Characterisation and source apportionment of chemical components in fine particulate matter from atmosphere in two districts of Lanzhou City
Qin Cui
A
B
C
Abstract
Exploring the characterisation and sources of the chemical composition of fine particulate matter in the atmosphere is critical to human health. The main sources of PM2.5 in Lanzhou City are dust, secondary pollution, industry, biomass burning, traffic and coal combustion. In Chengguan District, dust sources are the most significant contributors, whereas secondary pollution sources are dominant in Xigu District. This provides directions and ideas for future local ecological environment management, especially air pollution.
The characterisation and sources of the chemical composition of fine particulate matter in the atmosphere are of critical importance to the environment and human health. As the capital of Gansu Province with a population of more than 4 million people, and being one of important industrial cities in western China, it is of importance to study the characterisation and sources of atmospheric fine particulate matter chemical constituents in Lanzhou City.
In this study, monitoring was carried out from January 2018 to June 2022 in Chengguan and Xigu Districts, and a total of 702 valid samples were collected. Measurements included PM2.5 mass concentrations, water-soluble ions, metals, metalloids and polycyclic aromatic hydrocarbons (PAHs) in both districts.
The results showed that PM2.5 mass concentrations in both districts exhibited a decreasing trend throughout the study period, with seasonal variations characterised as high in winter and spring, and low in summer and autumn. The concentration of water-soluble ions follows the order of SO42− > NO3− > NH4+ > Cl−, with a seasonal distribution pattern of winter > autumn > spring > summer (P < 0.05). The concentrations of metals and metalloids were higher in Xigu District than in Chengguan District, following a seasonal pattern of spring > winter > autumn > summer (P < 0.05). PAHs concentrations were significantly higher in Chengguan District than in Xigu District (P < 0.05), with a seasonal pattern of being high in winter and low in summer (P < 0.05).
The main sources of PM2.5 in Lanzhou City comes from dust, secondary pollution, industry, biomass burning, traffic and coal combustion. Dust sources were the most significant contributors in Chengguan District (37.6%), whereas secondary pollution sources were predominant in Xigu District (40.9%). This provides the latest research evidence exploring feasible pollution reduction pathways for Lanzhou to achieve cleaner skies. The results provide valuable insights and a scientific basis for the prevention and control of atmospheric PM2.5 pollution in Lanzhou City.
Keywords: chemical composition characteristics, fine particulate matter, Lanzhou City, metals and metalloids, polycyclic aromatic hydrocarbons, positive matrix factorisation, source apportionment, water-soluble ions.
Introduction
Fine particulate matter (PM2.5) refers to atmospheric particles with an aerodynamic diameter of 2.5 μm or less, composed of various chemical components. Exposure to PM2.5 can cause various respiratory and cardiovascular diseases (Lepeule et al. 2012; Hodas et al. 2013; Lu et al.2015; Li Z et al. 2017; Canterbury et al. 2020), posing a significant threat to human health. Numerous studies have shown that the composition and sources of atmospheric PM2.5 are intricately linked to natural factors, human activities, industrial operations and urbanisation (Saide et al. 2011; Zhang S et al. 2016), with industrial activities being the primary contributors to PM2.5 pollution (Jiang P et al. 2018). Lanzhou City, a traditional industrial and energy hub in northwest China, hosts key industries such as metal smelting, machinery manufacturing and petrochemicals, which are major sources of PM2.5 emissions. The city has implemented numerous environmental air quality improvement measures, including upgrading and renovating coal-fired boilers, reducing dependence on traditional fossil fuels, promoting the use of clean fuels and controlling vehicle exhaust emissions (Gao and Li 2020). These initiatives have led to a gradual decrease in PM2.5 pollution levels, as reported in the Gansu Province Ecological Environment Bulletin 2018–2022 (Department of Ecology and Environment of Gansu Province 2018, 2019, 2020, 2021, 2022). However, with the ongoing global climate change and increasing complexity of atmospheric pollution, the sources, chemical composition and secondary formation processes of atmospheric PM2.5 have evolved significantly. Pollution now stems from multiple pollutants and sources, leading to regional composite pollution (Chen 2018). Consequently, it is crucial to investigate the composition characteristics and sources of atmospheric PM2.5 pollution to effectively prevent and control atmospheric pollution and protect public health.
Source apportionment of atmospheric particles is a method used to identify and quantify the contribution rates of various emission sources (Zhou 2021). This analysis is based on data such as emissions from pollutant sources, chemical composition characteristics of particles and meteorological elements. Common methods for particle source apportionment include dispersion modelling, source inventory methods and receptor model methods. Among these, the receptor model method, which encompasses techniques such as positive matrix factorisation (PMF), chemical mass balance (CMB), principal component analysis (PCA), enrichment factor (EF) and characteristic ratio (CR), is widely employed (Zheng et al. 2014). This method analyses the physical and chemical properties of particles using mathematical models based on pollution receptors independent of meteorological conditions, geographical information or direct pollution source data. Numerous studies have been conducted on the source apportionment of atmospheric PM2.5 in Lanzhou City. Wang X et al. (2016) utilised the EF and PCA methods to analyse the primary sources of inorganic elements and water-soluble ions in PM2.5 during the non-heating and heating periods in Lanzhou City. Li YH et al. (2016) employed the CR method to study the pollution characteristics and sources of polycyclic aromatic hydrocarbon (PAHs) in the atmospheric PM2.5 of Chengguan District during winter and summer. Similarly, Jiang HM et al. (2021) used the PCA method to explore the characteristics and sources of organic carbon, elemental carbon and water-soluble ions in atmospheric PM2.5 during these seasons. However, these studies primarily focused on qualitative or semi-quantitative methods, such as the CR, EF and PCA methods, which do not fully capture the sources and contribution proportions of PM2.5 in Lanzhou City.
The PMF model, recommended by the US Environmental Protection Agency (EPA) for source apportionment studies of atmospheric particles, addresses these limitations effectively. This model does not require the input of local source spectra. Utilising a large dataset, PMF analyses the composition spectra of various pollution sources under local conditions through detailed constraints, quantifying the contribution rates of various sources over time (Liang et al. 2016). Recent studies using the PMF model in Lanzhou City are scarce. Yang et al. (2022) applied the PMF model to analyse the pollution characteristics and sources of volatile organic compounds during summer in Lanzhou City. Additionally, Ma and Sun (2023) combined the PMF and potential source contribution function (PSCF) to study the local and potential pollution sources of PAHs in PM2.5. These studies, however, lacked a comprehensive analysis of multiple components in PM2.5 and a systematic analysis over an extended period. Therefore, this study systematically analyses the variation of PM2.5 concentration and its chemical composition (water-soluble ions, metals, and metalloids, as well as PAHs) in Chengguan District and Xigu District of Lanzhou City from 2018 to 2022 across all seasons. The EPA PMF model was used to analyse the sources of atmospheric PM2.5 in Lanzhou City and to calculate the contribution rates of various sources. This research aims to provide insights and scientific basis for monitoring and control of PM2.5 pollution in Lanzhou City and to contribute up-to-date research evidence to studies in the fields of climate change, air pollution, and population health in the city.
