Before the fire: predicting burn severity and potential post-fire debris-flow hazards to conservation populations of the Colorado River Cutthroat Trout (Oncorhynchus clarkii pleuriticus)
Adam G. Wells
A
B
C
D
E
F
Abstract
Colorado River Cutthroat Trout (CRCT; Oncorhynchus clarkii pleuriticus) conservation populations may be at risk from wildfire and post-fire debris flows hazards.
To predict burn severity and potential post-fire debris flow hazard classifications to CRCT conservation populations before wildfires occur.
We used remote sensing, spatial analyses, and machine learning to model 28 wildfire incidents (2016–2020) and spatially predict burn severity from pre-wildfire environmental factors to evaluate the likelihood (%) and volume (m3) hazard classification of post-fire debris flow.
Burn severity was best predicted by fuels, followed by topography, physical ecosystem conditions, and weather (mean adjusted R2 = 0.54). Predictions of high or moderate burn severity covered 1.1 (15% of study area) and 1.5 (19% of study area) million ha, respectively, and varied by watershed. Combined high or moderate debris flow hazard classification included 80% of stream reaches with conservation populations and 97% of conservation population point nodes.
Predicted burn severity and potential post-fire debris flow indicated moderate to high hazard for CRCT conservation populations native to the Green and Yampa rivers of the Upper Colorado River Basin.
Future management actions can incorporate predicted burn severity and potential post-fire debris flow to mitigate impacts to CRCT and other at-risk resource values before a wildfire occurs.
Keywords: burn severity, Colorado River Cutthroat Trout, differenced Normalised Burn Ratio (dNBR), machine learning, post-fire debris flow, Sentinel-2, stream reach, Upper Colorado River Basin, wildland fire.
Introduction
High severity wildfires impact ecosystem services and natural resources directly and indirectly. Direct effects, including habitat loss and human and wildlife mortalities, overshadow indirect effects of wildfire like post-fire debris flow (PFDF; Cannon and DeGraff 2009), which pose a hazard to areas long after containment (Chow et al. 2019). Initiated by precipitation and resulting runoff, PFDF can severely impact aquatic ecosystems. Threats to aquatic ecosystems from PFDF hazard include mass wasting, scouring, sedimentation, flow alteration, physical and chemical contamination (Proctor et al. 2020; Kieta et al. 2023), which induces changes to water quality (temperature, turbidity, pH, dissolved oxygen, and nutrients), and can lead to extirpation of local fish populations. Furthermore, fish populations in headwater streams are often subject to metapopulation dynamics due to habitat fragmentation and environmental stochasticity (Roberts et al. 2013). Consequently, PFDF hazards can affect the persistence of fish populations throughout a stream network in areas outside of burn scars influencing the overall risk of population decline.
Colorado River Cutthroat Trout (CRCT; Oncorhynchus clarkii pleuriticus) are restricted to relatively short, headwater stream fragments (Hirsch et al. 2013) with 63% of recognised populations vulnerable to stochastic events including wildfire and PFDF (Roberts et al. 2013). The occurrence of high severity wildfire (Williams et al. 2009) followed by destructive PFDF poses a significant hazard to CRCT particularly in the headwater areas where CRCT are concentrated (Haak et al. 2010a). These sensitive areas typically have shallow soils, high topographic relief, and steep slopes that are susceptible to PFDF. Increases in wildfire occurrence, size, intensity, and severity across the western United States (Westerling 2016; Holden et al. 2018; Wilmot et al. 2022) exacerbate the hazard of PFDF to aquatic habitats of CRCT and other subspecies of Cutthroat Trout. Understanding the hazard to CRCT from wildfire and PFDF is needed to devise assessments of extirpation risk, pre-fire emergency planning, and pre- and post-fire mitigation strategies.
Predicting PFDF is difficult due to stochasticity and temporal and spatial variation in controlling factors. Understanding the likelihood of PFDF requires, among other factors, predictions of burn severity (Keeley 2009), which is the degree to which a wildfire consumes above and belowground organic matter (NWCG [National Wildfire Coordinating Group] 2023). Past research supports the application of differenced Normalised Burn Ratio (dNBR) as an indicator of burn severity for post-wildfire hazard identification and mitigation (Eidenshink et al. 2007; Fassnacht et al. 2021). DNBR is calculated by differencing reflectance of post-fire from pre-fire shortwave and near-infrared spectral bands using multi-spectral imagery such as Landsat-8 (Soverel et al. 2010; Guo et al. 2022) and Sentinel-2 (Drusch et al. 2012; Gibson et al. 2020; Morresi et al. 2022). While post-fire burn severity is frequently assessed using dNBR, predictions of burn severity prior to a wildfire can also be made, although considerable variation exists in modelled results due to wildfire behaviour (Wells et al. 2023). Nonetheless, planning and mitigation efforts seek to understand possible impacts of wildfire prior to a wildfire event. When coupled with PFDF hazard analysis, the utility of predictive pre-fire burn severity models can be enhanced for managers seeking to understand risk to ecosystems and species, including populations of CRCT. The decision whether to salvage a high-value native trout population ahead of an encroaching wildfire is currently made with very limited information. Subsequent PFDF is the primary wildfire related driver of extirpation in native trout populations (Sedell et al. 2015), yet there is often little time to evaluate whether an emergency fish salvage effort should proceed prior to a post-fire rain event. Pre-fire estimation of PFDF hazard can indicate risk to fish populations and assist conservation efforts before a wildfire emergency develops.
Here, we predict pre-fire burn severity and potential PFDF hazard across the native range of a single lineage of CRCT, the blue-lineage CRCT (‘blue’ sensu Metcalf et al. 2012; Bestgen et al. 2019), henceforth bCRCT, of special management concern. We aim to improve understanding of the landscape and stream scale hazards and subsequent risks to known conservation populations (CP), which are generally restricted to upper elevations and headwater streams. Our overall goal was to expand upon a recent approach to predict pre-fire burn severity and potential PFDF (Wells et al. 2023) and in turn characterise hazard to populations of bCRCT. Our specific objectives were to: (1) develop spatially explicit predictions of pre-fire burn severity across the native range of bCRCT and determine the relative importance of predictor variables; (2) estimate potential PFDF hazards based on predicted pre-fire burn severity; and (3) estimate hazard of PFDFs to bCRCT CP. Results provide a hazard analysis of possible effects of wildfire over a wide swath of the Upper Colorado River Basin and a targeted hazard assessment for bCRCT CP, which can be adapted to other species and values at risk.
Materials and methods
Study area
Our study covers the putative native range of bCRCT (Metcalf et al. 2012; Bestgen et al. 2019) including parts of Colorado, Wyoming, and Utah (Fig. 1). We predict pre-fire burn severity and potential PFDF hazard across this distribution, but also present more detailed analyses focused on the CP, loosely defined as those displaying less than 10% admixture with non-native trout determined from molecular study (Hirsch et al. 2013). These CP are spatially nested within the historic and current distribution of bCRCT (Fig. 1). We selected watersheds from the Watershed Boundary Dataset WBD (2023) 12-digit Hydrologic Unit Codes (HUC-12) within 1 km (n = 723) of the historic distribution of bCRCT delineated by Hirsch et al. (2013). The extent (Fig. 1) covered roughly 7.5 million ha and includes five vegetation types (Kuchler 1964): (1) Great Basin shrub/steppe (35%); (2) Rocky Mountain conifer forest (34%); (3) Great Basin/Southwest forest (21%); (4) Great Basin shrub/grassland (8%); and (5) alpine meadow (2%). Our analysis involved multiple steps; these are summarised below and in Fig. 2.
Study area extent derived from 12-digit Hydrologic Unit Code (HUC-12) watershed boundaries within 1 km of the current and historic distributions of blue lineage Colorado River Cutthroat Trout-Blue Lineage (bCRCT), 4-digit HUC (HUC-4) watershed boundaries, Monitoring Trends in Burn Severity (MTBS) wildfire perimeters (n = 28) from 2016 to 2020, and nested distribution of bCRCT conservation populations. Note: map derived from fig. 1 in Bestgen et al. (2019).
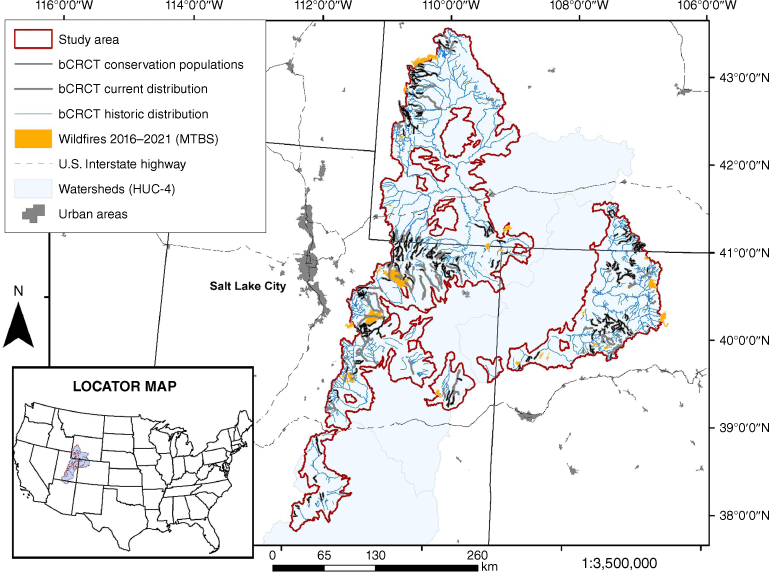
Flowchart of analysis steps including data inputs, linkages, algorithms, and hierarchy to model and quantify hazard of potential burn severity and potential post-fire debris flow to conservation populations of blue lineage Colorado River Cutthroat Trout (bCRCT; Oncorhynchus clarkii pleuriticus) in unburned areas.
