Ocean warming projected to increase ecotourism opportunities to encounter iconic marine megafauna (manta rays and zebra sharks) off south-eastern Australia
T. R. Davis
A
B
Abstract
Tourism is a major contributor to coastal economies, with climate change generating unquantified impacts on this sector. Specifically, marine ecotourism businesses often depend on encounters with iconic megafauna, but it is unknown how interactions with these species might change under ocean warming.
To assess how climate change may affect key dive-ecotourism species (manta rays and zebra sharks) in south-eastern Australia, where changes in temporal persistence are anticipated.
Habitat-suitability models were developed for manta rays and zebra sharks and applied to predict changes in suitable thermal habitat at popular scuba-diving locations in New South Wales (NSW).
Sea-surface temperature was the dominant factor associated with the presence of manta rays and zebra sharks. Modelling indicated that timings of seasonal migrations are likely to be altered by ocean warming, with temporal persistence in central NSW potentially increasing, from current low levels, by up to 4 months by 2060.
Prospects for divers to encounter manta rays and zebra sharks are likely to increase in central NSW because of climate-driven ocean warming.
New ecotourism opportunities will develop in NSW, through increased potential to encounter iconic marine megafauna, providing novel openings for coastal ecotourism industries.
Keywords: climate change, migration, Mobula alfredi, range shift, sea-surface temperature, species distribution, Stegostoma tigrinum, temporal persistence.
Introduction
Tourism is a major driver of coastal economies globally, with the intergovernmental Organisation for Economic Co-operation and Development (2016) estimating the direct value of maritime and coastal tourism at US$390 billion. Within this sector, marine ecotourism often depends on observing iconic wildlife, providing both a boost to local economies and opportunities for education and environmental stewardship (Topelko and Dearden 2005; Cisneros-Montemayor et al. 2013). However, what is largely unknown is how climate change might affect opportunities to encounter iconic wildlife, as species continue to respond to climate change through shifts in their geographic distributions (Pecl et al. 2017).
Future shifts in species distribution patterns will have flow-on effects for the people and tourism industries that rely on encounters with mobile species for their livelihoods. For example, encounters with migrating marine megafauna, particularly cetaceans and fishes, are an important tourism drawcard in many areas (Cisneros-Montemayor et al. 2013; O’Malley et al. 2013; Meynecke et al. 2017), contributing significantly to coastal economies. In particular, worldwide, many snorkel and scuba-diving tourist businesses (dive businesses) rely heavily on encounters with iconic fish megafauna, such as manta rays (Anderson et al. 2011; O’Malley et al. 2013) and sharks (Topelko and Dearden 2005; Cisneros-Montemayor et al. 2013). The global economic value of manta ray dive-tourism is estimated to exceed US$140 million per year (O’Malley et al. 2013; Murray et al. 2020), whereas shark tourism (sharks, rays and chimaeras) is worth more than US$310 million per year (Cisneros-Montemayor et al. 2013). Consequently, changes in migration patterns for shark and ray species, owing to the world’s changing climate, will affect these businesses, with further information on expected shifts in migration patterns needed to inform the future plans for these operations.
In Australia, encounters with both manta rays (Venables et al. 2016) and sharks (Huveneers et al. 2017) are important contributors to the success of dive businesses. In eastern Australia, manta rays (Mobula alfredi (Krefft, 1868)) and zebra sharks (Stegostoma tigrinum (Forster, 1781)) are highly sought-after by recreational divers (Couturier et al. 2011; Dudgeon et al. 2013). Both species are known to move seasonally along the eastern Australian coast, with water temperature strongly influencing these seasonal movements (Couturier et al. 2018). Typically, both species move southward during the warm austral summer–autumn period, potentially utilising the southward-flowing East Australian Current (EAC) to facilitate migrations (Couturier et al. 2011; Dudgeon et al. 2013). They then migrate northward during winter, as water temperatures fall at southern sites, to regions where water temperatures are more suitable (i.e. warmer) (Couturier et al. 2018). These movements are also influenced, and are therefore made more complex, by the search for food and more productive habitats (Couturier et al. 2018).
This movement of manta rays and zebra sharks, along the eastern Australian coastline, has been monitored using a range of techniques, including satellite-tagging (Jaine et al. 2014), acoustic tagging (Barnett et al. 2024) and citizen-science programs (Armstrong et al. 2019). In eastern Australia, the reported southern limit for the movement of manta rays is currently at South West Rocks (30.9°S) (Armstrong et al. 2020), whereas zebra sharks have been observed as far south as Jervis Bay (35.0°S) (Atlas of Living Australia, see bie.ala.org.au). These species are infrequent visitors at these southern latitudes, and it is unlikely that sightings south of these regions represent their current normal distributions (Armstrong et al. 2020). However, this is likely to change, with future shifts in the distributions and migration patterns of manta rays and zebra sharks expected, owing to ocean warming (Armstrong et al. 2020; AquaMaps, ver. 8, see https://www.aquamaps.org/).
Future climate-mediated shifts in migration patterns are likely to affect when these iconic species are present at key diving locations, with the potential to substantially affect dive-tourism businesses in south-eastern Australia. However, there are currently limited data available about the timing of current migrations at key diving locations in south-eastern Australia and limited understanding of how these migration patterns may shift under future climate change. Quantitative research is required to inform stakeholders about change in migrations patterns to enable adaptation and maintain economic viability of businesses. Analyses assessing changes to the amount of time that mobile species spend at their historical-range edges are particularly relevant for investigating changes in opportunities to encounter species (Champion et al. 2018). Changes in annual residency times have important implications for understanding opportunities for ecotourism encounters with range-shifting species (Rogers et al. 2019; Champion et al. 2022).
Here, we develop habitat-suitability models for iconic marine megafauna in New South Wales (NSW; manta rays and zebra sharks) by using tagged-animal detections and human observations, combined with environmental variables characterising ocean conditions at known locations of species occurrence. These models were then applied, in conjunction with projections of future environmental conditions, to predict changes in the temporal persistence of suitable thermal habitat for both species at popular scuba-diving locations in NSW on Australia’s eastern coast. These results provide the NSW diving industry and other stakeholders with an understanding of likely future changes to opportunities to encounter these iconic megafaunas under climate change and enable adaptation to ensure economic viability and capitalisation on new tourism opportunities.
Materials and methods
Study area
South-eastern Australia contains the poleward-range edge for the distributions of manta rays and zebra sharks in eastern Australia (Couturier et al. 2011; Dudgeon et al. 2013) and, therefore, is the region where changes in annual temporal persistence of these species are most likely to occur. Consequently, habitat-suitability models were built using occurrence data for manta rays (Fig. 1a) and zebra sharks (Fig. 1b) from the Arafura, Coral and Tasman Seas located in the southern hemisphere (0–40°S) on the eastern side of Australia (130–180°E).
Species and environmental data
Occurrence data were extracted from the Global Biodiversity Information Facility (see https://www.gbif.org, accessed 22 August 2023), the Reef Life Survey species occurrence database (see https://portal.aodn.org.au/search, accessed 22 August 2023), Atlas of Living Australia data (see bie.ala.org.au, accessed 28 August 2023), and dive-shop records (Supplementary Table S1). Data from all sources provided the location and date where a target-species presence was recorded. Data were screened to include only data from 2000 onward, with this period selected to match the period for the environmental data used in modelling and given that only very limited species data were available prior to this date.
