Does grazing exclusion in Australia’s rangelands affect biomass and debris carbon stocks?
David I. Forrester
A
B
C
Abstract
Australia is dominated by 6 million km2 of rangelands that contribute significantly to the livestock industry and carbon (C) market; yet, few studies have quantified the influence of grazing management on woody biomass and C stocks across these rangelands. Here, we quantified stand-level C stocks (Mg C ha−1) in live aboveground biomass (AGB), live belowground biomass (BGB), standing dead mass, coarse woody debris (CWD) and litter of rangeland vegetation at 46 long-term (average 40 year) grazing trials comprising grazed control plots paired with exclosures protected from grazers. Four major woody vegetation types were considered, namely, those dominated by Eucalyptus species, Acacia aneura, other Acacia species, and other species. Most C stocks (except litter C) were highest in Eucalyptus-dominated vegetation and lowest in the ‘other species’ types. There was high variability and few significant grazing effects on C stocks. There was a trend of higher AGB-C, BGB-C, woody basal area (m2 ha−1) and litter C with grazing exclusion at sites with mean annual precipitation of >300 mm, but the magnitude of increase was highly variable. The impact of the type of grazer was highly variable, although there was a trend of higher woody biomass C where rabbits and domestic livestock were excluded than with exclusion of domestic livestock only. The relative effects of grazing declined as the woody basal area of grazed control plots increased. Our results showed that although grazing exclusion can facilitate C stock accumulation in woody vegetation, it is difficult to predict when and where this may occur. To enable greater clarity, future studies may need to collect additional information, including data on soil water availability and current and historical grazing intensity.
Keywords: Acacia aneura, carbon sequestration, coarse woody debris, disturbance, eucalypts, grazing management, litter, livestock, macropod, rabbit control, rangeland ecology, rangeland management.
Introduction
Rangelands cover about 80% of Australia’s land area and support a multi-billion dollar grazing industry (NRMMC 2010). Total grazing pressure by native, feral and/or domestic animals in Australian rangelands can influence multiple ecosystem functions and services, including organic carbon (C) stocks for live biomass, litter, and soil, species diversity, soil water infiltration or water holding capacity, diversity or cover of herbaceous species and grasses and grazing land condition (Shaw et al. 2007; Fensham et al. 2011; Witt et al. 2011; Daryanto et al. 2013; Pringle et al. 2014; Eldridge et al. 2018; Beutel et al. 2021; Henry 2023). There is growing interest from policy makers, landowners and scientists in quantifying woody biomass and C stocks in rangelands and how they are influenced by grazing. However, there is insufficient information to predict the influence of domestic grazing on woody biomass or C stocks across Australia’s diverse rangelands.
Woody vegetation cover and hence aboveground C stocks in rangelands is influenced by rainfall (Friedel 1997; Witt et al. 2009a; Fensham et al. 2012), fire (Daly and Hodgkinson 1996; Beringer et al. 2007; Cowley et al. 2014; Hunt 2014), mechanical disturbances including clearing, thinning and fodder harvesting (Beale 1973; Back et al. 2009; Witt et al. 2009a), and grazing (Witt et al. 2009b; Witt et al. 2011; Daryanto et al. 2013; Bastin et al. 2024; Retallack et al. 2024). These influences can be nuanced according to the season, site and landscape context. For example, at regional scale in central Queensland, grazing was found to have a minor influence on woody vegetation cover between the 1940s and 1990s (Fensham et al. 2005). Instead, cover change was largely attributed to extremes in rainfall relative to the long-term average and stand density, whereas fire and grazing had smaller or even negligible influences (Fensham et al. 2005).
Even if the overall effect of grazing across a landscape is small, effects might be concentrated at specific sites and at particular times of year (Witt 2013). In this context, controlled grazing experiments where plots containing different treatments are established on the same site, thereby controlling for differences in vegetation, soil type and climate, can be used to better understand the local impacts of grazing. The few studies utilising controlled grazing experiments in Australian rangelands to examine the impacts of grazing on woody aboveground C stocks have shown variable results, including decreases (Witt et al. 2011; Daryanto et al. 2013), increases (Witt et al. 2009b) or no changes in live aboveground biomass and C stocks (Foran et al. 1982; Bray et al. 2014).
The effects of grazing or browsing will depend on the grazer species and the vegetation type. Domestic cattle or sheep, feral rabbits and macropods eat large amounts of green annual grasses, ephemeral forbs, and green foliage of perennial grasses (DSEWPC 2011; Pahl 2019). However, this overlap in preferences changes as these sources become less abundant (Wilson 1991; Pahl 2019). For example, as the abundance of green annual grasses, ephemeral forbs, and green foliage of perennial grasses declines, sheep, cattle and goats may consume more mature perennial grasses, chenopods and non-chenopod perennial forbs (Pahl 2019). Macropods may continue to graze mature perennial grasses for longer than sheep, cattle and goats, and graze perennial forbs only when the quantity and quality of perennial grasses is too low (Pahl 2019). Some dominant rangeland tree species, such as Acacia aneura (Mulga), are reasonably palatable to domestic animals, and branches are lopped, or trees are pushed or pulled down, by land managers to feed livestock during periods of drought (Witt 2013).
In Australia, the distribution of grazing animal species varies across the continent. Macropods are found across most of Australia, but their population densities decline when predators are present (e.g. Canis lupus, dingo) or environmental conditions become harsher (Pople et al. 2000). Rabbits are absent from the northern parts of Western Australia and Queensland, and most of the Northern Territory (DSEWPC 2011). Cattle and sheep are widely distributed, but both are almost absent from desert areas, particularly in areas distant from watering points, and sheep population densities are generally higher in the southern part of the continent, whereas cattle population densities are higher in the northern rangelands (Harper et al. 2019; MLA 2021a, 2021b). The grazing animal species that are present, and their population densities, will potentially influence the grazing effects observed.
This study focused on the influence of rangeland grazing on C stored in live woody vegetation (above- and belowground biomass C), and the C stocks into which this live biomass C flows, including standing dead C, coarse woody debris (CWD) C and litter C. The specific objectives were to utilise existing long-term grazing experiments that could be located across the Australian rangelands (n = 46) to
(1) accurately quantify woody C stocks by major vegetation type and examine whether grazing had a significant influence on C stocks, and if so,
(2) determine whether this grazing impact varied among vegetation types, grazing animal species/species combinations, site climatic characteristics, and time since exclosure.
Methods
Study sites
The study assessed 46 existing grazing experiments that could be located across Australia’s rangelands (Fig. 1, Table 1). These experiments were identified using a combination of literature review and extensive consultation with land managers and scientists involved in their establishment and management. The experiments were mostly paired grazed control plots and fenced exclosures to prevent grazing by specific animals, but a subset of six experiments included paired plots with rotational grazing treatments and exclosures with control plots of continuous grazing (Table 1). The experiments had been maintained for 9–98 years (mean of approximately 40 years) at the time of measurement. For each of the exclosure plots, any breaches in the fence were recorded. Where grazed control plots had not previously been established for grazing exclosures, new plots with a known grazing history were located immediately adjacent to the exclosure area for measurement.
Locations of the study sites and associated vegetation types. The distribution of rangelands was obtained from DCCEEW (2023). Note that the ‘non-rangeland site’ was included because it had vegetation and climate considered representative of Eucalyptus rangelands.
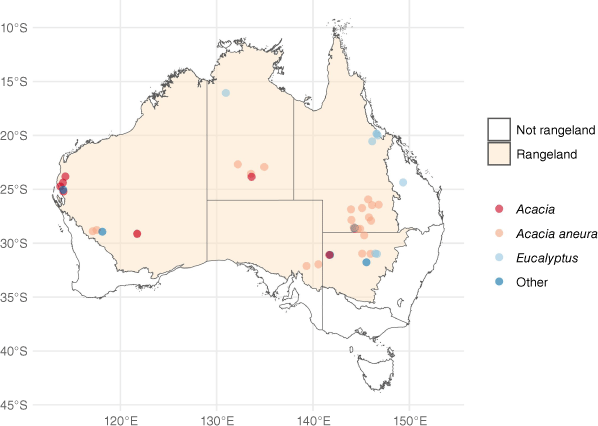
Site name (State or Territory) | Latitude | Longitude | Year exclosure established | Mean annual rainfall (mm) | Plot size (ha) | CWD/litter measured | Vegetation type | Treatments (control refers to grazed plots that are unfenced) | |
---|---|---|---|---|---|---|---|---|---|
1 (WA) | −24.73 | 113.76 | 1988 | 203 | 0.49–0.5 | ✓/✓ | Acacia | (i) Control; (ii) excluded livestock and rabbits. Not replicated. | |
2 (WA) A | −24.39 | 114.05 | 1986 | 213 | 0.47–0.5 | ✓/✓ | Acacia | (i) Control; (ii) excluded livestock and rabbits. Two replicates. | |
Ella Valla (WA) | −25.20 | 114.10 | 1986 | 195 | 0.49–0.5 | ✓/✓ | Acacia | (i) Control; (ii) excluded livestock and rabbits. Not replicated. | |
