Biogenic CO2 flux uncertainty: numerical experiments and validation over south-eastern South America
Nahuel E. Bautista
A
B
C
D
E
Abstract
Understanding carbon dioxide (CO2) surface fluxes is essential in the context of a changing climate. In particular, agriculture significantly contributes to CO2 fluxes. Recently, some studies have focused on understanding how synoptic-scale variability modulates CO2 fluxes associated with vegetation and agriculture, finding that frontal passages and precipitation events exert a strong influence on these fluxes. This variability is particularly relevant in the Argentinean Pampas, where large CO2 fluxes associated with extensive agriculture combine with strong synoptic variability. Numerical modelling provides a valuable tool for investigating surface CO2 fluxes and their variability, despite the uncertainties associated with the model’s limitations. In this work, we investigate simulated CO2 fluxes in the Argentinean Pampas using the Weather Research and Forecasting Model (WRF) coupled with the Vegetation, Respiration and Photosynthesis Model (VPRM) over three case studies representing different synoptic-scale conditions. In addition, we estimate the uncertainty in the simulations by comparing simulated CO2 fluxes using various WRF configurations and the ERA5 reanalysis. We found that the synoptic-scale conditions have a considerable impact on the magnitude of fluxes as well as the simulation uncertainty. Uncertainties in simulated CO2 fluxes can be as high as 60%, being larger at sunrise and sunset. Also, the largest uncertainty is associated with a case with a cold frontal passage and widespread precipitation. These results allow a more accurate estimation of CO2 flux uncertainty, which is key to understanding the effects of climate change.
Keywords: carbon dioxide, ecosystem respiration, ERA5 reanalysis, frontal passages, gross primary productivity, model validation, model uncertainties, net ecosystem exchange, numerical modelling, sensitivity analysis, Weather Research and Forecasting, WRF.
1.Introduction
Providing correct estimates of greenhouse gases (GHG) exchanged between the land and the atmosphere and of GHG atmospheric transport is mandatory to test the validity of bottom–up and top–down approaches employed to calculate the national and regional carbon budgets necessary to adapt to climate change (Parazoo et al. 2008; Chevallier et al. 2019; Peiro et al. 2022). Terrestrial ecosystems emit and capture carbon dioxide (CO2) to and from the atmosphere through their ecosystem respiration (Reco) and their gross primary productivity (GPP) respectively. The net ecosystem exchange (NEE) is defined as NEE = Reco – GPP, and it represents the net CO2 flux exchanged between the atmosphere and an ecosystem. Recently, how frontal passages and precipitation events influence NEE and CO2 atmospheric transport was analysed (Hurwitz et al. 2004; Huxman et al. 2004; Lee et al. 2012; Pal et al. 2020; Hu et al. 2021). For instance, Hurwitz et al. (2004) quantified the influence of precipitation on Reco, GPP and NEE across time, and Hu et al. (2021) found a CO2 concentration gradient across frontal zones. Furthermore, colder and anticyclonic circulation areas behind cold fronts can enhance GPP and diminish Reco, leading to an increase in carbon uptake by vegetation. However, it is unclear to what extent these conclusions can be extrapolated to other regions and climatological contexts because each location has its own topographic, climatological, soil and biological characteristics that influence NEE and CO2 atmospheric transport (Oke 2002; Parazoo et al. 2008; Baldocchi et al. 2018).
NEE measurements through the eddy covariance technique have provided significant understanding of a great deal of ecosystem functioning and its impact on CO2 atmospheric balance (Baldocchi 2020). These measurements are specific and representative of a very limited area associated with the annual footprint of a micrometeorological tower. However, the Vegetation Photosynthesis and Respiration Model (VPRM; Xiao et al. 2004; Mahadevan et al. 2008), recently implemented in the Weather Research and Forecasting Model coupled with Chemistry (WRF-Chem; Skamarock et al. 2019), has been an effective tool for modelling carbon dioxide exchange between vegetation and the atmosphere, and its atmospheric advection at a regional scale (Dayalu et al. 2018; Hu et al. 2021; Gourdji et al. 2022). VPRM needs as input variables WRF estimates of near-surface air temperature and solar radiation that arrives at the surface, which are highly correlated with Reco and GPP respectively (Lloyd and Taylor 1994; Lasslop et al. 2010; Baldocchi et al. 2018; Bautista et al. 2023).
Numerical simulations of the atmosphere’s [measured] parameters, as produced by the WRF model, consist of solving the governing equations of atmospheric flow over a predefined three-dimensional grid inside a region of interest or domain. However, subgrid processes, such as turbulent mixing in the atmospheric boundary layer (ABL), and soil processes are not solved explicitly by the model and they need to be estimated by one of the many parameterisation schemes included in WRF. The selection of these schemes is not trivial because it may lead to different forecast values of variables over time. Moreover, previous studies suggest that there is no single combination of schemes that provides the best performance under all weather conditions and for all simulated variables (e.g. Ruiz et al. 2010; Alvarez Imaz et al. 2021; Casaretto et al. 2022). The uncertainty associated with parameterisation schemes can propagate as uncertainties in estimating Reco and GPP, the two components of CO2 flux. As an example of this, Reco is positively correlated with temperature (Lloyd and Taylor 1994); thus, uncertainty in near-surface air temperature estimates will negatively influence the estimation of Reco fluxes. Moreover, errors in the estimation of other variables can also propagate to CO2 flux uncertainties (Hilton et al. 2014) if they influence air temperature. For instance, precipitation events generate cold pools at the surface level (Markowski and Richardson 2011). If they are poorly represented, the surface temperature will not be accurate, increasing the uncertainty in Reco estimation. As another example, ABL structure influences near-surface air temperature through turbulent mixing (Stull 1988); thus, incorrect estimates of temperatures along the ABL will propagate to the surface temperature and, after, to the fluxes. These examples illustrate that it is necessary to estimate all the variables in the simulations to accurately represent the CO2 fluxes.
Estimates from 2018 indicated that ~0.9% of total global GHG emissions (0.10 Pg C) came from Argentina, with 39% from agriculture, livestock, forestry and other activities, these being carried out in the most productive region of Argentina (MPRArg; Crippa et al. 2021; Ministerio de Ambiente y Desarrollo Sostenible 2021). The observed and projected increases in temperature, precipitation, global radiation and vegetation greenness have a positive effect on Reco and GPP (Barros et al. 2015; Long et al. 2023). However, changes in land cover types and management practices over the region have higher variability and uncertainty, which makes their effect on CO2 fluxes unclear (Stanimirova et al. 2022; Long et al. 2023). MPRArg latitudes are between 30 and 40°S, an area with high frontal activity, which is expected to increase as a result of global warming between 2070 and 2100 (Blázquez and Solman 2019). As this region is an important carbon source for the atmosphere, it is important to understand the impact of frontal passages on NEE and the atmospheric transport of CO2 over the territory. However, as a first step to conducting estimations of CO2 fluxes based on numerical simulations, it is important to investigate the uncertainty associated with these simulations and how these uncertainties can propagate to the estimation of CO2 fluxes over this region. Therefore, this study aims to evaluate the sensitivity of the most relevant variables directly or indirectly involved in CO2 flux computation (2-m temperature, shortwave radiation and precipitation) to the choice of model configuration. We also aim to quantify how their uncertainties are propagated into the estimation of CO2 surface fluxes. Simulated variables will be compared with in situ observations to quantify their total uncertainty. We also aim to provide insights on the most appropriate combinations of schemes, focusing on sensitivity to the representation of radiative fluxes, microphysical processes, ABL processes and the choice of the land surface model.
2.Material and methods
2.1. Model simulations and description of the cases
The WRF-Chem v.4.4.2 model was used to simulate the atmospheric dynamics over the MPRArg region with two nested domains. The resolutions of the outer and inner domains were 12 and 4 km in the horizontal direction respectively; thus, no deep moist convection scheme was required for the inner domain (Fig. 1). Eight different combinations of WRF ABL, cloud microphysics and radiative parameterisations were run for three cases with different meteorological conditions. The reanalysis from the European Centre for Medium-Range Weather Forecasts (ERA5; Hersbach et al. 2023a, 2023b) available every 3 h was used as input and boundary conditions, and the WRF outputs were saved every hour. The most relevant parameters and configurations of the WRF simulations are shown in Table 1.
Outer (a) and inner (b, c) domains of the WRF simulations. The symbols indicate the location of the surface synoptic (crosses and circles) and upper-level (diamonds) weather stations used to validate the simulations. The greyscale in (a, b) indicates altitude and in (c) the dominant vegetation class. Measurements include 2-m air temperature (T2), shortwave incoming radiation at the surface (SWDOWN) and precipitaion (PP).
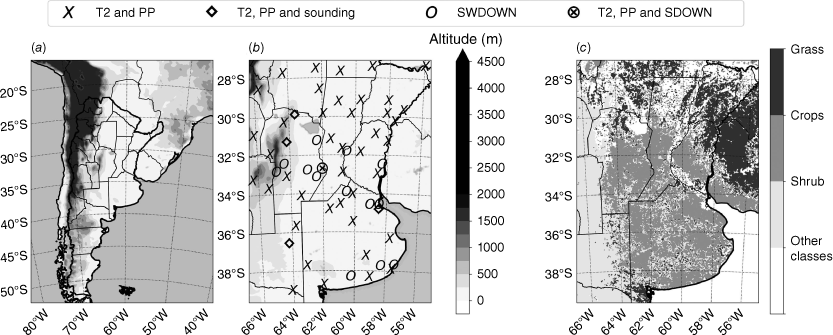
Outer domain | Inner domain | ||
---|---|---|---|
Initial and boundary conditions | ERA5 | Outer domain | |
Horizontal grid resolution (km) | 12 | 4 | |
Number of vertical levels | 50 | 50 | |
Vertical levels below 2500 m (approx.) | 23 | 23 | |
Time step (s) | 30 A | 10 A | |
Spin up (h) | 6 | 6 | |
Longwave radiation scheme | rrtmg (longwave; Iacono et al. 2008) | rrtmg (longwave; Iacono et al. 2008) | |
Convection scheme | Grell 3D (Grell and Devenyi 2002) | – |
Each WRF simulation lasted for 30 h and was initialised at 00:00 hours UTC (Coordinated Universal Time) on 19 November, 24 November and 17 November 2018 (local time GMT–3); these cases were named sunny, cloudy and rainy respectively, owing to their dominant synoptic situations. Fig. 2 shows the 2-m air temperature (T2) diel cycles at five surface weather stations, 925-hPa geopotential height from ERA5 and GOES-16 (Geostationary Operational Environmental Satellite) albedo from Band 2 centred at 0.64 µm for the three selected events. The sunny case had mainly clear skies over the whole domain, except for a small cloudy area that lasted a few hours in the south-western part of the domain. By contrast, the domain was mostly covered by thin, high-level clouds during the cloudy case, but no significant precipitation was recorded during this event. The rainy case was associated with a cold front moving from south-west to north-east across the domain. This cold front triggered organised deep moist convection in the form of a mesoscale convective system at the north-east corner of the study region during the local afternoon, and the station-averaged recorded precipitation was 12.2 mm day−1 and the maximum was 57.0 mm day−1. These three cases represent increasing levels of complexity and presumably increasing uncertainty associated with the computation of surface CO2 fluxes.