Data were screened to exclude records for preserved specimens, records for zebra shark egg cases and records with no date or location-coordinate information. All citizen-science records were verified by examination of photos, to confirm correct species identification. Additionally, multiple records from the same location (within ~5-km radius of each other) and from the same week (within a 7-day window of each other) were combined to form a single record, as recommended for filtering biological data (Johnston et al. 2021) and appropriate for handling spatial and temporal autocorrelation structures within species-occurrence datasets (Brodie et al. 2015; Champion et al. 2021). The resulting screened presence data consisted of a mixture of machine detections from tagged animals carrying satellite and acoustic transmitters (38.2% manta rays, 22.0% zebra sharks) citizen-science records (61.0% manta rays, 76.4% zebra sharks) and records from scientific field surveys (0.8% manta rays, 1.6% zebra sharks; Table 1). To ensure that habitat suitability models were fitted to the same amount of data, and thus their results were subsequently comparable, 700 occurrence records were randomly sampled for both species from the resulting dataset and retained for model selection and evaluation.
Data source | Manta rays (Mobula alfredi) | Zebra sharks (Stegostoma tigrinum) | |
---|---|---|---|
Citizen- science records | 532 | 558 | |
Scientific survey records | 7 | 12 | |
Tagged-animal detections | 333 | 161 | |
Total | 872 | 731 |
To create a binomial response variable for modelling the environmental habitat preferences of manta rays and zebra sharks, pseudo-absence data were spatially and temporally randomised in water shallower than 200 m (i.e. the depth of the continental shelf break) throughout the study extent and period to characterise unsuitable habitat for each species. A consistent dataset containing a total of 10,000 pseudo-absences was used for each species, on the basis of Barbet-Massin et al. (2012) who recommend a large number (i.e. 10,000 or more) of randomly selected pseudo-absences for statistical modelling of species habitat preferences.
Multiple, dynamic ocean variables that are known to influence the seasonal distribution patterns of mobile marine species off eastern Australia (Hobday and Hartog 2014; Champion et al. 2021) were downloaded from the Copernicus Marine Environment Monitoring Service (CMEMS, see https://data.marine.copernicus.eu/products) and matched to manta ray and zebra shark occurrence and pseudo-absence data. These variables were sea-surface temperature (SST; 0.05° spatial resolution; product #010_011), eddy kinetic energy (EKE; 0.25° spatial resolution; product #008_047), sea-level anomaly (SLA; 0.25° spatial resolution; product #008_047) and chlorophyll-a concentration (CHL; 0.04° spatial resolution; product #009_082).
Collinearity among ocean variables was assessed using Spearman rank correlation coefficients and variance inflation factors (VIFs). Spearman coefficients for pairwise comparisons among ocean variables did not show any collinearity (i.e. values for Spearman’s r were <0.5 or >−0.5). Furthermore, VIFs associated with all ocean variables were <1.5, indicating that collinearity among these variables was unlikely to affect model quality (Zuur et al. 2007).
Habitat-suitability modelling
Generalised additive mixed-effects models (GAMMs) were used to parameterise habitat suitability preferences for manta rays and zebra sharks. GAMMs used a logistic link function to relate the binomially distributed response variable (i.e. species occurrence or pseudo-absence) to multiple dynamic ocean-state covariates. Models also included ‘calendar year’ as a random intercept term to control for intra-class correlations among data collected during the same year, which may have been temporally biased towards certain years because of unmeasured annual variation in species observational effort over the study period. To accommodate non-linear responses, penalised regression spline smoothers were fitted using generalized cross-validation as recommended, to optimise smooth functions and avoid overfitting to the data (Zuur et al. 2009). Smoothers were removed from predictor variables in favour of linear model fits if their effective degrees of freedom were approximately equal to 1, which indicates linearity with log-of-odds transformed binomial response variables. These models estimate the probability of species occurrence in space and time. However, because absolute probabilities are contingent on the ratio of occurrence to absence data (Pearce and Boyce 2006), and given that pseudo-absences were randomly generated herein, probabilistic predicted values were rescaled to an index of environmental habitat suitability ranging between 0 (unsuitable) and 1 (optimal).
A backward model-selection procedure was initially employed to identify significant predictors of manta ray and zebra shark occurrence by using all ocean variables. Following this, models underwent further refinement via a stepwise selection process guided by covariate P-values. This yielded a set of explanatory models for each species, characterised by nested combinations of covariates of decreasing complexity. Among these models, the one displaying the lowest Akaike information criterion (AIC) value was identified as the most parsimonious (i.e. the best model). A key objective of this study was to develop a time-series of monthly habitat-suitability projections for both study species under future climate change. However, future data from the latest generation of global climate models (CMIP6) produced at monthly time-steps and downscaled to a spatial resolution relevant to dive location along Australia’s eastern coast were available only for the SST model covariate (see Future projections section). Therefore, we retained temperature-only models (i.e. thermal-habitat suitability models) from the nested set of habitat-suitability models for each study species for the purpose of creating future projections of thermal suitability for manta rays and zebra sharks. Assessments of model accuracy and predictive skill (described below) were compared between temperature-only and best habitat-suitability models for both species, with the best models being associated with only minor improvements in model quality over temperature-only models.
Given that the objective of this study was to project species habitat suitability for a forthcoming period, we utilised an out-of-sample evaluation method to gauge model accuracy and predictive capability (Champion et al. 2022). This involved training best and temperature-only habitat models for individual species by using subsets of occurrence and pseudo-absence data from the timeframe spanning 2000–15 (referred to as Period 1), followed by model testing utilising data from 2016 to 2023 (referred to as Period 2). These periods were selected as they each contained ~50% of the observational data for both study species. Therefore, model accuracy was tested between two periods that have experienced historical climate-driven environmental changes, which provides an appropriate assessment of model accuracy for future periods associated with continued climate change.
A six-fold cross-validation was undertaken by randomly extracting six subsets of species-occurrence and pseudo-absence data, each containing 50 occurrence records and 1000 pseudo-absences for every species. Of these, five subsets were drawn from Period 1 for model-training purposes, whereas the sixth subset was drawn from Period 2 for model testing. Subsequently, best and temperature-only habitat-suitability models for each species were fitted to each Period 1 subset and subsequently assessed against the Period 2 subset. The outcomes of these evaluations yielded a series of confusion matrices, which describe rates of true positive or negative outcomes as well as false positive or negative outcomes, which were then utilised to quantify two metrics of model accuracy. These metrics included the mean area under the receiver-operating characteristic curve (AUC; range between 0 and 1), where values exceeding 0.8 signify good to excellent model performance (Araújo et al. 2005), and the mean true skill statistic (TSS; range between −1 and 1), where positive values indicated models surpassing chance expectations (Allouche et al. 2006). Differences in AUC and TSS values between the best and temperature-only habitat-suitability models for each species were quantified to compare model performance.
Future projections
Monthly thermal-habitat projections for manta rays and zebra sharks over the period 2015–59 were created using an ensemble of sea-surface temperature (SST) data downscaled from seven global climate models (GCMs; CMIP6; Table 2). GCMs were selected using the IPCC Working Group 1 Interactive Atlas of Regional Information interface (see https://interactive-atlas.ipcc.ch/regional-information) to ensure that the GCMs used to develop the multi-model ensemble encompassed a range of ocean temperature futures projected for eastern Australia under climate change. CMIP6 SST projections under the SSP5-8.5 scenarios were extracted from the CMIP6 data portal for analysis (see https://esgf-node.llnl.gov/search/cmip6).
Model | Institution | Native resolution (°) | |
---|---|---|---|
ACCESS-CM2 | Commonwealth Scientific and Industrial Research Organisation (CSIRO) | 1.0 × 1.0 | |
ACCESS-ESM1-5 | Commonwealth Scientific and Industrial Research Organisation (CSIRO) | 1.0 × 1.0 | |
GFDL-CM4 | National Oceanic and Atmospheric Administration (NOAA) | 0.25 × 0.25 | |
GFDL-ESM4 | National Oceanic and Atmospheric Administration (NOAA) | 0.5 × 0.5 | |
MIROC6 | Japan Agency for Marine-Earth Science and Technology (MIROC) | 1.0 × 1.0 | |
NorESM2-LM | Norwegian Earth System Model Climate Modelling Consortium (NCC) | 1.0 × 1.0 | |
HadGEM3-GC31-LL | Met Office Hadley Centre (MOHC) | 1.0 × 1.0 |
Gridded monthly sea-surface temperature data produced by each GCM were downscaled under the SSP5-8.5 climate-change scenario.
