Regional differences in beef cattle trade and movements associated with the COVID-19 pandemic in Australia
Joshua Aboah

A
B
Abstract
The COVID-19 pandemic caused mandated restrictions on movements and distancing of people with implications for meat supply chains. Despite the COVID restrictions, agricultural activities were permitted to continue as essential services in Australia. However, there were reported shortages of meat on the shelves of supermarkets and a general increase in the price of meat in Australia during the COVID period. Hence, there is a need to establish whether the restrictions affected upstream activities in Australia’s beef supply chain.
This study aims to examine the impact of the COVID-19 pandemic and confounding factors including rainfall and export bans on beef cattle trade and movement in Australia.
Using annual data on beef cattle sold, purchased, transferred off-farm and transferred onto farms sourced from the database of the Australian Bureau of Agricultural and Resource Economics and Sciences, a t-test analysis was conducted to establish the significant changes in cattle movement and trade for the pre-COVID (2014–2019) and COVID (2020–2022) periods. Additionally, a flexible least squares model augmented with a flexible generalised least squares model was used to assess the impact of confounding factors on cattle trade and movement.
The results indicate no significant change in the proportion of beef cattle sold nationwide. Tasmania, New South Wales, and Western Australia (WA) recorded significant changes (1.4% increase, 3.6% increase, and 3.8% decrease, respectively) in the proportion of beef cattle purchased. For WA, these changes were localised to changes that occurred in WA Central and Southern Wheat Belt and WA Southwest Coastal regions. Moreover, rainfall did not significantly impact cattle trade but did affect cattle movements. The export ban only significantly impacted beef cattle sold in Queensland.
Overall, the findings suggest that most regions in Australia did not experience significant changes in farm-level decisions regarding beef cattle movement and trade during the COVID period. Instead, minor adjustments were made to address the demand changes.
The findings imply that adopted policies that facilitated the implementation of biosecurity bubbles and the movements of animal transport workers for breeding facilities and abattoirs during the COVID period were effective in normalising farm-level operations.
Keywords: abattoir, beef cattle, biosecurity, COVID-19, farm decisions, food chains, food security, meat works, trade.
Introduction
Farmers’ decisions regarding animal movement and trading are management activities that carry a substantial production risk (Brennan and Christley 2012; Iglesias and East 2015; Hidano et al. 2019; Murato et al. 2023). In response to this risk, government authorities and livestock producers have implemented controls on animal movements (Maye and Chan 2020). These controls are implemented to address consumer risks and off-farm production risks from disease outbreaks and food safety issues. Farmers’ decisions are dynamic in nature within regulatory bounds (Hidano and Gates 2019), and the decision-making process is not solely driven by the pursuit of maximising utility (Hayden et al. 2021). Farm-level decisions can be influenced by other intrinsic and social goals, which in turn, are influenced by the dynamic environment in which agri-businesses operate (Martin-Clouaire 2017). Consequently, farmers’ decisions can be altered by trigger events (Sutherland et al. 2012).
Farmers’ purchasing and trading decisions can be influenced by various triggers including factors related to farm business, farm household considerations, and farmers’ beliefs about farming. For instance, the meat price and cow mortality rates determine the farmers’ livestock selling decisions (Hidano et al. 2019; Kibona and Yuejie 2021). Extant studies have explored various trigger events, primarily focusing on farm-related factors, such as changes in the market prices or personal circumstances and animal disease outbreaks (Sutherland et al. 2012; Hidano and Gates 2019). The COVID-19 (hereafter COVID) pandemic was one such trigger event (Christianson and Barton 2021; Ogier et al. 2023) that has generated significant attention in the food chain literature. Despite the pandemic, agricultural activities were permitted to continue as essential services in Australia (Snow et al. 2021). However, restrictions on people’s movements within and across states, and social distance at the processor levels were mandated by the government (Bucci 2020). There were reported shortages of fresh foods and meat on the shelves of supermarkets (Godrich et al. 2022) and a general increase in the price of meat in Australia during the COVID period (Lewis et al. 2023).
The literature about COVID impacts on the food system in Australia shows that research focus has been on downstream consumption patterns concerning food insecurity (e.g. shift in the affordability of healthy diets and changes in eating habits) (Lee et al. 2021; Mckay et al. 2021; Bennett et al. 2022; Kent et al. 2022; Louie et al. 2022; Mihrshahi et al. 2022; Lewis et al. 2023; Vo et al. 2023) and midstream food distribution from retailers to consumers (Whelan et al. 2021; Godrich et al. 2022). However, the COVID impact on farm-level production decisions has received little attention. The snapshot of the results from the literature review is presented in the supplementary material.