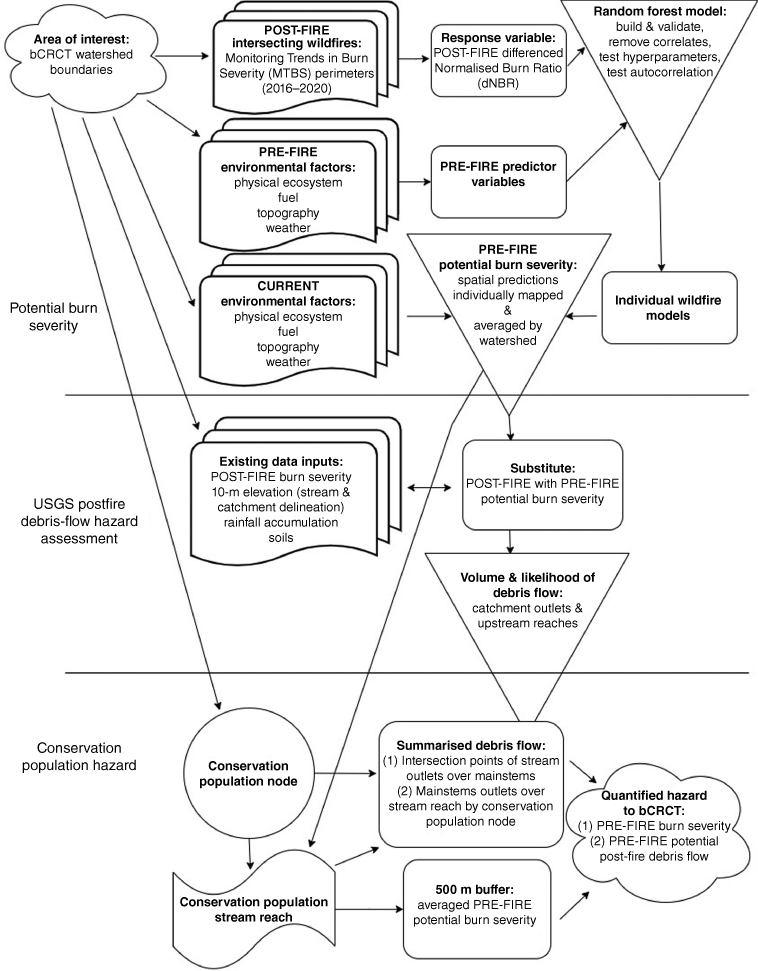
We identified 28 wildfires (Table 1) with perimeters that intersected the study area spanning 2016–2020 (Fig. 1) from the inter-agency database Monitoring Trends in Burn Severity (Eidenshink et al. 2007; MTBS [Monitoring Trends in Burn Severity program] 2023). We selected fires from 2016 onward to align with imagery available from the Sentinel-2 mission (European Space Agency 2023). We modelled pre-fire burn severity for individual wildfires and cast averaged predictions by associated HUC 4-digit (HUC-4) watershed boundaries that intersected our study area (Fig. 3). This included the Upper Green (8 wildfires), Lower Green (8 wildfires), Yampa (12 wildfires), and Dirty Devil watersheds. We modelled pre-fire burn severity in the Dirty Devil HUC-4 watershed, but because no large wildfires occurred there during 2016–2020, pre-fire burn severity was modelled based on wildfires from the closest adjoining watershed, the Lower Green. To quantify direct effects of potential wildfire, we tabulated predicted pre-fire burn severity within 500-m buffers around streams identified by the Geographic Names Information System (GNIS [Geographic Names Information System] 2023) of bCRCT CP, which were composed of 70% Douglas fir/spruce/fir (Psuedotsuga menziesii, Picea spp., Abies spp.) based on potential natural vegetation in the conterminous United States (Kuchler 1964). We quantified indirect effects of wildfire by modelling potential PFDF at the scale of stream reach associated with bCRCT CP (Fig. 3) and tabulated hazard to individually known localised populations of bCRCT CP by name (node ID).
Incident Name | Ignition Date | HUC-4 | Latitude (N) | Longitude (W) | Size (ha) | Unburned-Low (ha) | Low (ha) | Moderate (ha) | High (ha) | |
---|---|---|---|---|---|---|---|---|---|---|
Bear Trap | 21 July 2018 | LG | 39.295 | −109.882 | 3729 | 247 | 931 | 1511 | 1038 | |
Bender Mountain | 3 September 2018 | UG | 40.962 | −109.056 | 1537 | 127 | 820 | 418 | 170 | |
Big Red | 19 August 2017 | Y | 40.926 | −106.86 | 1161 | 165 | 419 | 338 | 222 | |
Boulder Lake | 17 August 2019 | UG | 42.856 | −109.674 | 551 | 11 | 300 | 239 | 0 | |
Cabin Lake | 29 July 2018 | Y | 39.919 | −107.593 | 2333 | 635 | 758 | 624 | 207 | |
Center Creek Trail | 25 August 2020 | LG | 40.65 | −110.445 | 715 | 249 | 397 | 55 | 11 | |
Cowboy | 9 September 2017 | UG | 41.399 | −110.757 | 680 | 28 | 146 | 438 | 0 | |
Deep Creek | 4 September 2017 | Y | 40.592 | −107.102 | 1626 | 308 | 499 | 398 | 419 | |
Dollar Ridge | 1 July 2018 | LG | 40.111 | −110.877 | 28,254 | 5053 | 9474 | 10,032 | 3525 | |
East Fork | 21 August 2020 | LG | 40.6 | −110.603 | 43,072 | 12,241 | 11,181 | 8954 | 9610 | |
Fawn | 7 July 2018 | Y | 39.755 | −108.406 | 419 | 78 | 139 | 202 | 0 | |
Fawn Creek | 13 July 2020 | Y | 39.788 | −108.386 | 1376 | 189 | 426 | 693 | 68 | |
Fly Canyon | 8 September 2016 | LG | 39.425 | −111.28 | 1082 | 184 | 288 | 255 | 331 | |
Hunt | 5 September 2019 | Y | 39.773 | −108.293 | 1298 | 149 | 251 | 551 | 348 | |
Laney Rim | 28 July 2018 | UG | 41.201 | −108.973 | 4690 | 543 | 2930 | 1219 | 0 | |
Lost Solar | 8 August 2016 | Y | 39.893 | −107.421 | 2080 | 780 | 768 | 266 | 267 | |
Marten Creek | 16 September 2018 | UG | 42.72 | −110.64 | 2583 | 344 | 994 | 749 | 492 | |
Middle Fork | 6 September 2020 | Y | 40.636 | −106.762 | 8452 | 1231 | 2242 | 2591 | 2326 | |
Murdock | 28 August 2018 | LG | 40.669 | −110.858 | 2092 | 424 | 902 | 503 | 248 | |
Pole Creek | 4 August 2017 | UG | 42.182 | −110.675 | 1374 | 366 | 517 | 332 | 134 | |
Red Canyon | 29 July 2018 | Y | 39.685 | −108.729 | 2307 | 238 | 781 | 1115 | 172 | |
Richard Mountain | 3 August 2020 | UG | 40.995 | −109.262 | 3157 | 690 | 1808 | 617 | 42 | |
Roosevelt | 15 September 2018 | UG | 43.06 | −110.387 | 22,391 | 5040 | 7814 | 3839 | 5691 | |
Silver Creek | 19 July 2018 | Y | 40.234 | −106.587 | 8387 | 2488 | 2466 | 1881 | 1504 | |
Snake | 10 September 2016 | Y | 41.049 | −107.061 | 1035 | 320 | 309 | 221 | 182 | |
Streeter | 7 July 2020 | Y | 40.288 | −107.781 | 734 | 84 | 542 | 108 | 0 | |
Tank Hollow | 11 August 2017 | LG | 40.006 | −111.252 | 4453 | 966 | 1895 | 1410 | 181 | |
Trail Mountain | 7 June 2018 | LG | 39.409 | −111.174 | 7423 | 1120 | 2254 | 2609 | 1412 | |
Total | 158,990 | 34,296 | 52,251 | 42,167 | 28,599 | |||||
Proportion of Total | 0.22 | 0.33 | 0.27 | 0.18 |
Note: 4-digit Hydrologic Unit Code (HUC-4) watersheds are Lower Green (LG), Upper Green (UG), and Yampa (Y). Italics, wildfire used to hindcast a singular test case pre-fire dNBR to wildfire shown in boldface (Fig. 5).
Predicted differenced Normalised Burn Ratio (dNBR) differentiated by 4-digit Hydrologic Unit Code (HUC-4) watersheds (Dirty Devil, Lower Green, Yampa, and Upper Green) for contemporary conditions (September 2022) used to assess hazard of potential post-fire debris flow for conservation populations of blue lineage Colorado River Cutthroat Trout (bCRCT; Oncorhynchus clarkii pleuriticus) in unburned areas.
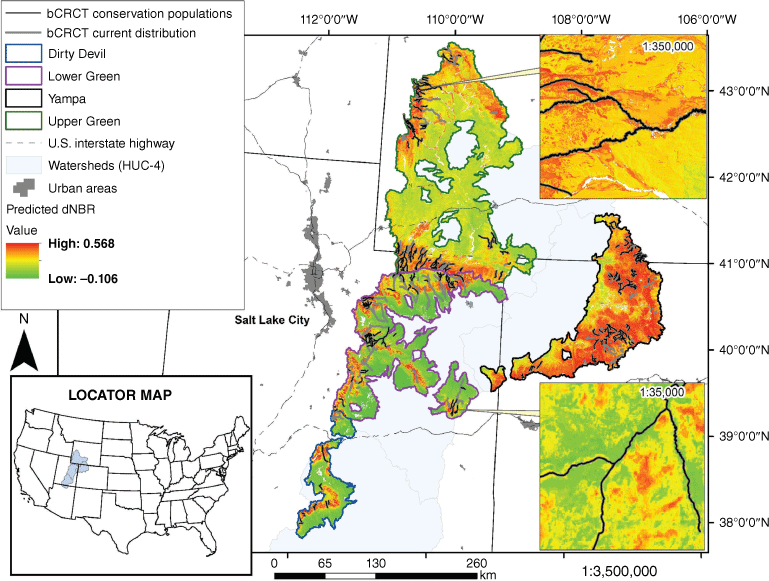
Predicting burn severity
We developed machine learning model of burn severity based on pre-fire conditions, henceforth referred to as predicted burn severity. Each wildfire was modelled individually using observed post-fire MTBS dNBR pixel values as a response variable. Research shows wildfire depends upon multiple interacting environmental factors, including: (1) physical ecosystem conditions (Hagmann et al. 2021); (2) fuel (Keeley and Syphard 2019); (3) topography (Povak et al. 2018); and (4) weather (Litschert et al. 2012), which formed the basis of our predictor variable development.