Material and methods
Monitoring sites and fine particulate matter (PM2.5) sampling
Lanzhou City, located at the interface of the Qinghai–Tibet Plateau and the Loess Plateau, has an altitude exceeding 1500 m and features a continental semi-arid climate. The city stretches from east to west and is narrow from north to south, encompassing five districts (Chengguan, Qilihe, Xigu, Anning and Honggu) and three counties (Yongdeng, Yuzhong and Gaolan). Among these, Chengguan District is the primary urban area with a high population density, characterised by a mix of residential, commercial and industrial activities. It hosts administrative bodies such as the Gansu Provincial Government, Lanzhou Municipal Government and Chengguan District Government. Xigu District is the core industrial zone of Lanzhou City and one of the earliest petrochemical bases in China, home to enterprises like China National Petroleum Corporation’s Lanzhou Petrochemical Company, and various thermal power plants and cement factories. Based on the urban layout of Lanzhou City and the regional characteristics of atmospheric pollutant concentration distribution, two monitoring sites were selected (Fig. 1): Changjiaxiang Primary School in Chengguan District (36°03′00″N, 103°49′48″E) and Yumen Street Primary School (36°06′00″N, 103°37′48″E) in Xigu District, which respectively represent residential and industrial areas. Using the TH-150C Atmospheric TSP Intelligent Mid-Flow PM2.5 Sampler (manufactured by Wuhan Tianhong Instrument Co., Ltd, Wuhan, PR China), PM2.5 was collected regularly from January 2018 to June 2022, from the 10th to the 16th of each month. The sampler operated at a flow rate of 100 L min–1, with sampling periods of no less than 20 h day–1. During each session, sampling time, airflow rate, atmospheric pressure, and temperature were recorded and converted into standard volumes. The sampling filters comprised 90-mm diameter glass fibre and quartz fibre membranes (from Whatman International Ltd, Maidstone, UK). Glass fibre membranes were used for analysing the mass concentration of PM2.5 and PAHs components, whereas quartz fibre membranes were employed for the analysis of water-soluble ions and metal and metalloid components. A total of 702 valid samples were collected throughout the monitoring period (no out-of-town sampling was conducted in February 2020, November 2021, March and April 2022 because of the control of the novel coronavirus pandemic), including 352 samples in Chengguan District and 350 samples in Xigu District. The mass concentrations, water-soluble ions, metals and metalloids, as well as PAHs contents in the samples, were determined, and the atmospheric pollution status of PM2.5 in Lanzhou City was comprehensively analysed and evaluated by comparing the data from the two districts.
Sample analysis
According to HJ 618-2011, the determination of atmospheric articles PM10 and PM2.5 in ambient air by gravimetric method (Ministry of Ecology and Environment 2011), the weight analysis method was used. Using a sampler with specific cutting characteristics, a constant volume of air was drawn to capture ambient PM2.5 on a pre-weighed filter. The PM2.5 concentration was calculated based on the difference in filter weight before and after sampling, and the volume of air sampled. The formulae used for calculation are:
and
where v is the actual sampling volume (m3), Q is the flow rate of the sampling line (L min–1), t is the sampling time (min), ρ is the PM2.5 concentration (mg m–3), w2 is the weight of the filter membrane after sampling (g), and w1 is the weight of the filter membrane before sampling (g). Filters were equilibrated in a chamber with constant temperature and humidity for 24 h before and after sampling, where equilibrium temperature and humidity were recorded. The filters were weighed on a Mettler electronic balance with precision of 0.01 mg. Filters were re-equilibrated under the same conditions for 1 h before a second weighing. For PM2.5 filter samples, a weight difference of less than 0.04 mg between two measurements met the criteria for constant weight. Filters were inspected prior to use to ensure they were free of pinholes or defects, and static electricity was eliminated during weighing. The same analytical balance was used for weighing filters before and after sampling.
According to HJ 799-2016, the ambient air – determination of the water soluble anions (F−, Cl−, Br−, , , , , ) from atmospheric particles – ion chromatography (Ministry of Ecology and Environment 2016a), and HJ 800-2016, the ambient air – determination of water soluble cations (Li+, Na+, , K+, Ca2+, Mg2+) from atmospheric particles – ion chromatography (Ministry of Ecology and Environment 2016b), the content of water-soluble ions in PM2.5 was determined. A one-eigth section of the sampled filter was cut, placed in a centrifuge tube, and submerged in 50 mL of ultrapure water. After soaking for 30 min with the lid on, the tube was sonicated for 20 min. The extract was then filtered through a 0.22-μm micropore filter. An ion chromatograph (Metrohm, Herisau, Switzerland), equipped with an 861 amperometric detector (gold electrode) and an online dialysis unit, was used to measure the content of water-soluble ions (sulfate , nitrate , ammonium , chloride Cl−) in PM2.5. The ion content in the atmosphere was calculated based on the sampling volume. Ion retention times were used for qualitative analysis, and instrument response values for quantitative analysis.
According to HJ 657-2013, the ambient air and stationary source emission – determination of metals in ambient particulate matter – inductively coupled plasma/mass spectrometry (ICP-MS) (Ministry of Ecology and Environment 2013a), the content of metals and metalloids in PM2.5 was determined. A one-eigth section of the sampling filter was taken out and weighed. It was then cut into small pieces using ceramic scissors and placed into a clean polypropylene centrifuge tube. Exactly 10.0 mL of 5% HNO3 was added, ensuring the filter material was fully submerged. The solution was then subjected to ultrasonic extraction at 70°C for 3 h, cooled to room temperature and vigorously shaken. It was subsequently filtered through a 0.22-μm filter membrane, and the filtrate was prepared for analysis. A blank filter was processed in the same manner. X2 type Inductively Coupled Plasma Mass Spectrometry (ICP-MS) (Thermo Fisher Scientific, USA) was used to detect the content of metals such as antimony (Sb), aluminium (Al), arsenic (As), beryllium (Be), cadmium (Cd), chromium (Cr), mercury (Hg), lead (Pb), manganese (Mn), nickel (Ni), selenium (Se) and thallium (Tl) in PM2.5. The content of corresponding metals and metalloids in the atmosphere was derived based on the sampling volume.
In accordance with HJ 647-2013, the ambient air and stationary source emissions – determination of gas and particle-phase polycyclic aromatic hydrocarbons – high performance liquid chromatography (Ministry of Ecology and Environment 2013b), the content of polycyclic aromatic hydrocarbons (PAHs) in PM2.5 was determined. The filter membrane was wrapped in aluminium foil and placed in a muffle furnace to be incinerated at 450°C for 4 h. After cooling, it was balanced in a constant temperature and humidity chamber at 25°C with a relative humidity of 50% for 24 h. It was then weighed on an analytical balance and finally stored in a Teflon-sealed bag for later use. The filter membrane was extracted with a 10/90 (v/v) ether/n-hexane mixture. The extract was then concentrated and purified, and 2695 High-Performance Liquid Chromatograph (from Waters Corporation, USA, equipped with a fluorescence detector) was employed to determine the content of naphthalene (Nap), acenaphthylene (Acy), fluorene (Flu), acenaphthene (Ace), phenanthrene (Phe), anthracene (Ant), fluoranthene (Fla), pyrene (Pyr), chrysene (chr), benzo[a]anthracene (BaA), Benzo[b]fluoranthene (BbF), benzo[k]fluoranthene (BkF), benzo[b]pyrene (BaP), benzo[a,h]anthracene (DahA), benzo[g,h,i]pyrene (BghiP) and indeno[1,2,3-cd]pyrene (IcdP).
Quality control
To ensure the smooth progress of experiments and reliable data analysis, all sampling and inspection personnel undertook identical training. Before each sampling, the sampling filter and a blank filter were balanced under the same conditions for 24 h, weighed and the environmental temperature and humidity were recorded. Both filters were then taken to the sampling site, exposing the blank filter to the same external conditions as the sampling filter. Each batch of samples contained three reagent blanks, three field blanks and three replicate sample analyses. Furthermore, 10% of the samples were taken for replicate analysis, with a relative deviation of less than 20%. It was mandated that after every 20 samples, a standard solution was introduced, and the values must be within 10% of the standard value. If they exceed this range, recalibration and reanalysis were required. All sampling and analysis results are corrected for blank values.
PMF model
The PMF model is widely used for receptor-based source apportionment studies of atmospheric particulate matter (Shi et al. 2021; Groma et al. 2022). The principle of the model involves calculating the errors (e.g. BS, displacement and BS-DISP error estimation) of various chemical components in the particulate matter using weights, and subsequently determining the main sources of the particulate matter and their contributions through the least squares method. This model is primarily employed for the source apportionment of atmospheric particulate matter that has complex origins. Before running the PMF model, concentration files of various chemical components in the samples along with corresponding uncertainty files must be inserted. These inputs are then broken down into factor contribution matrices and factor profile matrices. Subsequently, factors are identified and analysed based on emission sources or emission inventories to calculate parameters such as the contribution of each factor to the samples. The mathematical expression is given as follows:
where i denotes the number of samples, j represents the number of chemical components, p expresses the number of factors, and xij, gik, fkj and eij are the sample concentration matrix, factor contribution rate matrix, factor component spectrum matrix and residual matrix respectively.