The coarse spatial resolution of GCM data (0.25–1°) has limited utility for projecting changes in suitable thermal habitat for each study species at popular dive locations along the eastern Australian coastline. Therefore, we applied the delta ‘change-factor’ method to downscale future SST data throughout the study region to a 0.05° spatial resolution that is consistent with satellite SST observations. Delta downscaling was undertaken because it has proven utility for providing high-resolution mean climate conditions over decadal time periods for numerous climate-impact studies (e.g. Morley et al. 2018; Navarro-Racines et al. 2020; von Hammerstein et al. 2022).
This downscaling process involved the following: (1) re-mapping the curvilinear source GCM data to a global rectilinear grid by using the second-order conservative algorithm (remapcon2) in Climate Data Operators (ver. 1.9.6, see https://doi.org/10.5281/zenodo.2558193; Schulzweida 2019); (2) calculating the difference (i.e. delta value) between monthly aggregated data for successive 20-year periods centred on all years ranging between 2015 and 2059 and a modelled historical baseline period encompassing 1993–2012 for each GCM; (3) disaggregating delta value matrices from their native model resolution to the finer resolution of satellite-observed SST data (i.e. 0.05°) by using bilinear interpolation; and (4) adding delta value matrices centred on years ranging between 2015 and 2059 to an observed monthly SST climatology encompassing the period 1993–2012. Satellite SST data used consistently throughout this study are daily global SST from the CMEMS (product #010_011). A multi-model ensemble was then created using median SST values from downscaled projections across each of the seven GCMs. Median values were utilised to create the multi-model ensemble because this statistic is robust to outliers and does not assume that the data are normally distributed. This method produced a monthly time-series (2015–59) of future SST data downscaled to a 0.05° resolution required to facilitate projections of thermal-habitat suitability for manta rays and zebra sharks off eastern Australia.
To assess the accuracy of future habitat projections produced using the ensemble for CMIP6 SST data, monthly thermal-habitat suitability matrices nearshore of the continental shelf break were generated using satellite-derived and modelled SST for the 6-year period encompassing 2015–20. Collinearity among monthly spatial matrices of species thermal-habitat suitability created using observed and modelled data for the corresponding historical period (i.e. 2015–20) was assessed using Pearson’s correlation coefficient to infer the accuracy of future projections of thermal-habitat suitability (Spillman and Alves 2009; Eveson et al. 2015).
Spatial matrices of thermal-habitat suitability, for both study species, created nearshore of the continent shelf break at monthly timesteps between January 2015 and December 2020 by using observed and modelled SST data sources were positively correlated (Supplementary Fig. S1). Pearson correlation coefficients evaluating the collinearity of thermal-habitat suitability matrices produced using observed and modelled SST data ranged between 0.77 and 0.97 for manta rays and 0.47–0.95 for zebra sharks. This supports the use of thermal-habitat suitability projections created using downscaled SST data from an ensemble of CMIP6 GCMs for assessing future changes in manta ray and zebra shark temporal persistence off eastern Australia.
Temporal persistence at dive locations
Monthly spatial projections of manta ray and zebra shark thermal-habitat suitability, from January 2015 to December 2059, were used to assess changes in annual temporal persistence (i.e. number of months per year) at popular dive locations in south-eastern Australia. The dive locations assessed were Byron Bay (28.64°S), Coffs Harbour (30.30°S), South West Rocks (30.92°S), Forster (32.19°S), Port Stephens (32.69°S), Sydney (33.89°S) and Jervis Bay (35.19°S). These locations were selected because they are popular scuba-diving locations in NSW with currently operating recreational scuba-diving businesses (Harriott et al. 1997; Willis and Boshoff 2020). For each monthly projection, thermal-habitat suitability at each dive location had to exceed a species threshold that distinguishes suitable from unsuitable thermal habitat. Given that habitat models predicted thermal suitability on a scale ranging between 0 and 1, it was necessary to identify a threshold value for partitioning predictions into ‘suitable’ and ‘unsuitable’ habitat categories. A mid-point threshold of 0.5 is commonly used for this purpose in habitat-modelling studies (Bailey et al. 2002; Stockwell and Peterson 2002); however, arbitrarily adopting this value has been criticised for lacking ecological meaning (Liu et al. 2005). Instead we created daily predictions of thermal-habitat suitability for each study species at locations (n = 50) of their known occurrence randomly sampled from the historical occurrence dataset. The median of these values was then selected as the threshold for suitable thermal conditions for each species. Median values were selected (as opposed to minimum values or low quartiles) because these provide an estimate of the thermal conditions capable of supporting the occurrence of high abundances of the study species, rather than a lower level of thermal-habitat suitability that may support only the occurrence of smaller numbers of individuals in space and time. This was considered conservative, given that our analyses aim to relate changes in the temporal persistence of suitable thermal conditions for the study species to opportunities that divers have to encounter these species, and sufficiently high abundances are required to facilitate encounters. Furthermore, applying this data-driven approach for identifying threshold values maximises the agreement between modelled and observed species distributions, ultimately optimising the accuracy of temporal-persistence estimates (Champion et al. 2019).
Changes in the temporal persistence of suitable thermal conditions for manta rays and zebra sharks at dive locations over the future projections period was assessed using linear models. Slope coefficients and confidence intervals from model fits were used to estimate rates of change in the number of months per year that suitable thermal conditions were present at dive locations. P-values were assessed to determine whether slopes for each species at each dive location were significantly different from zero. Residuals from these models were linearly related to theoretical quantiles and no patterns in residual variation were evident, indicating that our analyses satisfied statistical assumptions. Data analysis was undertaken using the R software (ver. 4.2.3, R Foundation for Statistical Computing, Vienna, Austria, see https://www.r-project.org/), with GAMMs fitted using the gamm4 package (ver. 0.2-6, see https://cran.r-project.org/package=gamm4), k-fold cross-validation undertaken using the dismo package (ver 1.3-9, see https://cran.r-project.org/package=dismo), spatial data aggregated using the raster package (ver. 3.6-20, see https://cran.r-project.org/package=raster) and figures generated using the ggplot2 package (ver. 3.4.1, see https://CRAN.R-project.org/package=ggplot2; Wickham 2016).
Results
Habitat-suitability models
Multiple dynamic oceanographic variables were found to be significant linear and non-linear predictors of the occurrence of manta rays and zebra sharks off eastern Australia (Fig. 2, Table 3). SST had a unimodal effect on environmental habitat suitability for both study species, with peaks in optimal thermal habitat occurring between 23 and 24.5°C for manta rays and 24.5 and 26°C for zebra sharks (Fig. 2). The occurrence of both study species was associated with a negative linear response to EKE (i.e. current velocity), indicating a preference for environments characterised by low to moderate current velocity. Zebra shark occurrence was also associated with a negative linear response to SLA, whereas there was no significant association between manta ray occurrence and SLA. Both species also responded to the concentration of chlorophyll in seawater, with increases in chlorophyll concentration being generally associated with non-linear improvements in environmental habitat suitability (Fig. 2).
Partial effects of covariates on the fitted values of the best manta ray (Mobula alfredi) and zebra shark (Stegostoma tigrinum) environmental-suitability models. Grey shading denotes 95% confidence intervals around the model fit (black line). SST, sea-surface temperature; EKE, eddy kinetic energy; SLA, sea-level anomaly; CHL, chlorophyll-a concentration.