T2 station data during the simulated cases for the (a) sunny, (d) cloudy and (g) rainy cases in Gualeguaychú, Rosario, Marcos Juarez, Río Cuarto and Reconquista stations (solid, dashed, dot-dashed, densely dotted and loosely dotted lines respectively). Their geographical coordinates ae −33.00, −32.92, −32.70, 33.12 and −29.18°S; −58.62, −60.78, −62.15, 64.23 and −59.70°W respectively. ERA5 geopotential height at 925 hPa in geopotential metres (mgp) from 16:00 hours UTC (13:00 hours local time) for the (b) sunny, (e) cloudy and (h) rainy cases. GOES-16 albedo from Band 2 centred at 0.64 µm at 16:00 hours UTC for the (c) sunny, (f) cloudy and (i) rainy cases.
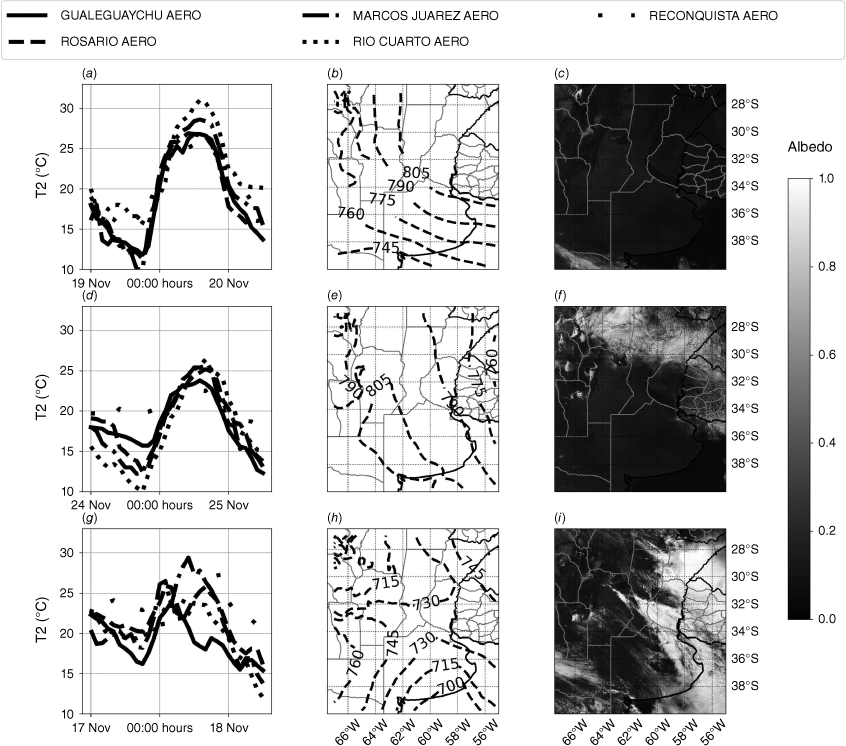
To evaluate the sensitivity of CO2 flux computation, we performed simulations over the three selected cases using different combinations of the parametrisation schemes of short-wave radiation, ABL, microphysics and soil model. The Control experiment of WRF was designed by selecting the same set of parameterisations used by the National Meteorological Service of Argentina (Servicio Meteorológico Nacional, SMN) for their 2-day forecasts (Dillon et al. 2020). To test the sensitivity of the input variables to the model configuration, different runs were carried out changing only one of the schemes used in the Control setting (Table 2). Currently, over 70 physical parameterisations are available in WRF. A full analysis of all these would increase the complexity of the study beyond its scope. Therefore, we limited the number of WRF scenarios to eight. The selected schemes have been used widely by other researchers in the region with positive results (e.g. Ruiz et al. 2010; Ferreyra et al. 2016; Müller et al. 2016; Dillon et al. 2020, 2021; Alvarez Imaz et al. 2021; Casaretto et al. 2022; Suárez et al. 2022; Luque et al. 2024; Merino 2024). A brief description of the eight WRF configurations is detailed in the following subsections.
Scenario | Shortwave radiation scheme | Land surface scheme | Microphysics scheme | ABL scheme | Surface Layer scheme | |
---|---|---|---|---|---|---|
Control | RRTMG_SW | Noah MP | WSM6 | MYJ | Janjic Eta | |
Dudhia | Dudhia | Noah MP | WSM6 | MYJ | Janjic Eta | |
Goddard | Goddard | Noah MP | WSM6 | MYJ | Janjic Eta | |
Noah | RRTMG_SW | Noah | WSM6 | MYJ | Janjic Eta | |
WDM6 | RRTMG_SW | Noah MP | WDM6 | MYJ | Janjic Eta | |
Thompson | RRTMG_SW | Noah MP | Thompson | MYJ | Janjic Eta | |
YSU | RRTMG_SW | Noah MP | WSM6 | YSU | Revised MM5 | |
ACM2 | RRTMG_SW | Noah MP | WSM6 | ACM2 | Revised MM5 |
The schemes that are different from Control are in bold. Acronyms are described in the text.
The shortwave radiation scheme used in most configurations was the Rapid Radiative Transfer Model for general circulation models (RRTMG_SW; Iacono et al. 2008), which solves the Radiative Transfer Equation (RTE) explicitly by integrating it using 14 spectral bands and the contribution of H2O, O2, O3, CO2 and CH4 at two vertical levels. Another shortwave radiation scheme tested was the Dudhia parameterisation (Dudhia; Dudhia 1989), which does not solve the RTE explicitly but by applying a set of experimental relationships. In this way, the opacity of the atmosphere is assumed to be fixed. The third shortwave radiation parameterisation used in our experiments was the Goddard scheme (Goddard; Chou and Suarez 1994), which solves the RTE explicitly by integrating it along 11 spectral bands. It calculates the effect of O3 with a climatological concentration value and of O2 and CO2 as empirical relationships (i.e. they are not explicitly included in the RTE). The CO2 default atmospheric concentration in RRTMG_SW is greater than in Goddard (409 and 401 ppm for 2018 respectively); thus, the atmosphere is less opaque in Goddard.
Two land surface models were tested in this study: the Noah Land Surface Model (Noah; Tewari et al. 2004) and the Noah-Multiparameterisation Land Surface Model (Noah MP; He et al. 2023). The former, Noah, is a scheme that estimates soil temperature and humidity in four layers and considers the snow fraction and frozen soil physics. Noah MP extends the original Noah by adding an extra vegetation layer over the soil and an explicit representation of its impact on the radiative balance. Furthermore, it adds more snow layers that are solved with more detail than in Noah.
The microphysics schemes used in this study were the Single-Moment 6-Class Scheme (WSM6; Hong and Lim 2006), the Thompson scheme (Thompson; Thompson et al. 2008) and the Double-Moment 6-Class Scheme (WDM6; Lim and Hong 2010). All of these classify hydrometeors into six categories (water vapour, cloud water, cloud ice, snow, graupel and rain). The drop size distribution of each category is described as a gamma function with either one or two degrees of freedom. In this way, WSM6 calculates only the mixing ratio of each category; that is, all the gamma functions have one degree of freedom. Thompson estimates the mixing ratio and the particle number for cloud ice and rain categories, and WDM6 does the same though for cloud water, rain and the cloud condensation nuclei number. This means that for each of these categories, there are two associated predicted variables, which results in larger variability and a more realistic representation of particle size distribution.
Three ABL parameterisations were analysed in this work. The Mellor–Yamada–Janjic scheme (MYJ; Janjic 1994) considers only local mixing by using a 1.5-order (2.5-level) turbulence closure model based on the prediction of the turbulent kinetic energy (TKE). By contrast, the Yonsei University parameterisation (YSU; Hong et al. 2006) is a non-local scheme with a first-order closure that diagnoses the eddy viscosity coefficient as a function of the altitude, K(z), for each ABL stability condition. The third model used, the Asymmetric Convective Model v.2 (ACM2; Pleim 2007), considers local and non-local contributions in a similar first-order closure employing K(z) profiles, but with the addition of an eddy diffusion term. In previous works over the region, schemes with local and non-local mixing showed large differences in the representation of non-resolved turbulence (Ruiz et al. 2010; Alvarez Imaz et al. 2021; Suárez et al. 2022; Luque et al. 2024; Merino 2024). Therefore, including local and non-local mixing parameterisations of the ABL, like MYJ and YSU, in our analysis would provide greater sensitivity in the results.
Surface layer schemes are predefined by the choice of the ABL scheme in WRF. That is, each ABL parameterisation is compatible with only a few surface layer models. In this way, Janjic Eta (Janjic 2019) is compatible with the MYJ ABL scheme, whereas the fifth-generation Penn State–National Center for Atmospheric Research mesoscale model scheme (Revised MM5; Jimenez et al. 2012) fits with YSU and ACM2. Both schemes make use of the Monin–Obukhov similarity theory (Monin and Obukhov 1954) and their main differences are in the stability functions used to calculate heat, water and momentum exchanges between the atmospheric surface layer and the surface, and between the surface layer and the residual or mixing layers. Moreover, Janjic Eta includes parameterisations of the laminar sublayer above land and water, which are not included in MM5.
2.2. Validation data
Conventional weather stations from the SMN and automatic weather stations from the Instituto Nacional de Tecnología Agropecuaria (INTA) inside the MPRArg were used to validate WRF-simulated T2, incoming shortwave radiation at the surface (SWDOWN) and precipitation (PP; Fig. 1). Among all available stations within the model domain, a subset was selected seeking a more homogeneous spatial distribution (i.e. reducing the station density in highly populated areas). The characteristics and locations of the surface weather stations used in the validation of the simulations are presented in the Supplementary material (Supplementary Tables S1–S3).
The selected dates allowed us to validate the results with measurements from RELAMPAGO-CACTI (Nesbitt et al. 2021; Varble et al. 2021) and Mar Chiquita (Bautista et al. 2023) micrometeorological field campaigns. The performance of the WRF simulations in representing ABL structure was validated using atmospheric soundings from the RELAMPAGO-CACTI campaign. The dataset consisted of 5, 5 and 13 sounding profiles respectively launched during the sunny, cloudy and rainy cases at Villa María del Río Seco, Córdoba, Ezeiza and Santa Rosa (Fig. 1). All radiosonde launching times were matched to the closest WRF output hour.
WRF-simulated SWDOWN was validated using data from the INTA automatic surface weather station network. This dataset was complemented with extraordinary observations from the flux station measurements from RELAMPAGO-CACTI (Nesbitt et al. 2021; National Center for Atmospheric Research Earth Observing Laboratory In situ Sensing Facility and Oncley 2021; Varble et al. 2021) and from the Mar Chiquita flux station (Bautista et al. 2023). It is important to highlight that, even with the extended database, the stations measuring radiation were limited to the centre and south-east regions of the inner domain; thus, SWDOWN validation was restricted to that area.
In addition to in situ measurements, hourly outputs from ERA5 were used to compare T2, SWDOWN, PP and ABL structure with the WRF simulations and with the surface and sounding measurements.