We developed predictions of potential burn severity using pre-fire environmental factors as previously demonstrated in Wells et al. (2023). To characterise the pre-fire physical ecosystem, we used Sentinel-2A/B (Drusch et al. 2012) imagery from Copernicus Open Access Hub https://scihub.copernicus.eu and the Sentinel Applications Platform (SNAP ver. 7.0; European Space Agency 2022) to pre-process digital imagery and create predictor variables. We created predictor variables for two periods prior to each wildfire. The first period was from as close to the ignition date as possible and the second was an early season period prior to the onset of the growing season and free of cloud or snow over the wildfire perimeter. Use of two periods provided a more robust range of physical ecosystem conditions and inherent delineation between deciduous and evergreen vegetation cover. Sentinel-2 images were corrected based on user specified inputs (Stand-alone sen2cor ver. 2.9; STEP 2024) for atmosphere, bidirectional reflectance, and topography and converted to reflectance. Individual spectral bands (1–8a; 11–12) were used along with Normalised Difference in Vegetation Index (NDVI, Huang et al. 2021), Soil-Adjusted Vegetation Index (SAVI, Rondeaux et al. 1996), and biophysical parameters of leaf area index, fraction of absorbed photolytically active radiation, chlorophyll content in leaf, fraction of vegetation cover, and canopy water content that were included in past work (Wells et al. 2023).
Fuel and topographic information were acquired from existing data products. To characterise surface fuels, we used yearly estimates of herbaceous cover (HERB) of annual and perennial grasses, and forbs (Rigge et al. 2022). To estimate overstorey fuels, we used LANDFIRE (2016) LF 1.4.0 Forest Canopy Cover (FCC), representing fuels prior to the earliest wildfire. Elevation variables were extracted from the National Elevation Data products at 1/3 arc second (Gesch et al. 2018). Additional topographic variables of slope, terrain position index, terrain roughness index, surface roughness, and cosine and sine transformation of aspect were calculated from elevation.
For weather information, we extracted gridded surface meteorological data (Abatzoglou 2013) and averaged daily values over three time-lags prior to ignition: (1) 7 days; (2) 30 days; and (3) one calendar year. These time-lags represented differing climate, fire weather, and fuel conditions that we expected to influence burn severity. Predictors included 100-h and 1000-h dead fuel moisture, specific humidity, maximum and minimum relative humidity, maximum and minimum air temperature, precipitation, wind speed, vapour pressure deficit, downward surface shortwave radiation, alfalfa and grass reference evapotranspiration, energy release component and burning index.
For each fire, we used a geographic information system (GIS) to sample pixels of MTBS datasets of observed dNBR and associated pre-fire predictor variables. We randomly sampled pixels within each fire perimeter to develop model building (training-testing; 40%), and validation (reserved; 10%) partitions. We used a random forest (Breiman 2001) in Anaconda Python 3.10.0 with the scikit library to fit and validate models of dNBR with the training-testing and validation data partitions, respectively (Pedregosa et al. 2011; Anaconda Software Distribution 2023). We removed highly correlated (R > 0.70) predictor variables with a forward stepwise routine (Sherrouse and Hawbaker 2023) and tested for spatial auto-correlation with regression kriging (Wells et al. 2023). The random forest algorithm used a 10-fold cross-validation (CV) based on root mean square error (RMSE) of the CV test fold, with 500 estimators and a minimum of 10 samples per leaf. To assess variable contributions to model compositions, we tallied variable frequency and normalised variable importance values within each model to calculate the summed proportional variable importance (SPVI) over all models. SPVI is calculated by taking the importance value of each selected variable per individual model, divided by the total variable importance of all variables from that individual model, and summing that particular variable over all models. For each variable we tabulated percentiles of SPVI over all models and averaged SPVI by environmental factor (physical ecosystem, fuel, topography, and weather). Spatial extrapolation of pre-fire burn severity, or model predictions, were based on the variables from each individual wildfire model with data acquired representing a contemporary period (11–19 September 2022), with early season imagery variables matched to May–June 2022. For each wildfire model, predictions were cast over the associated HUC-4 watershed and averaged by arithmetic mean. Averaged predicted dNBR by HUC-4 watershed were classified based on natural breaks (Jenks and Caspall 1971) into four severity classes to mirror MTBS burn severity (Fig. 4). Averaged predictions were merged and scaled for display to show relative dNBR over the entire study area (Fig. 3). To verify model functionality, we used hindcasting of the largest individual wildfires (East Fork) based on the Dollar Ridge Fire model (Fig. 5), which occurred within the same watershed (Lower Green) the year prior (Table 1) and compared predicted severity classes to observed MTBS post-fire burn severity. We tabulated a confusion matrix of predicted pre-fire burn severity classification (binned moderate and high) against observed MTBS thematic burn severity (MTBS [Monitoring Trends in Burn Severity program] 2023) and reported the overall accuracy and Kappa statistic.
Predicted burn severity, as of September 11–19 2022, based on classified predicted differenced Normalised Burn Ratio (dNBR Fig. 3) for portions of the (a) Yampa, (b) Lower Green, (c) Upper Green, and (d) Dirty Devil watersheds containing Colorado River Cutthroat Trout (Oncorhynchus clarkii pleuriticus) shown in style of Monitoring Trends in Burn Severity (MTBS) data and as used for input to estimating potential post-fire debris flow.
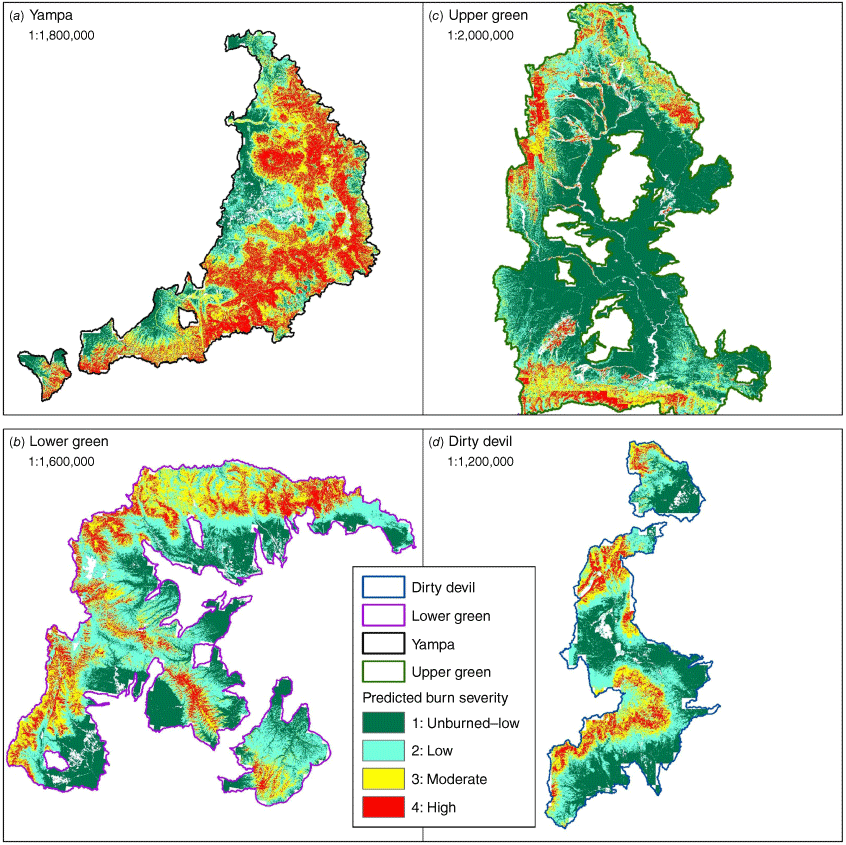
Hindcast of one wildfire model to another wildfire showing (a) pre-fire predicted differenced Normalised Burn Ratio (dNBR) of the East Fork Fire derived from the model of the Dollar Ridge Fire (blue-red = low-high), (b) predicted burn severity (green = unburned or low, red = moderate or high), (c) post-fire observed dNBR of the East Fork Fire from the Monitoring Trends in Burn Severity (MTBS) program (blue-red = low-high), and (d) observed MTBS burn severity (green = unburned or low, red = moderate or high). Note: blank spot in 4A and 4B are due to missing values.
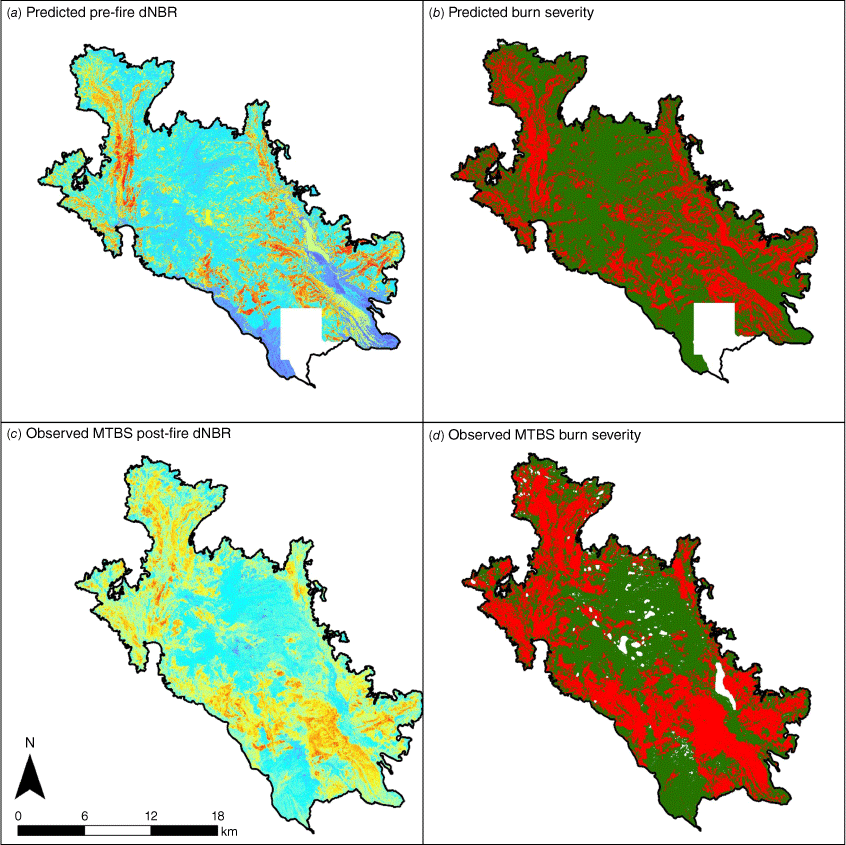
Potential for post-fire debris flow
We used the U.S. Geological Survey (USGS) post wildfire debris flow hazard assessment (U.S. Geological Survey 2023) to estimate potential for PFDFs based on our predictions of burn severity. The hazard assessment uses empirical models to estimate the likelihood (%) and potential volume (m3) of PFDF in response to a series of simulated storms with 15-min peak rainfall intensities (12–40 mm h−1 precipitation by 4 mm h−1 increments), which may be expected within a 1-year recurrence interval (NOAA [National Oceanic and Atmospheric Administration] 2023). We delineated the stream reach drainage network and associated catchments within each HUC-12 watershed based on 10-m elevational data and GIS stream analysis. PFDF model calculations were performed at each catchment outlet and along upstream drainage networks by stream reach. Debris-flow likelihood calculations used logistic regression (Staley et al. 2016) and our inputs of predicted pre-fire burn severity classified as moderate or high (described above), soil properties (K-factor of the universal soil loss equation; Wischmeier and Smith 1965) and rainfall accumulation for a given duration. Potential debris-flow volumes were predicted using multiple linear regression models (Gartner et al. 2014) with simulated rainfall intensity, basin morphology, and predicted burn severity (classified as moderate or high, as described above). Volume estimates were classified by order of magnitude from 0–1000 m3, 1000–10,000 m3, 10,000–100,000 m3, and greater than 100,000 m3. We combined likelihood and volume debris flow results (Table 2) following Cannon et al. (2010).
