yule et al. 2023). Exacerbated by high wind speeds, these grassland fires on the edge of built-up and densely populated areas caused unparalleled property damage in the UK context and put immense strain on Fire and Rescue Services.
The above results suggest that there are at least two distinct modes of fire activity in the UK. One activity peak primarily occurs in early spring when vegetation is still in dormancy or at an early stage of the growth phase, coinciding with more conductive fire weather conditions. During this period, peak ignitions and fire spread are driven by fuel factors: abundant dead grass fuels and low moisture content in live shrub fuels (Davies et al. 2010). Such ‘flashy’ fuels react rapidly and become easily flammable even under moderate fire weather conditions (Wotton 2009). Fire activity during this fire mode has been shown to correlate better with the fuel moisture and fire behaviour indicators that change rapidly and have a short-term memory, namely FFMC and ISI (de Jong et al., 2016; Davies and Legg 2016). Some fires during dormancy phase occur in autumn as well, but they are relatively rare in comparison with the early spring dormancy/transition to growing periods (Fig. 5).
The second mode of fire occurs when vegetation is approaching, or has reached, the phenological maturity stage, but is stressed by severe drought and extreme fire weather. In temperate north-west Europe, grasslands and shrublands are very resilient to fire during high-greenness phases owing to the high ratio of live-to-dead fuels and high moisture content in live fuels. This phenological fire control is particularly strong in ecosystems dominated by grasses. Experimental data from Swedish grasslands (Sjöström and Granström 2023) suggest that during the green-up phase, fire season is effectively over when live grass fraction exceeds 10–20% of the total fuel load. However, the normal functioning and phenological development of the ecosystems can be disrupted by persistent extreme fire weather, which drives live fuel moisture content below the combustion thresholds (Davies and Legg 2011; Cruz et al. 2015). Under such conditions, both above- and below-surface fuels are dry, and combustion of underground peat is possible, which can result in substantial emissions and loss of long-term landscape carbon stores. Fire occurrence during this activity mode has been shown to correlate with elevated levels of longer-term fire weather components that characterise moisture content in larger fuels (DMC) and potential release of energy from fuels (BUI) (de Jong et al. 2016).
Under current climatic conditions, fire regimes in the UK are dominated by fuel factors, whereas extreme drought- and fire weather-driven flare-ups in fire activity are rare. However, future climate projections indicate an increase in the frequency and severity of summer droughts across the UK, in particular for regions in the south of the country (Arnell et al. 2021). As a result, vegetation that is typically fire-resilient during the phenological maturity phase can be expected to become flammable more often owing to increased water stress. Indeed, drought-driven summer fire events may become the dominant mode of burning in the UK in the coming decades. This may already be the case for Central and South-east regions with the highest summer-time values of DMC (Fig. 2) and BUI (Supplementary Fig. S3). However, a longer record is needed to draw firmer conclusions. Transition to this mode of burning in UK regions further west and north could have profound implications for carbon emissions and the health of ecosystems. Furthermore, more frequent extreme summer fires have potential to exceed the resources of emergency responders and fire professionals. This was most recently seen during July 2022 when 14 Fire and Rescue Services across England declared major incidents due to wildfire during the record-breaking extreme heat.
Although this study focuses on fire regimes in the UK, analogous fire activity patterns are observed in other regions of north-west Europe with similar climates. An early season peak in fire activity has been documented in The Netherlands, Belgium, Ireland, Norway and Sweden (San-Miguel-Ayanz et al. 2022; Cardíl et al. 2023; Sjöström and Granström 2023), particularly in areas dominated by temperate grasslands and shrubs. Importantly, there is also evidence of a summertime surge in burning activity that is, like in the UK, driven by extreme drought conditions (Stoof et al. 2024). Further research is urgently needed to determine drought severity thresholds for different vegetation fuels and regions across the UK, and more widely across temperate north-west Europe, to enable updating, and integrating plant phenology into, operational fire danger and behaviour prediction systems.
Conclusion
Our analysis of active fire observations and vegetation phenological transition dates for the UK for the 2012–2023 period demonstrate that seasonal fire occurrence patterns in the semi-natural temperate grasslands and dwarf shrub-dominated ecosystems of the UK are generally out of phase with fire weather components. Large differences were found in fire occurrence between vegetation phenological phases, with fire activity being five to six times higher during dormant and early green-up phases, when compared with the late green-up and phenological maturity phases in the grassland- and dwarf shrub-dominated habitats that represent the most fire-prone land covers in the UK. The differences in fire occurrence rates were larger during years with less than extreme fire weather. A similar but less pronounced pattern was observed over the region’s woodlands. In contrast, on agricultural lands, fire detection rates were elevated during the senescence phenological phase, but with small differences between phases.