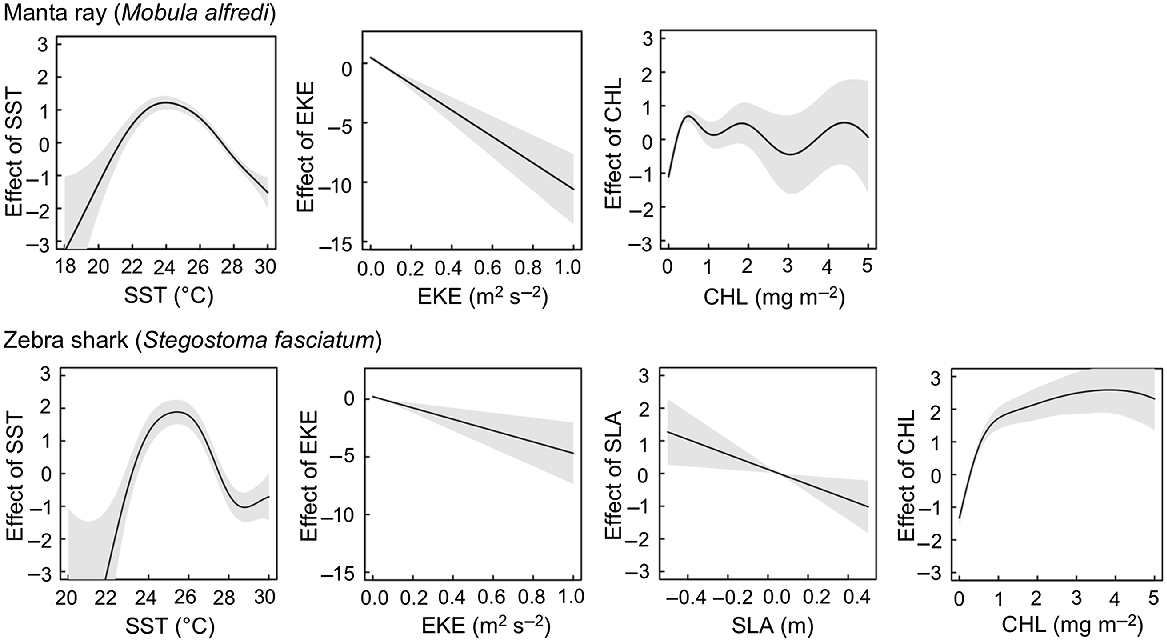
Variable | Effective degrees of freedom | P-value | |||
---|---|---|---|---|---|
Best model | Temperature-only model | Best model | Temperature-only model | ||
Manta ray | |||||
s(SST) | 4.77 | 5.10 | <0.001 | <0.001 | |
EKE | A | – | <0.001 | – | |
s(CHL) | 6.51 | – | <0.001 | – | |
Year(intercept) | A | A | <0.001 | <0.001 | |
Zebra shark | |||||
s(SST) | 5.58 | 5.72 | <0.001 | <0.001 | |
EKE | A | – | <0.001 | – | |
SLA | A | – | 0.01 | – | |
s(CHL) | 5.53 | – | <0.001 | – | |
Year(intercept) | A | A | <0.001 | <0.001 |
Importantly, SST was found to be a dominant predictor of the occurrence of manta rays and zebra sharks off eastern Australia. Six-fold model cross-validation showed that only minor reductions in model predictive skill, as assessed using AUC (Δ < 0.07) and TSS (Δ < 0.06) measures, occurred when the best models were reduced to temperature-only models (Table 4). Mean AUC and TSS values associated with temperature-only models for both species consistently exceeded 0.75 and 0.45 respectively (Table 4). These values denote models associated with fair to good performance (Swets 1988; Pearce and Ferrier 2000), indicating that our future projections of species thermal-habitat suitability are likely to provide accurate approximations of species spatial distributions under ocean warming.
Model type | Model variables | Mean AUC (±s.d.) | ΔAUC | Mean TSS (±s.d.) | ΔTSS | |
---|---|---|---|---|---|---|
Manta ray | ||||||
Best model | s(SST) + EKE + s(CHL) + (1|Year) | 0.792 ± 0.017 | 0.016 | 0.479 ± 0.053 | 0.017 | |
Temperature-only | s(SST) + (1|Year) | 0.776 ± 0.002 | 0.462 ± 0.001 | |||
Zebra shark | ||||||
Best model | s(SST) + EKE + SLA + s(CHL) + (1|Year) | 0.845 ± 0.010 | 0.066 | 0.547 ± 0.018 | 0.058 | |
Temperature-only | s(SST) + (1|Year) | 0.779 ± 0.013 | 0.489 ± 0.007 |
Smoothing factors are indicated by ‘s’. Area under the receiver-operating curve (AUC) statistic and true skill statistic (TSS) are derived from six-fold model validation and delta (Δ) AUC and TSS values quantify changes in model skill identified when the best models were reduced to temperature-only models. EKE, eddy kinetic energy; SLA, sea-level anomaly; CHL, chlorophyll-a concentration.
Spatial projections of thermal-habitat suitability
Spatial analyses highlighted that suitable thermal habitat for manta rays and zebra sharks undergo a poleward shift to higher latitudes along Australia’s eastern coast during the Austral summer and autumn, and an equatorward retreat during the cooler winter and spring seasons (Fig. 3, 4). Seasonal shifts in the distribution of thermal habitat for manta rays and zebra sharks along the eastern coast of Australia are projected to be exacerbated under future ocean warming, with suitable thermal habitat for both species projected to occur further south in all seasons under ocean warming (Fig. 3, 4).
Thermal-habitat suitability for manta rays (Mobula alfredi) off eastern Australia predicted for (a) the 20-year period centred on 2010, and projected for (b) the 20-year period centred on 2050. (c) The change in thermal-habitat suitability for manta rays between 2010- and 2050-centred periods. Monthly spatial predictions were averaged for each period and seasonally aggregated (summer, December–February; autumn, March–May; winter, June–August; spring, September–November). Predictions have been made nearshore of the continental shelf-break (i.e. 200-m isobath).