Combined debris-flow hazard | ||||||
---|---|---|---|---|---|---|
Volume (m3) | ||||||
% | <1000 | 1000–10,000 | 10,000–100,000 | >100,000 | ||
Likelihood | 0–20 | Low | Low | Moderate | Moderate | |
20–40 | Low | Moderate | Moderate | Moderate | ||
40–60 | Moderate | Moderate | Moderate | High | ||
60–80 | Moderate | Moderate | High | High | ||
80–100 | Moderate | High | High | High |
Conservation population hazard
We quantified hazard to bCRCT CP by assessing both direct effects of potential wildfire burn severity and indirect effects of potential PFDF. We spatially aggregated predicted burn severity and potential PFDF to stream reaches and CP node ID, respectively. We buffered (500 m) stream reaches of CP and quantified averaged predicted burn severity, (dNBR), by stream name following nomenclature from the GNIS since single CP often occupy more than one named stream. Since multiple PFDF stream segments conjoin stream reaches of bCRCT CP, we summarised potential PFDF linear stream segments by averaging to points at the intersections of stream outlets with mainstem segments. The mainstem outlet points were averaged over stream reaches, based on rankings of none (0), low (1), moderate (2), and high classification (3) for increasing simulated storm intensities, by bCRCT CP node ID. This final step (Fig. 2) where we averaged linear stream segments to outlet points on mainstem streams and mainstem outlets by stream reach, created a hazard index value of potential PFDF, per simulated storm intensity, for each bCRCT CP node ID.
Results
Predicting burn severity
Over the study area, the 28 wildfires analysed burned 159,000 ha from 2016 to 2020 (Table 1). Observed mean dNBR from individual wildfires ranged from a low of 0.13 to a high of 0.49 with an average of 0.29 (Table 3). Average RMSE values of the random forest model of pre-fire dNBR, based on validation dataset, were 57% of the averaged observed mean dNBR (Table 3). One model (Fly Canyon) showed large variation of >100% RMSE of observed mean dNBR. Based on validation data, the models had adjusted R2 ranging from 0.17 to 0.73, with an overall average adjusted R2 = 0.54 (Table 3). There was no significant difference in CV-test RMSE between any of the random forests models and regression kriging models, suggesting model residuals had little to no spatial auto-correlation. Model functionality based on hindcasting the East Fork Fire with the Dollar Ridge model showed strong spatial congruency compared with observed dNBR and burn severity from post-fire MTBS (Fig. 5). Prediction of categorised burn severity of the East Fork Fire based on the Dollar Ridge model was marginally accurate (overall accuracy of 0.64, κ = 0.28). Random forest models predicted 2.6 million ha (34%) of the study area (Fig. 3) as moderate to high burn severity. Categorised burn severity by HUC-4 watershed (Fig. 4) showed the Yampa (Fig. 4a) with the greatest amount (1.1 million ha; 63%) of expected moderate to high burn severity, followed by the Lower Green (Fig. 4b; 689,000 ha; 33%), the Upper Green (Fig. 4c; 645,000 ha; 20%), and the Dirty Devil (Fig. 4d) with the least (144,000 ha; 29%). Predicted dNBR ranged from a low of –0.11 to a high of 0.57 (Fig. 3).
Fire | Mean dNBR | Regression RMSE | Validation (20%) | Regression Adj. R2 | Validation (20%) | |
---|---|---|---|---|---|---|
CV test folds (80%) | CV test folds (80%) | |||||
Beartrap | 0.4481 | 0.1789 ± 0.0168 | 0.1633 | 0.34 ± 0.089 | 0.51 | |
Bender Mountain | 0.3340 | 0.1576 ± 0.0531 | 0.1454 | 0.14 ± 0.16 | 0.49 | |
Big red | 0.3618 | 0.2049 ± 0.0408 | 0.1910 | 0.22 ± 0.2 | 0.43 | |
Boulder Lake | 0.3188 | 0.0791 ± 0.0317 | 0.0810 | 0.53 ± 0.243 | 0.57 | |
Cabin Lake | 0.2046 | 0.1583 ± 0.0132 | 0.1424 | 0.57 ± 0.089 | 0.68 | |
Center Creek Trail | 0.1317 | 0.1353 ± 0.0429 | 0.1327 | 0.03 ± 0.187 | 0.26 | |
Cowboy | 0.3540 | 0.1057 ± 0.0229 | 0.1018 | 0.37 ± 0.106 | 0.48 | |
Deep Creek | 0.3682 | 0.1670 ± 0.0219 | 0.1589 | 0.58 ± 0.109 | 0.68 | |
Dollar Ridge | 0.3112 | 0.1411 ± 0.0326 | 0.1244 | 0.55 ± 0.121 | 0.73 | |
East Fork | 0.2110 | 0.1699 ± 0.0227 | 0.1403 | 0.30 ± 0.202 | 0.62 | |
Fawn | 0.2319 | 0.0995 ± 0.0171 | 0.0992 | 0.48 ± 0.156 | 0.55 | |
Fawn Creek | 0.1924 | 0.0832 ± 0.0152 | 0.0858 | 0.59 ± 0.108 | 0.59 | |
Fly Canyon | 0.3435 | 0.3297 ± 0.3999 | 0.9061 | 0.44 ± 0.246 | 0.17 | |
Hunt | 0.3634 | 0.1323 ± 0.0242 | 0.1194 | 0.35 ± 0.173 | 0.59 | |
Laney Rim | 0.2233 | 0.0685 ± 0.0073 | 0.0580 | 0.57 ± 0.083 | 0.73 | |
Lost Solar | 0.2234 | 0.1922 ± 0.0307 | 0.1838 | 0.14 ± 0.304 | 0.36 | |
Marten Creek | 0.2491 | 0.1879 ± 0.021 | 0.1681 | 0.36 ± 0.076 | 0.56 | |
Middle Fork | 0.3552 | 0.1759 ± 0.0183 | 0.1622 | 0.28 ± 0.172 | 0.45 | |
Murdock | 0.2706 | 0.2060 ± 0.0457 | 0.1755 | 0.05 ± 0.287 | 0.47 | |
Pole Creek | 0.2467 | 0.1911 ± 0.0218 | 0.1832 | 0.32 ± 0.121 | 0.43 | |
Red Canyon | 0.3334 | 0.1465 ± 0.0229 | 0.1327 | 0.31 ± 0.102 | 0.50 | |
Richard Mountain | 0.1253 | 0.0570 ± 0.0111 | 0.0495 | 0.48 ± 0.322 | 0.72 | |
Roosevelt | 0.3503 | 0.2165 ± 0.035 | 0.1903 | 0.43 ± 0.14 | 0.59 | |
Silver Creek | 0.3446 | 0.1933 ± 0.0205 | 0.1667 | 0.42 ± 0.137 | 0.67 | |
Snake | 0.2893 | 0.2076 ± 0.0161 | 0.1876 | 0.40 ± 0.124 | 0.51 | |
Streeter | 0.3107 | 0.1187 ± 0.0108 | 0.1098 | 0.41 ± 0.184 | 0.61 | |
Tank Hollow | 0.2292 | 0.1561 ± 0.0174 | 0.1453 | 0.09 ± 0.144 | 0.38 | |
Trail Mountain | 0.3578 | 0.1504 ± 0.0156 | 0.1348 | 0.62 ± 0.087 | 0.71 |
Wildfire models were unique in terms of variable selection. In total, there were 329 instances of variable inclusion across 28 wildfire-specific models, with ecosystem factors accounting for 140 instances of variable inclusion, topography 73, weather 71, and fuel 45 (Fig. 6). Accounting for variable overlap among models resulted in 76 unique variables used, averaging 12 variables per model. Controlling for period and lag resulted in 42 variables accounting for pre-fire predicted burn severity (Fig. 6). The most frequently used (25 of 28 models) predictor among all models was of HERB whereas the predictor with the greatest SPVI was elevation (19/28 models, Fig. 6). The most frequently selected predictors (in 14/28 fires or more) included both variables related to fuels (HERB and FCC), two variables related to topography (elevation and terrain position index), and three remotely sensed variables related to physical ecosystem (Sentinel-2 bands 9 (945 nm) & 12 (2190 nm), and SAVI; Fig. 6). Both Sentinel-2 bands 9 and 12 measure reflectance of short-wave infra-red radiation, useful for detecting water vapour and vegetation moisture stress, respectively (European Space Agency 2023). Based on SPVI, the most influential predictors included elevation and three variables related to ecosystem condition (Sentinel-2 band 12, Sentinel-2 band 9, and leaf area index; Fig. 6). The most frequently included weather variable (7/28 models) was the amount of precipitation with a 7-day lag. When SPVI was averaged by environmental factors the largest contributions were from fuel (1.20), followed by topography (0.83), physical ecosystem (0.81), and weather (0.28).