The objective of PMF model analysis is to determine the optimal solution by minimising the objective function Q, defined as follows:
where eij denotes the residual of component j in sample i, and uij represents the uncertainty of component j in sample i.
For this study, the United States Environmental Protection Agency (US EPA) PMF model software (ver. 5.0, see https://www.epa.gov/air-research/positive-matrix-factorization-model-environmental-data-analyses) released by the US EPA was employed for source apportionment. A total of 33 chemical components in PM2.5 samples from Chengguan District and Xigu District of Lanzhou City were analysed. Concentration files and uncertainty files for four water-soluble ions (, , Cl−, ), 16 PAHs (Nap, Acy, Flu, Ace, Phe, Ant, Fla, Pyr, Chr, BaA, BbF, BkF, BaP, DahA, BghiP, IcdP) and 12 metals and metalloids (Sb, Al, As, Be, Cd, Cr, Hg, Pb, Mn, Ni, Se, Tl) were fed into the PMF model. The PM2.5 mass concentration was set as the total variable, and the model was run 20 times with factor numbers ranging from 3 to 6. Through analysing the Q values and residuals, multiple iterations were conducted to finally determine the pollution sources and their contribution rates. Based on the source apportionment results of PM2.5 in the two districts, the characteristics of PM2.5 sources in Lanzhou City were explored.
Statistical analysis
A database was established using Excel 2010 (ver. 14.0.7182.5000, Microsoft, Redmond, WA, USA). When concentrations fell below the detection limit, half of the detection limit value was used for calculation. SPSS (ver. 22.0, see https://www.ibm.com/products/spss-statistics) was utilised for the statistical analysis of PM2.5 mass concentrations and its chemical component contents. Normality, tests indicated that the data were non-normally distributed. Therefore, the data were described using the median (P25, P75). Differences in median values between groups were compared using the Kruskal–Wallis test for multiple groups and the Mann–Whitney U non-parametric test for two groups. The significance level was set at α = 0.05 (two-tailed).
Results and analysis
PM2.5 mass concentration
Fig. 2 illustrates the monthly average variations in PM2.5 mass concentration in Chengguan District and Xigu District of Lanzhou City from 2018 to 2022. The peak concentrations in both districts occurred in March 2021, reaching 381 μg m–3 (Chengguan) and 238 μg m–3 (Xigu) – the highest values over the 5 years. This spike was attributed to a severe dust weather event on 14 March 2021, which affected northern China with the most intense dust storm in nearly a decade (Zhang 2021). Lanzhou City, being in the path of the dust storm, experienced severe impacts on air quality. Excluding the intense dust event in March 2021, the overall trend in atmospheric PM2.5 concentration in the two districts showed a monthly decline, with inter-month variations exhibiting a ‘U-shaped’ distribution. The concentration ranged from 19.3 to 116 μg m–3 in Chengguan District to 17.7–135 μg m–3 in Xigu District, with no statistical difference between the two districts (Z = −0.254, P > 0.05). Monthly analysis revealed that in 2018, only 6 months in both districts met the 24-hour average standard of 75 μg m–3 specified in GB 3095-2012, the Chinese Ambient Air Quality Standard (Chinese Research Academy of Environmental Sciences 2012), with the remaining months exceeding this limit. However, after 2021 – excluding the strong dust weather – both districts consistently has not exceeded the 24-h average standard throughout all 12 months, with PM2.5 mass concentrations in 6 months even dropped to the annual average standard of 35 μg m–3.
PM2.5 concentration (μg m–3) in Chengguan District and Xigu District of Lanzhou City from 2018 to 2022.
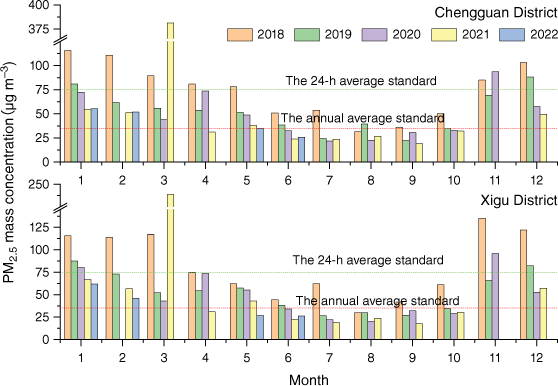
Seasonal differences, categorised into spring (March–May), summer (June–August), autumn (September–November) and winter (December–February), revealed varying PM2.5 concentration differences across the seasons and areas of Lanzhou City. Overall, there was a seasonal pattern of high in winter and spring, low in summer and autumn. Chengguan District, affected by spring dust weather, exhibited significantly higher PM2.5 concentrations in spring compared to other seasons, with a seasonal variation pattern of spring (median (P25, P75), 91.2 (36.8, 75.3) μg m–3) > winter (73.3 (47.0, 99.0) μg m–3) > autumn (46.0 (26.0, 55.0) μg m–3) > summer (32.0 (23.0, 38.0) μg m–3), showing statistical significance (H = 111.170, P < 0.05). By contrast, Xigu District displayed higher PM2.5 concentrations in winter, with a seasonal variation pattern of winter (78.2 (51.0, 100) μg m–3) > spring (76.8 (35.8, 75.3) μg m–3) > autumn (51.8 (27.0, 65.0) μg m–3) > summer (30.8 (22.0, 36.5) μg m–3), also showing statistical significance (H = 117.084, P < 0.05).
PM2.5 chemical components
The four water-soluble ions studied in this research exhibited the following mass concentration order: sulfate () > nitrate () > ammonium () > chloride (Cl−), with monthly average concentrations ranging from 2.24 to 58.7 μg m–3. Throughout the monitoring period, the concentrations of these four water-soluble ions in Chengguan District were ~14.4 (5.99, 17.3) μg m–3, and that in Xigu District were ~14.3 (5.31, 18.3) μg m–3, with no statistical difference between the two districts (Z = −1.286, P > 0.05). Over the 5 years, the total concentrations of the four water-soluble ions in both districts showed a decreasing trend. Seasonal variations (Tables 1 and 2) indicated a distribution pattern of winter > autumn > spring > summer, with all differences being statistically significant (P < 0.05).