During the past decade, the UKs fire regime appears to have been strongly modulated by fuel phenology. The UK’s main fire-prone land covers remain highly resistant to burning when vegetation is in late green-up and phenological maturity phases, despite elevated fire weather forcing during the summer months. However, there was also evidence that the phenological fire barrier was diminished over large parts of the UK, particularly during the summer months of 2018. Fire activity flare-up during severe drought and heatwave episodes illustrates the switch from a fire regime dominated by fuels factors to a regime where fire occurrence and behaviour are driven by extreme fire weather.
Drought conditions conductive to burning during the high-greenness phenological phases are predicted to occur more often and cover larger parts of the UK, as well as other temperate Atlantic regions in the coming decades. As a result, severe summer-time fire events are likely to become more frequent owing to more extreme fire weather and a longer fire season, inevitably increasing pressure on emergency services and resource managers. These projections emphasise the importance and urgency of establishing phenological state indicators and vegetation stress thresholds for fuels in the region and utilising them, as well as fire weather variables, for predictions of fire occurrence and behaviour. Such a holistic approach could inform fire management strategies and help to mitigate the impact of vegetation fires in the UK and more widely in regions with similar humid temperate climates.
Data availability
All datasets used in the study are publicly available. The VIIRS Suomi-NPP active fire detection dataset VNP14IMGML is available at: https://firms.modaps.eosdis.nasa.gov/active_fire/. UK Centre for Ecology & Hydrology (UKCEH) land cover for 2018 is available at https://www.ceh.ac.uk/data/ukceh-land-cover-maps. VIIRS vegetation product VPN13A1, which includes EVI2, can be downloaded from: https://developers.google.com/earth-engine/datasets/catalog/NOAA_VIIRS_001_VNP13A1. The phenological dates dataset VNP22Q2 is available at: https://developers.google.com/earth-engine/datasets/catalog/NOAA_VIIRS_001_VNP22Q2. The Global ECMWF Fire Forecast (GEFF) model Copernicus Emergency Management Service Fire danger indices dataset is available at: https://cds.climate.copernicus.eu.
Conflicts of interest
Stefan H. Doerr is an Editor of International Journal of Wildland Fire. To mitigate this potential conflict of interest he had no editor-level access to this manuscript during the peer review. The authors declare no other conflicts of interest.
Declaration of funding
This study was supported by NERC grant UK-FDRS ‘Toward a UK fire danger rating system: understanding fuels, fire behaviour and impacts’ (NE/T003553/1) and the EC project FirEUrisk, which has received funding from the European Union’s Horizon 2020 research and innovation programme under grant agreement No. 101003890.
Author contributions
G. C., S. H. D., C. M. B., N. K., T. S.: funding acquisition. T. N., S. H. D.: conceptualisation. T. N., S. H. D: methodology. T. N.: visualisation. All co-authors: writing – original draft. All co-authors: writing – review and editing.
References
Abatzoglou JT, Williams AP, Boschetti L, Zubkova M, Kolden CA (2018) Global patterns of interannual climate–fire relationships. Global Change Biology 24, 5164-5175.
| Crossref | Google Scholar | PubMed |
Albertson K, Aylen J, Cavan G, McMorrow J (2009) Forecasting the outbreak of moorland wildfires in the English Peak District. Journal of Environmental Management 90(8), 2642-2651.
| Crossref | Google Scholar | PubMed |
Alexander LV, Jones PD (2000) Updated precipitation series for the UK and discussion of recent extremes. Atmospheric Science Letters 1(2), 142-150.
| Crossref | Google Scholar |
Arnell NW, Freeman A, Gazzard R (2021) The effect of climate change on indicators of fire danger in the UK. Environmental Research Letters 16(4), 044027.
| Crossref | Google Scholar |
Beverly JL, Wotton BM (2007) Modelling the probability of sustained flaming: predictive value of Fire Weather Index components compared with observations of site weather and fuel moisture conditions. International Journal of Wildland Fire 16, 161-173.
| Crossref | Google Scholar |
Cardíl A, Tapia VM, Monedero S, Quiñones T, Little K, Stoof CR, Ramirez J, de-Miguel S (2023) Characterizing the rate of spread of large wildfires in emerging fire environments of northwestern Europe using Visible Infrared Imaging Radiometer Suite active fire data. Natural Hazards and Earth System Sciences 23(1), 361-373.
| Crossref | Google Scholar |
Cruz MG, Gould JS, Kidnie S, Bessell R, Nichols D, Slijepcevic A (2015) Effects of curing on grassfires: II Effect of grass senescence on the rate of fire spread. International Journal of Wildland Fire 24, 838-848.