Furthermore, studies that focused on animal movements during this period were only based on experts’ opinions (Iglesias and East 2015). Additionally, there are limited studies to determine whether rainfall patterns and export bans, which have been reported to influence cattle distribution and marketing decisions (Cobon et al. 2020; McKeon et al. 2021; Godrich et al. 2022; Rahmani et al. 2024), contributed to changes in the beef cattle trade and movement activities in Australia. The present study addressed the highlighted research gap by assessing whether the meat shortage and the consequential increase in meat price during the COVID period in Australia could be linked to changes in upstream (farm-level) trading activities emanating from changes in farmers’ decisions or potentially a disruption beyond the farmgate.
The study aimed to examine the impact of the COVID-19 pandemic and confounding factors including rainfall and export bans on beef cattle trade and movement in Australia. This objective was achieved by answering two research questions; (1) did beef cattle trade and movement change significantly across the different states and territories in Australia during the COVID period? and (2) did the rainfall patterns and export bans impact the changes in the beef cattle trade and movement?
Inter- and intra-state/territory differences in beef cattle movement and trade were assessed to provide insights into how these decisions were influenced by the COVID pandemic’s impact on the agricultural sector. Practically, the findings provide policymakers with information that can serve as a foundation in identifying farmers’ behavioural patterns in preparedness for future trigger events.
Methods
Data
Historical annual data on average beef cattle herd size at the farm level, beef cattle sold, purchased, transferred off-farm and transferred onto farms spanning from 2014 to 2022 at state/territory and regional levels were sourced from the Australian Bureau of Agricultural and Resource Economics and Sciences farm data portal (ABARES 2023). The Australian Capital Territory was excluded in this study due to data unavailability. The calendar years between 2014 and 2019 were regarded as the pre-COVID period and the COVID period was 2020 to 2022. The period from 2014 to 2019 was selected to capture short-term trends in cattle trade and movements before the COVID-19 pandemic. Since the first case of COVID-19 in Australia was reported in 2020 (Kleve et al. 2021), the years 2020 to 2022 were designated as the COVID period. For activities beyond the farmgate, the available state/territory-level data on cattle slaughter were sourced from the Australian Bureau of Statistics database, and the cattle price at the processors and the national retail price for beef were retrieved from the price and markets portal of the Meat and Livestock Australia database (MLA 2023). Data on annual average rainfall for all the weather stations at the state/territory level were sourced from Australia’s Bureau of Meteorology database (BoM 2024). Information on periods when export bans were in place was sourced from grey literature including news (Halter 2024).
Empirical analyses
Diagnostic checks were performed to ascertain the appropriate method for estimating the central tendency in the data. The diagnostic check involved plotting the autocorrelation function (ACF) and partial autocorrelation function for four focal indicators of the national-level data: beef cattle purchase, sales, transfer off-farm, and transfer onto-farm. Additionally, the presence of autocorrelation in the data was assessed using the Ljung-Box test, and the optimal model was fitted.
The Ljung-Box test results showed no significant autocorrelation in the residuals of the data. The best-fitted model for the focal indicators was determined to be a white-noise autoregressive integrated moving average model (ARIMA (0,0,0)) with or without a non-zero mean. Consequently, the data lacked an autoregressive component (i.e. the current value is independent of its past value), did not require differencing, and did not exhibit a moving average. Therefore, the mean of the data adequately represented the central tendency of the focal indicators. The proportions of the four indicators (sales, purchases, transfer off and transfer onto farms) were estimated based on the beef cattle herd size.
The t-test statistic (t) was used to establish the significant difference in average proportions of beef cattle herd size that were transferred onto and off the farm, and the proportion sold and purchased at the farm level for the pre-COVID and the COVID periods. The t-test statistic (t) was estimated as shown in Eqn 1, where s2 is the pooled variance of the two periods, and n represents the number of observations for each period.
Analysis of the impact of confounding factors
In this present study, rainfall patterns and export bans were considered as potential confounding factors on beef cattle trade and movement across Australia. The inherent variability in rainfall patterns in Australia has been reported to significantly influence pasture growth, and consequently, livestock production (Cobon et al. 2020; McKeon et al. 2021). The period between 2018 and 2020 was marked by a second prolonged drought period affecting eastern Australia (Hughes et al. 2022). This drought was further compounded by a devastating bushfire crisis that ravaged the landscape from December 2019 to January 2020 (Windsor 2021). In addition, flooding occurred in north Queensland between January and February 2019 (Cowan et al. 2019). Such climatic fluctuations pose ongoing challenges for farmers in managing their herds and maintaining productivity levels.