Factors, total predictor variable counts over all models, and percentile of Summed Proportional Variable Importance (SPVI), with period and lags removed, of random forest models used to predict burns severity from 28 wildfires that occurred between 2016 and 2020. Topography abbreviations: TPI, terrain position index; TRI, terrain roughness index; RUF, surface roughness; COS, cosine of aspect; SIN, sine of aspect. Fuel abbreviations: HERB, herbaceous cover (HERB); FFC, forest canopy cover. Ecosystem abbreviations: Sentinel-2 Bands (2 = B2, 3 = B3, 4 = B4, 5 = B5, 6 = B6, 7 = B7, 8 = B8, 8a = B8a, 9 = B9, 11 = B11, 12 = B12); NDVI, normalised difference in vegetation index; SAVI, soil-adjusted vegetation index; LAI, leaf area index; FAPAR, fraction of absorbed photolytically active radiation; CCL, chlorophyll content in leaf; FVC, fraction of vegetation cover; CWC, canopy water content. Weather abbreviations: FM100, 100-h dead fuel moisture; FM1000, 1000-h dead fuel moisture; TMMX, maximum air temperature; TMMN, minimum air temperature; PR, precipitation; SRAD, downward surface shortwave radiation; WS, windspeed; RMAX, maximum relative humidity; RMIN, minimum relative humidity; SPH, specific humidity; ETR, alfalfa reference evapotranspiration; PET, grass reference evapotranspiration; ERC, energy release component; BI, burning index; VPD, vapour pressure deficit.
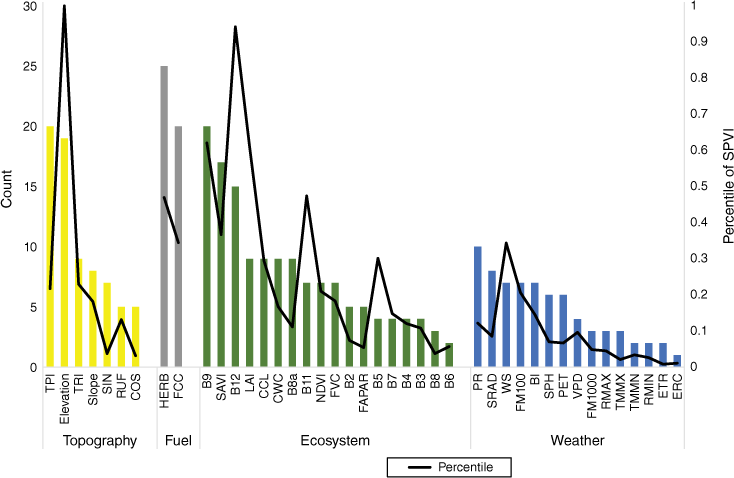
Potential for post-fire debris flow
The overall number and lengths of stream reaches of bCRCT CP (1903) analysed varied by HUC-4 watershed boundary: 727 stream reaches in the Upper Green (1067 km), 604 in the Yampa (718 km), 496 in the Lower Green (648 km), and 76 in the Dirty Devil (89 km). Excluding null values of predicted debris-flow reduced the number of bCRCT CP stream reaches with classified debris-flow of either low, medium, or high to 1643. By HUC-4 watershed boundary this resulted in 638 of 727 bCRCT stream reaches (88%) in the Upper Green, 532 of 604 in the Yampa (88%), 409 of 496 in the Lower Green (82%), and 64 of 76 in the Dirty Devil (84%) with an elevated hazard classification from PFD.
The combined hazard classifications of potential PFDF of stream reaches currently occupied by bCRCT CP increased with storm intensity (Figs. 7, 8). Overall, 86% of streams reaches with bCRCT CP exhibited elevated hazard classification of potential PFDF in response to increased storm intensity (Table 4, Fig. 7). At maximum storm intensity of 40 mm h−1, potential PFDF increased to the highest hazard classification in roughly a third of all stream reaches (Table 4 and Fig. 7). Above 28 mm h−1, more than two-thirds of stream reaches showed potential PFDF hazards of moderate or high, increasing up to 80% at 40 mm h−1 (Table 4, Fig. 8). Combined hazard classifications of the moderate category were present throughout all storm intensities; above 24 mm h−1 more than half of stream reaches were classified as such (Table 4). Potential PFDF over all stream reaches of bCRCT CP showed elevated moderate and high hazard as peak precipitation intensities increased. Similar patterns were present when tabulated by HUC-4 watershed (Table 4).
Potential post-fire debris flow (PFDF) combined (likelihood, %; volume, m3) hazard classifications based on a simulated peak 15-min rainfall intensity of 40 mm h−1 for stream reaches with blue lineage Colorado River Cutthroat Trout (bCRCT; Oncorhynchus clarkii pleuriticus) conservation populations and nodes. Note: inset maps show reference for scale and examples of mainstem outlets used for aggregation to node.
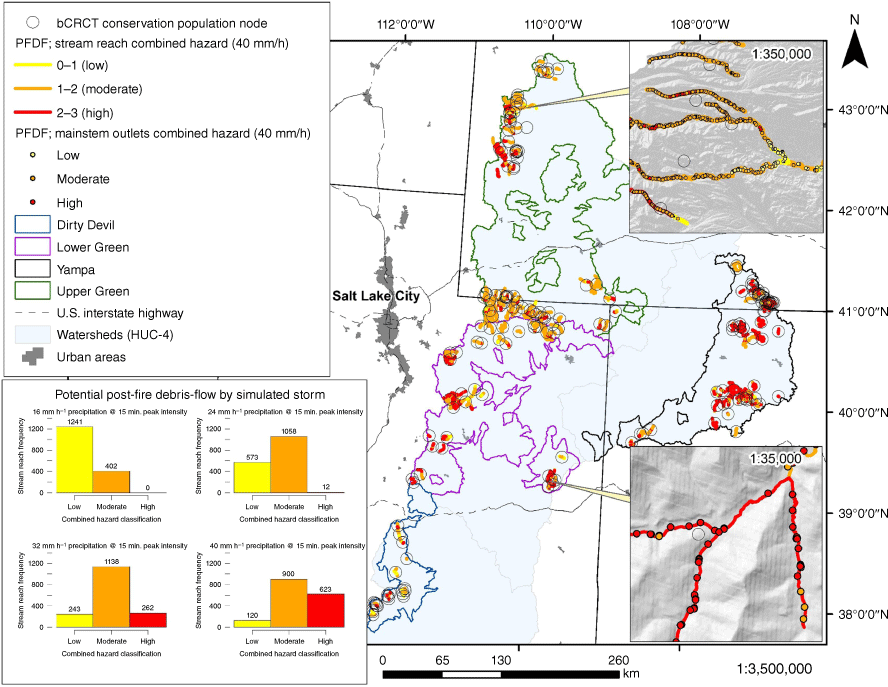
Proportion of the combined (likelihood, %; volume, m3) hazard classifications (high, and moderate or high) of potential post-fire debris flow with blue lineage Colorado River Cutthroat Trout (Oncorhynchus clarkii pleuriticus) Conservation Populations (bCRCT CP) and their associated stream reaches by 4-digit Hydrologic Unit Code (HUC-4) watershed boundaries based on predicted burn severity of wildfire in unburned areas and simulated peak 15-min rainfall intensities (mm h−1).
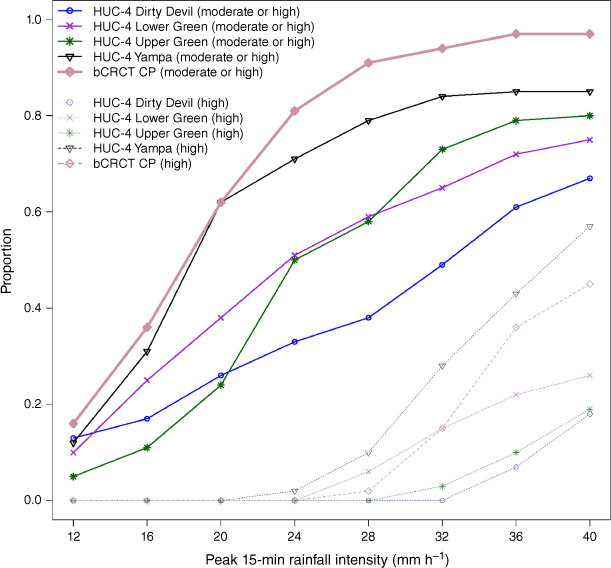
Intensity | Overall | Yampa (HUC-4) | Upper Green (HUC-4) | Lower Green (HUC-4) | Dirty Devil (HUC-4) | ||||||||||||||||
---|---|---|---|---|---|---|---|---|---|---|---|---|---|---|---|---|---|---|---|---|---|
Null | Lo | Mod | Hi | Null | Lo | Mod | Hi | Null | Lo | Mod | Hi | Null | Lo | Mod | Hi | Null | Lo | Mod | Hi | ||
12 | 0.14 | 0.78 | 0.09 | 0.00 | 0.12 | 0.76 | 0.12 | 0.00 | 0.12 | 0.83 | 0.05 | 0.00 | 0.18 | 0.72 | 0.10 | 0.00 | 0.16 | 0.71 | 0.13 | 0.00 | |
16 | 0.14 | 0.65 | 0.21 | 0.00 | 0.12 | 0.57 | 0.31 | 0.00 | 0.12 | 0.77 | 0.11 | 0.00 | 0.18 | 0.57 | 0.25 | 0.00 | 0.16 | 0.67 | 0.17 | 0.00 | |
20 | 0.14 | 0.46 | 0.40 | 0.00 | 0.12 | 0.26 | 0.62 | 0.00 | 0.12 | 0.63 | 0.24 | 0.00 | 0.18 | 0.44 | 0.38 | 0.00 | 0.16 | 0.58 | 0.26 | 0.00 | |
24 | 0.14 | 0.30 | 0.56 | 0.01 | 0.12 | 0.17 | 0.70 | 0.02 | 0.12 | 0.38 | 0.50 | 0.00 | 0.18 | 0.31 | 0.51 | 0.00 | 0.16 | 0.51 | 0.33 | 0.00 | |
28 | 0.14 | 0.22 | 0.59 | 0.05 | 0.12 | 0.09 | 0.69 | 0.10 | 0.12 | 0.29 | 0.58 | 0.00 | 0.18 | 0.24 | 0.53 | 0.06 | 0.16 | 0.46 | 0.38 | 0.00 | |
32 | 0.14 | 0.13 | 0.60 | 0.14 | 0.12 | 0.04 | 0.56 | 0.28 | 0.12 | 0.14 | 0.71 | 0.03 | 0.18 | 0.17 | 0.50 | 0.15 | 0.16 | 0.36 | 0.49 | 0.00 | |
36 | 0.14 | 0.08 | 0.55 | 0.23 | 0.12 | 0.03 | 0.42 | 0.43 | 0.12 | 0.09 | 0.69 | 0.10 | 0.18 | 0.11 | 0.50 | 0.22 | 0.16 | 0.24 | 0.54 | 0.07 | |
40 | 0.14 | 0.06 | 0.47 | 0.33 | 0.12 | 0.03 | 0.29 | 0.57 | 0.12 | 0.07 | 0.62 | 0.19 | 0.18 | 0.07 | 0.49 | 0.26 | 0.16 | 0.17 | 0.49 | 0.18 |
The number of bCRCT CP populations within high PFDF hazard potential varied greatly among basins. The Yampa had the greatest number of stream reaches (343) occupied by bCRCT CP in the highest hazard classification at maximum storm intensity, followed by the Upper Green (135), the Lower Green (131), and the Dirty Devil (14). Proportionally, the Yampa also had the greatest proportion of occupied stream reaches in the highest hazard classification at maximum storm intensity (0.57), followed by the Lower Green (0.26), the Upper Green (0.19), and the Dirty Devil (0.18; Table 4). By combined stream lengths, the Yampa had the greatest distances (480 km) in the highest hazard classification, followed by the Lower Green (245 km), the Upper Green (214 km), and the Dirty Devil (12 km). When both high and moderate hazard classifications were considered, the rankings among watersheds shifted subtly. Specifically, the Upper Green contained the greatest number of stream reaches (584; 80%) occupied by CP classified as moderate or high followed by the Yampa (516; 85%) indicating there were fewer stream reaches, but a higher percentage, in the Yampa classified as high or moderate then in the Upper Green. Analysis of the Lower Green showed 372, or 75%, (Fig. 8) of stream reaches with moderate or high combined hazard classification, and the Dirty Devil with 51, or 67% (Fig. 8).