2.3 Processing and validation
The model was evaluated using the bias, Root Mean Squared Error (RMSE) and Pearson correlation index (ρ), which are defined as follows:
where Xmodi and Xmeasi are the respective modelled and observed variables, and N is the number of available observations.
The indices from Eqn 1–3 were used to quantify and characterise the uncertainties of the model. In particular, non-zero biases are systematic errors that lead to over- and under-estimations by the model. However, this index can be zero even when there are large discrepancies between the model and the observations, as long as the mean is accurately estimated. In those cases, the RMSE comes in handy because large errors result in a high RMSE. Nevertheless, neither bias nor RMSE gives insights into the diel cycle of the variables. This aspect can be elucidated by examining ρ. A ρ close to 1 or −1 signifies that the two variables are respectively nearly in phase or counterphase. Conversely, a ρ value close to zero suggests that they share neither a phase nor a counterphase relationship.
The observation data were interpolated in time to the WRF output time using a nearest neighbour approach. This applies to INTA, RELAMPAGO-CACTI and Mar Chiquita datasets, whose sampling frequency is of the order of a few minutes. The nearest-neighbour approach was used to interpolate WRF model data to the location of the weather stations. In this way, the grid point closest to the observation location was selected in the WRF and ERA5 domains. The only exception was WRF PP, where the four grid points closest to the station’s location were averaged to smooth out the small-scale spatial variability from the model output. However, radiosonde data were compared with simulated data at the ERA5 vertical levels. WRF and radiosonde data were interpolated to ERA5 vertical levels using the same nearest-neighbour approach that was used to interpolate the horizontal grid.
2.4. CO2 flux uncertainties
We calculated uncertainties in the CO2 flux estimations for two Reco models and one GPP model: the Reco and GPP models developed by Mahadevan et al. (2008) and already implemented in VPRM-WRF (RecoM and GPPM), and a modified version of RecoM model developed by Gourdji et al. (2022) (RecoG). They are represented by Eqn 4–7:
In Eqn 4–7, where T is the near-surface temperature (in this study, we use T2), β, α, λ, PAR0, Tmin, Tmax and Topt are parameters that represent the baseline ecosystem respiration, the temperature sensitivity of ecosystem respiration, the maximum quantum yield, the half-saturation value of photosynthetic active radiation, and the minimum, maximum and optimal temperatures for photosynthesis respectively, whose value is defined in the VPRM-Europe table (Ahmadov et al. 2009). All these parameters depend on the type of vegetation. However, β1, α1, α2, γ, k1, k2 and k3 are parameters that quantify the influence of each term on Reco whose values are taken from Gourdji et al. (2022); EVI, Pscale, Wscale and Tscale are parameters ranging from 0 to 1 respectively representing greenness, leaf age, water stress and influence of T2 on vegetation photosynthesis. Absolute errors for all the models can be calculated after expanding Eqn 4–7 by the Gaussian Error Propagation method, resulting in the following error expressions:
Eqn 8–11 represent the absolute errors of RecoM due to T2 (Eqn 8), GPPM due to SWDOWN (Eqn 9), Tscale due to T2 (Eqn 10), and RecoG due to T2 (Eqn 11). Amin, Aopt and Amax are respectively T − Tmin, T − Topt and T − Tmax. We used the T2 RMSE obtained during the validation as dT and the SWDOWN RMSE as dSW. Then, we calculated relative errors for the main vegetation types over the inner domain, which are crops, grass and shrub. We fixed the parameters EVI, Pscale, Wscale and Tscale to 1 when calculating the relative errors of the RecoG model to get its upper bound. Regarding relative GPPM errors due to T2, the parameters EVI, Pscale and Wscale were not relevant because they cancelled each other out, which made their results equal to relative errors in Tscale.
3.Results
3.1. Overall performance of the simulations
As shown in Fig. 3, the simulation for the sunny case shows almost no quantity of condensates (QC) and no precipitation. The simulation for the cloudy case shows higher condensate contents but almost no precipitation, and the simulation for the rainy case shows substantially higher condensate concentrations as well as higher precipitation values. YSU and ACM2 had the lowest QC during the cloudy and rainy cases, although the lowest rainy case PP occurred in WDM6 and Thompson. However, in the cloudy and rainy cases, ERA5 had QC higher than the WRF simulations that used WSM6 as their microphysics scheme, but lower than WDM6 and Thompson. Regardless, the resulting ERA5 precipitation in the rainy case was the highest.
(a)Domain and temporally averaged QC, and (b) domain-averaged PP for each case and model configuration. Solid lines are Control scenario values for reference.
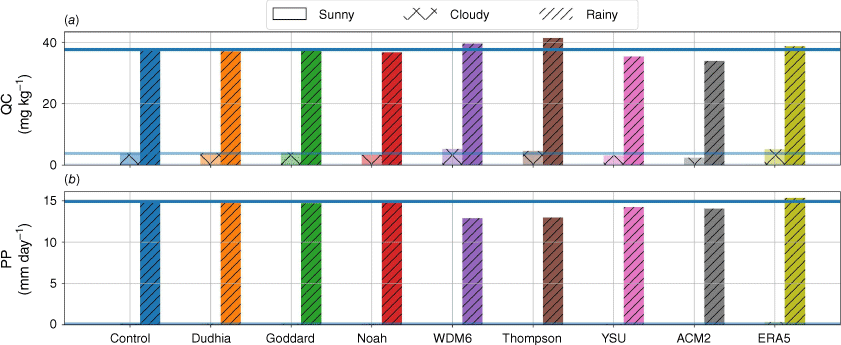
Front areas denote strong gradients of equivalent potential temperature (θe) due to large temperature and humidity contrasts. In each simulation made during the rainy case, the θe = 330 K line, which approximately follows the location of the cold front in this case, had a similar shape (Fig. 4a). In particular, in YSU and ACM2 runs, the front was located ~0.5° to the south, suggesting a slower front displacement than in the other scenarios. In the case of ERA5, its θe = 330 K line was ~1° further to the south between 64 and 60°W and ~0.5° furhter to the north between 60 and 57°W compared with the simulations (Fig. 4b).
(a) θe (greyscale) at 925 hPa for the Control configuration during the rainy case at 15:00 hours UTC. The solid line represents the θe = 330 K contour. (b) θe = 330 K contour for each WRF model configuration and for ERA5 during the rainy case at 15:00 hours UTC.
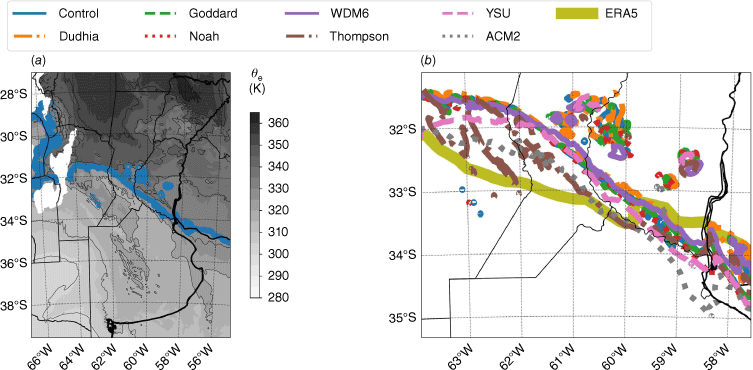
These results indicate that the WRF model adequately captured the main characteristics of these three events in terms of cloud formation and precipitation. The comparison also shows that differences among WRF simulations are smaller than the difference between WRF and ERA5. This is expected behaviour because ERA5 data are based on a global model with lower effective resolution in which processes such as deep convective clouds are parametrised.
3.2. Impact of different parametrisation schemes on VPRM input variables
In this subsection, we present the validation results for T2. We averaged the metrics from Eqn 1–3 over all the stations for each run. All configurations had ρ greater than 0.87, RMSE lower than 2.5°C and negative bias, except for YSU and ACM2, which had positive biases during the cloudy (both) and rainy (ACM2) cases (Fig. 5). ACM2 had the best performance of the WRF configurations during the sunny case. Dudhia and WDM6 had the lowest RMSE in the cloudy case, and ACM2 outperformed all the other configurations during the rainy case, even though the smallest bias was in YSU. However, ERA5 was the best model in the sunny and rainy cases, although it was slightly worse than Dudhia in the cloudy case.
RMSE vs bias in degrees Celsius for T2 and the mean ρ calculated over the hourly T2 time series for each run during the (a) sunny, (b) cloudy and (c) rainy cases. Solid lines represent the RMSE and bias from the Control case, the dashed line represents bias = 0°C and the symbol size is the mean ρ.
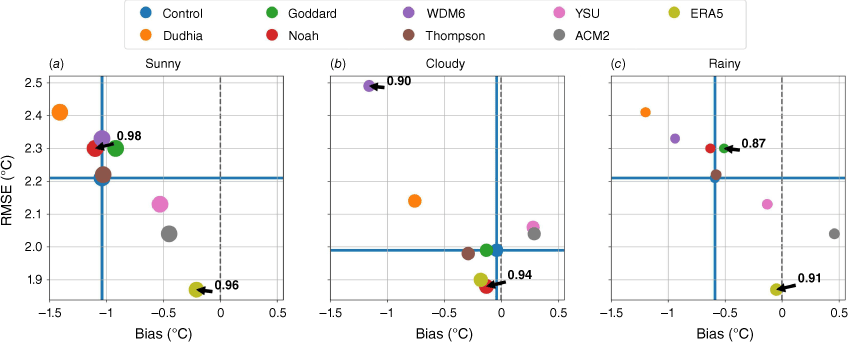
A full description of the spatial and temporal distribution of these errors is presented in the Supplementary material (Supplementary Fig. S1–S4). One advantage of high-resolution models is in the representation processes associated with complex terrain. To investigate if this has a particular impact on our simulations, Fig. 6 shows the performance of WRF simulations at stations located over 200 m in altitude and below that value. The 200-m threshold was reached after binning the stations by height into five categories, with 20% in each group. All the Student’s t-tests had P values higher than 0.15 except for WDM6 in the rainy case and for ERA5 in all cases (Fig. 6). This implies that ERA5 estimated T2 for stations located above 200 m worse than for those situated below 200 m, whereas all the WRF configurations but WDM6 during the rainy case performed similarly regardless of this threshold. Therefore, complex topography added uncertainty to ERA5 estimates that did not reduce the performance of the WRF configurations. The complete statistical results are presented in the Supplementary material (Supplementary Table S4).
Boxplots calculated over the hourly T2 time series for each run during the (a) sunny, (b) cloudy and (c) rainy cases in stations with altitude below or above 200 m. The boxes and whiskers indicate the 25th–75th and the 5th–95th percentiles respectively. White rectangles and triangles are the medians and means respectively. Horizontal lines represent the means from the Control scenario. Single and double asterisks indicate P-values from the mean t-test smaller than 0.15 or 0.01 respectively.