In response to these varying climatic conditions, strategies such as herd reduction, destocking through sales, and optimising culling ages have been implemented to improve productivity and profitability (Bowen and Chudleigh 2021; Bowen et al. 2022). Hence, considering the intertwined nature of these environmental factors and their impacts on agricultural practices, the variability in rainfall patterns during the COVID period may have exerted a moderating effect on changes in cattle trading activities. Additionally, export bans on live cattle and beef from Australia can significantly impact trading activities, often resulting in the redirection of these exports to local markets. Such a shift was notably observed during the COVID period (Godrich et al. 2022). Within the timeframe considered in this present study, the notable export restriction was the ban of beef from four meat processors (three in Queensland and one in New South Wales) to China between 2020 and 2021 (Halter 2024). However, it is noteworthy that beef production caters to both domestic and export markets, with some substitution between both markets. Therefore, shifts in cattle supply to export markets may not always directly impact domestic supply.
A flexible least squares (FLS) model was used to assess the impact of confounding factors on beef cattle movement and trade before and during the COVID pandemic. FLS, introduced by Kalaba and Tesfatsion (1989), accommodates time-varying regression coefficients, making it apt for cases where coefficients change over time. Unlike traditional approaches, such as those requiring probabilistic properties for residual errors, FLS is particularly suited for situations involving abrupt changes in regression coefficients (βt) (Montana et al. 2009). The FLS solution, outlined in Eqn 2, aimed to achieve a residual efficiency frontier, also known as the incompatibility cost (C) that entails minimising the sum of squared residual measurements (μ) for the total data points (p) and the dynamic error where T is a matrix transposition. T is the total data points and t represents the time period.
As a robustness check, a flexible generalised least square (FGLS) model was also estimated for the same variables shown in Table 1. Unlike the FLS which assumes homoscedasticity, FGLS provides a robust estimation by acknowledging that the variance of the error term may not be constant, thereby accommodating heteroskedasticity and serial correlation in the data.
Predictors | Description | |
---|---|---|
Herd | Average annual herd size at the farm level (number of cattle) | |
Rain(t-1) | Average annual rainfall at the state/territory level (mm) | |
Export | No export ban = 1, Export ban = 2 | |
Era | Period specified as pre-COVID = 1 and COVID = 2 | |
Outcome variables (y i) | ||
Sale(proportion) | Proportion of cattle herd sold each year | |
Sale | Average number of cattle sold each year | |
Buy(proportion) | Proportion of cattle herd purchased each year | |
Buy | Average number of cattle purchased onto farm each year |
The outcome variables were estimated as expressed in Eqn 3, where α, β, and ε represent the intercept, regression coefficient, and the error term, respectively. A linear relationship was assumed between the outcome variables and predictors except rainfall. A third-degree polynomial was specified to model the relationship between rainfall and the outcome variables, allowing for the capture of complex and non-linear relationships, namely, cubic and quadratic relationships in addition to the default linear relationship. A one-year lag was applied to the rainfall data to account for the delayed impact of rainfall on grazing conditions and, consequently, on farmer trading and movement decisions. The year was recoded as a nominal variable to represent the pre-COVID and COVID eras. Similarly, the export ban was coded as a dichotomous variable, indicating periods with or without export bans.
Results
Average beef cattle herd sizes (per farm) were largest in the Northern Territory (NT; 12,044 pre-COVID, 11,225 COVID) followed by Western Australia (WA; 3,316 pre-COVID, 3,989 COVID), Queensland (Qld; 2,243 pre-COVID, 2,223 COVID), Tasmania (Tas; 339 pre-COVID, 352 COVID), New South Wales (NSW; 234 pre-COVID, 213 COVID), South Australia (SA; 216 pre-COVID, 192 COVID), and Victoria (Vic; 89 pre-COVID, 87 COVID) in descending order. An overall decrease in the beef cattle herd size per farm was recorded during the COVID period, except for WA which recorded a 20% increase.
The results indicate no significant changes in the mean proportion of beef cattle herd size sold or purchased nationwide during the COVID period (Table 2). For beef cattle movement, the mean proportion of herd size transferred off-farm increased by 2.3% during the COVID period (from 2.5 to 4.8%) across the country (P-value = 0.04). Similarly, a significant change in the mean proportion of herd size transferred onto farms (P-value < 0.01) was recorded nationwide during the COVID period. The mean proportion transferred onto farms increased by 2.7% nationwide (from 1.2 to 3.9%).