Fowlers Gap-Emu (NSW) | −31.08 | 141.70 | 1975 | 238 | 15.41 | ✓/✓ | Acacia | (i) Control; (ii) excluded sheep + macropods. Not replicated. | |
Goorninya East (Qld) | −28.66 | 144.37 | 2014 | 234 | 0.06 | ✓/– | Acacia | (i) Control; (ii) excluded livestock; (iii) excluded livestock + macropods. No replication, but two grazing control plots. | |
Old Mans Plains (NT) | −23.84 | 133.62 | 1981 | 290 | 1 | ✓/✓ | Acacia | (i) Control; (ii) excluded livestock + rabbits. Seven replicates. | |
3 (WA) A | −29.13 | 121.74 | 1988 | 239 | 0.48–0.5 | ✓/✓ | Acacia | (i) Control; (ii) excluded livestock. Not replicated. | |
4 (WA) | −23.81 | 114.29 | 1986 | 230 | 0.5 | ✓/✓ | Acacia | (i) Control; (ii) excluded livestock and rabbits. Not replicated. | |
5 (Qld) A | −27.91 | 146.04 | 1995 | 356 | 0.28–0.3 | –/– | Acacia aneura | (i) Control; (ii) excluded sheep, goats + macropods; (iii) rotational grazing while excluding macropods. Not replicated. | |
6 (Qld) A | −26.43 | 146.82 | 1996 | 462 | 0.32–0.33 | ✓/✓ | Acacia aneura | (i) Control; (ii) excluded sheep + macropods + rabbits; (iii) rotational grazing while excluding macropods. Not replicated. | |
7 (Qld) | −27.85 | 143.99 | 1995 | 270 | 0.29–0.30 | ✓/✓ | Acacia aneura | (i) Control; (ii) excluded cattle + macropods; (iii) excluded cattle + macropods + rabbits. Not replicated but two control plots. | |
8 (SA) | −31.96 | 140.55 | 2010 | 233 | 0.50–1.67 | ✓/✓ | Acacia aneura | (i) Control; (ii) excluded livestock + macropods; (iii) excluded livestock + rabbits; (iv) excluded livestock + macropods + rabbits. Treatments (i) and (iv) replicated three times. | |
Boorara (Qld) | −28.69 | 144.28 | 1993 | 290 | 0.04–0.10 | ✓/– | Acacia aneura | (i) Control; (ii) excluded sheep, cattle, goats; (iii) excluded sheep, cattle, goats + macropods. Three replicates, except for an unreplicated treatment (ii). | |
9 (NT) | −22.68 | 132.20 | 1968 | 321 | 0.45–1.03 | ✓/✓ | Acacia aneura | (i) Control; (ii) excluded cattle. Not replicated. | |
10 (WA) A | −28.77 | 117.55 | 2008 | 270 | 0.25–1.00 | –/✓ | Acacia aneura | (i) Control; (ii) excluded cattle and goats. Three replicates. | |
Croxdale (Qld) A | −26.46 | 146.13 | 1981 | 455 | 0.25 | ✓/– | Acacia aneura | (i) Control; (ii) excluded livestock; (iii) excluded livestock + macropods. No replication. Grazed control established in 2020. | |
11 (WA) | −29.13 | 121.74 | 1988 | 239 | 0.56–0.63 | –/✓ | Acacia aneura | (i) Control; (ii) excluded livestock. Not replicated. | |
Glenvue (NSW) | −30.98 | 145.97 | 1996 | 384 | 0.3 | –/– | Acacia aneura | (i) Control (continuous grazing); (ii) control (rotational grazing); (ii) excluded sheep + macropods + rabbits. Not replicated. | |
Hamilton Downs (NT) | −23.56 | 133.57 | 1970 | 322 | 2.24 | ✓/✓ | Acacia aneura | (i) Control; (ii) excluded livestock + macropods. Not replicated. | |
12 (SA) | −32.11 | 139.33 | 1925 | 208 | 3.0–3.2 | –/– | Acacia aneura | (i) Control; (ii) excluded livestock + rabbits. Not replicated. Rabbits excluded since 1946. | |
Kyabra (Qld) | −28.62 | 144.40 | 2014 | 236 | 0.06 | ✓/– | Acacia aneura | (i) Control; (ii) excluded livestock; (iii) excluded livestock + macropods. No replication, but two grazing control plots. | |
13 (Qld) | −26.74 | 145.09 | 1984 | 366 | 0.23–0.25 | ✓/– | Acacia aneura | (i) Control; (ii) excluded livestock + macropods + rabbits. No replication, but two grazing control plots. | |
14 (Qld) A | −26.85 | 143.94 | 1995 | 309 | 0.31 | –/– | Acacia aneura | (i) Control; (ii) rotational grazing; (iii) excluded livestock + macropods + rabbits. No replication. | |
Mount Riddock (NT) | −22.92 | 134.94 | 1968 | 293 | 1.2–3.8 | ✓/– | Acacia aneura | (i) Control; (ii) excluded livestock. Two replicates. | |
Paradise (Qld) | −28.66 | 144.65 | 2014 | 242 | 0.06 | ✓/– | Acacia aneura | (i) Control; (ii) excluded livestock; (iii) excluded livestock + macropods. Two replicates, but only one plot for (iii). | |
Tolee (Qld) | −28.53 | 144.24 | 2014 | 228 | 0.06 | ✓/– | Acacia aneura | (i) Control; (ii) excluded livestock; (iii) excluded livestock + macropods. No replication, but two control plots. | |
15 (NSW) A | −30.96 | 145.10 | 1978 | 336 | 0.25 | ✓/– | Acacia aneura | (i) Control; (ii) excluded livestock. Two replicates. | |
16 (Qld) | −25.94 | 145.72 | 1964 | 468 | 0.25 | ✓/– | Acacia aneura | (i) Control; (ii) excluded livestock + macropods + rabbits. No replication. | |
17 – 1983 (Qld) | −27.60 | 145.80 | 1983 | 383 | 0.20–0.22 | ✓/✓ | Acacia aneura | (i) Control; (ii) excluded sheep; (ii) excluded sheep + rabbits. No replication. | |
18–1996 (Qld) | −27.66 | 145.77 | 1996 | 375 | 0.3 | ✓/✓ | Acacia aneura | (i) Control; (ii) excluded sheep; (ii) excluded sheep + macropods + rabbits. No replication. | |
19 (NSW) | −29.26 | 145.32 | 1996 | 307 | 0.13–0.99 | ✓/✓ | Acacia aneura | (i) Control; (ii) control (rotational grazing); (iii) excluded sheep, cattle (ploughed); (iv) excluded sheep, cattle (unploughed); three replicates (except for rotation grazing). In one replicate, macropods were also excluded from the exclosure. | |
Werai (Qld) | −28.66 | 144.90 | 2014 | 246 | 0.16 | ✓/– | Acacia aneura | (i) Control; (ii) excluded livestock + macropods. Not replicated. | |
20 (WA) | −28.89 | 117.13 | 2008 | 244 | 0.25–1.00 | –/✓ | Acacia aneura | (i) Control; (ii) excluded cattle and goats. Three replicates. | |
21 (NT) | −16.06 | 130.96 | 1973 | 789 | 6.76, 15.3 & 22.9 | –/✓ | Eucalyptus | (i) Control; (ii) excluded cattle. Three replicates. | |
22 (Qld) | −20.01 | 146.73 | 1985 | 658 | 0.2 | ✓/– | Eucalyptus | (i) Control; (ii) excluded cattle + macropods + rabbits. No replication. | |
23 (Qld) | −19.84 | 146.59 | 1950 | 691 | 0.24–0.8 | ✓/– | Eucalyptus | (i) Control; (ii) excluded livestock + rabbits; (iii) excluded livestock + rabbits + macropods. No replication. | |
Oaklands (Qld) | −24.36 | 149.36 | 2012 | 582 | 0.23–1.04 | ✓/✓ | Eucalyptus | (i) Control; (ii) excluded livestock during the wet season. Three replicates. | |
24 (NSW) | −30.95 | 146.46 | 1975 | 409 | 0.77–0.90 | ✓/✓ | Eucalyptus | (i) Control; (ii) excluded sheep, cattle, goats, pigs. Two replicates. | |
25 (Qld) | −20.55 | 146.15 | 1997 | 597 | 1 | –/✓ | Eucalyptus | (i) Control; (ii) excluded livestock. Eight plots of (i) and two of (ii). | |
26 (NSW) | −30.99 | 146.65 | 1996 | 410 | 0.28–0.31 | ✓/✓ | Eucalyptus | (i) Control (continous grazing); (ii) control (rotational grazing); (iii) excluded sheep, cattle. No replication. In one replicate, macropods were also excluded in the exclosure. | |
27 (NSW) | −31.77 | 145.56 | 1950 | 366 | 0.31 | –/– | Other | (i) Control; (ii) excluded livestock + macropods + rabbits. Not replicated. | |
Fowlers Gap (NSW) | −31.08 | 141.76 | 1954 | 229 | 0.79–2.8 | ✓/✓ | Other | (i) Control; (ii) excluded sheep; (iii) excluded sheep + macropods (established 2016). Not replicated. | |
Goorninya West (Qld) | −28.61 | 144.30 | 2014 | 233 | 0.06 | ✓/– | Other | (i) Control; (ii) excluded livestock; (iii) excluded livestock + macropods. No replication, but two grazing control plots. | |
Narndee (WA) A | −28.94 | 118.14 | 1986 | 250 | 0.29–0.30 | ✓/✓ | Other | (i) Control; (ii) excluded livestock and rabbits. Not replicated. | |
Tareen (Qld) | −28.56 | 144.32 | 2014 | 228 | 0.06 | ✓/– | Other | (i) Control; (ii) excluded livestock; (iii) excluded livestock + macropods. No replication, but two control plots. | |
28 (WA) | −25.04 | 114.09 | 1984 | 203 | 0.45–0.48 | ✓/✓ | Other | (i) Control; (ii) excluded livestock and rabbits. Not replicated. |
NSW, New South Wales; NT, Northern Territory; Qld, Queensland; SA, South Australia; WA, Western Australia.
Mean annual rainfall from the year of establishment until the year of measurement.
Site names given as numbers for confidentiality reasons.
Within the experimental sites, four main vegetation types were defined as dominated by Eucalyptus species, Acacia aneura, other Acacia species (possibly including, but not dominated by A. aneura), and other species.
Data collection
Carbon stocks in live aboveground biomass (AGB), belowground biomass (BGB) and standing dead biomass of trees and shrubs were determined at all sites, and C stocks in CWD and litter were measured at a subset of sites (n = 36 and 26 respectively).
Plot measurements were made between July 2022 and November 2023. Generally, the entire plot area was measured, except trial sites with plots of >1 ha. For these larger plots, live biomass and standing dead C stocks were measured using transects (100 m × 5 m), with the number of transects measured increasing with the variability of the soil and/or vegetation. Within each plot/transect, stem diameters of Eucalyptus, ‘Other trees’, and large Acacia trees were measured at 1.3 m height, whereas stem diameters of all other woody vegetation, including shrubs, mallees and multi-stemmed acacias, were measured at 0.1 m height. In plots/transects with high frequencies of small plants (<10 cm diameter at 1.3 m), counts of plants in each functional type (live and dead plants) in 2-cm diameter classes were taken instead of measuring the diameter of all small plants. AGB of individual woody plants (AGBi) and live BGB of individual woody plants (BGBi) were determined using allometric equations developed for these plant functional types (Paul et al. 2016, 2019). Standing dead AGBi and BGBi were determined using allometric equations developed for these plant functional types (Paul and Roxburgh 2024).