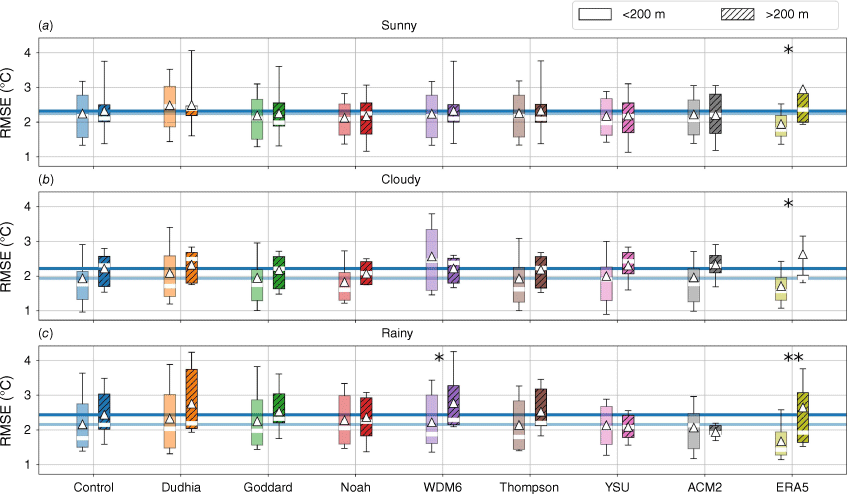
During the rainy case, the precipitation systems are associated with enhanced mesoscale variability in surface variables such as T2. This enhanced variability at smaller spatio-temporal scales is also linked with increased uncertainty in the simulations, which can result in higher uncertainty in the simulated CO2 fluxes. One feature frequently connected with mesoscale convective systems is the cold pool. All simulations in the rainy case show a large and intense cold pool over the north-eastern part of the domain at 15:00 hours UTC (Fig. 7). This cold pool is well ahead of the lower-temperature region that is observed in the south-western part of the domain and that is associated with the cold front.
T2 for each model configuration (a–h) and for ERA5 (i) during the rainy case at 15:00 hours UTC. The WRF model configurations were (a) Control, (b) Dudhia, (c) Goddard, (d) Noah, (e) WDM6, (f) Thompson, (g) YSU and (h) ACM2.
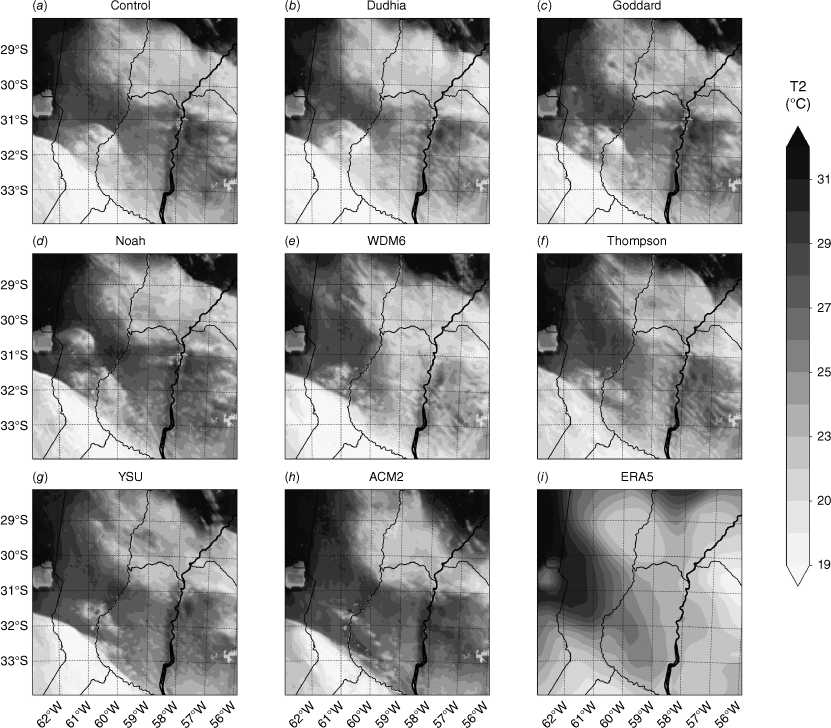
The cold pool varied its intensity depending on the radiation scheme used, but had the same location in all of them (Fig. 7a–c). However, in Thompson and WDM6, the cold pool extended to the south, merging with the colder air behind the cold front (Fig. 7e–f). The distance between the cold pool and the front was longest in ACM2 (Fig. 7h). Its position was very similar among the runs, where the most important differences were in Noah and YSU as it was located slightly to the north and south in these scenarios respectively (Fig 7d, g). ERA5 had the cold pool with the lowest intensity and split into two smaller pools in the west–east direction (Fig. 7i). This behaviour is expected considering that deep moist convection is not explicitly accounted for in ERA5 so that cold pool generation and maintenance processes are only partially represented.
In this subsection, we present the validation results for the 24-h integrated SWDOWN and for its diel cycle. Fig. 8 shows the RMSE and bias for the daily-averaged SWDOWN and the correlation coefficient computed from the hourly SWDOWN values. We averaged their values over all the stations for each run. The differences among the simulations were dominated by the radiation scheme in the sunny case, but the other parameterisations were also important in the other two cases. Error metrics were highest during the rainy case and smallest during the sunny case, whereas ρ had the opposite behaviour (Fig. 8). The only exception was WDM6 with a higher RMSE and smaller ρ in the cloudy case; this is consistent with the relatively worse performance of this configuration for T2 in this same case. In the sunny and rainy cases, Dudhia outperformed all the WRF simulations, but YSU had the best RMSE during the cloudy case. It is interesting to note that although Dudhia performs best in the sunny case in terms of shortwave radiation, it produces the worst results in the same case in terms of T2. This indicates that producing the right radiative forcing may not lead to the optimal results in terms of temperature, probably owing to model errors in terms of other processes such as turbulent mixing within the ABL or land surface processes.
RMSE vs bias for SWDOWN (MJ m−2 day−1) calculated over the daily time series, and the mean ρ calculated over the hourly time series for each different WRF configurations and for the ERA5 during the (a) sunny, (b) cloudy and (c) rainy cases. Solid lines represent the RMSE and bias from the Control case, the dashed line indicates when the bias = 0 MJ m−2 day−1, and the circle size is the mean ρ.
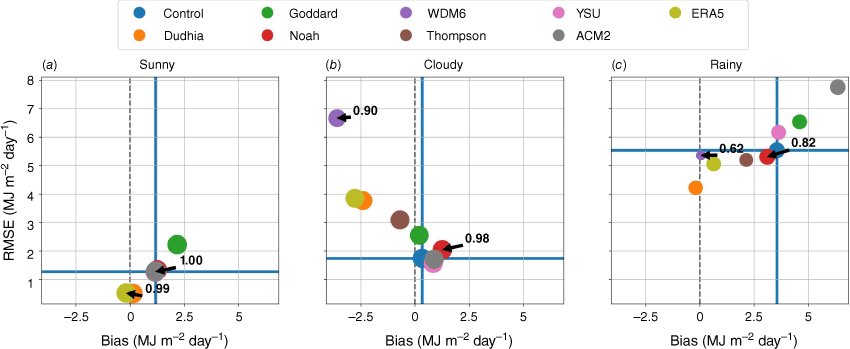
All the models simulated SWDOWN with similar biases during the sunny case, but there were large differences between the models in the cloudy and rainy cases. For instance, for the station with the largest differences across the configurations, located at −31.85°S, 60.54°W, its bias respectively ranged from −20.6 to 1.9 MJ m−2 day−1 and from −4.0 to 12.2 MJ m−2 day−1 in the cloudy and rainy cases. The SWDOWN diel cycle was similar to measured radiation in the sunny case, but the differences in its patterns were larger during the other two cases. A detailed presentation of the figures that illustrate these examples is given in the Supplementary material (Supplementary Fig. S5–S8).
The simulated PP was validated by applying Eqns 1, 2 to 24-h accumulated precipitation. As this variable has a higher small-scale spatial variability than T2 and SWDOWN, WRF-simulated PP was interpolated to the station location using the average of the closest four grid points to each station.
In the sunny and cloudy cases, PP errors were below 0.5 mm day−1; thus, the analysis was focused on the rainy case. In this case, all the simulations had a positive bias except WDM6, Thompson and ACM2, which respectively had negative, negative and zero biases (Fig. 9). However, the RMSE of PP was below 12.7 mm for all the simulations, which is of the same order of magnitude as the average of 12.2 mm measured across all the stations. The lowest RMSE of the WRF simulations was achieved in Goddard, with a value of 7.5 mm day−1. WDM6 and Thompson produced the largest difference in terms of RMSE with respect to the Control run. This is expected because the cloud microphysics parameterisation is the most closely related to rain. However, other schemes such as Noah (land surface processes) and Dudhia (radiation processes) produce similar impacts in terms of RMSE. This behaviour may be related to the complex dynamics associated with precipitating systems, which can be significantly affected by the ABL structure, which in turn depends on surface fluxes and radiative forcing. Moreover, the chaotic behaviour of the atmosphere is exacerbated in these situations owing to the existence of rapid instabilities that accelerate error growth.
Bias and RMSE (mm day−1) during the rainy case. Solid lines are the Control scenario values for reference.
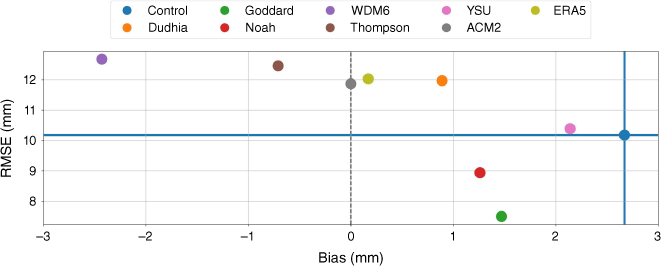
Regarding the spatial distribution of the errors, all the WRF simulations had a positive bias at most stations but a negative bias over the ones located near the north-east corner of the domain, where a pre-frontal mesoscale convective system took place. The largest differences among the WRF simulations were observed in this region. The spatial distribution of PP errors is presented in the Supplementary material (Supplementary Fig. S9–S10).
To validate how WRF simulations represent the ABL structure, we applied Eqn 1 and 2 to compare model outputs and observations vertically interpolated to a common set of 10 vertical levels between 975 and 750 hPa, evenly spaced 25 hPa apart. Error metrics were then averaged over all the available soundings. The resulting potential temperature RMSE and bias within the ABL were respectively below 1.3, 1.2 and 2.8°C during the sunny, cloudy and rainy cases. ACM2 had the best metrics among the WRF simulations, but ERA5 outperformed all of them. The only exceptions were the cloudy case bias where YSU was slightly better than ACM2 and the rainy case RMSE where ACM2 was better than ERA5.
Fig. 10 shows the vertical profiles of RMSE and bias averaged over all available soundings. Potential temperature bias and RMSE below 900 hPa were respectively between −3.5 and 3.0°C, and below 6.0°C. They were larger below 950 hPa, except in the rainy case, where the highest errors were between 950 and 850 hPa. The three cases had cold biases for all the WRF simulations and vertical levels, except for YSU and ACM2, which were hotter near the surface. These results are consistent with the warm T2 bias presented earlier for these two configurations. The WRF simulations had the worst performance in the rainy case, but ERA5 had the worst score in the sunny case. However, dew point temperatures showed the opposite behaviour. They were better below 950 hPa than in the upper layers, and they had the lowest errors in the rainy case. Readers interested in the individual soundings are referred to the Supplementary material (Supplementary Fig. S11–S13).