Minimum | 1st quartile | Median | Mean | 3rd quartile | Maximum | ||
---|---|---|---|---|---|---|---|
Pre-COVID (2014–2019) | |||||||
Number of beef cattle | |||||||
Sales | 3 | 72 | 156 | 665 | 629 | 10,510 | |
Transfer off-farm | 0 | 0 | 0 | 284 | 21 | 10,150 | |
Purchases | 0 | 7 | 17 | 50 | 36 | 951 | |
Transfer onto farms | 0 | 0 | 0 | 86 | 8 | 3561 | |
Proportion of beef cattle herd | |||||||
Sales | 9.8% | 28.9% | 38.1% | 40.7% | 51.0% | 90.0% | |
Transfer off-farm | 0% | 0% | 0% | 2.5% | 2.1% | 29.9% | |
Purchases | 0% | 1.2% | 4.2% | 8.2% | 8.7% | 90.6% | |
Transfer onto farms | 0% | 0% | 0% | 1.2% | 0.8% | 18.6% | |
COVID (2020–2022) | |||||||
Number of beef cattle | |||||||
Sales | 1 | 49 | 145 | 647 | 620 | 5901 | |
Transfer off-farm | 0 | 0 | 0 | 522 | 35 | 19,800 | |
Purchases | 0 | 8 | 21 | 49 | 42 | 849 | |
Transfer onto farms | 0 | 0 | 0 | 292 | 27 | 6068 | |
Proportion of beef cattle herd | |||||||
Sales | 3.0% | 25.7% | 38.3% | 41.5% | 49.0% | 223.0% | |
Transfer off-farm | 0% | 0% | 0% | 4.8% | 3.6% | 94.9% | |
Purchases | 0% | 0.9% | 3.5% | 8.1% | 9.8% | 91.3% | |
Transfer onto farms | 0% | 0% | 0% | 3.9% | 1.9% | 53.7% |
Overall, most of the beef cattle movement emanated from the NT as shown in Figs 1, 2. The proportions of cattle transferred onto farms in the NT increased from 2% pre-COVID to 15.6% during the COVID period. Similarly, the proportions of cattle transferred off farms in the NT increased from 6.7% pre-COVID to 18.3% during the COVID period. Queensland recorded the second-highest proportion of beef cattle herd size transferred onto and off farms during the COVID period (5% transfer onto and 4.9% transfer off farms). The results in Fig. 2 also show that despite recording the highest beef cattle sales (in terms of the number of head) before and during the COVID period, the NT recorded the lowest beef cattle sold in proportion to the beef cattle herd size at the farm level during the COVID period. Although Vic recorded the second-highest proportion of cattle purchased pre-COVID, it overtook SA as the state with the highest proportion of cattle purchased during the COVID period. NSW recorded the second-highest proportion of cattle purchased during the COVID period (Fig. 1). The highest beef cattle sold in proportion to the cattle herd size during the COVID period occurred in Vic, displacing SA as the state that recorded the highest proportion of cattle sold pre-COVID (Fig. 2).
Interstate/territory analysis of changes in cattle inflows onto farms
The interstate/territory analysis of the changes in the proportion of beef cattle inflows (cattle purchased, and cattle transferred onto the farm) before and during the COVID period (Table 3) indicates no statistically significant changes in the beef cattle purchased in proportion to the cattle herd size for four states/territory including the NT, Qld, SA, and Vic. The states that recorded significant changes in the proportion of herd size purchased were NSW, Tas and WA. For the proportion of herd size transferred onto the farm, statistically significant increases were observed in the NT and Qld. Detailed results of the interstate/territory analysis are presented in the supplementary material.
NSW | NT | Qld | SA | Tas | Vic | WA | ||
---|---|---|---|---|---|---|---|---|
Proportion of cattle purchased onto farms | + | + | − − − | |||||
Proportion of cattle transferred onto farms | ++ | + | ||||||
Proportion of cattle sold | ||||||||
Proportion of cattle transferred off farms | ||||||||
Cattle export | − − − | +++ | − − − | |||||
Cattle slaughter | − − − | − − − | − − − | − − | − − − |
There was no slaughter data for ACT.
+++, significant increase in proportion during the COVID period at P-value <0.01.
++, significant increase in proportion during the COVID period at P-value <0.05.
+, significant increase in proportion during the COVID period at P-value <0.1.
− − −, significant decrease in proportion during the COVID period at P-value <0.01.
− −, significant decrease in proportion during the COVID period at P-value <0.05.
−, significant decrease in proportion during the COVID period at P-value <0.1.
() Significant increase. (
) Significant decrease. (
) No significant change.
Intrastate/territory analysis of changes in cattle inflows onto farms
The changes in beef cattle inflows in proportion to the herd size in specific regions within each state/territory that recorded statistically significant differences (P-value < 0.05) were further examined to localise the change. For the first type of cattle inflows (i.e. beef cattle purchased), the localised change was assessed for the regions within WA. The results of the intrastate analysis, presented by the trends in Fig. 3, showed that the significant changes in the beef cattle purchased in proportion to the herd size during the COVID period can be localised to changes that occurred on farms in the WA Central and Southern Wheat Belt and the WA Southwest Coastal regions. There was a 5.3% decrease and a 5.7% decrease in the beef cattle purchased in proportion to the herd size in WA Central and Southern Wheat Belt and WA Southwest Coastal regions, respectively. However, no statistically significant difference was observed in the beef cattle purchased onto farms in proportion to beef cattle herd size during the COVID period in the WA Pilbara, Central Pastoral, and Kimberley regions.