| Crossref | Google Scholar |
Cruz MG, Alexander ME, Fernandes PM (2022) Evidence for lack of a fuel effect on forest and shrubland fire rates of spread under elevated fire danger conditions: implications for modelling and management. International Journal of Wildland Fire 31, 471-479.
| Crossref | Google Scholar |
Davies GM, Legg CJ (2011) Fuel moisture thresholds in the flammability of Calluna vulgaris. Fire Technology 47, 421-436.
| Crossref | Google Scholar |
Davies GM, Legg CJ (2016) Regional variation in fire weather controls the reported occurrence of Scottish wildfires. PeerJ 4, e2649.
| Crossref | Google Scholar | PubMed |
Davies GM, Legg CJ, Smith AA, MacDonald AJ (2009) Rate of spread of fires in Calluna vulgaris‐dominated moorlands. Journal of Applied Ecology 46(5), 1054-1063.
| Crossref | Google Scholar |
Davies GM, Legg CJ, O’Hara R, MacDonald AJ, Smith AA (2010) Winter desiccation and rapid changes in the live fuel moisture content of Calluna vulgaris. Plant Ecology & Diversity 3, 289-299.
| Crossref | Google Scholar |
Davies GM, Gray A, Rein G, Legg CJ (2013) Peat consumption and carbon loss due to smouldering wildfire in a temperate peatland. Forest Ecology and Management 308, 169-177.
| Crossref | Google Scholar |
de Jong MC, Wooster MJ, Kitchen K, Manley C, Gazzard R, McCall FF (2016) Calibration and evaluation of the Canadian Forest Fire Weather Index (FWI) System for improved wildland fire danger rating in the United Kingdom. Natural Hazards and Earth System Sciences 16(5), 1217-1237.
| Crossref | Google Scholar |
Dickman LT, Jonko AK, Linn RR, Altintas I, Atchley AL, Bär A, Collins AD, Dupuy JL, Gallagher MR, Hiers JK, Hoffman CM, Hood SM, Hurteau MD, Jolly WM, Josephson A, Loudermilk EL, Ma W, Michaletz ST, Nolan RH, O’Brien JJ, Parsons RA, Partelli-Feltrin R, Pimont F, Resco de Dios V, Restaino J, Robbins ZJ, Sartor KA, Schultz-Fellenz E, Serbin SP, Sevanto S, Shuman JK, Sieg CH, Skowronski NS, Weise DR, Wright M, Xu C, Yebra M, Younes N (2023) Integrating plant physiology into simulation of fire behavior and effects. New Phytologist 238, 952-970.
| Crossref | Google Scholar | PubMed |
Didan K, Barreto A (2018) VIIRS/NPP Vegetation Indices 16-Day L3 Global 500m SIN Grid V001. NASA EOSDIS Land Processes DAAC. Available at 10.5067/VIIRS/VNP13A1.001 [Verified 1 December 2023]
Forestry Commission (2023) Wildfire statistics for England 2009-10 to 2020–2021. Available at https://assets.publishing.service.gov.uk/media/63ecff77d3bf7f62edc835a1/FC-Wildfire-statistics-for-England-Report-to-2020-21-.pdf [Verified 12 December 2023]
Gazzard R, McMorrow J, Aylen J (2016) Wildfire policy and management in England: an evolving response from Fire and Rescue Services, forestry and cross-sector groups. Philosophical Transactions of the Royal Society B: Biological Sciences 371, 20150341.
| Crossref | Google Scholar | PubMed |
Gregory JM, Jones PD, Wigley TML (1991) Precipitation in Britain: an analysis of area-average data updated to 1989. International Journal of Climatology 11, 331-345.
| Crossref | Google Scholar |
Grillakis M, Voulgarakis A, Rovithakis A, Seiradakis KD, Koutroulis A, Field RD, Kasoar M, Papadopoulos A, Lazaridis M (2022) Climate drivers of global wildfire burned area. Environmental Research Letters 17, 045021.
| Crossref | Google Scholar |
Harper A, Doerr SH, Santín C, Froyd C, Sinnadurai P (2018) Prescribed fire and its impacts on ecosystem services in the UK. Science of the Total Environment 624, 691-703.