3.3. Impact of VPRM input variable uncertainties on the CO2 flux simulation
We propagated the T2 and SWDOWN RMSEs estimated in the previous sections into the Reco and GPP models to estimate their impact on CO2 flux simulation. In the case of the daily accumulated SWDOWN, we divided its RMSE by 13.5 h to convert it to the appropriate units required by WRF-VPRM (W m−2 s−1). We chose 13.5 h because it is close to the number of daytime hours for the domains and cases simulated. In the same way, we analysed the relative errors due to T2 and SWDOWN within the relevant ranges of these variables for the domain and cases simulated, which were ranged from 8 to 38°C and from 50 to 2500 W m−2. The following subsections illustrate RecoM, RecoG and GPPM errors through Fig. 11 and 12.
Relative errors estimated for GPPM and for the linear and polynomial Reco models due to errors in T2 and as a function of T2 for each run. Solid lines are the medians of the configurations and the hatched areas are bounded by their minimum and maximum errors. The upper (a, b, c), middle (d, e, f) and lower rows (g, h, i) correspond to the sunny, cloudy and rainy cases respectively. The left (a, d, g), middle (b, e, h) and right columns (c, f, i) correspond to the crop, grass and shrub vegetation types respectively.
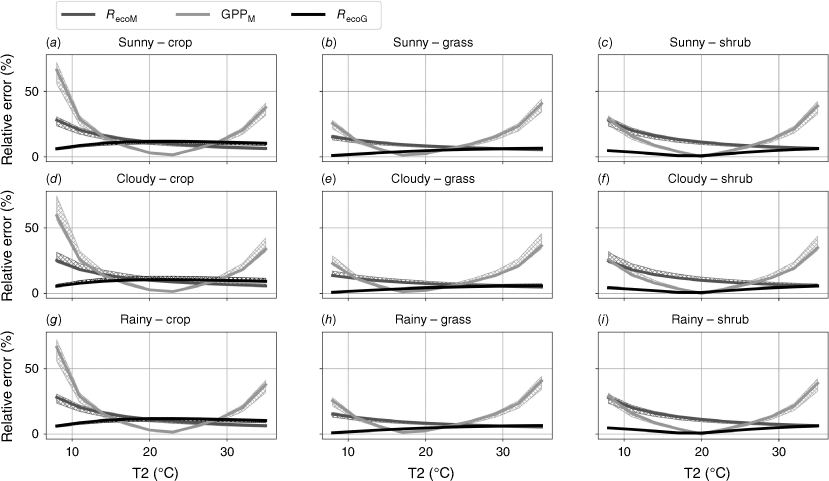
As in Fig. 11, but for the relative errors in GPPM due to the error in SWDOWN and as a function of SWDOWN. The upper (a, b, c), middle (d, e, f) and lower rows (g, h, i) correspond to the sunny, cloudy, and rainy cases respectively. The left (a, d, g), middle (b, e, h) and right columns (c, f, i) correspond to the crop, grass and shrub vegetation types respectively.
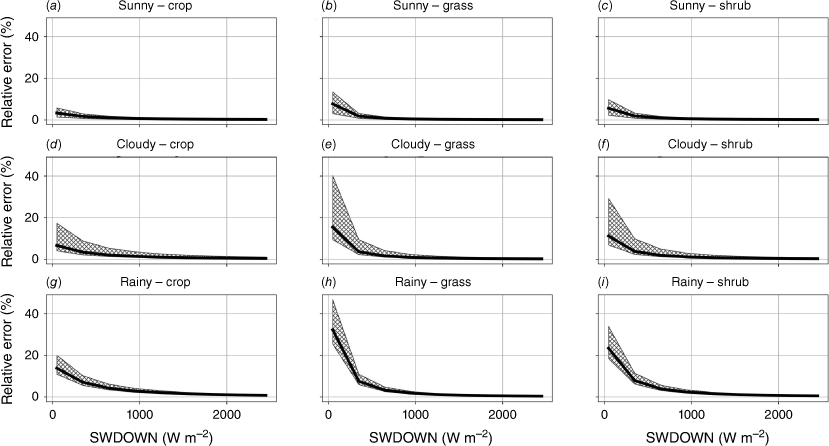
The impact of T2 on Reco estimation is illustrated in Fig. 11. Relative RecoM errors due to T2 were larger with lower temperatures because the fluxes approach zero when T2 is close to the critical temperature of the vegetation. By contrast, RecoG errors were smaller at colder temperatures because the choice of Wscale = 1 resulted in larger – and non-zero – fluxes at 0°C. Shrub land use type and the rainy case errors were the highest, whereas grass land use type and the cloudy case produced the lowest errors. All the runs had similar errors, with the highest differences occurring when T2 was below 15°C.
Temperature affects CO2 uptake by vegetation when estimated by the GPPM model. Relative errors in GPPM due to T2, which are equivalent to relative errors in Tscale due to T2, were lowest when T2 was close to Topt and highest when it approached the parameters Tmin or Tmax (Fig. 11). This is expected behaviour because GPP is highest at the optimal temperature for photosynthesis, Topt, and zero when T2 is close to the minimum and maximum temperatures for photosynthesis, Tmin and Tmax. When looking at the different cases, the sunny and rainy cases had similar errors, being smaller in the cloudy case, except for WDM6, which had the highest in that case. In a similar way, the difference between the vegetation types was small at higher temperatures. Errors for grass and shrub were close to each other, but errors for crops were larger at colder temperatures. As with Reco models, all runs had similar GPPM errors because their T2 RMSEs were within a narrow range.
The impact of SWDOWN on GPP estimation is illustrated in Fig. 12. GPPM relative errors due to SWDOWN were larger when this variable approached zero because photosynthesis ceases. This was most evident in the rainy case, where relative errors increased faster. In these situations, Dudhia had the lowest errors in the sunny and rainy cases, and YSU in the cloudy case. However, relative errors diminished rapidly with increasing SWDOWN values in every case. When looking at the vegetation types or at different runs, their differences were small because their SWDOWN RMSEs were within a narrow range. The highest differences among the vegetation types were between crop and shrub, which had the lowest and highest relative errors, espectively.
4.Discussion
4.1. Sensitivity of the most relevant variables involved in the CO2 flux simulation to the choice of model configuration
Hourly T2 errors from the WRF simulations made in this study were smaller than 2.5°C in all cases (Fig. 7). On average, ERA5 performed better than WRF with errors below 1.9°C, but its performance was worse than WRF for stations with complex topography. Over the MPRArg, Ruiz et al. (2010) tested different schemes in lower-resolution forecasts (40 km) and obtained a spatially averaged RMSE between 2.4 and 3.1°C in the hourly T2 prediction for all schemes evaluated. Another study from the same region, Müller et al. (2016), evaluated 15-km resolution WRF forecasts for a 2-year period between 2012 and 2014. They reported absolute mean errors of daily mean temperatures up to 2.7°C. In the same way, Dillon et al. (2021) validated 10-km resolution WRF forecasts with different microphysics and ABL schemes during 44 days from 2018 over the MPRArg. Their averaged RMSE and bias over the 36-h forecasts were respectively below 3.0 and 1.0°C. In summary, other studies from the region had errors larger than in our study. Given that other sensitivity analyses conducted in this region and documented in the scientific literature exhibit lower resolution, a comparison will be drawn with simulations from different regions such as the United States, China and Greece (Giannaros et al. 2013; Wyszogrodzki et al. 2013; Ju et al. 2022). These authors performed simulations with respective horizontal grid spacings of 4, 3 and 2 km, and the maximum reported error in T2 was 3.1°C.
Daily integrated SWDOWN errors were respectively smaller than 2.2, 6.7 and 7.8 MJ m−2 day−1 during sunny, cloudy and rainy situations (Fig. 8). Over the southern portion of the domain, monthly mean SWDOWN ranges between 8.0 MJ m−2 day−1 in winter and 30.0 MJ m−2 day−1 in summer, and has been increasing over the past decades (Fernández et al. 2021). Numerical estimations for this variable over the region are scarce and involve models different from WRF. Among them, Pessacg et al. (2014) validated several regional models with a grid spacing of 50 km using satellite data. They found biases up to 1.7 MJ m−2 day−1 for the period 1990–2008. In other regions, there are numerical studies focusing on cloudy cases. For instance, 1.3-km resolution simulations of the AROME model over France during 2020 produced a maximum RMSE of 4.8 MJ m−2 day−1 in cases where there were clouds in the model and in the observations (Magnaldo et al. 2023). As another example, 4- and 1.5-km resolution runs of the Met Office Unified Model (MetUM) during 2017–18 over South Africa had respective errors of 4.6 and 5.2 MJ m−2 day−1 (Mendes et al. 2023). SWDOWN estimations by WRF have been validated in regions different from the MPRArg. Examples of these studies involving high-resolution WRF simulations include a 5-km resolution experiment over the Iberian Peninsula during the period 2000–2009. It produced daily SWDOWN RMSEs up to 3.1 MJ m−2 day−1 (Perdigão et al. 2017). Also, a validation of 3-km resolution simulations over north-eastern Germany in 87 frontal passages resulted in a maximum RMSE of 9.3 MJ m−2 day−1 (Mierzwiak et al. 2023). In all the numerical experiments mentioned, we assumed an average of 12 daytime hours to extrapolate their results from watts per square metre (W m−2) to megajoules per square metre per day (MJ m−2 day−1). In consequence, from the information stated before, SWDOWN errors from the present study share the same order of magnitude as other simulations and expand previous works by providing high-resolution WRF simulations over the region.
Accumulated PP forecasts inside the MPRArg have been studied using WRF in Ruiz et al. (2010), Müller et al. (2016), Casaretto et al. (2022) and Dillon et al. (2021). In our rainy case, PP averaged 12.2 mm across all stations, and its bias and RMSE were respectively bounded between −2.5 and 2.7 mm day−1 and between 7.5 and 12.7 mm day−1 (Fig. 9). This range is smaller than the bias range found in Ruiz et al. (2010), which was between −10.0 and 15.0 mm day−1 in the MPRArg during summer (December, January and February) with a 40-km resolution model, and higher than the 5.0 mm day−1 from Müller et al. (2016) with a 15-km resolution model in a 2-year period between 2012 and 2014. In the same way, using 4- and 3-km resolution models, Casaretto et al. (2022) obtained PP biases between −5.0 and 5.0 mm day−1 during 43 days of November–December in a smaller region with complex terrain inside the MPRArg, with an average of 3.3 mm day−1. However, Dillon et al. (2021) compared 10-km resolution WRF forecasts with PP satellite estimates over 44 days inside the MPRArg during 2018. They found the average bias of precipitation events to be below 3.0 mm grid−1. Even though PP is particularly sensitive to the method of validation chosen, like using nearest-neighbour interpolation with one grid point or averaging a few grid points surrounding a station, daily PP from our simulations is in agreement with other assessments from the same region.