Trend of changes in cattle purchased in Western Australia. The shaded area delineates pre-COVID and COVID periods, and ***, **, *, and † represent P-value <0.001, P-value <0.01, P-value <0.05, and P-value <0.1, respectively.
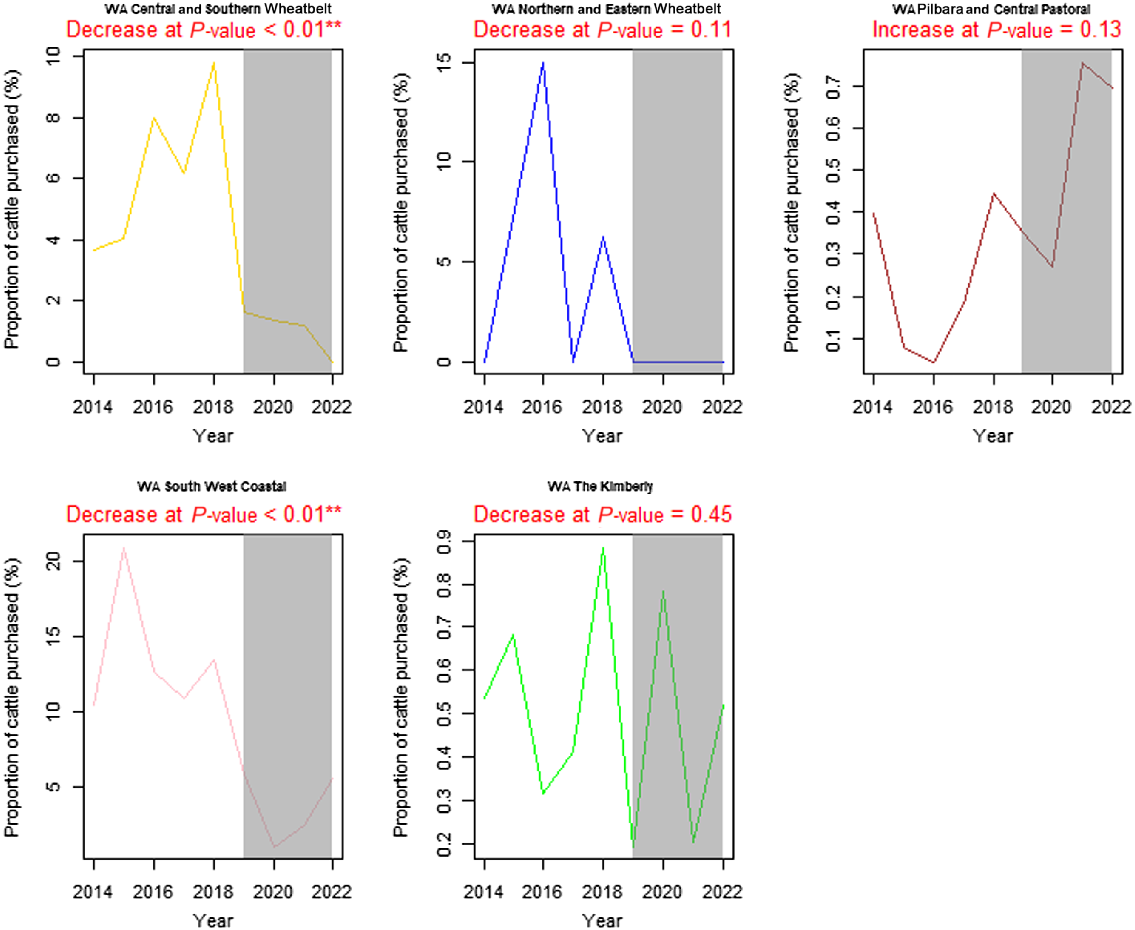
For the second cattle inflows (i.e. cattle transferred onto farms), the localised change was established via intrastate analysis of regions in SA. Fig. 4 shows the trend of the beef cattle transferred onto the farms in proportion to the total herd size. The results indicate that the significant change (decrease) during the COVID period can be traced to the SA Northern Pastoral region (specifically from 2021 to 2022). There were no transfers of beef cattle onto farms in SA Eyre Peninsula and SA Murray Lands and Yorke Peninsula before and during the COVID periods. Although SA Southeast recorded an increase in the proportion of cattle transferred onto farms during the COVID period, the results of the intrastate analysis showed no statistically significant difference. The significant increase in the proportions of cattle transferred onto farms in the Northern Territory can be localised to changes that occurred in the NT Barkly Tablelands, NT Top End Darwin, and NT Vicotria River District – Katherine, as shown in Fig. 5.