For senescing and senesced trees with stem diameters (at 1.3 m) of >55 cm, individual tree biomass estimates were reduced by multiplying the fraction remaining of aboveground structure by the biomass based on allometric relationships developed for Australian rangelands (M. Piper, pers. comm., January 2023). Specifically, dead plants were given a score that quantifies the remaining proportion of biomass relative to a live plant, as follows: 1, if it still has small branches in the crown; 0.8, if it has only large branches in the crown; 0.6, if only the main stem and few large branches remain; 0.4, if only the main bole is remaining or most of stem height remains; and 0.2, if more than half the height of the main stem is missing (M. Piper, pers. comm., January 2023).
Stand-level live AGB, BGB and standing dead mass (Mg dry matter (DM) ha−1) were calculated by summing all individual live and dead biomass estimates respectively, per plot and dividing by the plot area. Stand-level basal area was calculated by summing the basal area of each tree and dividing it by the plot area. Since the height at which stem diameters were measured depended on plant function types, allometric equations (Paul et al. 2016) were used to calculate a diameter at 0.1 and 1.3 m for each tree, and hence stand basal area values for 0.1 and 1.3 m were calculated for each plot. Unless otherwise stated, stand basal area values are provided for a height of 0.1 m.
At the 26 sites where litter was sampled, at least 24 litter samples were collected from random locations within each plot/transect by using 0.0625 or 0.25 m2 quadrats. All material with a diameter of <25 mm was collected, and all samples were combined into a single sample for each plot. Litter was sorted into foliage or bark/twigs/capsules from woody plants, discarding any grass and soil present in the samples. Litter was then oven dried at 70°C to a constant weight and weighed. Litter stocks were calculated in Mg DM ha−1 for each sample from oven-dry litter mass and quadrat area.
CWD was measured at 36 sites by using an approach based on the line intersect method (van Wagner 1968) (Eqn 1). In plots where CWD was measured, the sum of transect lengths was at least 300 m, and CWD was defined as all woody material with a diameter of >25 mm where it crossed the transect line.
where d is the diameter (cm) of each piece of CWD where it crossed the transect, l is the length of the transect (m), g is the weighted average (by piece diameter) of the proportion of wood remaining, and ρ is the wood density (oven dry mass per pre-dried volume, Mg m−3). The ρ used was 0.55, which was the average of many studies from Australian ecosystems (Kingston and Risdon 1961; Attiwill 1979; Stewart et al. 1979; Gifford 2000; Ilic et al. 2000; Raymond and Muneri 2000; Miranda et al. 2001; Raymond and Muneri 2001; Slijepcevic 2001; Bridges 2005; Searle and Owen 2005; Ximenes et al. 2008; Callister and England 2010; Sillett et al. 2010; Ximenes et al. 2016; Falster et al. 2021). Dry-mass values were converted to carbon mass by multiplying live or standing dead mass by 0.5 (Gifford 2000; Mathers et al. 2003; Possell et al. 2015; Neumann et al. 2020), CWD by 0.49 (Stewart and Burrows 1994; Chee 1999; Woldendorp 2000; Harms and Dalal 2003; Roxburgh et al. 2006b; Hilbig 2009; Volkova and Weston 2013; Aponte et al. 2014; Possell et al. 2015), and litter by 0.51 (Hutson 1985; Chee 1999; Gifford 2000; Woldendorp 2000; Russell-Smith et al. 2009; Possell et al. 2015; Volkova and Weston 2015).
Climatic data for each site were obtained from Queensland Government’s Silo database (www.longpaddock.qld.gov.au/silo/) by using the R package cropgrowdays (ver. 0.2.0.; see https://CRAN.R-project.org/package=cropgrowdays) and R software (R Core Team 2023). Daily precipitation, minimum and maximum temperatures, and evapotranspiration were used to calculate monthly and annual values. For example, the mean annual precipitation (MAP) for each site was derived by taking the sum of all the annual values since the exclosure was established, divided by the number of years since the exclosure was established. Experimental sites were initially defined as arid (≤300 mm MAP) or non-arid >300 mm MAP. In Australia, arid zones are often defined as areas with ≤250 mm MAP (DCCEEW 2024); therefore, this was used as an additional alternative cut-off for arid and non-arid zones in subsequent analyses. Further, an aridity index defined by Eamus et al. (2000) was also derived for each experimental site. This index was the ratio of monthly mean daily pan evaporation rate to monthly rainfall. When monthly rainfall was zero, a value of 0.1% of annual rainfall was used for that month to avoid division by zero.
Statistical analysis
Differences among vegetation types or between grazed and control treatment means were examined using linear mixed models. A natural logarithmic transformation was applied to each C stock so that the residuals were centred at zero and approximately normally distributed. Treatment effects were examined when combining data, as well as splitting the data into the four vegetation types (Eucalyptus species, Acacia aneura, other Acacia species and Other), and/or into different regions (e.g. Queensland, New South Wales, Northern Territory, Western Australia, South Australia, and groups of these), and into the individual sites that contained replication. This was performed while including all data within these groups, as well as after excluding sites where exclosures had been breached at some point in time since establishment, and when dividing the dataset between arid and non-arid MAP. When testing for differences between treatments at individual sites that contained replication, the replicate was used as a random effect. These were fitted using the R package nlme (ver. 3; see https://CRAN.R-project.org/package=nlme; Pinheiro et al. 2018) and R software (R Core Team 2023).
For the total dataset, linear mixed models were used to test for correlations between C stocks and climatic variables, or the time since exclosure. ‘Site’ was included as a random effect. Linear mixed models were also used to test whether the relative difference between the C stock of a given exclosure treatment, and the grazed control plot at the same site, was correlated with climate variables, the time since exclosure or stand basal area of the grazed control plots.
To complement the mixed models, a non-parametric test was used to test whether a given exclosure treatment differed from the grazed plots. Using the available pairs of exclosure plots and grazed plots at each site or replicate, the observed test statistic was calculated as the proportion of pairs where the C stock of the exclosure was greater than that of the grazed plot. The null hypothesis was that there was no difference between the exclosures and grazed plots. The observed test statistic was compared with the proportion of plots where the exclosure C stocks were greater than the grazed plots after randomly assigning the treatment (exclosure or grazed) to each plot. This was repeated 100,000 times to calculate the probability of the observed test statistic being greater or smaller (i.e. two-tailed test) than the statistic calculated after randomly assigning the treatments, which was a distribution of probabilities centred on 0.5. As for the mixed models, this test was performed when combining data, as well after splitting the data into groups relating to vegetation type and region.
We also performed a meta-analysis on the effects of grazing exclusion using the R packages metafor (ver. 4.6-0; see https://CRAN.R-project.org/package=metafor; Viechtbauer 2010) and foresplot (ver. 3.1.3; see https://gforge.se/packages/url), following the methods described by Borenstein et al. (2009). R codes for the meta-analysis were based on those described in Zhang et al. (2020). The control mean (Cmean), treatment mean (Tmean), their corresponding standard deviations (Csd and Tsd), and sample size (n) were calculated and are provided in the Supplementary material (Tables S13-S26). Overall, 27 of the 46 grazing sites were unreplicated in the grazed and/or exclosure treatments. For treatments without replicates, the biomass-C or basal area was multiplied by the average coefficient of variation derived from the rest of the dataset for the parameter where standard deviations were available (van Groenigen et al. 2011). We used multilevel random effect meta-analysis to account for variable dependencies, such as when an experiment had rotation and exclusion treatments that shared a set of common controls. The statistical analysis was conducted on natural log-transformed response ratios (ln RR), where the ratio was the ungrazed exclosure mean divided by the grazed control mean. The effect sizes were back-transformed from ln RR to response ratio on the natural scale (RR) to aid interpretation. Treatment was considered to represent a significant (P < 0.05) increase or decrease compared with the control when the 95% CI of the response variable (i.e. AGB-C, BGB-C, basal area, CWD-C or litter C) did not overlap with zero.
Results
C stocks in relation to vegetation type and climate
Most C stocks (except litter C) were significantly higher in the Eucalyptus plots than in the ‘other’ plots (Fig. 2). Biomass C stocks were positively correlated with mean annual precipitation (Supplementary Fig. S1) and potential evaporation (Fig. S3), but not correlated with mean maximum temperature in January (Fig. S2), usually the hottest month. CWD C and litter C followed the same trends. The low pseudo-R2 (mR2) but relatively high conditional pseudo-R2 (cR2) suggests that much of the variability in C stocks was explained by the variability within sites rather than the differences in climate among sites (Figs S1, S3).
Box plots comparing (a–e) the C stocks and (f) basal areas (at height of 0.1 m) of different vegetation types when combining data from exclosures and grazed control plots. The y-axes are ln-transformed. Different letters indicate significant differences between individual vegetation types (P < 0.05). AGB, aboveground biomass; BGB, belowground biomass.
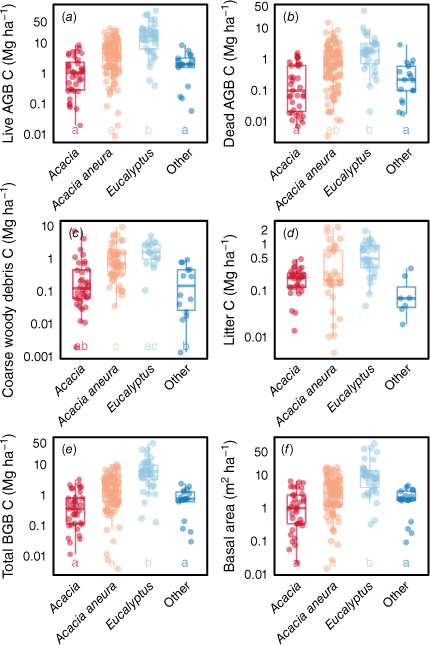
Influence of domestic grazers on C stocks
There were no significant grazing effects on C stocks when combining all vegetation types (Fig. 3), or when considering individual vegetation types (Supplementary Table S12). Nor were there any significant differences between treatments at individual sites that contained replication (except for litter C stocks at Site 19, and several stocks at Site 10, Table S12). Similarly, there were no significant differences when focusing on individual vegetation types within a region, such as A. aneura in Queensland + New South Wales (except for litter C stocks), or Acacia and A. aneura in the Northern Territory (Table S12). Whether the grazers were cattle, sheep or combinations of these also had no significant effect (Table S12). The treatment effects were also not changed by excluding all sites where exclosures had been compromised at some point during the experimental period (Table S12). The non-parametric tests were usually consistent with the results from the mixed models (Table S12).