Temperature biases and RMSEs within the ABL were respectively between −3.5 and 3.0°C, and below 6.0°C (Fig. 10). Over the region, the ABL structure has been analysed in Ruiz et al. (2010) for YSU and MYJ schemes using a 40-km resolution model and soundings from Resistencia and Santiago del Estero stations (27.45°S, 59.05°W; 27.77°S, 64.30°W). In Resistencia, the biases were bounded by 2.0°C and they were cold near the surface and warm at higher levels. However, the bias at Santiago del Estero was cold, reaching up to 3.0°C within the ABL, with YSU errors being warmer at lower heights, as happened in our work. The ABL structure showed greater sensitivity to the choice of both ABL and surface layer schemes in the rainy case. Therefore, warmer, wetter and more unstable ABLs in YSU and ACM2 were generated. The ABL structure has been studied with higher-resolution models in other regions of the world. Nevertheless, our RMSE values were higher than those calculated by Coniglio et al. (2013) where the RMSE for the ABL potential temperature was below 1.0°C for a 4-km resolution model over the United States. Similarly, their ABL structure sensitivity was smaller than in our case. In their experiments, the errors were almost constant above 100 m, which is similar behaviour to that found for the sunny case in the present study. This is an expected result because Coniglio et al. (2013) considered only the soundings obtained under clear-sky conditions. Our study expands their analysis by showing that potential temperature errors can show a different vertical structure within the ABL under synoptically active conditions.
Each target variable was more sensitive to a particular physical process depending on the synoptic situation. For instance, the ABL–surface layer and radiation schemes analysed had the largest T2 and SWDOWN bias spread in most cases. This result highlights the importance of choosing appropriate ABL–surface layer and radiation schemes when analysing CO2 fluxes in simulations. Selecting ill-performing parameterisations for these processes can increase the CO2 flux errors. For that reason, it is important for future studies to carefully consider their selected schemes. Specifically, based on our results, we recommend including a set of ABL parameterisations with local and non-local mixing schemes. Future research can enhance the robustness of this analysis by incorporating additional case studies, extending time periods and using independent validation data. It would also be beneficial to evaluate the performance of similar analyses using different combinations of schemes than those selected here. Attention should be paid to the ABL–surface layer combination, as Maroneze et al. (2021) demonstrated that they significantly affect the simulation of the stable boundary layer.
4.2. Uncertainties of CO2 flux simulations
In this study, we investigated the impact of temperature and SWDOWN errors on CO2 fluxes. The Reco and GPP models from the original VPRM – RecoM and GPPM – had larger relative errors when either T2 or SWDOWN approached zero, which are common daytime conditions in the anticyclonic flow behind a cold front. The former was due to Reco and GPP being smaller at colder temperatures when the vegetation is close to its Tmin. In a similar way, as plants decrease their photosynthesis rate when SWDOWN is scarce, GPPM relative errors are larger at sunrise or sunset. As a consequence, when both effects are considered, the highest relative errors are expected during sunrise and just behind the front zone, where temperature and incoming radiation are low. This outcome can be mitigated if a respiration model with low uncertainties at cold temperatures is used, like RecoG, instead of RecoM.
Li et al. (2020) performed a sensitivity analysis of the original VPRM to its parameters. They found the highest NEE uncertainties to be up to 60%, occurring near the local solar noon when SWDOWN was highest. Our study complements their results by pointing out that uncertainties in the environmental variables simulated by a numerical model increase the NEE relative errors during the morning and evening, accounting for ~20% of the cases assessed in their work (Fig. 11 and 12). Furthermore, at least over the MPRArg, the CO2 flux uncertainty will be larger in areas with complex topography during rainy cases because T2 and SWDOWN errors were larger. Therefore, when both sources of uncertainty are taken into account, daytime relative errors may be greater than 60%.
Even though the simulations from our study were similar, slight differences will change the fluxes. For instance, as the nocturnal cooling rate in ERA5 was slower than in the WRF simulations in most locations, nighttime Reco fluxes calculated with ERA5 will be higher, leading to a more positive CO2 daily budget. By contrast, the nighttime carbon balance will be more negative in simulations that use Noah as the land surface scheme because its nocturnal cooling rate was faster.
Regarding the effect of precipitation on near-surface temperatures, there were runs with larger cold pools, like Thompson and WDM6, that result in smaller Reco fluxes. Moreover, the position of the cold pool may also influence Reco in the presence of high spatial variability in the vegetation type. For example, the cold pool simulated in the Control setting covered a smaller percentage of grass than in YSU because it was slightly shifted to the west. Therefore, differences in the location of the cold pools result in uncertainties in the NEE fluxes. Mislocation of cold pools is not the objective of our study and should be studied in depth in future work.
It is important to highlight that most of the runs that used MYJ as the ABL scheme underestimated T2 and overestimated SWDOWN. This will result in Reco and GPP fluxes smaller and larger than the real ones respectively, and the combined effect over NEE will depend on the specific values of both variables. This suggests that ABL schemes with non-local mixing, like YSU or ACM2, are more appropriate for the region. Even though these schemes had positive T2 biases during the cloudy and rainy cases, they would result in lower Reco and GPP errors.
The CO2 flux uncertainty depended on the dominant synoptic situation of the case. In this way, GPP errors in cloudy weather were slightly larger than in fair (sunny) weather but much smaller than in rainy conditions. This implies that the uncertainty added by the front and the mesoscale convective system was more important than that produced by clouds alone. In consequence, future GPP estimates should consider each particular synoptic situation separately and then adjust its uncertainty level accordingly.
The Intergovernmental Panel on Climate Change (IPCC) includes CO2 atmospheric concentration estimates for the near, mid and long-term futures based on global climate models able to account for CO2 emissions from biogenic sources (Canadell et al. 2021; Friedlingstein et al. 2022). These models utilise flux inventories as prior information through a Bayesian approach. The priors can be based on observations, simulations from models like VPRM or a combination of both. As we have shown here, the uncertainty in the CO2 fluxes can be very high and may depend on the synoptic situation. This fact added to other sources of error not analysed in this study, like model selection, parameters and resolution, contribute to the total uncertainty. Therefore, our results strongly suggest a more extensive investigation of the uncertainties associated with the representation of physical processes in the global and regional climate models, in particular those that are directly associated with the variables that modulate surface CO2 emissions from biogenic sources. We recommend that future studies employ global and/or regional climate models capable of accounting for CO2 emissions from biogenic sources to conduct sensitivity analyses similar to ours, while carefully considering all sources of error, particularly the uncertainty associated with the parametrisation of unresolved scale processes in the CO2 flux.
4.3. Most appropriate WRF schemes for simulating CO2 fluxes on each dominant synoptic situation
The performance of the WRF parameterisations tested in this study characterised the uncertainty associated with the selection of different parameterisations on atmospheric variables, essential for computing surface CO2 fluxes over the MPRArg. Overall, all performed better than ERA5 in stations surrounded by complex topography. Among the radiation schemes, RRTMG_SW and Goddard outperformed Dudhia in the estimation of near-surface temperatures, but the latter produced more accurate estimates of shortwave radiation fluxes during the three synoptic situations simulated. The Noah MP land surface model produced better simulated near-surface temperatures and shortwave radiative fluxes than Noah. Also, Thompson microphysics parameterisations were better than WDM6 in the simulation of near-surface temperature. WSM6 and WDM6 were the best microphysics schemes to simulate shortwave radiation fluxes during the cloudy and rainy cases respectively. Non-local ABL schemes, YSU and ACM2, surpassed MYJ when simulating near-surface temperatures in the sunny and rainy cases. The opposite occurred in the cloudy case and with shortwave radiation fluxes in all situations. All in all, even when the sensitivity to a change in the parameterisation chosen was very small in many cases, it was possible to provide insights about an appropriate set of WRF schemes for each particular synoptic situation that can be used in future study cases.
Overall, relative NEE errors due to T2 were similar in all the configurations and cases tested, with the exception of WDM6 in the cloudy case, which propagated larger errors. When considering relative NEE errors due to SWDOWN, Dudhia outperformed the other configurations in the sunny and rainy cases, whereas the same happened for ACM2 in the cloudy case. Relative errors were smaller when T2 was close to Topt and when SWDOWN was high; thus, all the configurations tested in this analysis will have a similar performance in study cases with these atmospheric conditions, like, for example, temperate and sunny cases. However, the spread of relative errors due to T2 and SWDOWN was larger when T2 was close to Tmax or Tmin and when SWDOWN was low respectively. These conditions happen at sunrise and sunset and just behind the front zone. Therefore, it is possible to get smaller relative NEE errors in study cases involving frontal passages when choosing Dudhia or ACM2 over the other schemes tested.
5.Conclusions
This study expanded previous results about high-resolution WRF simulations and CO2 flux uncertainties. In particular, we implemented a high-resolution model in the most productive region of Argentina, an area with high frontal activity and high CO2 fluxes from agriculture. We added a new description of the errors in the simulated near-surface temperatures, shortwave radiation fluxes, precipitation and atmospheric boundary layer structure over the region. All the error ranges were in agreement with previous work done in similar conditions, i.e. the same region, dominant synoptic situation, or model configuration. Although fair weather conditions are expected to be associated with low uncertainties, cloudy conditions introduce challenges in the computation of shortwave radiation at the surface, involving complex interactions between the microphysics and the radiation schemes, whereas rainy conditions introduce complex feedbacks between precipitating systems, radiation and surface temperature. The CO2 flux relative uncertainties due to errors in the near-surface temperature and shortwave radiation fluxes from this work were larger at sunrise and sunset and just behind the front zone, adding to previous studies that linked other sources of errors with a high CO2 flux uncertainty during the afternoon. It is important to highlight that the effect on CO2 flux will depend on the flux model chosen and on its specific coefficients, which in turn rely on vegetation type. This implies that other systematic errors, like cold pool mislocation, will propagate to the flux, a topic that should be studied in depth in future work. Lastly, this study provided insights on the choice of appropriate combinations of WRF schemes to estimate CO2 fluxes. Therefore, the results presented here allow a better quantification of the uncertainty of WRF simulations and CO2 flux estimates in different weather conditions, both of which are key to improve the reliability of GHG budgets and to understand their role in the past and future effects of climate change.
Data availability
The data that support this study will be shared on reasonable request to the corresponding author.
Declaration of funding
María Isabel Gassmann received support for this work from Consejo Nacional de Investigaciones Científicas y Técnicas (CONICET) (grant number PIP11220200100794CO) and the Universidad de Buenos Aires (UBA) (grant number UBACYT20020190100128BA).
Acknowledgements
We acknowledge the grant funding received from CONICET and UBA. We are grateful to María José Denegri who helped us to expand the radiation database with the data provided by the Grupo de Estudios de la Radiación Solar (GERSolar) del Instituto de Ecología y Desarrollo Sustentable (INEDES UNLu–CONICET) and to the Servicio Meteorológico Nacional for sending us their sounding and station data. Simulations were made with the high-performance computing clusters available at CIMA/UBA–CONICET, Argentina.