Interstate/territory analysis of changes in cattle outflows from farms
There was no statistically significant difference in the number of cattle sold from farms and beef cattle transferred off farms in proportion to the beef cattle herd size nationwide (Table 3). The highest increase in the beef cattle sold in proportion to the on-farm beef cattle herd size during the COVID period was recorded in Vic, followed by the NT. Although not significant, there were decreases in the beef cattle sold in proportion to the herd size in NSW, Qld, Tas, and WA during the COVID period. For these states, the proportion of beef cattle herd size sold was less than 49% in the COVID period, representing an average decrease of 2.5%.
Vic recorded the highest proportion of beef cattle herd size sold (60%) during the COVID period. This proportion represented a 9.2% increase compared to the proportions sold before the COVID period. Similarly, SA recorded a 2.5% increase in the proportion of herd size sold during the COVID period. Another cattle outflow considered in this study was live beef cattle exports. The results of the interstate/territory analysis, represented by the trends in Fig. 6, indicate that these states/territory – Vic, NT, Qld, and WA – recorded an increase in cattle exports during the COVID period. However, changes in the cattle export recorded during the COVID period were significant for Vic, Qld, and WA. Although in NSW and SA there was no significant decrease in beef cattle exports during the COVID period, significant decreases in live cattle exports were recorded in Qld and WA during that time. In sum, the results of the changes in the beef cattle movement and trade at the interstate/territory level indicate that a general decrease in beef cattle trade was recorded in NSW. The NT, which did not have any lockdown, recorded increases in both cattle movement and trade during the COVID period. SA recorded increases in cattle movement and trade, except for cattle export. Vic, which experienced the longest lockdown, recorded decreases in cattle movement on and off-farm but increases in cattle trade.
Activities beyond the farmgate
The cattle slaughtered quarterly before and during the COVID period were compared across the country at the state/territory level. At the national level, the results, as shown in Fig. 7, indicate a statistically significant decrease (22% at a P-value of <0.001) in the total cattle slaughtered during the COVID period. The detailed results of the interstate/territory analysis to localise where the significant changes in the cattle slaughtered occurred at the state/territory level are presented in the supplementary material. The results indicate that there was a decrease in cattle slaughter for all the states across the country during the COVID period. This decrease was statistically significant for all states and territories, except for WA.
A comparison of quarterly cattle slaughtered in Australia before and during COVID. ***, **, *, and † represent P-value <0.001, P-value <0.01, P-value <0.05, and P-value <0.1, respectively.