Box plots comparing (a–e) the C stocks and (f) basal areas (at height of 0.1 m) of exclosures and grazed control plots. The y-axes are ln-transformed. AGB, aboveground biomass; BGB, belowground biomass.
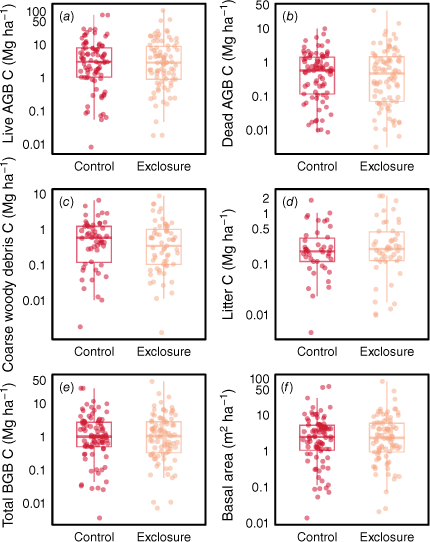
Results from the meta-analysis showed high variability and low frequency of significant grazing effects on biomass parameters across the vegetation types (Fig. 4, Table S1). Although mean response ratios were often >1, indicating that grazing exclusion increased the response variable relative to the grazed control treatment, owing to the high variability among grazing response observations, the 95% confidence intervals for response ratios generally included one for AGB-C, BGB-C and CWD-C, whether considering each vegetation type, or averaged across vegetation types. Basal area was higher, on average between 1.08 and 1.59 times, with grazing exclusion, but a consistent increase in basal area with grazing exclusion was observed only in A. aneura-dominated vegetation. However, there was some indication of a consistent trend whereby the mean response ratio in Eucalyptus-dominated vegetation was more positive with grazing exclusion than in the other vegetation types for AGB-C and BGB-C. Total litter C increased on average between 1.16 and 1.96 times with grazing exclusion. The response was largest within Acacia aneura-dominated vegetation, with litter C increasing between 1.27 and 3.08 times with grazing exclusion. When excluding plots from the analysis where exclosure fences had some damage, the trends remained similar.
The impact of grazing exclusion on the response variables aboveground biomass carbon (AGB-C), belowground biomass carbon (BGB-C), basal area (at height of 0.1 m), coarse woody debris carbon (CWD-C) and litter C by vegetation type. Blue lines represent the 95% confidence intervals. Red lines show the 95% confidence intervals for the overall effect of exclosure on the response variables. A response ratio of one indicates that grazing exclusion did not affect the response variable measured, whereas a ratio greater than one indicates that grazing exclusion increased the response variable relative to the grazed control treatment. The I2 statistic estimates the amount of variability in effect size that can be attributed to heterogeneity among true effects (genuine variation among experiments). I2 indices of zero indicate that the variability within experimental trials was high.
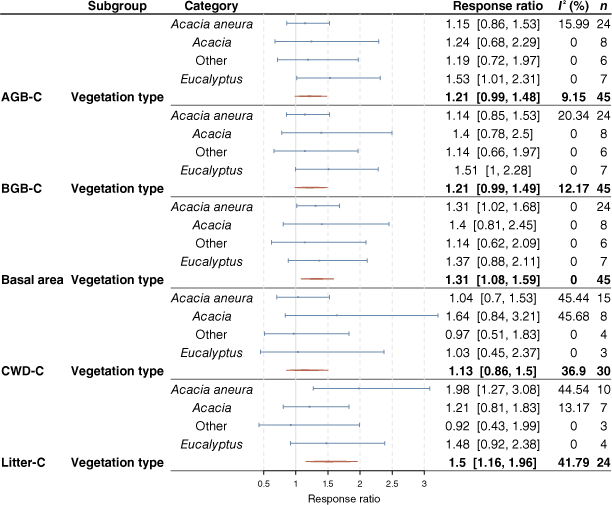
In non-arid regions (MAP > 300 mm), AGB-C, BGB-C, basal area and litter C stocks were consistently higher where grazing was excluded (Fig. 5, Table S4). The 95% CIs were generally large. When the impact of grazing exclusion was evaluated using 250 mm as a cut-off for defining aridity, these trends were generally weaker or no longer observed (Fig. S6, Table S5). However, the impact of grazing exclusion evaluated using an aridity index defined by Eamus et al. (2000) found similar trends to the 300 mm precipitation cut-off (Fig. S7, Table S6).
The impact of grazing exclusion on aboveground biomass carbon (AGB-C), belowground biomass C (BGB-C), basal area (at height of 0.1 m), coarse woody debris C (CWD-C) and litter C by arid and non-arid regions as defined by mean annual precipitation above and below 300 mm. Blue lines represent the 95% confidence intervals. Red lines show the 95% confidence interval for the overall effect of exclosure on the response variable. A response ratio of one indicates that grazing exclusion did not affect the response variable measured, whereas a ratio greater than one indicates that grazing exclusion increased the response variable relative to the grazed control treatment. The I2 statistic estimates the amount of variability in effect size that can be attributed to heterogeneity among true effects (genuine variation among experiments). I2 indices of zero indicate that the variability within experimental trials was high.
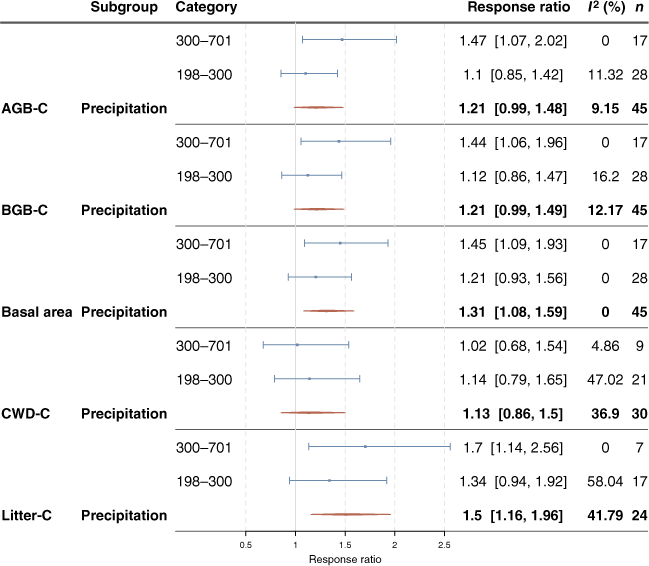
Across the total dataset, relative differences between treatments were highly variable (Fig. 6). As implied by the low frequency of significant effects mentioned above, most relative differences were close to zero or centred around zero; however, there were many pairs (counts provided in Fig. 6) with relative differences >0 (exclosure C stock > grazed C stocks). These relative differences in C stocks were not correlated with any climate variables, or the duration of the experiment on the basis of linear mixed modelling (P > 0.05, data not shown). However, the relative differences in live C stocks declined as the basal area of the grazed control plots increased (Fig. S4).
Frequency distributions showing the relative differences between ungrazed plots and grazed plots of (a–e) C stocks or (f) basal areas (at height of 0.1 m), i.e. (Cstockungrazed – Cstockgrazed) / Cstockgrazed. For sites with multiple exclosure treatments, each treatment is considered (not the average from each site). The legends show the number of ungrazed plots where relative differences were either >0 or <0. AGB, aboveground biomass; BGB, belowground biomass; CWD, coarse woody debris.
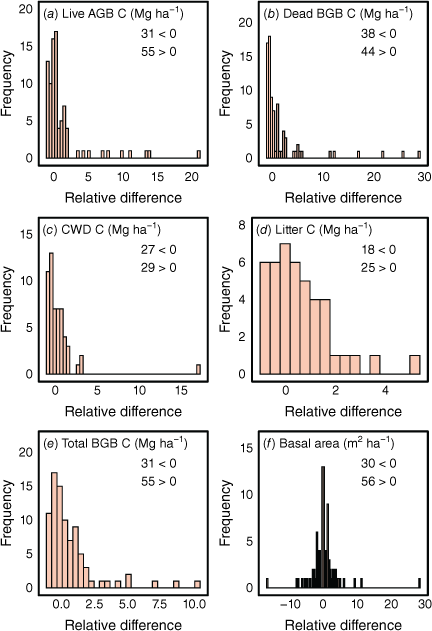
Additional random effect meta-regression was used to assess whether the effect sizes of grazing exclusion on C stocks were correlated with climate variables (mean annual precipitation, mean maximum temperature in January, mean annual evaporation), the duration of the experiment or latitude. These results were generally in agreement with the linear mixed modelling. The 95% CIs of the response gradients or slopes of the regression were generally large and were a mix of negatives on the lower limit and positives on the upper limit (Figs S7–S11, Tables S7–S11), indicating that there were no clear linear relationships between vegetation response to grazing exclusion and climatic variables, latitude and age of the experiment within the ranges we have investigated. The exceptions were for AGB-C and BGB-C in response to age of experiment (Fig. S10, Table S9). For every additional year of experiment, total AGB-C increased in response to grazing exclosure with the response gradient of 0.013 [95% CI: 0.003, 0.023], corresponding to a response ratio of 1.013 [1.003, 1.023]. This equates to a mean effect of between ~0.3% and 2% increase in AGB-C with every year of grazing exclusion. For every additional year of experiment, BGB-C increased in response to grazing exclosure with the response gradient of 0.014 [0.004, 0.023], corresponding to response ratio of 1.014 [1.004, 1.024] or a mean effect of between 0.4% and 2.4% increase in BGB-C with every additional year of experiment. The trends remained the same after excluding the experimental plots with damaged fences from the meta-regression analyses (data not shown).
Influence of domestic grazers and rabbits or macropods on C stocks
Across the total dataset, although treatments where rabbits and domestic livestock were excluded often had higher mean live AGB-C, BGB-C and CWD-C stocks than did domestic livestock exclusion alone, increases were significant only for basal area (Fig. 7). Exclosures where rabbits were excluded (in addition to domestic grazers) had significantly higher live above- and belowground C at the Old Mans Plains site, and for the Acacia vegetation type (Table S12). There were no clear trends when considering other vegetation types or states (e.g. Western Australia, Queensland) individually (Table S12). At the Boorara site, exclosures where domestic animals + macropods were removed had lower live above- and belowground C stocks than when only domestic animals were excluded. For the Eucalyptus vegetation type, basal area was highest in exclosures where domestic animals + macropods were excluded. There were no significant effects of excluding domestic animals and macropods (Fig. 8). These results are consistent with the meta-analysis results (Fig. 9, Table S2). When the types of grazers excluded were considered, grazing exclusion significantly affected only litter C and basal area. Specifically, litter accumulation was seen in experimental sites where only domestic animals were excluded (1.41–2.34). Basal area was highly variable but was on average between 1.05 and 3.66 times higher with grazing exclusion when domestic animals, macropods and rabbits were excluded, but this consistent positive trend was lost when we excluded exclosures that had been compromised at some point during the experimental period (data not presented). The trends remained similar for the rest of the variables when we excluded plots where the exclosure that had been compromised at some point during the experimental period.