| Crossref | Google Scholar | PubMed |
Jackson DL (2000) Guidance on the interpretation of the Biodiversity Broad Habitat Classification (terrestrial and freshwater types): definitions and the relationship with other classifications, JNCC Report No. 307, JNCC, Peterborough, ISSN 0963-8091. Available at https://data.jncc.gov.uk/data/0b7943ea-2eee-47a9-bd13-76d1d66d471f/JNCC-Report-307-SCAN-WEB.pdf [Verified 15 September 2023]
Jiang Z, Huete AR, Didan K, Miura T (2008) Development of a two-band enhanced vegetation index without a blue band. Remote Sensing of Environment 112(10), 3833-3845.
| Crossref | Google Scholar |
Jolly WM, Johnson DM (2018) Pyro-ecophysiology: shifting the paradigm of live wildland fuel research. Fire 1, 8.
| Crossref | Google Scholar |
Jolly WM, Hintz J, Linn RL, Kropp RC, Conrad ET, Parsons RA, Winterkamp J (2016) Seasonal variations in red pine (Pinus resinosa) and jack pine (Pinus banksiana) foliar physio-chemistry and their potential influence on stand-scale wildland fire behavior. Forest Ecology and Management 373, 167-178.
| Crossref | Google Scholar |
Jones MW, Abatzoglou JT, Veraverbeke S, Andela N, Lasslop G, Forkel M, Smith AJ, Burton C, Betts RA, van der Werf GR, Sitch S, Canadell JG, Santín C, Kolden C, Doerr SH, Quere C (2022) Global and regional trends and drivers of fire under climate change. Reviews of Geophysics 60, e2020RG000726.
| Crossref | Google Scholar |
Kelly J, Ibáñez TS, Santín C, Doerr SH, Nilsson MC, Holst T, Lindroth A, Kljun N (2021) Boreal forest soil carbon fluxes one year after a wildfire: effects of burn severity and management. Global Change Biology 27(17), 4181-95.
| Crossref | Google Scholar | PubMed |
Kidnie S, Wotton BM (2015) Characterisation of the fuel and fire environment in southern Ontario’s tallgrass prairie. International Journal of Wildland Fire 24, 1118-1128.
| Crossref | Google Scholar |
Kidnie S, Cruz MG, Gould J, Nichols D, Anderson W, Bessell R (2015) Effects of curing on grassfires: I. Fuel dynamics in a senescing grassland. International Journal of Wildland Fire 24, 828-837.
| Crossref | Google Scholar |
Lees KJ, Artz RRE, Chandler D, Aspinall T, Boulton CA, Buxton J, Cowie NR, Lenton TM (2021) Using remote sensing to assess peatland resilience by estimating soil surface moisture and drought recovery. Science of the Total Environment 761, 143312.
| Crossref | Google Scholar | PubMed |
Macias Fauria M, Michaletz ST, Johnson EA (2011) Predicting climate change effects on wildfires requires linking processes across scales. WIREs Climate Change 2, 99-112.
| Crossref | Google Scholar |
Maltby E, Legg CJ, Proctor MCF (1990) The ecology of severe moorland fire on the North York Moors: effects of the 1976 fires, and subsequent surface and vegetation development. Journal of Ecology 78, 490-518.
| Crossref | Google Scholar |
Met Office (2023) England and Wales Fire Severity Index. Available at https://www.metoffice.gov.uk/public/weather/fire-severity-index [Verified 18 September 2023]
Morton RD, Marston CG, O’Neil AW, Rowland CS (2020a) Land Cover Map 2018 (20-m classified pixels, GB). NERC Environmental Information Data Centre. Available at https://doi.org/10.5285/b3dfc4c7-c9bd-4a02-bed8-46b2a41be04a [Verified 18 September 2023]
Morton RD, Marston CG, O’Neil AW, Rowland CS (2020b) Land Cover Map 2018 (20m classified pixels, N. Ireland). NERC Environmental Information Data Centre. Available at https://doi.org/10.5285/cf5050d8-495d-45a6-9e2e-bba5239284c2 [Verified 18 September 2023]
Parisien MA, Barber QE, Flannigan MD, Jain P (2023) Broadleaf tree phenology and springtime wildfire occurrence in boreal Canada. Global Change Biology 29, 6106-6119.
| Crossref | Google Scholar | PubMed |
Perry MC, Vanvyve E, Betts RA, Palin EJ (2022) Past and future trends in fire weather for the UK. Natural Hazards and Earth System Sciences 22(2), 559-575.
| Crossref | Google Scholar |
Pimont F, Ruffault J, Martin-StPaul NK, Dupuy JL (2019) Why is the effect of live fuel moisture content on fire rate of spread underestimated in field experiments in shrublands? International Journal of Wildland Fire 28, 127-137.
| Crossref | Google Scholar |
Rein G, Cleaver N, Ashton C, Pironi P, Torero JL (2008) The severity of smouldering peat fires and damage to the forest soil. CATENA 74(3), 304-309.