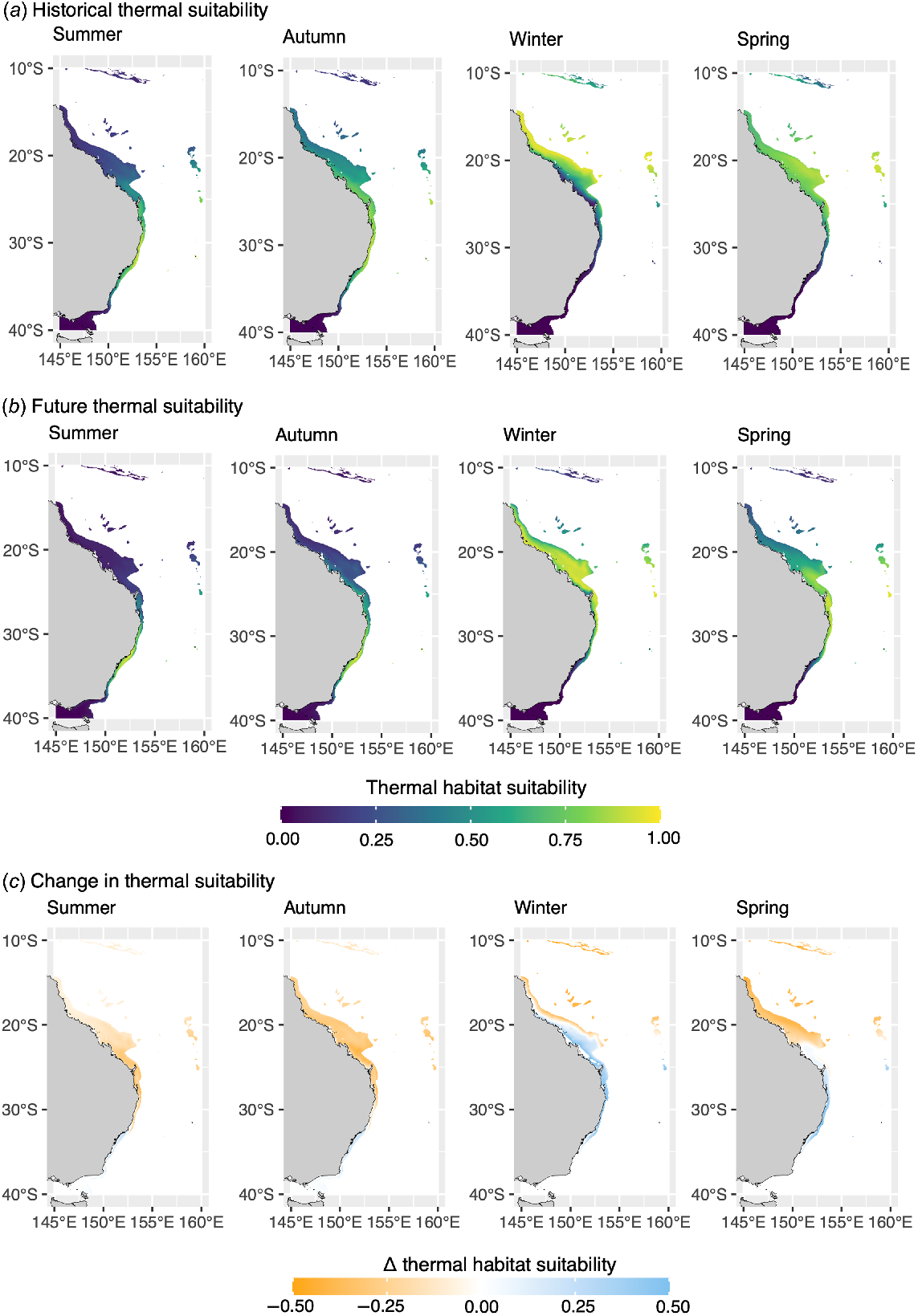
Thermal-habitat suitability for zebra sharks (Stegostoma tigrinum) off eastern Australia predicted for (a) the 20-year period centred on 2010, and projected for (b) the 20-year period centred on 2050. (c) The change in thermal habitat suitability for zebra sharks between 2010- and 2050-centred periods. Monthly spatial predictions were averaged for each period and seasonally aggregated (summer, December–February; autumn, March–May; winter, June–August; spring, September–November). Predictions have been made nearshore of the continental shelf-break (i.e. 200-m isobath).
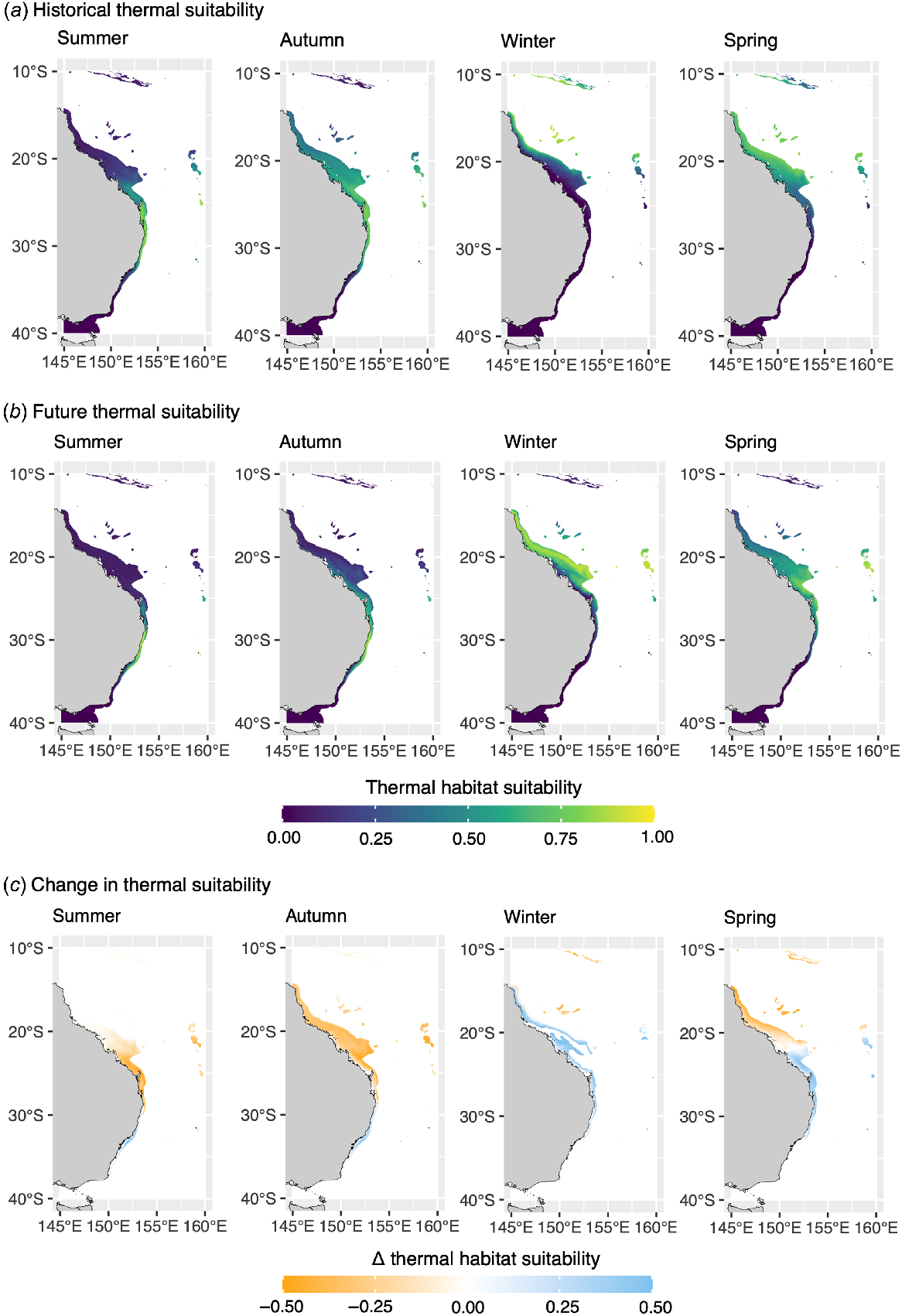
Future temporal persistence at dive locations
Significant increases in the temporal persistence of suitable thermal habitat for manta rays and zebra sharks are projected at all dive locations distributed between Forster (32.19°S) and Jervis Bay (35.19°S) under future ocean warming (Fig. 5, Table 5). Furthermore, a significant increase in the temporal persistence of suitable thermal habitat for zebra sharks at Coffs Harbour is also projected in the future (Fig. 5, Table 5). Contrastingly, no significant changes in suitable thermal habitat for manta rays are projected for sites from South West Rocks to Byron Bay and for zebra sharks at South West Rocks and at Byron Bay (Fig. 5, Table 5).
Temporal trends in the projected annual persistence of suitable thermal habitat for manta rays (left-side panel) and zebra sharks (right-side panel) at key dive locations from Australia’s eastern coast. Data presented are based on monthly spatial projections of thermal-habitat suitability by using an ensemble of downscaled sea-surface temperature data forced under the SSP5-8.5 scenario between 2015 and 2059.