Conservation population hazard
In total, CP of bCRCT spanned ~2500 km within 422 spatially distinct GNIS streams comprised of 1903 reaches that constituted the 190 nodes (Fig. 7): 66 in the Yampa; 65 in the Upper Green; 37 in the Lower Green; and 22 in the Dirty Devil. (Fig. 7). Within 500-m buffers of streams with bCRCT CP average predicted dNBR (Fig. 3) ranged from 0.17 to 0.46 (see Supplementary Table S1). When tabulated by HUC-4 watershed, the proportion of predicted moderate or high burn severity classifications (Fig. 4) within 500-m buffers was highest in the Yampa watershed (0.76), followed by the Upper Green (0.47), Lower Green (0.43), and the Dirty Devil (0.41). Overall, proportions of moderate or high severity were greater within 500-m buffers around streams occupied by bCRCT CP than across HUC-4 watershed.
The mainstem outlet points averaged over stream reaches of associated bCRCT CP nodes (Fig. 7) produced hazard index values per simulated storm intensity (Table S2). Hazard index values showed a steady increase in hazard to bCRCT CP from PFDF classifications as storm intensity increased (Table S2). At peak storm intensity (40 mm h−1) 184 of 190 bCRCT CP (Fig. 8; 97%) were at risk from moderate (52%) or high (45%) potential PFDF hazards. Reflecting overall trends in PFDF hazard classifications by stream reach (Fig. 8), the bCRCT CP accumulated high hazard classifications at peak storm intensities exceeding 24 mm h−1 (Fig. 8). At the lowest storm intensity (12 mm h−1) 16% of bCRCT CP were at risk from moderate potential PFDF, with the remaining 84% classified as low. At the finest spatial scale of analysis, that of the bCRCT conservation population node (Fig. 7), cumulative combined hazard of potential PFDF was greatest, relative to stream reach and watershed.
Discussion
We predicted pre-fire dNBR, burn severity classification, and potential PFDF hazard across the range (Fig. 1) of bCRCT (Bestgen et al. 2019) and quantified the hazard to CP at risk. Our predictions reflect multiple underlying environmental factors that interact to elevate the occurrences of high severity wildfire and post-fire hazards. Our results can be used to assess other values at risk from wildfire as well as enabling targeted management actions to mitigate these risks.
Predicting burn severity
Our models of dNBR had an overall average adjusted R2 = 0.54, which is within the range (0.26–0.63) of values reported for other models (Birch et al. 2015; Kane et al. 2015; Picotte et al. 2021; Pascolini-Campbell et al. 2022; Wells et al. 2023). Our hindcasting of one model predicting the outcome of a different wildfire resulted in a lower R2 value (0.25) but provided confirmation of general spatial patterning. Use of Relativised dNBR in future work may improve model fit as we expect a high degree of heterogeneity in vegetation, and therefore spectral reflectance, of pre-fire factors (Cansler and McKenzie 2012), however this would necessitate recalibration of the PFDF equations. Our study represents an advance in understanding potential burn severity before a wildfire occurs by employing pre-fire conditions of fuel, topography, physical ecosystem, and weather. Much of the unexplained variation between predicted and observed dNBR and burn severity is likely due to the inherently dynamic behaviour of wildfire that can rapidly change with prevailing weather and fuel conditions. Incorporating diurnal fluctuations (Poulos et al. 2021; Pascolini-Campbell et al. 2022) in ambient air temperature, humidity, and wind speed that are specific to the location of the wildfire may improve future predictions.
The threat of wildfire was high across HUC-4 watersheds. The Yampa watershed showed the greatest areal extent of expected moderate to high burn-severity, which influence PFDF. Recent wildfires, such as the East Troublesome Fire within similar ecosystems and fuels conditions (beetle-killed conifer) have exhibited a rapid rate of spread and high burn severity (Meldrum et al. 2022). The spruce/fir vegetation type existing at upper elevations within the Yampa basin has a low fire return interval of ~300 years (Schoennagel et al. 2004) and high predicted burn severity. Similarly, high predicted burn severity was reflected in upper elevations of the Upper Green (Fig. 4a) and Lower Green (Fig. 4b) HUC-4 watersheds, with forested assemblages of Douglas fir/spruce/fir. The lower, more arid elevations of the Upper and Lower Green primarily support sagebrush (Artemisia spp.) and pinyon/juniper (Pinus edulis, Juniperus spp.) vegetation, respectively. Consequently, we expect to have lower predicted burn severity primarily due to the lower amount of woody biomass and the greater spatial heterogeneity of these fuel types. This pattern was mirrored in the arid and southerly Dirty Devil watershed, largely composed of pinyon/juniper but with forested Douglas fir assemblages occurring at higher elevations. These relationships to severity were supported by the frequency and importance of elevation and FCC variables. We expected HERB, which was frequently included in models, to influence burn severity through its influence on rate of fire spread and intensity. Despite its importance, fine fuel is often not well characterised in existing fire models and can be improved upon with future efforts (Wells et al. 2021). Both environmental factors of fuel and topography were readily apparent in the spatial distribution of predicted burn severity. Large swaths of the Upper and Lower Green and Dirty Devil joined the Yampa watersheds with high burn severity classification, comprising roughly 1.1 million ha, or 15% of the study area. Combined with moderate burn severity, those classes used to estimate PFDF, covered roughly 2.6 million ha of the study area. Future research should investigate the differences in variables used to model pre-fire burn severity based on plant community (i.e. lower elevation shrublands vs higher elevation forests) to better understand the drivers of burn severity across different ecotypes.
Potential for post-fire debris flow
We took a novel approach to modelling potential PFDF at the stream reach scale by including predicted burn severity before a wildfire occurs (Cannon et al. 2010; Sedell et al. 2015). Current operational USGS PFDF models (U.S. Geological Survey 2023) rely on burn severity estimated post-fire. Prediction of burn severity using our methodology represents an important step in developing and refining hazard classifications for broad application to unburned but potentially at-risk areas. Additionally, our PFDF estimates reflect landscape factors that likely initiate, propagate, and accumulate debris-flow based on basin morphology, slope, soils, and precipitation. Adjustment of soil parameters including hydrophobicity, the loss of infiltration, and other physical changes due to fire effects (Lopes et al. 2021; Ebel et al. 2023) were not considered in the model but offer opportunities for further study. Likewise, additional research focused on sediment delivery downstream of watershed outlets may improve hazard assessment, as this physical process is not accounted for by the USGS operational model. The application of the debris flow assessment prior to wildfires and across large spatial extents has been historically restricted by the requirement of post-fire dNBR and burn severity estimates. Here, we have presented an opportunity to shift the assessment framework from a post-fire emergency reaction to a pre-fire early warning, enabling wide-spread assessment. This can increase the robustness of the emergency assessment system as well as proactively identifying the risk to streams, aquatic species, or other water resources before a real hazard emerges.
The potential PFDF combined hazard over all stream reaches of bCRCT CP was almost entirely moderate to high for high intensity rainfall events. The hazard in the Yampa was more pronounced based on the greater amount of moderate to high predicted burn severity, but the hazard in the Upper Green was collectively broader based on the greater number of CRCT stream reaches with moderate to high potential PFDF. Hazard was similar between Upper Green and Lower Green in terms of number and length of streams in the highest hazard class. Spatially, the Lower Green had a similar overall length of stream reaches of bCRCT populations as that of the Yampa, but the Yampa was proportionally much greater in terms of overall number and length of streams with the highest potential PFDF. The Dirty Devil watershed showed the lowest potential PFDF by number and length of stream reaches with bCRCT populations. Risk in Dirty Devil should not be disregarded on the basis of lower hazard classifications however, as small population size and structure may increase the vulnerability to localised extinction (Cook et al. 2010).
Past research to assess risk to Cutthroat Trout populations from wildfire (Williams et al. 2009) or PFDF (Sedell et al. 2015) or wildfires and PFDFs (Miller and Bassett 2013) have used a variety of GIS (Williams et al. 2009; Sedell et al. 2015) and statistical tools (Cannon et al. 2010; Miller and Bassett 2013) to identify hazards. This includes analysis based on landscape characteristics derived from elevation models (10-m DEM; Sedell et al. 2015), in conjunction with LANDFIRE data products (Williams et al. 2009) and FlamMap analysis (Miller and Bassett 2013) at the stream reach (Sedell et al. 2015) or basin (Williams et al. 2009) scale. Our framework melds a singular approach for estimating of hazards derived from both wildfire (Wells et al. 2023) and debris flow (U.S. Geological Survey 2023) for both basin and stream reach scales. While this was an important modelling improvement, the emerging effects of climate change increase the need for an early warning system integrated with near-term climate forecasting (Bradford et al. 2018). Similarly, validation efforts to describe model fitting of PFDF events will benefit from further research to detect PFDF based on hydrologic data from widespread monitoring. Our efforts to validate potential PFDF classifications were hindered by the sparsity of data on PFDF events that may have occurred originating from within a study wildfire. Improvements to PFDF predictions can be advanced through further research that detects (Hürlimann et al. 2019) and identifies the impact of PFDF on hydrologic function.