Chemical composition | Chengguan District | H | P | |||||
---|---|---|---|---|---|---|---|---|
Spring | Summer | Autumn | Winter | |||||
PM2.5 (μg m– 3) | 91.2 (36.8, 75.3) | 32.0 (23.0, 38.0) | 46.0 (26.0, 55.0) | 73.3 (47.0, 99.0) | 111.17 | <0.05 | ||
Water-soluble ions (μg m– 3) | 10.7 (5.58, 13.6) | 7.41 (5.12, 9.04) | 14.5 (5.84, 19.1) | 24.9 (11.1, 33.3) | 100.209 | <0.05 | ||
Metals and metalloids (ng m– 3) | 789 (215, 791) | 299 (159, 257) | 303 (167, 345) | 459 (262, 532) | 41.46 | <0.05 | ||
PAHs (ng m– 3) | 28.5 (17.6, 35.2) | 27.5 (14.9, 36.1) | 34.1 (16.5, 47.7) | 87.9 (41.1, 119) | 98.837 | <0.05 |
Chemical composition | Xigu District | H | P | ||||
---|---|---|---|---|---|---|---|
Spring | Summer | Autumn | Winter | ||||
PM2.5 (μg m– 3) | 76.8 (35.8, 75.3) | 30.8 (22.0, 36.5) | 51.8 (27.0, 65.0) | 78.2 (51.0, 100) | 117.084 | <0.05 | |
Water-soluble ions (μg m– 3) | 11.0 (5.23, 13.9) | 6.12 (3.62, 8.00) | 15.1 (4.93, 17.3) | 25.3 (11.9, 32.4) | 114.628 | <0.05 | |
Metals and metalloids (ng m– 3) | 909 (278, 880) | 264 (146, 338) | 372 (167, 411) | 488 (288, 549) | 62.786 | <0.05 | |
PAHs (ng m– 3) | 30.3 (16.5, 36.6) | 19.5 (9.20, 25.2) | 26.3 (16.1, 34.4) | 55.9 (27.1, 76.2) | 83.867 | <0.05 |
The and in PM2.5 in the urban atmosphere are mainly derived from the secondary conversion of NOx and SOx produced by fossil fuel combustion. There are significant differences in NOx and SOx emissions from different fossil fuels during combustion (Li et al. 2016). So the ratio of to mass concentrations is commonly used to compare the relative contributions of coal combustion emissions and vehicular exhaust emissions to atmospheric PM2.5. A higher to ratio suggests a greater contribution from mobile sources, whereas a lower ratio indicates the influence of stationary combustion sources (Feng et al. 2020). From Fig. 3, it is evident that during the winters of 2018, 2019 and 2020, the concentration of exceeded that of , with the ratio being significantly higher in winter compared to other seasons, ranging between 1.0 and 2.5. This indicates a significant impact from mobile sources on winter PM2.5 in Lanzhou City during these 3 years. However, after 2021, the monthly average concentration of significantly decreased, with concentrations consistently lower than those of . The ratio of NO3− to SO42− mass concentrations dropped below 1, indicating a dominance of stationary combustion sources.
Heavy metal presence in PM2.5 varies across different regions, influenced by factors such as industrial emissions, population variations and atmospheric transport. During the monitoring period, the average concentrations of 12 metals and metalloids in Chengguan District were recorded at 472 (188, 479) ng m–3. In Xigu District, concentrations were slightly higher, recorded at 519 (198, 518) ng m–3, with no statistically significant difference between the districts (Z = −1.033, P > 0.05). As depicted in Fig. 4, the order of metal and metalloid concentrations in Chengguan District was Al > Pb > Mn > As > Ni > Se > Cd > Cr > Sb > Hg > Tl > Be, whereas the order in Xigu District was Al > Pb > Mn > As > Ni > Cr > Se > Cd > Sb > Hg > Tl > Be. Notably, the average concentration of Sb, Al, As, Be, Cr and Tl were higher in Xigu District than in Chengguan District. Crust metals such as Al, Be, Mn and Cr made up a significant portion of the metal elements (Fig. 4). Seasonal variations showed that metal and metalloid concentrations in both districts were significantly higher in winter compared to other seasons, following the seasonal pattern of winter > autumn > spring > summer (P < 0.05).
The average concentrations of 16 PAHs in Chengguan District of Lanzhou City stood at 44.8 (18.5, 53.6) ng m–3, which was higher than those in Xigu District, also recorded at 33.3 (15.5, 40.5) ng m–3. The difference in concentrations between the two districts was statistically significant (Z = −4.043, P < 0.05). As depicted in Fig. 5, concentrations of three-ring PAHs such as Flu and Ace were relatively low, whereas those of Ant, Fla and Pyr were comparatively high. Seasonal variations showed that PM2.5 mass concentrations of PAHs in both districts were generally higher in winter and lower in summer. This seasonal trend was evident in Chengguan district, with the pattern of winter (87.9 (41.1, 119) ng m–3) > autumn (34.1 (16.5, 47.7) ng m–3) > spring (28.5 (17.6, 35.2) ng m–3) > summer (27.5 (14.9, 36.1) ng m–3) (P < 0.05), whereas with the pattern of winter (55.9 (27.1, 76.2) ng m–3) > spring (30.3 (16.5, 36.6) ng m–3) > autumn (26.3 (16.1, 34.4) ng m–3) > summer (19.5 (9.20, 25.2) ng m–3) in Xigu District (P < 0.05).
PM2.5 source apportionment in Lanzhou City
Some key parameters in running the PMF model include the signal-to-noise (S/N) ratio, concentration relationships, and weighting categories, which are used to assess the performance of the PMF. Upon adding the concentration and uncertainty files into the PMF model, an initial analysis of the source data is necessary to assign strong, weak, or bad attribute to different source components, indicating their relevance in the optimisation process. For example, setting as strong means that the data in that column carries a higher weight, weak reduces the weight, and bad excludes it from calculations. Typically, for component concentrations, a S/N ratio greater than 2 is considered acceptable piece of data, which can be directly used in the model. For components with a S/N ratio between 0.2 and 2, their uncertainty (UNC) is increased to lower the calculation weight, whereas components with a S/N ratio less than 0.2 are not used in the model. Among the 33 components involved in the model calculations, except for setting the PM2.5 mass concentration as the total variable to weak, beryllium (Be) has a S/N ratio of 1.1; therefore, it is also assigned a weak data weight. The remaining components are set to strong. Then, outliers are removed because their presence can easily distort the analytical results. Finally, the number of factors is determined; based on preliminary understanding of local sources, the factors are adjusted and the model optimised incrementally from fewer to more factors. In this study, the seed value is set to 60, and the model is run 20 times, trying 3–6 factors, followed by trial runs. To validate the reasonableness of the approach, the results are evaluated and judged based on the Q value test, residual analysis, linear fit between simulated and actual observational values, time series, factor correlation analysis, and the physical significance of the factor composition spectra. The optimal results are obtained when the number of factors in Chengguan District is 5, as the Q (Robust) value is close to theoretical values, and most parameters meet the requirements. For Xigu District, the results are most consistent with reality when the number of factors is 6.
The source apportionment of PM2.5 in Chengguan and Xigu districts of Lanzhou City is illustrated in Fig. 6. After several optimisations and adjustments, the PMF model’s factor spectra were interpreted using a detailed analysis of source component spectra and emission characteristics. In Chengguan District, five major source categories were identified (Fig. 6).
Source apportionment of PM2.5 in Chengguan District and Xigu District of Lanzhou City (Chengguan District is left, Xigu Districtis right).
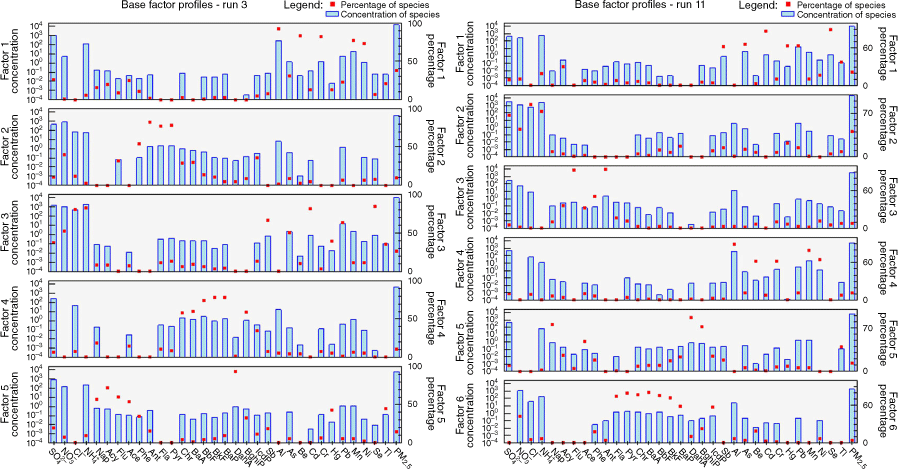
High contributions from Al (92.5%), Be (83.4%), Cr (82.4%), Mn (77.2%) and Ni (73.5%) suggest a strong link with dust sources (Li et al. 2020; Zhang W et al. 2020), identifying this as a soil dust source.
Elevated contributions from Phe (54.0%), Ant (82.5%), Fla (77.8%) and Pyr (78.3%) are markers of coal combustion (Jiang et al. 2013; Dong et al. 2020), identifying this as a coal combustion source.