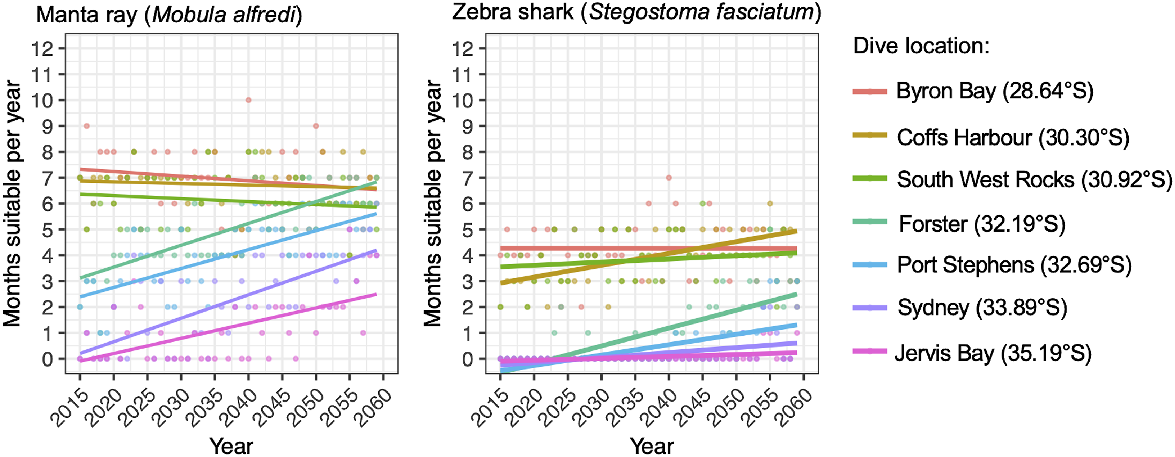
Dive location | F1,43 | P-value | Slope (±95% CI) | Projected change in temporal persistence (months per year ± 95% CI) | |
---|---|---|---|---|---|
Manta ray (Mobula alfredi) | |||||
Byron Bay | 1.73 | 0.195 | – | – | |
Coffs Harbour | 0.294 | 0.590 | – | – | |
South West Rocks | 0.802 | 0.375 | – | – | |
Forster | 70.88 | <0.001 | 0.085 (±0.020) | 3.80 (±0.91) | |
Port Stephens | 46.64 | <0.001 | 0.073 (±0.022) | 3.29 (±0.97) | |
Sydney | 49.21 | <0.001 | 0.091 (±0.026) | 4.08 (±1.17) | |
Jervis Bay | 21.27 | <0.001 | 0.058 (±0.026) | 2.64 (±1.16) | |
Zebra shark (Stegostoma tigrinum) | |||||
Byron Bay | <0.001 | 0.983 | – | – | |
Coffs Harbour | 20.71 | <0.001 | 0.046 (±0.020) | 2.06 (± 0.19) | |
South West Rocks | 1.24 | 0.27 | – | – | |
Forster | 45.05 | <0.001 | 0.069 (±0.021) | 3.11 (±0.94) | |
Port Stephens | 25.43 | <0.001 | 0.040 (±0.016) | 1.81 (±0.72) | |
Sydney | 12.58 | <0.001 | 0.019 (±0.011) | 0.87 (±0.50) | |
Jervis Bay | 7.76 | 0.008 | 0.008 (±0.005) | 0.34 (±0.24) |
Significant P-values (<0.05) are shown in bold.
The number of months per year that suitable thermal conditions for manta rays are projected to occur, are projected to increase between 2015 and 2059, with increases ranging between ~2.5 (i.e. at Jervis Bay) and 4 (i.e. at Sydney) months per year. For zebra sharks, increases of between ~0.5 and 3 months per year are projected across dive locations in the future-projection period (Table 5). Importantly, the absolute number of months per year that suitable thermal conditions are projected to occur in the future follows a latitudinal trend for both study species, with larger increases in the number of months per year projected at lower-latitude dive locations, albeit with this trend being more consistent for manta rays (Table 5, Fig. 5).
Discussion
Tourism is the largest industry connected to the NSW marine estate, contributing an estimated A$4.3 billion to this sector during the 2021–22 period (Deloitte 2023). This economic contribution represents 27% of the total income generated by the NSW marine estate, with a portion of this being derived from expenditure on trips that involve marine-based activities, such as snorkelling, scuba-diving and dolphin and whale watching (Deloitte 2023). The success of these ecotourism activities often relies on encounters with iconic marine megafauna, and climate-driven shifts in the distribution of marine species in NSW (Gervais et al. 2021) have the potential to affect (both positively and negatively) opportunities for encounters. Here, we show that the timing of manta ray and zebra shark seasonal migrations in eastern Australia is likely to be altered by future ocean warming, with the persistence of suitable thermal conditions for manta rays and zebra sharks rising by up to 4 months by the mid-21st century in central NSW (32–36°S) during the austral spring and summer.
While manta ray and shark tourism industries are currently in their infancy in NSW, projected increases to the temporal persistence of suitable thermal conditions for manta rays and zebra sharks is likely to increase the ecotourism potential for these species in this region. Specifically, tourism operators in central NSW are expected to benefit from novel ecotourism opportunities, owing to increased residence of manta rays and zebra sharks over the austral spring and summer period. Contrastingly, in northern NSW, changes to temporal persistence for manta rays and zebra sharks are likely to be negligible, with existing tourism operators unlikely to benefit substantially from shifting migration patterns. Instead, the timings of manta ray and zebra shark migrations into northern NSW are likely to shift as oceans warm and tourism operators will need to plan for corresponding shifts in the timing of their peak dive-tourism season going forward. Overall, the impacts of ocean warming on the temporal persistence for manta rays and zebra sharks will vary among regions in eastern Australia and is likely to result in both increases (i.e. central NSW) and negligible change (i.e. northern NSW) in the annual persistence of these species across the popular diving locations assessed here.
Effect of environmental variables on current species-migration patterns
Sea-surface temperatures were found to be the dominant factor associated with the presence of manta rays and zebra sharks off eastern Australia, with suitability for the occurrence of manta rays peaking when sea-surface temperatures range between 23 and 24.5°C for manta rays and between 24.5 and 26°C for zebra sharks. Spatial analyses highlighted that suitable thermal conditions for these species undergo a poleward shift towards higher latitudes during the austral summer and autumn, and an equatorward retreat during the cooler winter and spring seasons. Our results are consistent with previous research on movements of manta rays, conducted at Lady Elliott Island in Queensland, which found that some manta rays migrate south, to North Stradbroke Island and Byron Bay, from mid-spring to mid-autumn (Couturier et al. 2011). Model predictions are also consistent with seasonal occurrence data for zebra sharks in south-eastern Queensland, which identified that zebra sharks start to move into this area in November, once ocean temperatures reached 22°C and then migrate further south as temperature increased above this threshold (Dudgeon et al. 2013).
In addition to moving in response to shifting ocean temperatures, occurrences of manta rays and zebra sharks were also found to vary with current velocity and chlorophyll concentration, with both species exhibiting a preference for regions with low to moderate current velocities and increased chlorophyll concentrations. Increased presence of manta rays in areas with high chlorophyll concentrations is understandable, given that high chlorophyll concentrations generally indicate high concentrations of plankton (Jakobsen and Markager 2016) and manta rays are planktonic filter feeders that can migrate large distances to feed in plankton-rich waters (Graham et al. 2012; Jaine et al. 2014). For zebra sharks, increased presence in regions with high chlorophyll concentrations is harder to explain, although potentially higher chlorophyll concentrations and associated high plankton concentrations may increase abundance of their food (molluscs), with molluscs forming the bulk of the diet for zebra sharks (Cortés 1999). The effects of current strength on manta ray and zebra shark distributions are likely to be more complex, although it can be argued that light–moderate currents provide preferrable conditions, allowing easy movement and feeding, whereas stronger currents are known to inhibit fish movement (Caldwell and Gergel 2013), with strong currents also being associated with lower manta ray sightings in Mozambique (Rohner et al. 2013).