| Crossref | Google Scholar |
Ruffault J, Martin-StPaul N, Pimont F, Dupuy JL (2018) How well do meteorological drought indices predict live fuel moisture content (LFMC)? An assessment for wildfire research and operations in Mediterranean ecosystems. Agricultural and Forest Meteorology 262, 391-401.
| Crossref | Google Scholar |
Salim KA, Carter PL, Shaw S, Smith CA (1988) Leaf abscission zones in Molinia caerulea (L.) Moench, the purple moor grass. Annals of Botany 62, 429-434.
| Crossref | Google Scholar |
San-Miguel-Ayanz J, Durrant T, Boca R, Maianti P, Libertá G, Artés-Vivancos T, Oom, D, Branco A, de Rigo D, Ferrari D, Pfeiffer H, Grecchi R, Onida M, Löffler P (2022) Forest fires in Europe, Middle East and North Africa 2021. (Publications Office of the European Union: Luxembourg) 10.2760/34094, JRC130846
Santana VM, Marrs RH (2014) Flammability properties of British heathland and moorland vegetation: models for predicting fire ignition. Journal of Environmental Management 139, 88-96.
| Crossref | Google Scholar | PubMed |
Santín C, Moustakas A, Doerr HS (2023) Searching the flames: trends in global and regional public interest in wildfires. Environmental Science & Policy 146, 151-161.
| Crossref | Google Scholar |
Schroeder W, Oliva P, Giglio L, Csiszar I (2014) The new VIIRS 375-m active fire detection data product: algorithm description and initial assessment. Remote Sensing of Environment 143, 85-96.
| Crossref | Google Scholar |
Sedano F, Randerson JT (2014) Multi-scale influence of vapor pressure deficit on fire ignition and spread in boreal forest ecosystems. Biogeosciences 11, 3739-3755.
| Crossref | Google Scholar |
Sjöström J, Granström A (2023) A phenology-driven fire danger index for northern grasslands. International Journal of Wildland Fire 32, 1332-1346.
| Crossref | Google Scholar |
Stoof CR, Kok E, Cardil A, van Marle MJE (2024) In temperate Europe, fire is already here: the case of The Netherlands. Ambio 53, 604-623.
| Crossref | Google Scholar | PubMed |
Vandvik V, Töpper JP, Cook Z, Daws MI, Heegaard E, Måren IE, Velle LG (2014) Management-driven evolution in a domesticated ecosystem. Biology Letters 10, 20131082.
| Crossref | Google Scholar | PubMed |
Van Wagner CE (1977) Conditions for the start and spread of crown fire. Canadian Journal of Forest Research 7, 23-34.
| Crossref | Google Scholar |
Van Wagner CE (1987) Development and structure of the Canadian Forest Fire Weather Index system. Vol. 35. Forestry Technical Report. p. 37. (Ottawa, Ontario) Available at https://cfs.nrcan.gc.ca/publications?id=19927 [accessed 1 December 2023]
Viegas DX, Piñol J, Viegas MT, Ogaya R (2001) Estimating live fine fuels moisture content using meteorologically based indices. International Journal of Wildland Fire 10, 223-240.
| Crossref | Google Scholar |
Vitolo C, Di Giuseppe F, Barnard C, et al. (2020) ERA5-based global meteorological wildfire danger maps. Scientific Data 7, 216.
| Crossref | Google Scholar | PubMed |
Weir JR, Limb RF (2013) Seasonal variation in flammability characteristics of Quercus marilandica and Quercus stellata leaf litter burned in the laboratory. Fire Ecology 9, 80-88.
| Crossref | Google Scholar |
Weise DR, Zhou X, Sun L, Mahalingam S (2005) Fire spread in chaparral—‘go or no-go?’. International Journal of Wildland Fire 14, 99-106.
| Crossref | Google Scholar |
Wotton BM, Beverly JL (2007) Stand-specific litter moisture content calibrations for the Canadian Fine Fuel Moisture Code. International Journal of Wildland Fire 16, 463-472.
| Crossref | Google Scholar |
Yule EL, Hegerl G, Schurer A, Hawkins E (2023) Using early extremes to place the 2022 UK heat waves into historical context. Atmospheric Science Letters 24, e1159.
| Crossref | Google Scholar |
Zhang X, Liu L, Liu Y, Jayavelu S, Wang J, Moon M, Henebry GM, Friedl MA, Schaaf CB (2018) Generation and evaluation of the VIIRS land surface phenology product. Remote Sensing of Environment 216, 212-229.
| Crossref | Google Scholar |