High characteristic values of water-soluble ions (82.7%), Cl− (80.8%), (37.7%) and (52.3%), representing the primary components of secondary aerosols, closely associated with atmospheric gas precursors such as SOx, HCl, NH3 and NOx, typically indicating secondary pollutants (Liang et al. 2016). The high loadings of Sb (66.2%), As (50.2%), Cd (82.1%), Hg (39.4%), Pb (63.5%) and Se (84.7%) suggested their association with metal smelting and processing, with significant industrial emissions (Cao et al. 2019; Cui et al. 2019; He et al. 2019; Meng et al. 2020), leading to the comprehensive judgment of Factor 3 as a secondary pollution–industrial mixed pollution source.
Predominantly reflects vehicular exhaust (Wang L et al. 2019; Xu et al. 2021), as indicated by high loadings of Chr (57.8%), BaA (60.0%), BbF (74.0%), BkF (77.6%), BaP (78.0%), BghiP (58.4%) and IcdP (34.8%).
High loadings of Nap (57.1%), Acy (72.2%), Flu (59.9%), Ace (53.5%), DahA (93.6%), Hg (42.8%) and TI (44.4%), typical of 2–3 ring PAHs, are significantly associated with biomass burning (Künzli et al. 2010), categorising this as a biomass combustion source.
The proportional contribution of the five sources ranks as follows: dust sources (37.6%) > secondary pollution sources and industrial mixed pollution sources (26.6%) > biomass combustion sources (14.6%) > traffic sources (10.8%) > coal combustion sources (10.3%).
In Xigu District of Lanzhou City, six major source categories were identified (see Fig. 6). Factor 1 exhibited significant contributions from Sb (61.6%), As (65.7%), Cd (87.4%), Hg (64.0%), Pb (63.7%), Se (89.6%) and TI (37.1%), indicating their crucial role in industrial production and marking them as industrial emission indicators (Bowe et al. 2019; Li et al. 2021). Given that Xigu District is part of the petrochemical industrial area, this factor was identified as an industrial source. Factor 2 demonstrated high loadings of (66.3%), (44.8%), Cl− (84.3%) and (72.3%), which are representative of secondary pollution sources. Factor 3 displayed elevated contributions from Acy (37.1%), Flu (93.6%), Ace (32.9%), Phe (51.2%) and Ant (94.5%), and it was identified as a biomass burning source, accounting for 8.3% of the emissions. Factor 4 was primarily associated with soil dust sources, characterised by high loadings of crust elements such as Al (89.5%), Be (62.4%), Cr (62.0%), Mn (80.0%) and Ni (64.8%). Factor 5 showed significant contributions from Nap (75.0%), Ace (47.8%), DahA (87.0%), BghiP (71.3%) and TI (39.6%), primarily originating from the combustion of diesel and gasoline in vehicles (Künzli et al. 2010), thus identified as traffic sources. Factor 6 exhibited high loadings of Fla (74.7%), Pyr (80.4%), Chr (77.3%), BaA (81.7%), BbF (76.3%), BkF (72.0%), BaP (59.3%) and IcdP (57.5%), indicative of coal combustion sources.
In Fig. 7, the proportional size of the six sources is depicted as follows: secondary pollution sources (40.9%) > industrial sources (21.2%) > traffic sources (13.5%) > dust sources (11.9%) > biomass combustion sources (8.3%) > coal combustion sources (4.3%).
Discussion
PM2.5 mass concentration
The research results indicate that the PM2.5 mass concentration in Lanzhou City decreases over the monitoring period, except during the strong dust weather event in March 2021. In 2018, only 6 of the 12 months in both districts met the 24-h average standard of 75 μg m–3, with the remaining months exceeding these limits. However, after excluding the strong dust event in March 2021, both districts consistently met the 24-h average standard throughout the year. During six of these months, the PM2.5 mass concentration even met the more stringent annual average standard of 35 μg m–3. This demonstrates a significant improvement in air quality in Lanzhou City over the past 5 years, attributed to comprehensive measures for atmospheric pollution control. PM2.5 concentrations varied across different seasons and regions within Lanzhou City, exhibiting a characteristic seasonal pattern of being high in winter and spring, and low in summer and autumn. Chengguan District was notably affected by spring dust weather, showing a seasonal variation pattern of spring > winter > autumn > summer (P < 0.05). Conversely, Xigu District experienced a significant increase in air pollutant concentrations during the winter heating season. Additionally, the high calm wind rate combined with low snowfall and rainfall in winter hinder atmospheric circulation, showing the seasonal variation of winter > spring > autumn > summer (P < 0.05). This conclusion is consistent with the conclusion of Zhang GZ et al. (2021). This seasonal variation aligns with the typical ‘U-shaped’ distribution trend of PM2.5 mass concentration observed in many cities across northern China, where concentrations peak during the heating season. The primary cause of this phenomenon is the increased demand for coal during winter for centralised heating, which results in heightened emissions of atmospheric particulate matter. Research by Wang Lili and others on the spatiotemporal characteristics and driving factors of PM2.5 in 89 prefecture-level cities in the Yellow River Basin has also identified a ‘U-shaped’ pattern in monthly average PM2.5 mass concentration changes (Wang LL et al. 2022). During the heating season, concentrations were several times higher than those in the non-heating season in cities such as Xi’an, Yinchuan and Taiyuan (Lin et al. 2020; Peng et al. 2020). Furthermore, the atmospheric structure of Lanzhou in spring is unstable, and the weather process such as strong cooling leads to the dust in the upstream sand source area moving to the downstream Lanzhou. This exogenous transport also aggravates the pollution of particulate matter in Lanzhou in spring (Zhang Y et al. 2015). Additionally, the frequent severe spring dust weather events in Lanzhou, combined with the unique terrain of the river valley basin and a lower atmospheric boundary layer that hinders pollutant diffusion, have contributed to relatively high PM2.5 mass concentrations in the spring. Thus, the challenge of controlling atmospheric PM2.5 pollution remains significant.
PM2.5 chemical composition
Water-soluble ions are the primary components of atmospheric PM2.5 in Lanzhou. In this study, the total concentration of four key water-soluble ions demonstrated a decreasing trend over the years. Due to the heating demand in northern cities, agricultural activities, and coal combustion during autumn and winter, the sources of precursors such as , , and Cl− have increased. Additionally, stable meteorological conditions have led to the continuous accumulation of these primary and secondary ions, resulting in higher concentrations of water-soluble ions in Lanzhou City during the colder months. Conversely, wet deposition and favourable meteorological conditions, such as strong winds during spring and summer, have facilitated the removal of ions, leading to lower total concentrations of water-soluble ions in these seasons. The ratio of to mass concentrations is indicative of the impact of mobile sources on winter PM2.5 levels in Lanzhou City for the years 2018, 2019 and 2020. The cold weather in winter is not conducive to the complete combustion of fuels such as gasoline, exacerbating the emission of NOx, a precursor to nitrate. Furthermore, low temperatures inhibit the volatilisation of , resulting in a significantly higher ratio during winter compared to other seasons. After 2021, there was a significant decrease in the concentration of , indicating a shift toward stationary combustion sources. This suggests that in recent years, the contribution of mobile sources, primarily vehicle exhaust, to PM2.5 in Lanzhou City during winter has gradually decreased, transitioning to a predominance of fixed combustion sources. The content of metals and metalloids in PM2.5 is higher in Xigu District than in Chengguan District, which corresponds with the fact that Xigu District is the core industrial area of Lanzhou City. This district hosts chemical enterprises such as petrochemical plants, thermal power plants, cement factories, and rubber factories, which emit significant amounts of heavy metal particles containing elements like As, Cd, Hg and Pb. Consequently, the total content of metals and metalloids is higher in Xigu District. Affected by spring dust events, the proportion of crust metals such as Al, Be, Cr and Mn among metal elements is higher, with concentrations significantly higher in spring than in other seasons in both districts, following a seasonal distribution of spring > winter > autumn > summer (P < 0.05). Compared to studies on metal content in PM2.5 in both districts of Lanzhou City from 2015 to 2018, which showed higher concentrations in winter (Cheng et al. 2021), there has been a gradual decrease in winter concentrations over the past 5 years, with a trend toward higher concentrations in spring. This shift may be attributed to the implementation of clean heating projects and strengthened comprehensive control of coal combustion pollution in winter in Lanzhou City. These measures have reduced the contribution of anthropogenic activities such as coal combustion to atmospheric pollution, leading to a change in the major sources of metal and metalloid pollutants in atmospheric PM2.5.