Implications for fisheries management
In addition to affecting dive tourism in NSW, changes to the migration patterns for manta rays and zebra sharks have implications for fisheries management. Zebra sharks are known to be caught as bycatch in Queensland (Chin et al. 2012) and elsewhere (Pottie et al. 2021). Manta rays have been recorded as bycatch under the NSW Shark Meshing (Bather Protection) Program (SMP; Broadhurst and Cullis 2020), with 0.30 entanglements per year from 1950 to 1993 (Krogh and Reid 1996) and 0.53 per year from 2009 to 2024 (M. Green, pers. comm.). Given the projected increased presence and southward range extensions of these species in NSW, there is potential for increasing exposure to SMP and fishing gears. Consequently, fisheries management plans should continue to monitor interactions between species and SMP and other fishing gear into the future and examine whether changes to management policies are needed to address these and any other identified threats.
Conclusions
Changes to future climatic conditions, particularly rising ocean temperatures, will increase habitat suitability for manta rays and zebra shark in central NSW, with suitable thermal conditions for both species projected to occur further south in all seasons by 2050 and temporal persistence of suitable conditions rising by up to 4 months over the austral spring and summer. Consequently, novel ecotourism opportunities are likely to emerge that enable interactions with these iconic marine megafauna in NSW. These changes represent a novel future opportunity to enhance people’s engagement with iconic marine megafauna in the NSW marine estate, which has the potential to provide additional economic benefits to the tourism industry that facilitates these interactions.
Data availability
Most of the data that support this study are available in the article and accompanying online supplementary material. Data not able to be presented in this way will be shared upon reasonable request to the corresponding author.
Declaration of funding
This research was funded by the New South Wales Marine Estate Management Strategy.
Acknowledgements
We are grateful to Dr James R. Lawson for his valuable technical assistance downscaling future sea-surface temperature data from a suite of global climate models.
References
Allouche O, Tsoar A, Kadmon R (2006) Assessing the accuracy of species distribution models: prevalence, kappa and the true skill statistic (TSS). Journal of Applied Ecology 43, 1223-1232.
| Crossref | Google Scholar |
Anderson RC, Adam MS, Kitchen-Wheeler A-M, Stevens G (2011) Extent and economic value of manta ray watching in Maldives. Tourism in Marine Environments 7, 15-27.
| Crossref | Google Scholar |
Araújo MB, Pearson RG, Thuiller W, Erhard M (2005) Validation of species–climate impact models under climate change. Global Change Biology 11, 1504-1513.
| Crossref | Google Scholar |
Armstrong AO, Armstrong AJ, Bennett MB, Richardson AJ, Townsend KA, Dudgeon CL (2019) Photographic identification and citizen science combine to reveal long distance movements of individual reef manta rays Mobula alfredi along Australia’s east coast. Marine Biodiversity Records 12, 14.
| Crossref | Google Scholar |
Armstrong AJ, Armstrong AO, Bennett MB, McGregor F, Abrantes KG, Barnett A, Richardson AJ, Townsend KA, Dudgeon CL (2020) The geographic distribution of reef and oceanic manta rays (Mobula alfredi and Mobula birostris) in Australian coastal waters. Journal of Fish Biology 96, 835-840.
| Crossref | Google Scholar | PubMed |
Bailey S-A, Haines-Young RH, Watkins C (2002) Species presence in fragmented landscapes: modelling of species requirements at the national level. Biological Conservation 108, 307-316.
| Crossref | Google Scholar |
Barbet-Massin M, Jiguet F, Albert CH, Thuiller W (2012) Selecting pseudo-absences for species distribution models: how, where and how many? Methods in Ecology and Evolution 3, 327-338.
| Crossref | Google Scholar |
Barnett A, Jaine FRA, Bierwagen SL, Lubitz N, Abrantes K, Heupel MR, Harcourt R, Huveneers C, Dwyer RG, Udyawer V, Simpfendorfer CA, Miller IB, Scott-Holland T, Kilpatrick CS, Williams SM, Smith D, Dudgeon CL, Hoey AS, Fitzpatrick R, Osborne FE, Smoothey AF, Butcher PA, Sheaves M, Fisher EE, Svaikauskas M, Ellis M, Kanno S, Cresswell BJ, Flint N, Armstrong AO, Townsend KA, Mitchell JD, Campbell M, Peddemors VM, Gustafson JA, Currey-Randall LM (2024) From little things big things grow: enhancement of an acoustic telemetry network to monitor broad-scale movements of marine species along Australia’s east coast. Movement Ecology 12, 31.
| Crossref | Google Scholar |
Broadhurst MK, Cullis BR (2020) Mitigating the discard mortality of non-target, threatened elasmobranchs in bather-protection gillnets. Fisheries Research 222, 105435.
| Crossref | Google Scholar |
Brodie S, Hobday AJ, Smith JA, Everett JD, Taylor MD, Gray CA, Suthers IM (2015) Modelling the oceanic habitats of two pelagic species using recreational fisheries data. Fisheries Oceanography 24, 463-477.
| Crossref | Google Scholar |
Caldwell IR, Gergel SE (2013) Thresholds in seascape connectivity: influence of mobility, habitat distribution, and current strength on fish movement. Landscape Ecology 28, 1937-1948.
| Crossref | Google Scholar |
Champion C, Hobday AJ, Tracey SR, Pecl GT (2018) Rapid shifts in distribution and high-latitude persistence of oceanographic habitat revealed using citizen science data from a climate change hotspot. Global Change Biology 24, 5440-5453.
| Crossref | Google Scholar | PubMed |
Champion C, Hobday AJ, Zhang X, Pecl GT, Tracey SR (2019) Changing windows of opportunity: past and future climate-driven shifts in temporal persistence of kingfish (Seriola lalandi) oceanographic habitat within south-eastern Australian bioregions. Marine and Freshwater Research 70, 33-42.
| Crossref | Google Scholar |
Champion C, Brodie S, Coleman MA (2021) Climate-driven range shifts are rapid yet variable among recreationally important coastal-pelagic fishes. Frontiers in Marine Science 8, 622299.
| Crossref | Google Scholar |
Champion C, Hobday AJ, Zhang X, Coleman MA (2022) Climate change alters the temporal persistence of coastal-pelagic fishes off eastern Australia. ICES Journal of Marine Science 79, 1083-1097.
| Crossref | Google Scholar |
Chin A, Tobin A, Simpfendorfer C, Heupel M (2012) Reef sharks and inshore habitats: patterns of occurrence and implications for vulnerability. Marine Ecology Progress Series 460, 115-125.
| Crossref | Google Scholar |
Cisneros-Montemayor AM, Barnes-Mauthe M, Al-Abdulrazzak D, Navarro-Holm E, Sumaila UR (2013) Global economic value of shark ecotourism: implications for conservation. Oryx 47, 381-388.
| Crossref | Google Scholar |
Cortés E (1999) Standardized diet compositions and trophic levels of sharks. ICES Journal of Marine Science 56, 707-717.
| Crossref | Google Scholar |
Couturier LIE, Jaine FRA, Townsend KA, Weeks SJ, Richardson AJ, Bennett MB (2011) Distribution, site affinity and regional movements of the manta ray, Manta alfredi (Krefft, 1868), along the east coast of Australia. Marine and Freshwater Research 62, 628-637.
| Crossref | Google Scholar |
Couturier LIE, Newman P, Jaine FRA, Bennett MB, Venables WN, Cagua EF, Townsend KA, Weeks SJ, Richardson AJ (2018) Variation in occupancy and habitat use of Mobula alfredi at a major aggregation site. Marine Ecology Progress Series 599, 125-145.