Box plots comparing (a–e) the C stocks and (f) basal area (at height of 0.1 m) of grazed control plots and exclosures with or without rabbits. The y-axes are ln-transformed. Letters indicate significant differences between grazing and exclosure treatments. AGB, aboveground biomass; BGB, belowground biomass; Dom exc, domestic animals excluded; Dom + rabbits exc, domestic animals and rabbits excluded.
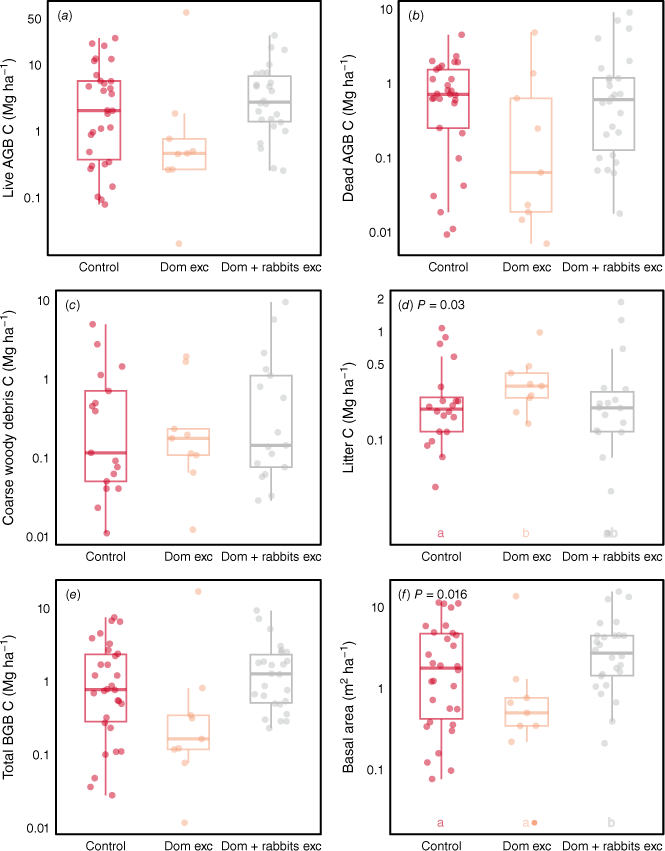
Box plots comparing (a–e) the C stocks and (f) basal area (at height of 0.1 m) of grazed control plots and exclosures with or without macropods. The y-axes are ln-transformed. Letters indicate significant differences between grazing and exclosure treatments. AGB, aboveground biomass; BGB, belowground biomass; Dom exc, domestic animals excluded; Dom + macropods exc, domestic animals and macropods excluded.
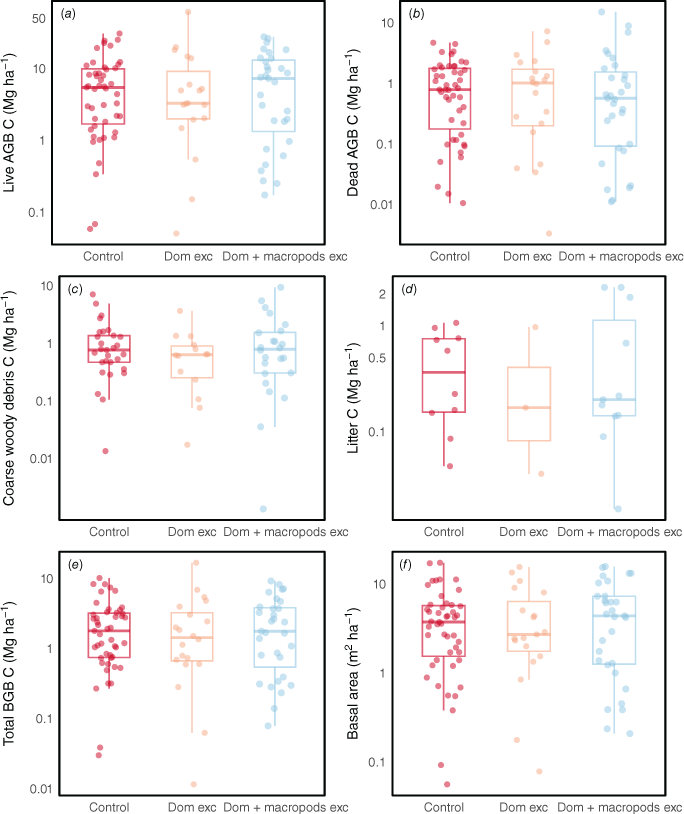
The impact of grazing exclusion on aboveground biomass C (AGB-C), belowground biomass C (BGB-C), basal area (at height of 0.1 m), coarse woody debris C (CWD-C) and litter C by the type of grazer excluded. Blue lines represent the 95% confidence intervals. Red lines show the 95% confidence interval for the overall effect of exclosure on the response variable. A response ratio of one indicates that grazing exclusion did not affect the response variable measured, whereas a ratio greater than one indicates that grazing exclusion increased the response variable relative to the grazed control treatment. The I2 statistic estimates the amount of variability in effect size that can be attributed to heterogeneity among true effects (genuine variation between experiments). I2 indices of zero indicate that the variability within experimental trials was high.
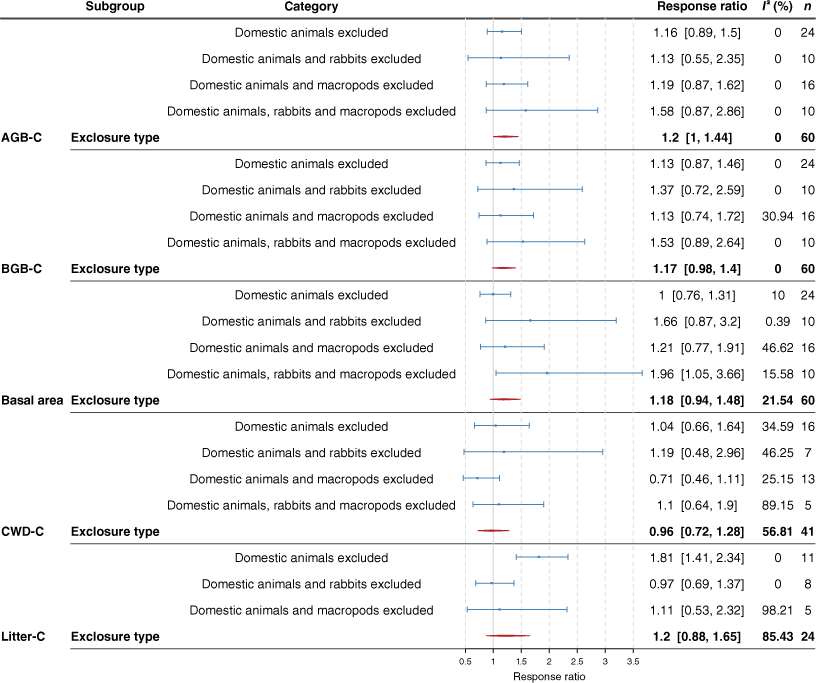
Discussion
Influence of vegetation and climate on C stocks
Eucalyptus-dominated vegetation had the highest live AGB-C stocks (mean of 24, and maximum of 115 Mg C ha−1), and these sites also had higher annual precipitation and lower temperatures than did the remaining vegetation types (Figs S1, S2). AGB-C stocks from other studies in Eucalyptus-dominated rangeland vegetation were 6–80 Mg C ha−1 (Burrows et al. 2002; Cook et al. 2005; Harms et al. 2005). The Acacia-dominated vegetation type averaged the lowest live AGB-C stocks (mean 1.8, and maximum of 8.6 Mg C ha−1) and these were generally on the drier sites.
The ‘other’ and A. aneura vegetation types averaged intermediate live aboveground C stocks with means of 2.5–6.4 Mg C ha−1 and maximum of 8.5–30.6 Mg C ha−1 respectively. Previous studies have reported up to 49 Mg C ha−1 for A. aneura (Witt et al. 2009b; Witt et al. 2011; Daryanto et al. 2013; Williams et al. 2024). The correlations between live C stocks and annual precipitation and evaporation observed here (Figs S1, S3) are consistent with the strong influence of precipitation on biomass and soil C stocks in Australian rangelands (Fensham et al. 2012; Allen et al. 2013; Bray et al. 2016; Henry 2023).
Mean CWD-C was 1.4 Mg C ha−1, with a maximum of 9.4 Mg C ha−1 in A. aneura vegetation. This may reflect the practice of felling or pruning A. aneura to feed livestock during dry periods or tree clearing or thinning to enhance forage growth, which can increase CWD (Pringle et al. 2021). Previous studies in rangeland vegetation dominated by Eucalyptus, Acacia, A. aneura, Callitris or shrubs have recorded a mean CWD of about 3.4 C Mg ha−1, and a maximum of 36 Mg C ha−1 (Burrows 1976; Woldendorp et al. 2002; Murphy et al. 2003; Harms et al. 2005; Daryanto et al. 2013; Prior et al. 2017).
The litter C pool was generally the smallest of the C pools examined, ranging from 0.005 to 2.31, with a mean of 0.41 Mg C ha−1, and did not vary among vegetation types. These values are comparable to those in other Australian rangeland studies, with litter C ranging from close to zero up to 8.7 Mg C ha−1 (Burrows 1976; Roxburgh et al. 2006a; Fontaine et al. 2012; Bray et al. 2014; Hunt 2014; Dalgleish et al. 2015; Williams et al. 2024).