PM2.5 source apportionment
Source apportionment serves as a crucial foundation and prerequisite for regional atmospheric particulate pollution prevention and control, enhancing the targeted, scientific nature, and effectiveness of particulate pollution prevention and control measures. The results of the PMF source apportionment model in this study indicate that Lanzhou City has experienced mixed pollution from various sources over the past 5 years, including dust, secondary pollution, industrial emissions, coal combustion, traffic emissions and biomass burning. The contribution rates of different pollution sources vary; in Chengguan District, dust sources are the largest contributors to atmospheric PM2.5, accounting for 37.6% of the total contribution. This is followed by secondary pollution and industrial mixed pollution sources, biomass burning, traffic emissions, and coal combustion, each also accounting for 26.6, 14.6, 10.8 and 10.3% of the total. In Xigu District, the largest contributor to atmospheric PM2.5 is secondary pollution, accounting for 40.9% of the total contribution, followed by industrial emissions, traffic emissions, soil dust, biomass burning, and coal combustion, each also contributing 21.2, 13.5, 11.9, 8.3 and 4.3%. Comparing this data with previous studies reveals (Wei et al. 2017) that the sources and contributions of PM2.5 pollutants in Lanzhou City have varied to different degrees after implementing a series of atmospheric pollution control measures. Therefore, to improve the quality of atmospheric PM2.5 in Lanzhou City, efforts should focus on controlling major pollution sources. In addition to strengthening control over primary emissions, attention should also be given to the control and prevention of precursors for secondary components, especially secondary inorganic ion formation. Moreover, it is essential to establish regional air pollution prevention and control cooperation mechanisms, strengthen comprehensive air pollution control, achieve synergistic effects in pollution and carbon reduction, and enhance the air quality of Lanzhou City to promote green economic development.
Conclusions
From 2018 to 2022, the overall concentration of atmospheric PM2.5 in both districts of Lanzhou City exhibited a decreasing trend, excluding March 2021, which experienced severe dust weather. The seasonal distribution pattern shows higher concentrations in winter and spring, and lower in summer and autumn (P < 0.05). Chengguan District displayed higher PM2.5 concentrations in spring, whereas Xigu District recorded higher concentrations in winter. Tailored measures can be taken to actively address these regional characteristics and further improve environmental air quality.
Different regions exhibit variations in the chemical composition of PM2.5. The concentration of water-soluble ions follows the order of , with a seasonal distribution pattern of winter > autumn > spring > summer (P < 0.05). The annual decrease in the ratio indicates a gradual reduction in the contribution of mobile sources, primarily vehicle exhaust, to PM2.5 in Lanzhou City during winter, transitioning to a predominance of fixed combustion sources. The content of metals and metalloids is higher in the industrial core area of Xigu District compared to Chengguan District, following a seasonal distribution pattern of spring > winter > autumn > summer (P < 0.05). Additionally, the concentration of PAHs in Chengguan District is significantly higher than in Xigu District, exhibiting a seasonal distribution of higher in winter and lower in summer (P < 0.05).
The PMF model revealed that the main sources of PM2.5 in Lanzhou City from 2018 to 2022 were dust, secondary pollution, industrial emissions, biomass burning, traffic emissions, and coal combustion. Chengguan District was primarily affected by dust sources (37.6%), whereas Xigu District was predominantly affected by secondary pollution sources (40.9%). These results provide scientific evidence for the prevention and control of atmospheric PM2.5 in Lanzhou City.
Data availability
The data used in this thesis are available upon request and free of charge from the corresponding author.
Declaration of funding
This study is supported by the Natural Science Foundation of Gansu Province (grant number 22JR5RA711).
References
Bowe B, Xie Y, Yan Y, Al-Aly Z (2019) Burden of cause – specific mortality associated with PM2.5 air pollution in the United States. JAMA Network Open 2(11), e1915834.
| Crossref | Google Scholar | PubMed |
Canterbury A, Echouffo-Tcheugui JB, Shpilsky D, Aiyer A, Reis SE, Erqou S (2020) Association between cumulative social risk, particulate matter environmental pollutant exposure, and cardiovascular disease risk. BMC Cardiovascular Disorders 20, 76.
| Crossref | Google Scholar | PubMed |
Cao N, Huang XM, Zhu Y, Liu H (2019) 西安冬季重污染过程PM2.5理化特征及来源解析 [Physicochemical characteristics and source analysis of PM2.5 during winter heavy pollution in Xi’an]. 中国环境科学 [China Environmental Science] 39(1), 32-39 [In Chinese].
| Crossref | Google Scholar |
Cheng Y, Liu X, Xia Z, Li Y, Sun J (2021) 2015—2018年兰州市两区大气PM2.5中10种金属和类金属元素浓度变化 [Concentration changes of ten metal and metalloid elements in atmospheric PM2.5 in two districts of Lanzhou City from 2015 to 2018.]. 卫生研究 [Journal of Hygiene Research] 50(1), 79-85 [In Chinese].
| Crossref | Google Scholar | PubMed |
Chinese Research Academy of Environmental Sciences (2012) GB 3095-2012. 中国环境空气质量标准 [‘Ambient Air Quality Standard.’] (中国环境科学出版社 [China Environmental Science Press]: Beijing, PR China) Available at https://www.mee.gov.cn/ywgz/fgbz/bz/bzwb/dqhjbh/dqhjzlbz/201203/W020120410330232398521.pdf
Cui Y, Ji D, Chen H, Gao M, Maenhaut W, He J, Wang Y (2019) Characteristics and sources of hourly trace elements in airborne fine particles in urban Beijing, China. Journal of Geophysical Research: Atmospheres 124(21), 11595-11613.
| Crossref | Google Scholar |
Department of Ecology and Environment of Gansu Province (2018) 2018年甘肃省生态环境状况公报 [Gansu Province Ecological Environment Bulletin 2018]. (甘肃省生态环境厅 [Department of Ecology and Environment of Gansu Province]: Lanzhou City, PR China) Available at https://sthj.gansu.gov.cn/sthj/c114873/201906/d080c44dce6343f6b00f7c5890c70438.shtml
Department of Ecology and Environment of Gansu Province (2019) 2019年甘肃省生态环境状况公报 [Gansu Province Ecological Environment Bulletin 2019]. (甘肃省生态环境厅 [Department of Ecology and Environment of Gansu Province]: Lanzhou City, PR China) Available at https://sthj.gansu.gov.cn/sthj/c114873/202006/401887274c6446b9b7c9c9aa6be7507f.shtml
Department of Ecology and Environment of Gansu Province (2020) 2020年甘肃省生态环境状况公报 [Gansu Province Ecological Environment Bulletin 2020]. (甘肃省生态环境厅 [Department of Ecology and Environment of Gansu Province]: Lanzhou City, PR China) Available at https://sthj.gansu.gov.cn/sthj/c114873/202106/1782239.shtml
Department of Ecology and Environment of Gansu Province (2021) 2021年甘肃省生态环境状况公报 [Gansu Province Ecological Environment Bulletin 2021]. (甘肃省生态环境厅 [Department of Ecology and Environment of Gansu Province]: Lanzhou City, PR China) Available at https://sthj.gansu.gov.cn/sthj/c114873/202206/2060986.shtml
Department of Ecology and Environment of Gansu Province (2022) 2022年甘肃省生态环境状况公报 [Gansu Province Ecological Environment Bulletin 2022]. (甘肃省生态环境厅 [Department of Ecology and Environment of Gansu Province]: Lanzhou City, PR China) Available at https://sthj.gansu.gov.cn/sthj/c114873/202306/169872567.shtml
Dong Z, Jiang N, Wang J, Li LP, Guo MM, Zhang RQ (2020) 郑州市大气PM2.5中多环芳烃的污染特征及健康风险评价 [Pollution characterisation and health risk assessment of PM2.5-bound PAHs in ambient air in Zhengzhou.]. 郑州大学学报(理学版) [Journal of Zhengzhou University (Natural Science Edition)] 52(2), 108-113 [In Chinese].