| Crossref | Google Scholar |
Dudgeon CL, Lanyon JM, Semmens JM (2013) Seasonality and site fidelity of the zebra shark, Stegostoma fasciatum, in southeast Queensland, Australia. Animal Behaviour 85, 471-481.
| Crossref | Google Scholar |
Eveson JP, Hobday AJ, Hartog JR, Spillman CM, Rough KM (2015) Seasonal forecasting of tuna habitat in the Great Australian Bight. Fisheries Research 170, 39-49.
| Crossref | Google Scholar |
Gervais CR, Champion C, Pecl GT (2021) Species on the move around the Australian coastline: a continental-scale review of climate-driven species redistribution in marine systems. Global Change Biology 27, 3200-3217.
| Crossref | Google Scholar | PubMed |
Graham RT, Witt MJ, Castellanos DW, Remolina F, Maxwell S, Godley BJ, Hawkes LA (2012) Satellite tracking of manta rays highlights challenges to their conservation. PLoS ONE 7, e36834.
| Crossref | Google Scholar |
Harriott VJ, Davis D, Banks SA (1997) Recreational diving and its impact in marine protected areas in eastern Australia. Ambio 26, 173-179.
| Google Scholar |
Hobday AJ, Hartog JR (2014) Derived ocean features for dynamic ocean management. Oceanography 27, 134-145.
| Crossref | Google Scholar |
Huveneers C, Meekan MG, Apps K, Ferreira LC, Pannell D, Vianna GMS (2017) The economic value of shark-diving tourism in Australia. Reviews in Fish Biology and Fisheries 27, 665-680.
| Crossref | Google Scholar |
Jaine FRA, Rohner CA, Weeks SJ, Couturier LIE, Bennett MB, Townsend KA, Richardson AJ (2014) Movements and habitat use of reef manta rays off eastern Australia: offshore excursions, deep diving and eddy affinity revealed by satellite telemetry. Marine Ecology Progress Series 510, 73-86.
| Crossref | Google Scholar |
Jakobsen HH, Markager S (2016) Carbon-to-chlorophyll ratio for phytoplankton in temperate coastal waters: seasonal patterns and relationship to nutrients. Limnology and Oceanography 61, 1853-1868.
| Crossref | Google Scholar |
Johnston A, Hochachka WM, Strimas-Mackey ME, Gutierrez VR, Robinson O, Miller E, Auer T, Kelling S, Fink D (2021) Analytical guidelines to increase the value of communityscience data: an example using eBird data to estimate species distributions. Diversity and Distributions 27, 1265-1277.
| Crossref | Google Scholar |
Krogh M, Reid D (1996) Bycatch in the protective shark meshing programme off south-eastern New South Wales, Australia. Biological Conservation 77, 219-226.
| Crossref | Google Scholar |
Liu C, Berry PM, Dawson TP, Pearson RG (2005) Selecting thresholds of occurrence in the prediction of species distributions. Ecography 28, 385-393.
| Crossref | Google Scholar |
Meynecke J-O, Richards R, Sahin O (2017) Whale watch or no watch: the Australian whale watching tourism industry and climate change. Regional Environmental Change 17, 477-488.
| Crossref | Google Scholar |
Morley JW, Selden RL, Latour RJ, Frölicher TL, Seagraves RJ, Pinsky ML (2018) Projecting shifts in thermal habitat for 686 species on the North American continental shelf. PLoS ONE 13, e0196127.
| Crossref | Google Scholar |
Murray A, Garrud E, Ender I, Lee-Brooks K, Atkins R, Lynam R, Arnold K, Roberts C, Hawkins J, Stevens G (2020) Protecting the million-dollar mantas; creating an evidence-based code of conduct for manta ray tourism interactions. Journal of Ecotourism 19, 132-147.
| Crossref | Google Scholar |
Navarro-Racines C, Tarapues J, Thornton P, Jarvis A, Ramirez-Villegas J (2020) High-resolution and bias-corrected CMIP5 projections for climate change impact assessments. Scientific Data 7, 7.
| Crossref | Google Scholar |
Organisation for Economic Co-operation and Development (2016) ‘The ocean economy in 2030.’ (OECD: Paris, France) Available at https://www.oecd.org/en/publications/2016/04/the-ocean-economy-in-2030_g1g6439e.html
O’Malley MP, Lee-Brooks K, Medd HB (2013) The global economic impact of manta ray watching tourism. PLoS ONE 8, e65051.
| Crossref | Google Scholar |
Pearce JL, Boyce MS (2006) Modelling distribution and abundance with presence-only data. Journal of Applied Ecology 43, 405-412.
| Crossref | Google Scholar |
Pearce J, Ferrier S (2000) Evaluating the predictive performance of habitat models developed using logistic regression. Ecological Modelling 133, 225-245.
| Crossref | Google Scholar |
Pecl GT, Araújo MB, Bell JD, Blanchard J, Bonebrake TC, Chen I-C, Clark TD, Colwell RK, Danielsen F, Evengård B, Falconi L, Ferrier S, Frusher S, Garcia RA, Griffis RB, Hobday AJ, Janion-Scheepers C, Jarzyna MA, Jennings S, Lenoir J, Linnetved HI, Martin VY, McCormack PC, McDonald J, Mitchell NJ, Mustonen T, Pandolfi JM, Pettorelli N, Popova E, Robinson SA, Scheffers BR, Shaw JD, Sorte CJB, Strugnell JM, Sunday JM, Tuanmu M-N, Vergés A, Villanueva C, Wernberg T, Wapstra E, Williams SE (2017) Biodiversity redistribution under climate change: impacts on ecosystems and human well-being. Science 355, eaai9214.
| Crossref | Google Scholar |
Pottie S, Flam AL, Keeping JA, Chivindze C, Bull JC (2021) Quantifying the distribution and site fidelity of a rare, non-commercial elasmobranch using local ecological knowledge. Ocean & Coastal Management 212, 105796.
| Crossref | Google Scholar |
Rogers LA, Griffin R, Young T, Fuller E, St. Martin K, Pinsky ML (2019) Shifting habitats expose fishing communities to risk under climate change. Nature Climate Change 9, 512-516.
| Crossref | Google Scholar |
Rohner CA, Pierce SJ, Marshall AD, Weeks SJ, Bennett MB, Richardson AJ (2013) Trends in sightings and environmental influences on a coastal aggregation of manta rays and whale sharks. Marine Ecology Progress Series 482, 153-168.
| Crossref | Google Scholar |
Schulzweida U (2019) CDO user guide: Climate Data Operator, Version 1.9.6, February 2019. In Zenodo, 6 February 2019. 10.5281/zenodo.2558193
Spillman CM, Alves O (2009) Dynamical seasonal prediction of summer sea surface temperatures in the Great Barrier Reef. Coral Reefs 28, 197-206.
| Crossref | Google Scholar |
Stockwell DRB, Peterson AT (2002) Effects of sample size on accuracy of species distribution models. Ecological Modelling 148, 1-13.
| Crossref | Google Scholar |
Swets JA (1988) Measuring the accuracy of diagnostic systems. Science 240, 1285-1293.
| Crossref | Google Scholar | PubMed |
Topelko KN, Dearden P (2005) The shark watching industry and its potential contribution to shark conservation. Journal of Ecotourism 4, 108-128.
| Crossref | Google Scholar |
Venables S, McGregor F, Brain L, van Keulen M (2016) Manta ray tourism management, precautionary strategies for a growing industry: a case study from the Ningaloo Marine Park, Western Australia. Pacific Conservation Biology 22, 295-300.
| Crossref | Google Scholar |
von Hammerstein H, Setter RO, van Aswegen M, Currie JJ, Stack SH (2022) High-resolution projections of global sea surface temperatures reveal critical warming in humpback whale breeding grounds. Frontiers in Marine Science 9, 837772.
| Crossref | Google Scholar |