Conservation population hazard
For bCRCT CP, estimates of the combined hazard classification of potential PFDF were cumulatively compiled by stream reaches and outlets to quantify hazard (Table S2), similar to past work assessing vulnerability of CRCT habitat (Conservation Science Partners [Theobald D, Leinwand I, Harrison-Atlas D] 2018). Hazard of PFDF to bCRCT CP was greatest for the Yampa, followed by the Upper Green, Lower Green, and Dirty Devil watersheds. The higher hazard to bCRCT CP in the Yampa basin was a result of the greatest number, length, and proportion of moderate or high classifications of PFDF over all simulated storm intensities. Hazard indices can be included in population viability assessments that predict population persistence in a changing climate (e.g. Zeigler et al. 2019), and more importantly, enable CRCT managers to consider risk from wildfire to individual habitat patches defined by Hirsch et al. (2013) and develop mitigation strategies accordingly. Management actions that could help secure native trout diversity in a more fire-prone future could include founding new CP with translocations of CRCT from high-risk patches or important peripheral populations (Haak et al. 2010b), reducing burn severity by defining and implementing strategic fuel reduction opportunities, reducing the chances of ignition (e.g. public access restrictions or powerline blackouts), and engineered debris-flow mitigation (retention structures e.g. Volkwein et al. 2011). Most importantly, the ability to predict PFDF hazard will help inform pre-fire planning to determine whether emergency fish salvage operations should proceed when a fire threatens or has burned through a CP. These efforts involve risk to personnel as well as resident trout and are therefore generally missions of last resort but have been used with good success to save valuable native trout populations, such as those at Hayden Creek, CO (Rogers et al. 2020).
Our approach provides a quantifiable characterisation of hazard based on the long-term indirect effects of wildfire. These results build upon past work to forecast burn severity and subsequent PFDF before they happen, helping to inform conservation planning and long-term risk management of valued fisheries. By using an explicit spatial evaluation and assessment, we are able to bridge an implicit understanding of burn severity from both direct and indirect effects of wildfire to an at-risk aquatic species in western North America.
Data availability
Data generated from this study are available from Wells et al. (2024) at the USGS ScienceBase: https://doi.org/10.5066/P13KYQVK.
Disclaimer
Any use of trade, firm, or product names is for descriptive purposes only and does not imply endorsement by the US Government.
Declaration of funding
Funding for this study was provided by the U.S. Geological Survey Ecosystems and Water Mission Areas.
References
Abatzoglou JT (2013) Development of gridded surface meteorological data for ecological applications and modelling. International Journal of Climatology 33, 121-131.
| Crossref | Google Scholar |
Anaconda Software Distribution (2023) Anaconda version 3.10.0. Available at https://www.anaconda.com/ [verified 21 March 2023]
Bestgen KR, Rogers KB, Granger R (2019) Distinct phenotypes of native cutthroat trout emerge under a molecular model of lineage distributions. Transactions of the American Fisheries Society 148, 442-463.
| Crossref | Google Scholar |
Birch DS, Morgan P, Kolden CA, Abatzoglou JT, Dillon GK, Hudak AT, Smith AMS (2015) Vegetation, topography and daily weather influenced burn severity in central Idaho and western Montana forests. Ecosphere 6, 1-23.
| Crossref | Google Scholar |
Bradford JB, Betancourt JL, Butterfield BJ, Munson SM, Wood TE (2018) Anticipatory natural resource science and management for a changing future. Frontiers in Ecology and the Environment 16, 295-303.
| Crossref | Google Scholar |
Breiman L (2001) Random forests. Machine Learning 45, 5-32.
| Crossref | Google Scholar |
Cannon S, DeGraff J (2009) The increasing wildfire and post-fire debris-flow threat in western USA, and implications for consequences of climate change. In ‘Landslides – disaster risk reduction’. (Eds K Sassa, P Canuti) pp. 177–190. (Springer-Verlag: Berlin, Heidelberg) 10.1007/978-3-540-69970-5_9
Cannon SH, Gartner JE, Rupert MG, Michael JA, Rea AH, Parrett C (2010) Predicting the probability and volume of postwildfire debris flows in the intermountain western United States. Geological Society of America Bulletin 122, 127-44.
| Crossref | Google Scholar |
Cansler CA, McKenzie D (2012) How robust are burn severity indices when applied in a new region? Evaluation of alternate field-based and remote-sensing methods. Remote Sensing 4, 456-483.
| Crossref | Google Scholar |
Chow AT, Tsai KP, Fegel TS, Pierson DN, Rhoades CC (2019) Lasting effects of wildfire on disinfection by-product formation in forest catchments. Journal of Environmental Quality 48, 1826-1834.
| Crossref | Google Scholar |
Conservation Science Partners [Theobald D, Leinwand I, Harrison-Atlas D] (2018) ‘Vulnerability of Colorado River Cutthroat Trout habitat to climate change in the Green River Basin.’ (U.S. Geological Survey) Available at https://www.sciencebase.gov/catalog/item/5a500a42e4b0d05ee8c7dad9
Cook N, Rahel FJ, Hubert WA (2010) Persistence of Colorado River Cutthroat Trout populations in isolated headwater streams of Wyoming. Transactions of the American Fisheries Society 139, 1500-1510.
| Crossref | Google Scholar |
Drusch M, Del Bello U, Carlier S, Colin O, Fernandez V, Gascon F, Hoersch B, Isola C, Laberinti P, Martimort P, Meygret A, Spoto F, Sy O, Marchese F, Bargellini P (2012) Sentinel-2: ESA’s optical high-resolution mission for GMES operational services. Remote Sensing of Environment 120, 25-36.
| Crossref | Google Scholar |
Ebel BA, Shephard ZM, Walvoord MA, Murphy SF, Partridge TF, Perkins KS (2023) Modeling post-wildfire hydrologic response: review and future directions for applications of physically based distributed simulation. Earth’s Future 11, e2022EF003038.
| Crossref | Google Scholar |
Eidenshink J, Schwind B, Brewer K, Zhu ZL, Quayle B, Howard S (2007) A project for monitoring trends in burn severity. Fire Ecology 3, 3-21.
| Crossref | Google Scholar |
European Space Agency (2022) STEP – Science Toolbox Exploitation Platform. Available at http://step.esa.int/main/. [verified 27 November 2023]
European Space Agency (2023) User Guide. Available at https://sentinel.esa.int/web/sentinel/user-guides/sentinel-2-msi/resolutions/spectral/. [Verified 27 November 2023]
Fassnacht FE, Schmidt-Riese E, Kattenborn T, Hernández J (2021) Explaining Sentinel 2-based dNBR and RdNBR variability with reference data from the bird’s eye (UAS) perspective. International Journal of Applied Earth Observation and Geoinformation 95, 102262.
| Crossref | Google Scholar |
Gartner JE, Cannon SH, Santi PM (2014) Empirical models for predicting volumes of sediment deposited by debris flows and sediment-laden floods in the transvers range of southern California. Engineering Geology 176, 45-56.
| Crossref | Google Scholar |
Gesch DB, Evans GA, Arundel S (2018) The national elevation dataset. In ‘Digital elevation model technologies and applications: The DEM users manual 3rd edn’. (Eds DF Maune, A Nayegandhi) pp. 83–110. (American Society for Photogrammetry and Remote Sensing) Available at https://pubs.usgs.gov/publication/70201572
Gibson R, Danaher T, Hehir W, Collins L (2020) A remote sensing approach to mapping fire severity in south-eastern Australia using sentinel 2 and random forest. Remote Sensing of Environment 240, 111702.
| Crossref | Google Scholar |
GNIS [Geographic Names Information System] (2023) Available at https://www.usgs.gov/tools/geographic-names-information-system-gnis [verified 27 November 2023]
Guo L, Li S, Wu Z, Parsons RA, Lin S, Wu B, Sun L (2022) Assessing spatial patterns and drivers of burn severity in subtropical forests in Southern China based on Landsat 8. Forest Ecology and Management 524, 120515.
| Crossref | Google Scholar |
Haak AL, Williams JE, Isaak D, Todd A, Muhlfeld C, Kershner J, Gresswell R, Hostetler S, Neville HM (2010a) The potential influence of changing climate on the persistence of salmonids of the inland west: U.S. Geological Survey Open-File Report 2010–1236. (Reston, VA) Available at https://pubs.usgs.gov/of/2010/1236/
Haak AL, Williams JE, Neville HM, Dauwalter DC, Colyer WT (2010b) Conserving peripheral trout populations: The values and risks of life on the edge. Fisheries 35, 530-549.
| Crossref | Google Scholar |
Hagmann RK, Hessburg PF, Prichard SJ, Povak NA, Brown PM, et al. (2021) Evidence for widespread changes in the structure, composition, and fire regimes of western North American forests. Ecological Applications 31, e02431.
| Crossref | Google Scholar |
Hirsch CL, Dare MR, Albeke SE (2013) Range-wide status of Colorado River cutthroat trout (Oncorhynchus clarkii pleuriticus): 2010. Colorado River Cutthroat Trout Conservation Team Report. (Colorado Parks and Wildlife: Fort Collins, CO) Available at https://cpw.state.co.us/Documents/Research/Aquatic/CutthroatTrout/CRCTRangewideAssessment-08.04.2013.pdf
Holden ZA, Swanson A, Luce CH, Affleck D (2018) Decreasing fire season precipitation increased recent western US forest wildfire activity. Proceedings of the National Academy of Sciences 115, E8349-E8357.
| Crossref | Google Scholar |
Huang S, Tang L, Hupy JP, Wang Y, Shao G (2021) A commentary review on the use of normalized difference vegetation index (NDVI) in the era of popular remote sensing. Journal of Forestry Research 32, 1-6.
| Crossref | Google Scholar |
Hürlimann M, Coviello V, Bel C, Guo X, Berti M, Graf C, Hübl J, Miyata S, Smith JB, Yin HY (2019) Debris-flow monitoring and warning: review and examples. Earth-Science Reviews 199, 102981.
| Crossref | Google Scholar |
Jenks GF, Caspall FC (1971) Error on choroplethic maps: definition, measurement, reduction. Annals of the Association of American Geographers 61, 217-244.
| Crossref | Google Scholar |
Kane VR, Cansler CA, Povak NA, Kane JT, McGaughey RJ, Lutz JA, Churchill DJ, North MP (2015) Mixed severity fire effects within the Rim fire: Relative importance of local climate, fire weather, topography, and forest structure. Forest Ecology and Management 358, 62-79.
| Crossref | Google Scholar |
Keeley J (2009) Fire intensity, fire severity and burn severity: a brief review and suggested usage. International Journal of Wildland Fire 18, 116-126.