| Crossref | Google Scholar |
Feng YP, Wang S, Xu JY, Liu D, Yao B, Wang Y, Cui J (2020) 承德市细颗粒物水溶性无机离子特征及解析 [Characteristics and source apportionment of water-soluble inorganic ions in atmospheric fine particles in Chengde.]. 环境化学 [Environmental Chemistry] 39(12), 3353-3361 [In Chinese].
| Google Scholar |
Gao XD, Li HJ (2020) 兰州市大气污染治理成效的初步分析 [Preliminary analysis of the effect of air pollution control in Lanzhou City.]. 农业灾害研究 [Journal of Agricultural Catastropholgy] 10(4), 69-70 [In Chinese].
| Crossref | Google Scholar |
Groma V, Alföldy B, Börcsök E, Czömpöly O, Füri P, Kéri AH, et al. (2022) Sources and health effects of fine and ultrafine aerosol particles in an urban environment. Atmospheric Pollution Research 13(2), 101302.
| Crossref | Google Scholar |
He M, Wang N, Long X, Zhang C, Ma C, Zhong Q, et al. (2019) Antimony speciation in the environment: recent advances in understanding the biogeochemical processes and ecological effects. Journal of Environmental Sciences 75, 14-39.
| Crossref | Google Scholar | PubMed |
Hodas N, Turpin BJ, Lunden MM, Baxter LK, Özkaynak H, Burke J, et al. (2013) Refined ambient PM2.5 exposure surrogates and the risk of myocardial infarction. Journal of Exposure Science & Environmental Epidemiology 23(6), 573-580.
| Crossref | Google Scholar | PubMed |
Jiang HM, Li ZQ, Zhang X, Wang FT, Zhou X, Wang FL, Zhang YF, Zheng CY, Song MY, Chen TT (2021) 兰州市冬夏季大气PM2.5化学组分特征及来源分析 [Chemical components characteristics and source analysis of PM2.5 over Lanzhou City in winter and summer.]. 环境科学学报 [Journal of Environmental Science] 41, 1690-1702 [In Chinese].
| Crossref | Google Scholar |
Jiang P, Yang J, Huang C, Liu H (2018) The contribution of socioeconomic factors to PM2.5 pollution in urban China. Environmental Pollution 233, 977-985.
| Crossref | Google Scholar | PubMed |
Jiang QJ, Li YY, Hu XX, Lu B, Tao S, Wang R (2013) 太原市多环芳烃(PAHs)排放清单与分布特征分析 [Estimation of annual emission and distribution characteristics of polycyclic aromatic hydrocarbons (PAHs) in Taiyuan.]. 中国环境科学 [China Environmental Science] 33(1), 14-20 [In Chinese].
| Crossref | Google Scholar |
Künzli N, Jerrett M, Garcia-Esteban R, Basagaña X, Beckermann B, Gilliland F, Medina M, Peters J, Hodis HN, Mack WJ (2010) Ambient air pollution and the progression of atherosclerosis in adults. PLoS ONE 5(2), e9096.
| Crossref | Google Scholar | PubMed |
Lepeule J, Laden F, Dockery D, Schwartz J (2012) Chronic exposure to fine particles and mortality: an extended follow-up of the Harvard Six Cities study from 1974 to 2009. Environmental Health Perspectives 120(7), 965-970.
| Crossref | Google Scholar | PubMed |
Li DY, Qi XB, Wu J, Huang SF, Wang M, Sha CY, Shen C (2021) 大型石化企业邻近区域大气沉降中多环芳烃赋存特征及源解析 [Distribution characteristics and source apportionment of polycyclic aromatic hydrocarbons in atmospheric deposition in areas adjacent to a large petrochemical enterprise.]. 环境科学 [Environmental Science] 42(1), 106-113 [In Chinese].
| Crossref | Google Scholar | PubMed |
Li SW, Chang M, Li H, Cui XY, Ma LQ (2020) Chemical compositions and source apportionment of PM2.5 during clear and hazy days: seasonal changes and impacts of Youth Olympic Games. Chemosphere 256, 127163.
| Crossref | Google Scholar |
Li YH, Rao ZG, Tan JH, Duan JC, Ma YL, He KB (2016) 兰州大气细颗粒物中多环芳烃污染特征及来源分析 [Pollution characteristics and sources analysis of polycyclic aromatic hydrocarbons in atmospheric fine particulate matter in Lanzhou City.]. 环境科学 [Environmental Science] 37(7), 2428-2435 [In Chinese].
| Crossref | Google Scholar | PubMed |
Li Z, Wen Q, Zhang R (2017) Sources, health effects and control strategies of indoor fine particulate matter (PM2.5): a review. Science of The Total Environment 586, 610-622.
| Crossref | Google Scholar | PubMed |
Liang CS, Duan FK, He KB, Ma YL (2016) Review on recent progress in observations, source identifications and countermeasures of PM2.5. Environment International 86, 150-170.
| Crossref | Google Scholar | PubMed |
Lin Y, Yang X, Li Y, Yao S (2020) The effect of forest on PM2.5 concentrations: a spatial panel approach. Forest Policy and Economics 118, 102261.
| Crossref | Google Scholar |
Lu F, Xu D, Cheng Y, Dong S, Guo C, Jiang X, Zheng X (2015) Systematic review and meta-analysis of the adverse health effects of ambient PM2.5 and PM10 pollution in the Chinese population. Environmental Research 136, 196-204.
| Crossref | Google Scholar | PubMed |
Ma KJ, Sun LJ (2023) 兰州市PM2.5中多环芳烃的污染特征和来源解析 [Characterisation and source apportionment of polycyclic aromatic hydrocarbons in PM2.5 in Lanzhou.]. 环境科学 [Environmental Science] 44(11), 5997-6006 [In Chinese].
| Crossref | Google Scholar | PubMed |
Meng Z, Lei P, Zhang T, Ding Y, Chang F (2020) 2016—2018年西安市两城区PM2.5中金属和类金属元素污染特征及来源 [Pollution characteristics and source analysis of metal and metalloid elements in PM2.5 in two districts of Xi’an City from 2016 to 2018.]. 卫生研究 [Journal of Hygiene Research] 49(3), 416-421 [In Chinese].