Influence of grazing on C stocks, and interactions with climate
A key finding was that relative differences between grazing treatments were highly variable, and as a result of that variability, were generally not significant. The variability in responses to grazing found here is consistent with that in previous studies. For example, a higher AGB-C was reported for a cohort of trees with a diameter of <15 cm in Queensland A. aneura experiments, whereas larger cohorts (>15 cm) contained more biomass in the grazed treatments, leading to similar total AGB-C (Witt et al. 2009b; Witt et al. 2011). Tree densities were higher within exclosures at the Kidman Springs experiment (Bastin et al. 2003). However, no grazing effects on AGB-C were found in the central Australian Acacia experiment at Central Mount Wedge (Foran et al. 1982) or the Wapweelah A. aneura experiment in western New South Wales (Daryanto et al. 2013). Similarly, there was little difference in woody biomass between moderate and heavily grazed plots in the Wambiana experiment in Queensland (Eucalyptus vegetation) (Bray et al. 2014).
As the basal area of the grazed control plots increased, the increase in live C stocks of exclosures relative to grazed controls decreased (Fig. S4). This might be expected because when live C stocks are already high, the C stocks may be limited more by soil and climatic conditions than grazing, and therefore, removing grazing animals may have a lesser influence.
Meta-regression analysis suggested that response ratios for AGB-C and BGB-C consistently increased linearly with time since the exclosures were established (Fig. S10), suggesting that significant effects of grazing exclusion are more likely after long durations of grazing exclosure. However, the linear mixed model analysis did not find this correlation. The incongruence suggests uncertainties driven by the lack of replication in 27 of 46 grazing experiments.
The influence of grazing on woody vegetation may be masked by the effects of other factors that have greater influences on woody vegetation in rangelands, such as precipitation, temperature or pre-existing biomass stocks (Fensham et al. 2005; Fensham et al. 2012). Temporal changes in precipitation can have greater effects than grazing, at least on non-woody vegetation (Foran et al. 1982) or on woody vegetation cover (Fensham et al. 2005). Here, we found no significant linear relationships between site-level climate averages and biomass response to grazing exclusion, but AGB-C, BGB-C, basal area and litter C were consistently, albeit variably, higher with grazing exclusion in non-arid regions with MAP of >300 mm. Similarly, the mean response ratio for the Eucalyptus-dominated vegetation type, which experienced the highest MAP, was more positive than that in other vegetation types for AGB-C and, BGB-C with grazing exclusion. Given that regeneration is facilitated by precipitation (e.g. Hodgkinson 1991), it is likely that there are more opportunities for woody vegetation to regenerate and survive in regions with MAP of >300 mm.
Exclusion of domestic grazers can increase soil water infiltration and, subsequently, soil water-holding capacity (Witt et al. 2011), which could potentially enhance woody vegetation growth and recruitment while reducing mortality rates. The presence of grasses has been associated with lower water stress and higher recruitment of A. aneura than in locations where grasses were heavily grazed (Anderson and Hodgkinson 1997). Although this example may not hold for all vegetation types and locations, given that precipitation and soil water availability can have a strong temporal and spatial influence on rangeland vegetation (Foran et al. 1982; Fensham et al. 2005; Fensham et al. 2012), combining C stock measurements with additional data on soil moisture availability may help explain some of the observed variability in grazing effects. Furthermore, grazing intensity may modify the effect of a broad ‘grazing’ category on woody plants in arid and semi-arid rangelands. Without more detailed data, distance to water point could be used as a proxy for grazing intensity in future studies, although changes in water infrastructure during the experimental period would need to be considered. As an example, sheep grazing in a chenopod-dominated rangeland increased adult shrub mortality close to water and reduced recruitment, resulting in a decline in the shrub population, with the largest decline closer to water (Hunt 2010).
We were unable to obtain data on the stocking density of grazing animals during the experimental period, either domestic livestock or other grazers, including macropods and feral animals. However, six studies had rotational grazing treatments in addition to total exclusion plots. These studies showed more negative or less positive trends in biomass C in rotational grazing treatments than in grazing exclusion (Fig. S6, Table S3). The stocking density of grazing animals can be difficult to obtain (Allen et al. 2013), but would allow for more accurate measures of grazing intensity than the discrete treatments used here. This should include the stocking density of non-domestic animals, such as rabbits, feral goats and macropods, which may have a strong confounding influence or even a major influence on rangeland biodiversity in some regions (Mutze 2016).
Other factors that could be considered include fire and the palatability and accessibility of tree and shrub species for grazing at various stages of their lifecycle. It is worth noting that grazing can lead to stunted growth forms of some species, such as A. aneura (e.g. ‘cattle mulga’ Everist 1949), which could also potentially influence biomass C stocks. However, no such effects on A. aneura were observed in the 46 grazed A. aneura plots at 23 sites within the paired-plot network.
Implications
This study shows that responses to grazing exclusion are variable and difficult to predict, not that there are no grazing effects on C stocks. The effects of grazing exclusion on most C stocks ranged from moderate reductions to large increases in C stocks or basal area following grazing exclusion (Figs 4, 6). AGB-C, BGB-C, basal area and litter C were significantly, albeit variably, higher with grazing exclusion in regions with annual precipitation of >300 mm. The large variability within and across sites, as well as the small sample sizes, and the fact that woody vegetation C stock sizes are small in rangelands, all contributed to the difficulty in quantifying grazing effects in rangelands. It is important to note that although a sample size of 46 experiments includes all existing long-term exclosure experiments that were still intact and could be located and measured, it is a small sample relative to the large area of Australian rangelands.
Furthermore, the underlying drivers of the variability in C stocks could not be identified. As discussed above, future studies may benefit from combining C stock data with information on soil moisture availability, total grazing pressure, including grazing intensities of major native and feral animals such as macropods and rabbits, and repeated measurements. This information, although difficult to source broadly across the rangelands, could provide the foundations for a model capable of predicting where and when grazing effects might be significant and their impact on regional C stocks. The impact of fire, if grazing is removed from large areas, should also be considered. Given that rangelands cover 80% of the Australian continent, even if grazing has a small influence per area, or has an influence only in specific locations, altering grazing could have large implications for regional and national C accounting, warranting further investigation.
It is also important to note that maximising C stocks may not necessarily be the most ecologically sustainable objective (Witt et al. 2009b; Witt et al. 2011). For example, higher stand densities in terms of leaf area can increase water use (Chen et al. 2014) and thereby reduce soil water availability, potentially exacerbating the effects of low precipitation, and create ‘boom–bust’ cycles in response to climate variability. Increases in woody C stocks nearly always lead to reductions in pasture biomass, thereby negatively influencing livestock production (McIvor and Gardener 1995; Jackson and Ash 1998; Scanlan 2002; Dyer and Stafford Smith 2003; Witt 2013; Cowley et al. 2014).
Conclusions
Overall, the results illustrated the difficulty in deriving general rules or conditions under which exclusion of grazing can consistently generate positive biomass carbon accumulation in Australia’s rangelands. Across all experiments, there were few significant woody biomass C and CWD-C effects from grazing exclusion, even when considering factors such as vegetation type or grazer category. On the basis of the meta-analysis, exclusion was consistently, albeit variably, beneficial for (i) total litter-C, particularly under A. aneura-dominated vegetation, (ii) AGB-C, BGB-C and litter C in regions with precipitation of >300 mm, and (iii) for increasing AGB-C and BGB-C with time since exclosure. However, the magnitude of increase was highly variable, with the 95% CI upper limit about twice the value of the lower limit. The relative effects of grazing on live biomass or basal area declined, as the basal area of grazed control plots increased. We recommend that to enable greater clarity, future studies may need to collect additional information, including data on soil water availability and current and historical grazing intensity. Although grazing exclusion sometimes facilitated vegetation C stock accumulation, the effects were very variable, and based on these data, it is difficult to predict when and where grazing exclusion would consistently lead to higher C stock in woody vegetation.
Conflicts of interest
Steven Bray is the Associate Editor of The Rangeland Journal but was not involved in the peer review or any decision-making process for this paper. Authors have no other conflicts of interest to declare.
Declaration of funding
This work was funded by CSIRO and the Australian Government Department of Climate Change, Energy, the Environment and Water.
Acknowledgements
We thank the land holders and managers who provided access to their properties and maintained the experiments (Caris Vuckovic, Keith Leggett, James Christian, Andrew McCartney, John and Rana Manns, Steven Cadzow, John and Colin Dunne, and those preferring to remain anonymous). We are also grateful to the organisations that contributed to establishing and maintaining the experiments including universities (University of Queensland (Jenny Silcock, Bradd Witt, Rod Fensham, Manda Page), Southern Cross University (Greg Carroll), University of NSW (David Eldrige, Keith Leggett, Adrian Fisher), Adelaide University (Jose Facelli), Curtin university (Amu Elkington, CSIRO (Anna Richards, Brett Abbott, Bruce Murray, Ian Watson), Murdoch University (Richard Harper), State Governments (Qld DPI (Steven Bray, Peter O’Reagain, Ric Silcock, Tony Pressland), Qld DES (Shellie Cash), NSW DPI (Susan Orgill), NT DPI (Gary Bastin, Chris Materne, Robyn Cowley), WA DBCA (John Bruce), WA DPIRD (Rob Sudmeyer, Wayne Fletcher, Philip Thomas), South Queensland Landscapes (Andrew McCartney) and Bush Heritage Australia (Angela Hawdon, Tim Zwiersen, Graeme Finlayson). We also thank the staff who assisted with field and laboratory work, including Joel Armstrong, Micah Davies, Jodie Hayward, Stephanie Johnson, John Larmour, Jess Langridge, Kiara L’herpiniere, Tenneal Maskell, Aaron Midson, Doug Mohr, Eva Moore, Bruce Murray, Louis O’Neill, Magen Pettit, Josh Piper, Jaxon Reid, Ross Shadbolt, and Jon Schatz. We are also thankful to Dan Rosauer and Gerald Page who provided comments that helped improve the paper.