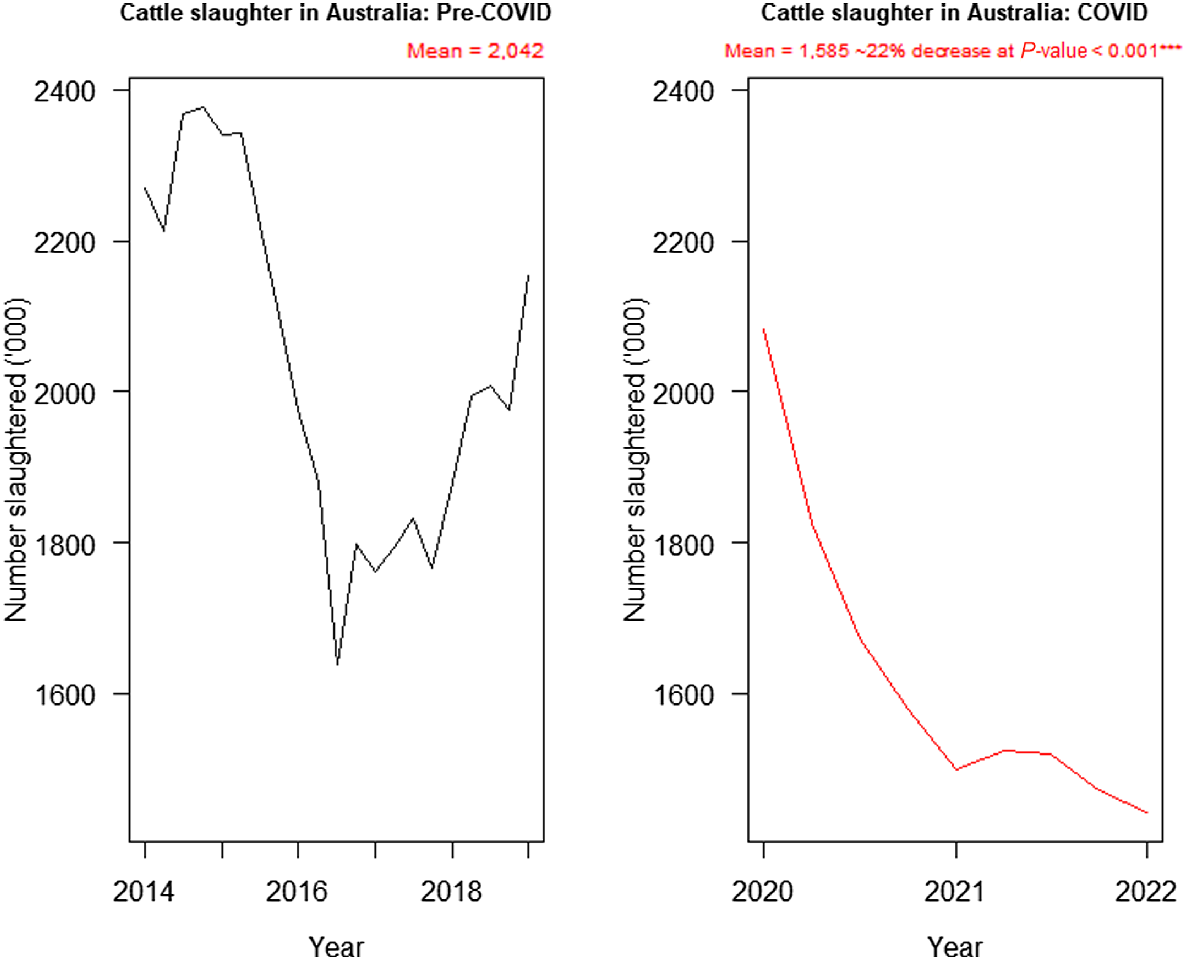
An exploration of changes in price revealed that the price of the processor cattle for three weight categories, 0–200 kg, 280–330 kg, and 330–400 kg, increased by 11.3%, 7.4%, and 12.9%, respectively during the COVID period. (A one-year lag of the price was applied.) A significant increase was recorded for the 280–330 kg weight category. Likewise, the retail price for beef increased by 29% nationwide during the COVID period. Details of the trends for the cattle processor and beef retail prices are presented in the supplementary material.
Confounding factors influencing changes in cattle trade and movement
The average cattle herd size on farms significantly impacted the number of cattle sold in all states and territories except Tas. Results in Table 4 indicate that, ceteris paribus, an increase in herd size led to higher cattle sales. Similarly, an increase in the herd size led to a significant increase in the number of cattle purchased on farms in NSW, SA, and WA, while holding all other factors constant. Additionally, ceteris paribus, an increase in herd size caused a significant increase in cattle transferred off farms in NT, Qld, SA, and WA. Conversely, in Tas, an increase in herd size led to a significant decrease in cattle transferred onto farms. The impact of herd size on transfer of cattle onto farms mirrors the pattern seen with cattle transferred off farms, with Vic also showing a significant positive linear relationship between herd size and cattle transfers onto farms. Moreover, the results showed that the COVID-19 pandemic did not significantly impact the number of cattle sold across Australia. However, there was a significant increase in the cattle purchased on farms in Qld and SA.
Outcomes | Predictors | NSW | NT | Qld | SA | Tas | Vic | WA | |
---|---|---|---|---|---|---|---|---|---|
Number of cattle sold | Herd size | 234.6** | 11193.3** | 3600.5** | 622.3** | 181.2** | 8697.8** | ||
Annual rainfall | |||||||||
Export ban | 288.6† | ||||||||
COVID era | |||||||||
Number of cattle purchased | Herd size | 37.7** | 29.0† | 155.8** | |||||
Annual rainfall | |||||||||
Export ban | |||||||||
COVID era | 114.1† | 43.1* | |||||||
Number of cattle | Herd size | 19186.0** | 2367.1** | 117.9** | −4.7* | 4111.9** | |||
Annual rainfall | −8167.3* | −325.5†(Q) | |||||||
transferred off farm | Export ban | ||||||||
COVID era | |||||||||
Number of cattle transferred onto farm | Herd size | 6702.1** | 336.5* | 92.4** | −4.5† | 1.64* | 2258.5** | ||
Annual rainfall | −3397.0** | ||||||||
Export ban | |||||||||
COVID era | 3547.9* |
(Q) is a quadratic relationship between predictor and outcome variable. The remaining are all linear relationships.
**significant at 1%; *significant at 5%; †significant at 10%. The estimated coefficient precedes the significance level.
Significant with a positive coefficient;
Significant with a negative coefficient;
No significant impact.