Author contributions
Nahuel Bautista contributed conceptualisation, formal analysis, methodology, investigation, data and image visualisation, and writing, review and editing of the original draft. Juan Ruiz provided conceptualisation, methodology, funding acquisition, supervision, and review and editing of the writing. Paola Salio added conceptualisation, funding acquisition, supervision, and review and editing of the writing. Lucas Burgos provided data curation and review and editing of the writing. María Isabel Gassmann added conceptualisation, methodology, funding acquisition, supervision, and review and editing of the writing.
References
Ahmadov R, Gerbig C, Kretschmer R, Körner S, Rödenbeck C, Bousquet P, Ramonet M (2009) Comparing high resolution WRF-VPRM simulations and two global CO2 transport models with coastal tower measurements of CO2. Biogeosciences 6(5), 807-817.
| Crossref | Google Scholar |
Alvarez Imaz M, Salio P, Dillon ME, Fita L (2021) The role of atmospheric forcings and WRF physical set-up on convective initiation over Córdoba, Argentina. Atmospheric Research 250, 105335.
| Crossref | Google Scholar |
Baldocchi DD (2020) How eddy covariance flux measurements have contributed to our understanding of Global Change Biology. Global Change Biology 26(1), 242-260.
| Crossref | Google Scholar | PubMed |
Baldocchi D, Chu H, Reichstein M (2018) Inter-annual variability of net and gross ecosystem carbon fluxes: a review. Agricultural and Forest Meteorology 249, 520-533.
| Crossref | Google Scholar |
Barros VR, Boninsegna JA, Camilloni IA, Chidiak M, Magrín GO, Rusticucci M (2015) Climate change in Argentina: trends, projections, impacts and adaptation. Wiley Interdisciplinary Reviews: Climate Change 6(2), 151-169.
| Crossref | Google Scholar |
Bautista NE, Gassmann MI, Pérez CF (2023) Gross primary production, ecosystem respiration, and net ecosystem production in a southeastern South American salt marsh. Estuaries and Coasts 46, 1923-1937.
| Crossref | Google Scholar |
Blázquez J, Solman SA (2019) Relationship between projected changes in precipitation and fronts in the austral winter of the Southern Hemisphere from a suite of CMIP5 models. Climate Dynamics 52, 5849-5860.
| Crossref | Google Scholar |
Canadell JG, Monteiro PMS, Costa MH, Cotrim da Cunha L, Cox PM, Eliseev AV, Henson S, Ishii M, Jaccard S, Koven C, Lohila A, Patra PK, Piao S, Rogelj J, Syampungani S, Zaehle S, Zickfeld K (2021) Global Carbon and other Biogeochemical Cycles and Feedbacks. In ‘Climate Change 2021: The Physical Science Basis. Contribution of Working Group I to the Sixth Assessment Report of the Intergovernmental Panel on Climate Change’. (Eds V Masson-Delmotte, P Zhai, A Pirani, SL Connors, C Péan, S Berger, N Caud, Y Chen, L Goldfarb, MI Gomis, M Huang, K Leitzell, E Lonnoy, JBR Matthews, TK Maycock, T Waterfield, O Yelekçi, R Yu, B Zhou) pp. 673–816. (Cambridge University Press: Cambridge, UK, and New York, NY, USA) 10.1017/9781009157896.007
Casaretto G, Dillon ME, Salio P, Skabar YG, Nesbitt SW, Schumacher RS, García CM, Catalini C (2022) High-resolution NWP forecast precipitation comparison over complex terrain of the Sierras de Córdoba during RELAMPAGO-CACTI. Weather and Forecasting 37(2), 241-266.
| Crossref | Google Scholar |
Chevallier F, Remaud M, O’Dell CW, Baker D, Peylin P, Cozic A (2019) Objective evaluation of surface- and satellite-driven carbon dioxide atmospheric inversions. Atmospheric Chemistry and Physics 19(22), 14233-14251.
| Crossref | Google Scholar |
Chou M-D, Suarez MJ (1994) An efficient thermal infrared radiation parameterization for use in general circulation models. NASA Technical Memorandum 3, 104606 Available at https://archive.org/details/nasa_techdoc_19950009331.
| Google Scholar |
Coniglio MC, Correia J, Jr, Marsh PT, Kong F (2013) Verification of convection – allowing WRF model forecasts of the planetary boundary layer using sounding observations. Weather and Forecasting 28(3), 842-862.
| Crossref | Google Scholar |
Crippa M, Guizzardi D, Solazzo E, Muntean M, Schaaf E, Monforti-Ferrario F, Banja M, Olivier J, Grassi G, Rossi S, Vignati E (2021) ‘GHG emissions of all world countries.’ (Publications Office of the European Union) Available at https://publications.jrc.ec.europa.eu/repository/handle/JRC126363
Dayalu A, Munger JW, Wofsy SC, Wang Y, Nehrkorn T, Zhao Y, McElroy MB, Nielsen CP, Luus K (2018) Assessing biotic contributions to CO 2 fluxes in northern China using the Vegetation, Photosynthesis and Respiration Model (VPRM-CHINA) and observations from 2005 to 2009. Biogeosciences 15(21), 6713-6729.
| Crossref | Google Scholar |
Dillon ME, Matsudo C, García Skabar Y, Sacco M (2020) Implementación del sistema de pronóstico numérico en el HPC: Configuración de los pronósticos determinísticos. Nota Técnica SMN 2020-78. (Servicio Meteorológico Nacional: Buenos Aires, Argentina) Available at http://hdl.handle.net/20.500.12160/1402 [In Spanish with abstract in Spanish and English]
Dillon ME, Maldonado P, Corrales P, Skabar YG, Ruiz J, Sacco M, Cutraro F, Mingari L, Matsudo C, Vidal L, Rugna M (2021) A rapid refresh ensemble based data assimilation and forecast system for the RELAMPAGO field campaign. Atmospheric Research 264, 105858.
| Crossref | Google Scholar |
Dudhia J (1989) Numerical study of convection observed during the Winter Monsoon Experiment using a mesoscale two-dimensional model. Journal of the Atmospheric Sciences 46, 3077-3107.
| Crossref | Google Scholar |
Fernández ME, Gentili JO, Casado AL, Campo AM (2021) Global horizontal irradiation: spatio-temporal variability on a regional scale in the south of the Pampeana region (Argentina). AUC Geographica 56(2), 220-233.
| Crossref | Google Scholar |
Ferreyra MFG, Curci G, Lanfri M, et al. (2016) First implementation of the WRF-CHIMERE-EDGAR modeling system over Argentina. IEEE Journal of Selected Topics in Applied Earth Observations and Remote Sensing 9(12), 5304-5314.
| Crossref | Google Scholar |
Friedlingstein P, O’Sullivan M, Jones MW, Andrew RM, Gregor L, Hauck J, Le Quéré C, et al. (2022) Global carbon budget 2022. Earth System Science Data 14(11), 4811-4900.
| Crossref | Google Scholar |
Giannaros TM, Melas D, Daglis IA, Keramitsoglou I, Kourtidis K (2013) Numerical study of the urban heat island over Athens (Greece) with the WRF model. Atmospheric Environment 73, 103-111.
| Crossref | Google Scholar |
Gourdji SM, Karion A, Lopez‐Coto I, Ghosh S, Mueller KL, Zhou Y, Williams CA, Baker IT, Haynes KD, Whetstone JR (2022) A modified Vegetation Photosynthesis and Respiration Model (VPRM) for the eastern USA and Canada, evaluated with comparison to atmospheric observations and other biospheric models. Journal of Geophysical Research: Biogeosciences 127(1), e2021JG006290.
| Crossref | Google Scholar |
Grell GA, Devenyi D (2002) A generalized approach to parameterizing convection combining ensemble and data assimilation techniques. Geophysical Research Letters 29, 38-1-38-4.
| Crossref | Google Scholar |
He C, Valayamkunnath P, Barlage M, et al. (2023) The community Noah–MP land surface modelling system technical description version 5.0 number. NCAR Technical Note NCAR/TN-575+STR. (National Center for Atmospheric Research: Boulder, CO, USA) 10.5065/ew8g-yr95
Hersbach H, Bell B, Berrisford P, Biavati G, Horányi A, Muñoz Sabater J, Nicolas J, Peubey C, Radu R, Rozum I, Schepers D, Simmons A, Soci C, Dee D, Thépaut J-N (2023a) ERA5 hourly data on pressure levels from 1940 to present. (Copernicus Climate Change Service, C3S; Climate Data Store, CDS) [Dataset] doi:10.24381/cds.bd0915c6
Hersbach H, Bell B, Berrisford P, Biavati G, Horányi A, Muñoz Sabater J, Nicolas J, Peubey C, Radu R, Rozum I, Schepers D, Simmons A, Soci C, Dee D, Thépaut J-N (2023b) ERA5 hourly data on single levels from 1940 to present. (Copernicus Climate Change Service, C3S; Climate Data Store, CDS) [Dataset] doi:10.24381/cds.adbb2d47
Hilton TW, Davis KJ, Keller K (2014) Evaluating terrestrial CO2 flux diagnoses and uncertainties from a simple land surface model and its residuals. Biogeosciences 11(2), 217-235.
| Crossref | Google Scholar |
Hong S-Y, Lim J-OJ (2006) The WRF single-moment 6-class microphysics scheme (WSM6). Jourmal Korean Meteorological Society 42, 129-151 Available at http://uci.or.kr/G704-000384.2006.42.2.006.
| Google Scholar |
Hong S-Y, Noh Y, Dudhia J (2006) A new vertical diffusion package with an explicit treatment of entrainment processes. Monthly Weather Review 134, 2318-2341.
| Crossref | Google Scholar |
Hu XM, Gourdji SM, Davis KJ, Wang Q, Zhang Y, Xue M, Feng S, Moore B, Crowell SM (2021) Implementation of improved parameterization of terrestrial flux in WRF‐VPRM improves the simulation of nighttime CO2 peaks and a daytime CO2 band ahead of a cold front. Journal of Geophysical Research: Atmospheres 126(10), e2020JD034362.
| Crossref | Google Scholar |
Hurwitz MD, Ricciuto DM, Bakwin PS, Davis KJ, Wang W, Yi C, Butler MP (2004) Transport of carbon dioxide in the presence of storm systems over a northern Wisconsin forest. Journal of the Atmospheric Sciences 61(5), 607-618.
| Crossref | Google Scholar |
Huxman TE, Snyder KA, Tissue D, Leffler AJ, Ogle K, Pockman WT, Sandquist DR, Potts DL, Schwinning S (2004) Precipitation pulses and carbon fluxes in semiarid and arid ecosystems. Oecologia 141, 254-268.
| Crossref | Google Scholar | PubMed |
Iacono MJ, Delamere JS, Mlawer EJ, Shephard MW, Clough SA, Collins WD (2008) Radiative forcing by long-lived greenhouse gases: calculations with the AER radiative transfer models. Journal of Geophysical Research 113, D13103.
| Crossref | Google Scholar |
Janjic ZI (1994) The Step-Mountain Eta Coordinate Model: further developments of the convection, viscous sublayer, and turbulence closure schemes. Monthly Weather Review 122, 927-945.