References
Allen DE, Pringle MJ, Bray S, Hall TJ, O’Reagain PO, Phelps D, Cobon DH, Bloesch PM, Dalal RC (2013) What determines soil organic carbon stocks in the grazing lands of north-eastern Australia? Soil Research 51, 695-706.
| Crossref | Google Scholar |
Anderson VJ, Hodgkinson KC (1997) Grass-mediated capture of resource flows and the maintenance of banded Mulga in a semi-arid woodland. Australian Journal of Botany 45, 331-342.
| Crossref | Google Scholar |
Aponte C, Tolhurst KG, Bennett LT (2014) Repeated prescribed fires decrease stocks and change attributes of coarse woody debris in a temperate eucalypt forest. Ecological Applications 24, 976-989.
| Crossref | Google Scholar | PubMed |
Attiwill PM (1979) Nutrient cycling in a Eucalyptus obliqua (L’Hérit.) forest. III Growth, biomass, and net primary production. Australian Journal of Botany 27, 439-458.
| Crossref | Google Scholar |
Back PV, Anderson ER, Burrows WH, Playford C (2009) Woody plant responses to various clearing strategies imposed on a poplar box (Eucalyptus populnea) community at Dingo in central Queensland. Tropical Grasslands 43, 37-52.
| Google Scholar |
Bastin GN, Ludwig JA, Eager RW, Liedloff AC, Andison RT, Cobiac MD (2003) Vegetation changes in a semiarid tropical savanna, northern Australia: 1973-2002. Rangeland Journal 25, 3-19.
| Crossref | Google Scholar |
Bastin G, Cowley R, Friedel M, Materne C (2024) Applying two remotely-sensed methods for monitoring grazing impacts in the Australian arid zone. The Rangeland Journal 45, 141-159.
| Crossref | Google Scholar |
Beale IF (1973) Tree density effects on yields of herbage and tree components in south west Queensland mulga (Acacia aneura F. Muell.) scrub. Tropical Grasslands 7, 135-142.
| Google Scholar |
Beringer J, Hutley LB, Tapper NJ, Cernusak LA (2007) Savanna fires and their impact on net ecosystem productivity in North Australia. Global Change Biology 13, 990-1004.
| Crossref | Google Scholar |
Beutel TS, Shepherd R, Karfs RA, Abbott BN, Eyre T, Hall TJ, Barbi E (2021) Is ground cover a useful indicator of grazing land condition? The Rangeland Journal 43, 55-64.
| Crossref | Google Scholar |
Bray S, Doran-Browne N, O’Reagain P (2014) Northern Australian pasture and beef systems. 1. Net carbon position. Animal Production Science 54, 1988-1994.
| Crossref | Google Scholar |
Bray SG, Allen DE, Harms BP, Reid DJ, Fraser GW, Dalal RC, Walsh D, Phelps DG, Gunther R (2016) Is land condition a useful indicator of soil organic carbon stock in Australia’s northern grazing land? The Rangeland Journal 38, 229-243.
| Crossref | Google Scholar |
Burrows WH, Henry BK, Back PV, Hoffmann MB, Tait LJ, Anderson ER, Menke N, Danaher T, Carter JO, McKeon GM (2002) Growth and carbon stock change in eucalypt woodlands in northeast Australia: ecological and greenhouse sink implications. Global Change Biology 8, 769-784.
| Crossref | Google Scholar |
Callister AN, England NF (2010) How dense is my blue gum? prediction of whole-tree basic density of Eucalyptus globulus. New Forests 40, 153-164.
| Crossref | Google Scholar |
Chen C, Eamus D, Cleverly J, Boulain N, Cook P, Zhang L, Cheng L, Yu Q (2014) Modelling vegetation water-use and groundwater recharge as affected by climate variability in an arid-zone Acacia savanna woodland. Journal of Hydrology 519, 1084-1096.
| Crossref | Google Scholar |
Cook GD, Liedloff AC, Eager RW, Chen X, Williams RJ, O’Grady AP, LBH D (2005) The estimation of carbon budgets of frequently burnt tree stands in savannas of northern Australia, using allometric analysis and isotopic discrimination. Australian Journal of Botany 53, 621-630.
| Crossref | Google Scholar |
Cowley RA, Hearnden MH, Joyce KE, Tovar-Valencia M, Cowley TM, Pettit CL, Dyer RM (2014) How Hot? How Often? Getting the fire frequency and timing right for optimal management of woody cover and pasture composition in northern Australian grazed tropical savannas. Kidman Springs Fire Experiment 1993–2013. The Rangeland Journal 36, 323-345.
| Crossref | Google Scholar |
Dalgleish SA, van Etten EJB, Stock WD, Knuckey C (2015) Fuel dynamics and vegetation recovery after fire in a semiarid Australian shrubland. International Journal of Wildland Fire 24, 613-623.
| Crossref | Google Scholar |
Daly RL, Hodgkinson KC (1996) Relationships between grass, shrub and tree cover on four landforms of semi-arid eastern Australia, and prospects for change by burning. The Rangeland Journal 18, 104-117.
| Crossref | Google Scholar |
Daryanto S, Eldridge DJ, Throop HL (2013) Managing semi-arid woodlands for carbon storage: grazing and shrub effects on above- and belowground carbon. Agriculture, Ecosystems and Environment 169, 1-11.
| Crossref | Google Scholar |
DCCEEW (2023) Australian Rangeland Boundaries. Available at https://fed.dcceew.gov.au/datasets/australian-rangeland-boundaries/about
DCCEEW (2024) Outback Australia – the rangelands. Available at https://www.dcceew.gov.au/environment/land/rangelands#:~:text=The%20rangelands%20of%20arid%20and%20semi%2Darid%20Australia&text=The%20arid%20zone%20is%20defined,average%20rainfall%20between%20250%2D350mm.
Dyer R, Stafford Smith M (2003) Ecological and economic assessment of prescribed burning impacts in semi-arid pastoral lands of northern Australia. International Journal of Wildland Fire 12, 403-413.
| Crossref | Google Scholar |
Eamus D, O’Grady AP, Hutley L (2000) Dry season conditions determine wet season water use in the wet-dry tropical savannas of northern Australia. Tree Physiology 20(18), 1219-1226.
| Crossref | Google Scholar | PubMed |
Eldridge DJ, Delgado-Baquerizo M, Travers SK, Val J, Oliver I, Dorrough JW, Soliveres S (2018) Livestock activity increases exotic plant richness, but wildlife increases native richness, with stronger effects under low productivity. Journal of Applied Ecology 55, 766-776.
| Crossref | Google Scholar |
Everist SL (1949) Mulga (Acacia aneura F.Muell.) in Queensland. Queensland Journal of Agricultural Science 6, 87-139.
| Google Scholar |
Falster D, Gallagher R, Wenk EH, et al. (2021) AusTraits, a curated plant trait database for the Australian flora. Scientific Data 8, 254.
| Crossref | Google Scholar | PubMed |
Fensham RJ, Fairfax RJ, Archer SR (2005) Rainfall, land use and woody vegetation cover change in semi-arid Australian savanna. Journal of Ecology 93, 596-606.
| Crossref | Google Scholar |
Fensham RJ, Silcock JL, Dwyer JM (2011) Plant species richness responses to grazing protection and degradation history in a low productivity landscape. Journal of Vegetation Science 22, 997-1008.
| Crossref | Google Scholar |
Fensham RJ, Fairfax RJ, Dwyer JM (2012) Potential aboveground biomass in drought-prone forest used for rangeland pastoralism. Ecological Applications 22, 894-908.
| Crossref | Google Scholar | PubMed |
Fontaine JA, Westcott VC, Enright NJ, Lade JC, Miller BP (2012) Fire behaviour in south-western Australian shrublands: evaluating the influence of fuel age and fire weather. International Journal of Wildland Fire 21, 385-395.
| Crossref | Google Scholar |
Foran BD, Bastin G, Remenga E, Hyde KW (1982) The response to season, exclosure, and distance from water of three central Australian pasture types grazed by cattle. Australian Rangeland Journal 4, 5-15.
| Crossref | Google Scholar |
Friedel MH (1997) Discontinuous change in arid woodland and grassland vegetation along gradients of cattle grazing in central Australia. Journal of Arid Environments 37, 145-164.
| Crossref | Google Scholar |
Harms BP, Dalal RC, Cramp AP (2005) Changes in soil carbon and soil nitrogen after tree clearing in the semi-arid rangelands of Queensland. Australian Journal of Botany 53, 639-650.
| Crossref | Google Scholar |
Henry B (2023) ‘Potential for soil carbon sequestration in Northern Australian grazing lands: a review of the evidence.’ (Department of Agriculture and Fisheries: Qld). Available at www.futurebeef.com.au
Hodgkinson KC (1991) Shrub recruitment response to intensity and season of fire in a semi-arid woodland. Journal of Applied Ecology 28, 60-70.
| Crossref | Google Scholar |
Hunt LP (2010) Spatial variation in the demography and population dynamics of a perennial shrub (Atriplex vesicaria) under sheep grazing in semi-arid Australian rangelands. Austral Ecology 35, 794-805.
| Crossref | Google Scholar |
Hunt LP (2014) Aboveground and belowground carbon dynamics in response to fire regimes in the grazed rangelands of northern Australia: initial results from field studies and modelling. The Rangeland Journal 36, 347-358.
| Crossref | Google Scholar |
Hutson BR (1985) Rates of litterfall and organic matter turnover at three South Australian indigenous forest sites. Australian Journal of Ecology 10, 351-359.
| Crossref | Google Scholar |
Jackson J, Ash AJ (1998) Tree-grass relationships in open eucalypt woodlands of northeastern Australia: Influence of trees on pasture productivity, forage quality and species distribution. Agroforestry Systems 40, 159-176.
| Crossref | Google Scholar |
Mathers NJ, Xu Z, Blumfield TJ, Berners-Price SJ, Saffigna PG (2003) Composition and quality of harvest residues and soil organic matter under windrow residue management in young hoop pine plantations as revealed by solid-state 13C NMR spectroscopy. Forest Ecology and Management 175, 467-488.
| Crossref | Google Scholar |
McIvor JG, Gardener CJ (1995) Pasture management in semi-arid tropical woodlands: effects on herbage yields and botanical composition. Australian Journal of Experimental Agriculture 35, 705-715.
| Crossref | Google Scholar |
Miranda I, Almeida MH, Pereira H (2001) Provenance and site variation of wood density in Eucalyptus globulus Labill. at harvest age and its relation to a non-destructive early assessment. Forest Ecology and Management 149, 235-240.
| Crossref | Google Scholar |
MLA (2021a) Cattle numbers, Meat and livestock Australia, Market Information. Available at https://www.mla.com.au/prices-markets/Trends-analysis/livestock-distribution-maps/ [Verified 4 February 2024]
MLA (2021b) ‘Sheep numbers, Meat and livestock Australia, Market Information. Available at https://www.mla.com.au/prices-markets/Trends-analysis/livestock-distribution-maps/ [Verified 4 February 2024]
Mutze G (2016) Barking up the wrong tree? Are livestock or rabbits the greater threat to rangeland biodiversity in southern Australia? The Rangeland Journal 38, 523-531.