| Crossref | Google Scholar |
Keeley JE, Syphard AD (2019) Twenty-first century California, USA, wildfires: fuel-dominated vs. wind-dominated fires. Fire Ecology 15, 24.
| Crossref | Google Scholar |
Kieta KA, Owens PN, Petticrew EL (2023) Post-wildfire contamination of soils and sediments by polycyclic aromatic hydrocarbons in north-central British Columbia, Canada. International Journal of Wildland Fire 32, 1071-1088.
| Crossref | Google Scholar |
Kuchler AW (1964) ‘Potential natural vegetation of the conterminous United States’. Special Publication No. 36. (American Geographical Society) Available at https://databasin.org/datasets/1c7a301c8e6843f2b4fe63fdb3a9fe39/ [verified 27 November 2023]
LANDFIRE (2016) ‘Forest Canopy Cover Layer, LANDFIRE 1.4.0.’ (U.S. Department of the Interior, Geological Survey, and U.S. Department of Agriculture) Available at https://www.landfire.gov/viewer/ [verified 27 November 2023]
Litschert SE, Brown TC, Theobold DM (2012) Historic and future extent of wildfires in the Southern Rockies Ecoregion, USA. Forest Ecology and Management 69, 124-133.
| Crossref | Google Scholar |
Lopes AR, Girona-García A, Corticeiro S, Martins R, Keizer JJ, Vieira DCS (2021) What is wrong with post-fire soil erosion modelling? A meta-analysis on current approaches, research gaps, and future directions. Earth Surface Processes and Landforms 46, 205-219.
| Crossref | Google Scholar |
Meldrum JR, Barth CM, Goolsby JB, Olson SK, Gosey AC, White J, Brenkert-Smith H, Champ PA, Gomez J (2022) Parcel-level risk affects wildfire outcomes: Insights from pre-fire rapid assessment data for homes destroyed in 2020 East Troublesome Fire. Fire 5, 24.
| Crossref | Google Scholar |
Metcalf JL, Love Stowell S, Kennedy CM, Rogers KB, McDonald D, Epp J, Keepers K, Cooper A, Austin JJ, Martin AP (2012) Historical stocking data and 19th century DNA reveal human-induced changes to native diversity and distribution of cutthroat trout. Molecular Ecology 21, 5194-5207.
| Crossref | Google Scholar |
Miller LW, Bassett S (2013) ‘Rio Grande Cutthroat Trout wildfire risk assessment.’ (Nature Conservancy: Santa Fe, New Mexico) Available at https://www.wildlife.state.nm.us/fishing/native-new-mexico-fish/rio-grande-cutthroat-trout/ [verified 26 September 2023]
Morresi D, Marzano R, Lingua E, Motta R, Garbarino M (2022) Mapping burn severity in the western Italian Alps through phenologically coherent reflectance composites derived from Sentinel-2 imagery. Remote Sensing of Environment 269, 112800.
| Crossref | Google Scholar |
MTBS [Monitoring Trends in Burn Severity program] (2023) Available at https://www.mtbs.gov/ [verified 24 May 2023]
NOAA [National Oceanic and Atmospheric Administration] (2023) Precipitation Frequency Data Server. Available at https://hdsc.nws.noaa.gov/pfds/ [verified 17 July 2024]
NWCG [National Wildfire Coordinating Group] (2023) ‘NWCG Glossary of Wildland Fire, PMS 205 (National Interagency Fire Center: Boise, ID, USA) Available at https://www.nwcg.gov/publications/pms205/nwcg-glossary-of-wildland-fire-pms-205 [verified 23 Oct 2024]
Pascolini-Campbell M, Lee C, Stavros N, Fisher JB (2022) ECOSTRESS reveals pre-fire vegetation controls on burn severity for Southern California wildfires of 2020. Global Ecology and Biogeography 31, 1976-1989.
| Crossref | Google Scholar |
Pedregosa F, Varoquaux G, Gramfort A, Michel V, Thirion B, Grisel O, Blondel M, Prettenhofer P, Weiss R, Dubourg V, Vanderplas J, Passos A, Cournapeau D, Brucher M, Perrot M, Duchesnay E (2011) Scikit-learn: machine learning in Python. Journal of Machine Learning Research 12, 2825-2830.
| Google Scholar |
Picotte JJ, Cansler CA, Kolden CA, Lutz JA, Key C, Benson NC, Robertson KM (2021) Determination of burn severity models ranging from regional to national scales for the conterminous United States. Remote Sensing of Environment 263, 112569.
| Crossref | Google Scholar |
Poulos HM, Barton AM, Koch GW, Kolb TE, Thode AE (2021) Wildfire severity and vegetation recovery drive post-fire evapotranspiration in a southwestern pine-oak forest, Arizona, USA. Remote Sensing in Ecology and Conservation 7, 579-591.
| Crossref | Google Scholar |
Povak NA, Hessburg PF, Salter RM (2018) Evidence for scale-dependent topographic controls on wildfire spread. Ecosphere 9, e02443.
| Crossref | Google Scholar |
Proctor CR, Lee J, Yu D, Shah AD, Whelton AJ (2020) Wildfire caused widespread drinking water distribution network contamination. AWWA Water Science 2, e1183.
| Crossref | Google Scholar |
Rigge MB, Bunde B, Postma K, Shi H (2022) Rangeland Condition Monitoring Assessment and Projection (RCMAP) fractional component time-series across the western U.S. 1985–2021: U.S. Geological Survey data release. Available at https://doi.org/10.5066/P9ODAZHC [verified 28 November 2023]
Roberts JJ, Fausch KD, Peterson DP, Hooten MB (2013) Fragmentation and thermal risks from climate change interact to affect persistence of native trout in the Colorado River basin. Global Change Biology 19, 1383-1398.
| Crossref | Google Scholar |
Rogers KB, Firestone S, Whiteley A, Lukacs PM (2020) Spawn matrixing fails to improve survival in a unique Cutthroat Trout population following fire-mediated extirpation in the wild. In ‘Cutthroat Trout Studies, Colorado Parks and Wildlife Annual Research Report’. (Ed. K Rogers) pp. 21–27. (Colorado Parks and Wildlife: Steamboat Springs, CO)
Rondeaux G, Steven M, Baret F (1996) Optimization of soil-adjusted vegetation indices. Remote Sensing of Environment 55, 95-107.
| Crossref | Google Scholar |
Schoennagel T, Veblen TT, Romme WH (2004) The interaction of fire, fuels, and climate across Rocky Mountain forests. BioScience 54, 661-676.
| Crossref | Google Scholar |
Sedell ER, Gresswell RE, McMahon TE (2015) Predicting spatial distributions of postfire debris flows and potential consequences for native trout in headwater streams. Freshwater Science 34, 1558-1570.
| Crossref | Google Scholar |
Sherrouse BC, Hawbaker TJ (2023) HOPS: Hyperparameter optimization and predictor selection v1.0. U.S. Geological Survey Software Release. 10.5066/P9P81HUR [verified 30 November 2023]
Soverel NO, Perrakis DDB, Coops NC (2010) Estimating burn severity from Landsat dNBR and RdNBR indices across western Canada. Remote Sensing of Environment 114, 1896-1909.
| Crossref | Google Scholar |
Staley D, Negri JA, Kean JW, Laber JM, Tillery AC, Youberg AM, Kimball SM (2016) ‘Updated logistic regression equations for the calculation of post-fire debris-flow likelihood in the western United States.’ Open-File Report 2016–1106. (U.S. Geological Survey: Reston, VA) 10.3133/OFR20161106
Science Toolbox Exploitation Platform (STEP) (2024) Sens2Cor v2.9. https://step.esa.int/main/snap-supported-plugins/sen2cor/sen2cor-v2-9. [verified 28 November 2023]
U.S. Geological Survey (2023) Emergency Assessment of Post-fire Debris-flow Hazards. Available at https://landslides.usgs.gov/hazards/postfire_debrisflow [verified 05 May 2023]
Volkwein A, Wendeler C, Guasti G (2011) Design of flexible debris flow barriers. Italian Journal of Engineering Geology and Environment 1093-1100.
| Crossref | Google Scholar |
Watershed Boundary Dataset [WBD] (2023). Available at https://www.usgs.gov/national-hydrography/watershed-boundary-dataset [verified 27 November 2023]
Wells AG, Munson SM, Sesnie SE, Villarreal ML (2021) Remotely Sensed Fine-Fuel Changes from Wildfire and Prescribed Fire in a Semi-Arid Grassland. Fire 4(4), 84.
| Crossref | Google Scholar |
Wells AG, Hawbaker TJ, Hiers KJ, Kean J, Loehman RA, Steblein PF (2023) Predicting burn severity for integration with post-fire debris-flow hazard assessment: a case study from the Upper Colorado River Basin, USA. International Journal of Wildland Fire 32, 1315-1331.
| Crossref | Google Scholar |
Wells AG, Kostelnik J, Bock A (2024) Pre-fire predicted burn severity for estimating hazard of post-fire debris flow for conservation populations of blue-lineage Colorado River Cutthroat Trout (Oncorhynchus clarkii pleuriticus) in the Upper Colorado River Basin: U.S. Geological Survey data release, doi:10.5066/P13KYQVK
Westerling AL (2016) Increasing western US forest wildfire activity: sensitivity to changes in the timing of spring. Philosophical Transactions of the Royal Society B: Biological Sciences 371, 20150178.
| Crossref | Google Scholar |
Williams JE, Haak AL, Neville HM, Colyer WT (2009) Potential consequences of climate change to persistence of Cutthroat Trout populations. North American Journal of Fisheries Management 29, 533-548.
| Crossref | Google Scholar |
Wilmot TY, Mallia DV, Hallar AG, Lin JC (2022) Wildfire plumes in the Western US are reaching greater heights and injecting more aerosols aloft as wildfire activity intensifies. Scientific Reports 12, 12400.
| Crossref | Google Scholar |
Wischmeier WH, Smith DD (1965) Predicting rainfall-erosion losses from cropland east of the Rocky Mountains: a guide for selecting practices for soil and water conservation. USDA Agricultural Handbook No. 282 https://www.ars.usda.gov/ARSUserFiles/50201000/USLEDatabase/AH_282.pdf [verified 3 November 2023].
| Google Scholar |
Zeigler MP, Rogers KB, Roberts JJ, Todd AS, Fausch KD (2019) Predicting persistence of Rio Grande Cutthroat Trout populations in an uncertain future. North American Journal of Fisheries Management 39, 819-848.
| Crossref | Google Scholar |