| Crossref | Google Scholar |
Janjic Z (2019) The surface layer parameterization in the NMM models. Office Note 497, NOAA/NWS/NCEP Environmental Modeling Center, National Oceanic and Atmospheric Administration, US Department of Commerce, College Park, MD, USA. 10.25923/9qej-k604
Jimenez PA, Dudhia J, Gonzalez-Rouco JF, Navarro J, Montavez JP, Garcia-Bustamante E (2012) A revised scheme for the WRF surface layer formulation. Monthly Weather Review 140, 898-918.
| Crossref | Google Scholar |
Ju C, Li H, Li M, Liu Z, Ma Y, Mamtimin A, Sun M, Song Y (2022) Comparison of the forecast performance of WRF using Noah and Noah-MP land surface schemes in Central Asia arid region. Atmosphere 13(6), 927.
| Crossref | Google Scholar |
Lasslop G, Reichstein M, Papale D, Richardson AD, Arneth A, Barr A, Stoy P, Wohlfahrt G (2010) Separation of net ecosystem exchange into assimilation and respiration using a light response curve approach: critical issues and global evaluation. Global Change Biology 16(1), 187-208.
| Crossref | Google Scholar |
Lee TR, De Wekker SF, Andrews AE, Kofler J, Williams J (2012) Carbon dioxide variability during cold front passages and fair weather days at a forested mountain top site. Atmospheric Environment 46, 405-416.
| Crossref | Google Scholar |
Li X, Hu X-M, Cai C, Jia Q, Zhang Y, Liu J, Xue M, Xu J, Wen R, Crowell SM (2020) Terrestrial CO2 fluxes, concentrations, sources and budget in northeast China: observational and modeling studies. Journal of Geophysical Research: AtmospheresAnnals of Botany 125(6), e2019JD031686.
| Crossref | Google Scholar |
Lim K-SS, Hong S-Y (2010) Development of an effective double–moment cloud microphysics scheme with prognostic cloud condensation nuclei (CCN) for weather and climate models. Monthly Weather Review 138, 1587-1612.
| Crossref | Google Scholar |
Lloyd J, Taylor JA (1994) On the temperature dependence of soil respiration. Functional Ecology 8, 315-323.
| Crossref | Google Scholar |
Long Q, Wang F, Ge W, Jiao F, Han J, Chen H, Roig FA, Abraham EM, Xie M, Cai L (2023) Temporal and spatial change in vegetation and its interaction with climate change in Argentina from 1982 to 2015. Remote Sensing 15(7), 1926.
| Crossref | Google Scholar |
Luque SE, Fita L, Pineda Rojas AL (2024) Performance evaluation of the WRF model under different physical schemes for air quality purposes in Buenos Aires, Argentina. Atmósfera 38, 235-262.
| Crossref | Google Scholar |
Magnaldo MA, Libois Q, Riette S, Lac C (2023) Evaluation of surface shortwave downward radiation forecasts by the numerical weather prediction model AROME. EGUsphere 2023, 1-32.
| Crossref | Google Scholar |
Mahadevan P, Wofsy SC, Matross DM, Xiao X, Dunn AL, Lin JC, Gerbig C, Munger JW, Chow VY, Gottlieb EW (2008) A satellite-based biosphere parameterization for net ecosystem CO2 exchange: Vegetation Photosynthesis and Respiration Model (VPRM). Global Biogeochemical Cycles 22(2), GB2005.
| Crossref | Google Scholar |
Markowski P, Richardson Y (2011) ‘Mesoscale meteorology in midlatitudes.’ (Wiley) 10.1002/9780470682104
Maroneze R, Acevedo OC, Costa FD, Puhales FS, Anabor V, Lemes DN, Mortarini L (2021) How is the two-regime stable boundary layer reproduced by the different turbulence parametrizations in the weather research and forecasting model? Boundary-Layer Meteorology 178, 383-413.
| Crossref | Google Scholar |
Mendes J, Zwane N, Mabasa B, Tazvinga H, Walter K, Morcrette CJ, Botai J (2023) An analysis of the effects of clouds in high-resolution forecasting of surface short-wave radiation in South Africa. Journal of Applied Meteorology and Climatology 63, 227-244.
| Crossref | Google Scholar |
Mierzwiak M, Kroszczyński K, Araszkiewicz A (2023) WRF Parameterizations of short-term solar radiation forecasts for cold fronts in central and eastern Europe. Energies 16(13), 5136.
| Crossref | Google Scholar |
Ministerio de Ambiente y Desarrollo Sostenible (2021) Cuarto informe bienal de actualización de Argentina a la Convención Marco de las Naciones Unidas para el Cambio Climático (CMNUCC). Publicación del Ministerio de Ambiente y Desarrollo Sostenible. (MAyDS: Argentina) Available at https://www.argentina.gob.ar/sites/default/files/2022/01/4to_informe_bienal_de_la_republica_argentina.pdf [In Spanish]
Monin AS, Obukhov AM (1954) Основные правила турбулентного перемешивания в приземном слое атмосферы [Basic laws of turbulent mixing in the surface layer of the atmosphere.]. Труды Геофизического Института, Академия наук СССР [Proceedings of the Geophysical Institute, Academy of Sciences of the USSR] 24(151), 163-187 [In Russian].
| Google Scholar |
Müller OV, Lovino MA, Berbery EH (2016) Evaluation of WRF model forecasts and their use for hydroclimate monitoring over southern south America. Weather and Forecasting 31(3), 1001-1017.
| Crossref | Google Scholar |
National Center for Atmospheric Research Earth Observing Laboratory In situ Sensing Facility, Oncley S (2021) NCAR/EOL ISFS Surface Meteorology and Flux Products, 5-minute. Version 2.0. UCAR/NCAR - Earth Observing Laboratory. [Dataset] doi:10.26023/ZPHJ-JW9W-2B0Y
Nesbitt SW, Salio PV, Ávila E, Bitzer P, Carey L, Chandrasekar V, Deierling W, Dominguez F, Dillon ME, Garcia CM, Gochis D (2021) A storm safari in subtropical South America: Proyecto RELAMPAGO. Bulletin of the American Meteorological Society 102(8), E1621-E1644.
| Crossref | Google Scholar |
Oke TR (2002) ‘Boundary layer climates.’ (Routledge) 10.4324/9780203407219
Pal S, Davis KJ, Lauvaux T, Browell EV, Gaudet BJ, Stauffer DR, Obland MD, Choi Y, DiGangi JP, Feng S, Lin B (2020) Observations of greenhouse gas changes across summer frontal boundaries in the eastern United States. Journal of Geophysical Research: Atmospheres 125(5), e2019JD030526.
| Crossref | Google Scholar |
Parazoo NC, Denning AS, Kawa SR, Corbin KD, Lokupitiya RS, Baker IT (2008) Mechanisms for synoptic variations of atmospheric CO2 in North America, South America and Europe. Atmospheric Chemistry and Physics 8, 7239-7254.
| Crossref | Google Scholar |
Peiro H, Crowell S, Schuh A, Baker DF, O’Dell C, Jacobson AR, Chevallier F, Liu J, Eldering A, Crisp D, Deng F (2022) Four years of global carbon cycle observed from the Orbiting Carbon Observatory 2 (OCO-2) version 9 and in situ data and comparison to OCO-2 version 7. Atmospheric Chemistry and Physics 22(2), 1097-1130.
| Crossref | Google Scholar |
Perdigão J, Salgado R, Magarreiro C, Soares PM, Costa MJ, Dasari HP (2017) An Iberian climatology of solar radiation obtained from WRF regional climate simulations for 1950–2010 period. Atmospheric Research 198, 151-162.
| Crossref | Google Scholar |
Pessacg NL, Solman SA, Samuelsson P, Sanchez E, Marengo J, Li L, Remedio ARC, Da Rocha RP, Mourao C, Jacob D (2014) The surface radiation budget over South America in a set of regional climate models from the CLARIS-LPB project. Climate Dynamics 43, 1221-1239.
| Crossref | Google Scholar |
Pleim JE (2007) A combined local and non-local closure model for the atmospheric boundary layer. Part I: model description and testing. Journal of Applied Meteorology and Climatology 46, 1383-1395.
| Crossref | Google Scholar |
Ruiz JJ, Saulo C, Nogués-Paegle J (2010) WRF model sensitivity to choice of parameterization over South America: validation against surface variables. Monthly Weather Review 138(8), 3342-3355.
| Crossref | Google Scholar |
Skamarock WC, Klemp JB, Dudhia J, Gill DO, Liu Z, Berner J, Wang W, Powers JG, Duda MG, Barker DM, Huang X-Y (2019) A description of the advanced research WRF Version 4. NCAR Technical Note NCAR/TN-556+STR. (National Center for Atmospheric Research: Boulder, CO, USA) 10.5065/1dfh-6p97
Stanimirova R, Graesser J, Olofsson P, Friedl MA (2022) Widespread changes in 21st century vegetation cover in Argentina, Paraguay, and Uruguay. Remote Sensing of Environment 282, 113277.
| Crossref | Google Scholar |
Stull RB (1988) ‘An introduction to boundary layer meteorology. Vol. 13.’ (Springer Science & Business Media) 10.1007/978-94-009-3027-8
Suárez M, Poffo D, Pierobon E, Martina A, Saffe J, Rodríguez A (2022) Wind and gust forecasts assessment of Weather Research and Forecast (WRF) model in Córdoba, Argentina. Asian Journal of Atmospheric Environment 16(1), 2021133.
| Crossref | Google Scholar |
Tewari M, Chen F, Wang W, Dudhia J, LeMone MA, Mitchell K, Ek M, Gayno G, Wegiel J, Cuenca RH (2004) Implementation and verification of the unified NOAH land surface model in the WRF model. In ‘84th AMS Annual Meeting: 20th conference on weather analysis and forecasting/16th conference on numerical weather prediction’, 10–15 January 2004, Seattle, WA, USA. Paper 14.2a. (American Meteorological Society) Available at https://ams.confex.com/ams/84Annual/techprogram/paper_69061.htm
Thompson G, Field PR, Rasmussen RM, Hall WD (2008) Explicit forecasts of winter precipitation using an improved bulk microphysics scheme. Part II: implementation of a new snow parameterization. Monthly Weather Review 136, 5095-5115.
| Crossref | Google Scholar |
Varble AC, Nesbitt SW, Salio P, Hardin JC, Bharadwaj N, Borque P, DeMott PJ, Feng Z, Hill TC, Marquis JN, Matthews A (2021) Utilizing a storm-generating hotspot to study convective cloud transitions: the CACTI experiment. Bulletin of the American Meteorological Society 102(8), E1597-E1620.
| Crossref | Google Scholar |
Wyszogrodzki AA, Liu Y, Jacobs N, et al. (2013) Analysis of the surface temperature and wind forecast errors of the NCAR-AirDat operational CONUS 4-km WRF forecasting system. Meteorology and Atmospheric Physics 122, 125-143.
| Crossref | Google Scholar |
Xiao X, Hollinger D, Aber J, Goltz M, Davidson EA, Zhang Q, Moore B, III (2004) Satellite-based modeling of gross primary production in an evergreen needleleaf forest. Remote Sensing of Environment 89(4), 519-534.
| Crossref | Google Scholar |