| Crossref | Google Scholar |
Neumann M, Eastaugh C, Adams M (2020) Managing mixed Callitris–Eucalyptus forests for carbon and energy in central-eastern Australia. Biomass and Bioenergy 140, 105656.
| Crossref | Google Scholar |
NRMMC (2010) Principles for sustainable resource management in the rangelands. (CoA). Available at https://www.dcceew.gov.au/sites/default/files/env/pages/c61919d9-599e-451d-960e-364e03170e8d/files/rangelands-principles.pdf
Pahl L (2019) Macropods, feral goats, sheep and cattle. 2. Equivalency in what and where they eat. The Rangeland Journal 41, 519-533.
| Crossref | Google Scholar |
Paul KI, Roxburgh SH (2024) A national accounting framework for fire and carbon dynamics in Australian savannas. International Journal of Wildland Fire 33, WF23104.
| Crossref | Google Scholar |
Paul KI, Roxburgh SH, Chave J, England JR, Zerihun A, Specht A, Lewis T, Bennett LT, Baker TG, Adams MA, Huxtable D, Montagu KD, Falster DS, Feller M, Sochacki S, Ritson P, Bastin G, Bartle J, Wildy D, Hobbs T, Larmour J, Waterworth R, Stewart HTL, Jonson J, Forrester DI, Applegate G, Mendham D, Bradford M, O’Grady A, Green D, Sudmeyer R, Rance SJ, Turner J, Barton C, Wenk EH, Grove T, Attiwill PM, Pinkard E, Butler D, Brooksbank K, Spencer B, Snowdon P, O’Brien N, Battaglia M, Cameron DM, Hamilton S, McAuthur G, Sinclair J (2016) Testing the generality of above-ground biomass allometry across plant functional types at the continent scale. Global Change Biology 22, 2106-2124.
| Crossref | Google Scholar | PubMed |
Paul KI, Larmour J, Specht A, Zerihun A, Ritson P, Roxburgh SH, Sochacki S, Lewisg T, Barton CVM, England JR, Battaglia M, O’Grady A, Pinkard E, Applegate G, Jonson J, Brooksbank K, Sudmeyer R, Wildy D, Montagu KD, Bradford M, Butler D, Hobbs T (2019) Testing the generality of below-ground biomass allometry across plant functional types. Forest Ecology and Management 432, 102-114.
| Crossref | Google Scholar |
Pinheiro J, Bates D, DebRoy S, Sarkar D R Core Team (2018) nlme: linear and nonlinear mixed effects models. R package version 3. 1–137. Available at http://CRAN.R-project.org/package=nlme
Pople AR, Grigg GC, Cairns SC, Beard LA, Alexander P (2000) Trends in the numbers of red kangaroos and emus on either side of the South Australian dingo fence: evidence for predator regulation? Wildlife Research 27, 269-276.
| Crossref | Google Scholar |
Possell M, Jenkins M, Bell TL, Adams MA (2015) Emissions from prescribed fires in temperate forest in south-east Australia: implications for carbon accounting. Biogeosciences 12, 257-268.
| Crossref | Google Scholar |
Pringle MJ, Allen DE, Phelps DG, Bray SG, Orton TG, Dalal RC (2014) The effect of pasture utilization rate on stocks of soil organic carbon and total nitrogen in a semi‐arid tropical grassland. Agriculture, Ecosystems & Environment 195, 83-90.
| Google Scholar |
Pringle MJ, Bray SG, Carter JO (2021) Modelling the disappearance of coarse woody debris, following a land clearing event. Carbon Balance and Management 16, 36.
| Crossref | Google Scholar | PubMed |
Prior LD, Murphy BP, Williamson GJ, Cochrane MA, Jolly WM, Bowman DMJS (2017) Does inherent flammability of grass and litter fuels contribute to continental patterns of landscape fire activity? Journal of Biogeography 44, 1225-1238.
| Crossref | Google Scholar |
Raymond CA, Muneri A (2000) Effect of fertilizer on wood properties of Eucalyptus globulus. Canadian Journal of Forest Research 30, 136-144.
| Crossref | Google Scholar |
Raymond CA, Muneri A (2001) Non-destructive sample of Eucalyptus globulus and E. nitens for wood properties. I. Basic density. Wood Science Technology 35, 27-39.
| Crossref | Google Scholar |
R Core Team (2023) R: a language and environment for statistical computing. (R Foundation for Statistical Computing: Vienna, Austria). Available at http://www.R-project.org/
Retallack A, Rifai S, Finlayson G, Ostendorf B, Lewis M (2024) Remote sensing for rangeland conservation monitoring: impacts of livestock removal after 15 years. Journal of Applied Ecology 61, 2111-2122.
| Crossref | Google Scholar |
Roxburgh SH, Mackey BG, Dean C, Randall L, Lee A, Austin J (2006a) Organic carbon partitioning in soil and litter in subtropical woodlands and open forests: a case study from the Brigalow Belt, Queensland. The Rangeland Journal 28, 115-125.
| Crossref | Google Scholar |
Roxburgh SH, Wood SW, Mackey BG, Woldendorp G, Gibbons P (2006b) Assessing the carbon sequestration potential of managed forests: a case study from temperate Australia. Journal of Applied Ecology 43, 1149-1159.
| Crossref | Google Scholar |
Russell-Smith J, Murphy BP, Meyer CP, Cook GD, Maier S, Edwards AC, Schatz J, Brocklehurst P (2009) Improving estimates of savanna burning emissions for greenhouse accounting in northern Australia: limitations, challenges, applications. International Journal of Wildland Fire 18, 1-18.
| Crossref | Google Scholar |
Scanlan JC (2002) Some aspects of tree-grass dynamics in Queensland’s grazing lands. The Rangeland Journal 24, 56-82.
| Crossref | Google Scholar |
Searle SD, Owen JV (2005) Variation in basic wood density and percentage heartwood in temperate Australian Acacia species. Australian Forestry 68, 126-136.
| Crossref | Google Scholar |
Shaw KA, Rolfe JW, English BH, Kernot JC (2007) A contemporary assessment of land condition in the Northern Gulf region of Queensland. Tropical Grasslands 41, 245-252.
| Google Scholar |
Sillett SC, Van Pelt R, Koch GW, Ambrose AR, Carroll AL, Antoine ME, Mifsud BM (2010) Increasing wood production through old age in tall trees. Forest Ecology and Management 259, 976-994.
| Crossref | Google Scholar |
Slijepcevic A (2001) Loss of carbon during controlled regeneration burns in Eucalyptus obliqua forest. Tasforests 13, 281-290.
| Google Scholar |
Stewart GH, Burrows LE (1994) Coarse woody debris in old-growth temperate beech (Nothofagus) forests of New Zealand. Canadian Journal of Forest Research 24, 1989-1996.
| Crossref | Google Scholar |
Stewart HTL, Flinn DW, Aeberli BC (1979) Above-ground biomass of a mixed eucalypt forest in eastern Victoria. Australian Journal of Botany 27, 725-740.
| Crossref | Google Scholar |
van Groenigen KJ, Osenberg CW, Hungate BA (2011) Increased soil emissions of potent greenhouse gases under increased atmospheric CO2. Nature 475, 214-216.
| Crossref | Google Scholar | PubMed |
van Wagner CE (1968) The line intersect method in forest fuel sampling. Forest Science 14, 20-26.
| Google Scholar |
Viechtbauer W (2010) Conducting meta-analyses in R with the metafor package. Journal of Statistical Software 36, 1-48.
| Crossref | Google Scholar |
Volkova L, Weston C (2013) Redistribution and emission of forest carbon by planned burning in Eucalyptus obliqua (L. Hérit.) forest of south-eastern Australia. Forest Ecology and Management 304, 383-390.
| Crossref | Google Scholar |
Volkova L, Weston CJ (2015) Carbon loss from planned fires in southeastern Australian dry Eucalyptus forests. Forest Ecology and Management 336, 91-98.
| Crossref | Google Scholar |
Williams A, Russell P, Blood D (2024) Above-ground carbon in mulga-dominated rangelands, Murchison Bioregion, Western Australia. The Rangeland Journal 45, 211-223.
| Crossref | Google Scholar |
Wilson AD (1991) Forage utilization by sheep and kangaroos in a semi-arid woodland. The Rangeland Journal 13, 81-90.
| Crossref | Google Scholar |
Witt GB (2013) Vegetation changes through the eyes of the locals: the ‘artificial wilderness’ in the mulga country of south-west Queensland. The Rangeland Journal 35, 299-314.
| Crossref | Google Scholar |
Witt GB, Harrington RA, Page MJ (2009a) Is ‘vegetation thickening’ occurring in Queensland’s mulga lands – a 50-year aerial photographic analysis. Australian Journal of Botany 57, 572-582.
| Crossref | Google Scholar |
Witt GB, Noël MV, Bird MI, Beeton RJS (2009b) Investigating long-term grazing exclosures for the assessment of carbon sequestration and biodiversity restoration potential of the mulga lands. Report to the Department of the Environment, Water, Heritage and the Arts. (School of Integrative Systems, The University of Queensland: Australia)
Witt GB, Noël MV, Bird MI, Beeton RJS, Menzies NW (2011) Carbon sequestration and biodiversity restoration potential of semi-arid mulga lands of Australia interpreted from long-term grazing exclosures. Agriculture, Ecosystems and Environment 141, 108-118.
| Crossref | Google Scholar |
Ximenes FA, Gardner WD, Kathuria A (2008) Proportion of above-ground biomass in commercial logs and residues following the harvest of five commercial forest species in Australia. Forest Ecology and Management 256, 335-346.
| Crossref | Google Scholar |
Zhang D, Ng EL, Hu W, Wang H, Galaviz P, Yang H, Sun W, Li C, Ma X, Fu B, Zhao P, Zhang F, Jin S, Zhou M, Du L, Peng C, Zhang X, Xu Z, Xi B, Liu X, Sun S, Cheng Z, Jiang L, Wang Y, Gong L, Kou C, Li Y, Ma Y, Huang D, Zhu J, Yao J, Lin C, Qin S, Zhou L, He B, Chen D, Li H, Zhai L, Lei Q, Wu S, Zhang Y, Pan J, Gu B, Liu H (2020) Plastic pollution in croplands threatens long-term food security. Global Change Biology 26, 3356-3367.
| Crossref | Google Scholar | PubMed |