| Crossref | Google Scholar | PubMed |
Ministry of Ecology and Environment (2011) 中华人民共和国国家环境保护标准 [National Environmental Protection Standards of the People’s Republic of China] HJ 618-2011. 代替GB 6921-86 [Replaces: GB 6921-86] 环境空气 PM10和PM2.5的测定 重量法 Determination of Ambient air PM10 and PM2.5 of gravimetric method. (中国环境科学出版社 [China Environmental Science Press]: Beijing, PR China) Available at https://www.mee.gov.cn/ywgz/fgbz/bz/bzwb/jcffbz/201109/W020120130460791166784.pdf
Ministry of Ecology and Environment (2013a) 中华人民共和国国家环境保护标准 [National Environmental Protection Standards of the People’s Republic of China] HJ 657-2013. 空气和废气 颗粒物中铅等金属元素的测定 电感耦合等离子体质谱法 Ambient air and stationary source emission – determination of metals in ambient particulate matter – inductively coupled plasma/mass spectrometry (ICP-MS). (中国环境科学出版社 [China Environmental Science Press]: Beijing, PR China) Available at https://www.mee.gov.cn/ywgz/fgbz/bz/bzwb/jcffbz/201308/W020130820360019796077.pdf
Ministry of Ecology and Environment (2013b) 中华人民共和国国家环境保护标准 [National Environmental Protection Standards of the People’s Republic of China] HJ 647-2013. 环境空气和废气 气相和颗粒物中多环芳烃的测定 高效液相色谱法 Ambient air and stationary source emission – determination of gas and particle-phase polycyclic aromatic hydrocarbons – high performance liquid chromatography. (中国环境科学出版社 [China Environmental Science Press]: Beijing, PR China) Available at https://www.mee.gov.cn/ywgz/fgbz/bz/bzwb/jcffbz/201306/W020130608556228038217.pdf
Ministry of Ecology and Environment (2016a) 中华人民共和国国家环境保护标准 [National Environmental Protection Standards of the People’s Republic of China] HJ 799-2016. 环境空气 颗粒物中水溶性阴离子 (F−, Cl−, Br−, NO2–, NO3–, PO43–, SO32–, SO42–) 的测定 离子色谱法 Ambient air – determination of the water soluble anions (F−, Cl−, Br−, NO2–, NO3–, PO43–, O32–, SO42–) from atmospheric particles – ion chromatography. (中国环境科学出版社 [China Environmental Science Press]: Beijing, PR China) Available at https://www.mee.gov.cn/ywgz/fgbz/bz/bzwb/jcffbz/201605/W020160519531058998154.pdf
Ministry of Ecology and Environment (2016b) 中华人民共和国国家环境保护标准 [National Environmental Protection Standards of the People’s Republic of China] HJ 800-2016. 环境空气 颗粒物中水溶性阳离子(Li+, Na+, NH4+, K+, Ca2+, Mg2+)的测定 离子色谱法 Ambient air – determination of water soluble cations (Li+, Na+, NH4+, K+, Ca2+, Mg2+) from atmospheric particles – ion chromatography. (中国环境科学出版社 [China Environmental Science Press]: Beijing, PR China) Available at https://www.mee.gov.cn/ywgz/fgbz/bz/bzwb/jcffbz/201605/W020160519529912052417.pdf
Peng J, Dong FQ, Huo TT, Li HL, Li DK (2020) 中国北方典型中小盆地城市大气颗粒物污染特征及趋势对比 [Pollution characteristics and trend comparison of atmospheric particles in the typical small–medium basin cities of northern China.]. 岩石矿物学杂志 [Journal of Petrology and Mineralogy] 39(1), 105-112 [In Chinese].
| Crossref | Google Scholar |
Saide PE, Carmichael GR, Spak SN, Gallardo L, Osses AE, Mena-Carrasco MA, Pagowski M (2011) Forecasting urban PM10 and PM2.5 pollution episodes in very stable nocturnal conditions and complex terrain using WRF–Chem CO tracer model. Atmospheric Environment 45(16), 2769-2780.
| Crossref | Google Scholar |
Shi J, Zhao C, Wang Z, Pang X, Zhong Y, Han X, Ning P (2021) Chemical composition and source apportionment of PM2.5 in a border city in southwest China. Atmosphere 13(1), 7.
| Crossref | Google Scholar |
Wang L, Dong S, Liu M, Tao W, Xiao B, Zhang S, et al. (2019) Polycyclic aromatic hydrocarbons in atmospheric PM2.5 and PM10 in the semi-arid city of Xi’an, northwest China: seasonal variations, sources, health risks, and relationships with meteorological factors. Atmospheric Research 229, 60-73.
| Crossref | Google Scholar |
Wang LL, Liu XJ, Li D, Sun YQ (2022) 黄河流域PM2.5时空特征及驱动因素 [Spatial–temporal characteristics and drivers of PM2.5 in the Yellow River Basin.]. 兰州大学学报(自然科学版) [Journal of Lanzhou University (Natural Sciences)] 58(4), 427-442 [In Chinese].
| Crossref | Google Scholar |
Wang X, Nie Y, Chen H, Wang B, Huang T, Xia DS (2016) 兰州城区大气 PM2.5污染特征及来源解析 [Pollution characteristics and source apportionment of PM2.5, in Lanzhou City.]. 环境科学 [Environmental Science] 37(5), 1619-1628 [In Chinese].
| Crossref | Google Scholar | PubMed |
Wei QZ, Wang YH, Li S, Luo B, Jia Q, Jia L, Zhao H, Yuan XR, Niu JP (2017) 基于PCA-MLR模型的兰州市大气PM2.5污染来源解析 [The source apportionment of PM2.5 based on PCA-MLR model in Lanzhou City.]. 环境卫生学杂志 [Journal of Environmental Hygiene] 7(4), 267-273 [In Chinese].
| Crossref | Google Scholar |
Xu A, Mao Y, Su Y, Shi M, Li X, Chen Z, Qi S (2021) Characterization, sources and risk assessment of PM2.5-bound polycyclic aromatic hydrocarbons (PAHs) in Huanggang City, central China. Atmospheric Environment 252, 118296.
| Crossref | Google Scholar |
Yang YP, Chen Q, Meng XH, Sun J, Wang LN, Tao HJ, Yang LL (2022) 兰州市夏季挥发性有机物污染特征及来源解析 [Summer pollution characteristics and sources of volatile organic compounds in Lanzhou.]. 环境科学 [Environmental Science] 43(12), 5442-5452 [In Chinese].
| Crossref | Google Scholar |
Zhang L (2021) 2021年春季兰州市典型沙尘天气空气质量特征研究 [Study on air quality characteristics of typical dust weather in Lanzhou in spring of 2021.]. 区域治理 [Regional Governance] 24, 35-45 [In Chinese].
| Crossref | Google Scholar |
Zhang GZ, Li DD, Yue YL, Liu PF (2021) 兰州西固区PM2.5和烷醇类分布特征及其与气象因素相关性分析 [The distribution characteristics of PM2.5, alkanol and their correlation with meteorological factors in Xigu District of Lanzhou.]. 兰州理工大学学报 [Journal of Lanzhou University of Technology] 47(02), 65-71 [In Chinese].
| Crossref | Google Scholar |
Zhang S, Han L, Zhou W, Li WF (2016) 城市规模对大气污染物NO2和PM2.5浓度的影响 [Impact of urban population on concentrations of nitrogen dioxide (NO2) and fine particles (PM2.5) in China.]. 生态学报 [Chinese Ecological Journal] 36(16), 5049-5057 [In Chinese with title, author list, keywords and abstract in Chinese and English].
| Crossref | Google Scholar |
Zhang W, Liu B, Zhang Y, Li Y, Sun X, Gu Y, et al. (2020) A refined source apportionment study of atmospheric PM2.5 during winter heating period in Shijiazhuang, China, using a receptor model coupled with a source-oriented model. Atmospheric Environment 222, 117157.
| Crossref | Google Scholar |
Zhang Y, Liu LW, Ning GC, Wang SG, Shang KZ, Zhao WJ (2015) 兰州市大气污染物的分布特征及其对人体健康的影响 [Distribution characteristics of atmospheric pollutants and their effects on human health in Lanzhou City.]. 卫生研究 [Journal of Hygiene Research] 44(5), 723-729 [In Chinese].
| Crossref | Google Scholar | PubMed |
Zheng M, Zhang YJ, Yan CQ, Zhu X, Schauer JJ, Zhang Y (2014) 中国PM2.5来源解析方法综述 [Review of PM2.5 source apportionment methods in China.]. 北京大学学报(自然科学版) [Journal of Natural Sciences of Peking University] 50(6), 1141-1154 [In Chinese].
| Crossref | Google Scholar |
Zhou JX (2021) 南昌市PM2.5的组分特征及来源解析 [Characteristics and sources of PM2.5 component in Nanchang]. PhD dissertation, Nanchang University, Nanchang, PR China. [In Chinese] 10.27232/d.cnki.gnchu.2021.002874