The results on the impact of confounding factors indicate that rainfall did not significantly impact cattle trading activities but did significantly impact cattle movement activities in the NT and Qld (Table 4). Ceteris paribus, an increase in the amount of rainfall in the NT led to a significant decrease in the cattle transferred off and onto farms. However, a non-linear (quadratic) relationship was observed between rainfall and the number of cattle transferred off farms in Qld. This non-linear relationship suggests the presence of an inflexion point where the impact of rainfall on cattle transferred off farms changes. The negative coefficient indicates a U-shaped relationship. Initially, as the amount of rainfall increases, the number of cattle transferred off farms decreases. However, beyond the inflexion point, further increases in rainfall led to an increase in cattle transfers.
Export bans did not significantly impact cattle movement activities; however, they only affected the number of cattle sold in Qld, where ceteris paribus, an increase in cattle sales occurred. There was no significant impact on cattle sales in the other states and territories. Details of the results from the regression model are in the supplementary material.
Discussion
This study aimed to investigate the changes in beef cattle movement and trade during the COVID period across and within states/territory in Australia. The findings indicate no significant change in beef cattle movement and the proportion of herd size sold across the country during the COVID period and suggest that the government’s decision to ensure continuous agricultural activities during the COVID period was effective in creating a seemingly business-as-usual condition. Another measure implemented during the COVID period was the demarcation of biosecurity bubbles (Steele et al. 2021). Biosecurity bubbles are controlled zones designated to prevent the entry and spread of potential diseases (in this case COVID). According to Steele et al. (2021), the implementation of biosecurity bubbles and the facilitation of free movement for animal transport workers ensured the prompt transfer of animals to abattoirs.
In addition, the use of online bidding as an alternative to saleyard activities by livestock industry representatives and the extension of visas for agricultural workers during the COVID period may have contributed to the normalcy of trading activities (Baptista et al. 2021). Given the importance of live animal export to the Australian economy, the government ensured that export chains were maintained during the COVID period (Baptista et al. 2021). The present study’s findings showed that there was no statistically significant change in live cattle exports in four states/territory, including NSW, NT, SA and Tas.
Despite the insignificant change in beef cattle sold, Whelan et al. (2021) reported a notable shift in supply and demand dynamics during the COVID period, with demand shocks impacting production activities. Kent et al. (2022) and Godrich et al. (2022) emphasised significant changes in consumer purchasing behaviour due to the COVID-19 pandemic. For instance, consumers resorted to panic buying of unhealthy foods and foods with longer shelf-life (Godrich et al. 2022) and red meat during the COVID period (MLA 2020). Panic buying was associated with supply shortages (Louie et al. 2022), especially in rural areas where access and distribution channels are limited (Whelan et al. 2021). This present study’s findings corroborate previous reports of increased beef cattle sales from farms in some parts of the country, with no delays in transferring cattle to abattoirs (Steele et al. 2021). However, the findings also show a significant decrease in the cattle slaughtered across the country during the COVID period, suggesting potential delays midstream the supply chain, particularly between abattoirs and retailers.
The impact of rainfall on cattle movement is reported with some ambivalence in the literature. For instance, although Tomkins et al. (2009) found no significant effect of rainfall on cattle distribution, Iglesias and East (2015) reported that seasonal weather patterns influenced cattle movement in Australia’s Northern region, with most movement occurring during the dry season. This present study’s findings highlight that increased rainfall led to fewer cattle being transferred onto and off farms. According to Godde et al. (2019), emergency sales of cattle decreased when forage was available. Increased rainfall likely implied improved forage availability, which can reduce the need for emergency sales. However, maintaining an optimal stocking rate is crucial for improving the viability of farm operations (Bowen et al. 2022). Hence, the increase in cattle sold and the insignificant impact of rainfall on cattle sold could be due to herd reduction or destocking strategy to maintain optimal herd size (Bowen et al. 2022) as well as a response from increased demand. This inference is corroborated by the findings on the significant impact of herd size on cattle trade.
The potential moderating effect of lockdowns
Although agricultural activities were classified as essential services and allowed to continue during the COVID period (Snow et al. 2021), the varying durations of lockdowns across the country suggest potential differences in the response from downstream actors. Compared to the other states, the Northern Territory, which has the bigger farm herd size and did not implement any lockdown (Kleve et al. 2021), recorded increases in beef cattle movements and trade during the COVID period. Victoria, which experienced the longest lockdown (Kleve et al. 2021), recorded a decline in beef cattle transfers both off and onto farms; however, cattle sales, purchases, and exports increased during the COVID period. These findings suggest that farmers’ decisions were more triggered by downstream activities rather than upstream production activities. For instance, the findings from the present study on the non-significant decrease in beef cattle sold from farms and the corresponding non-significant decrease in the slaughtered cattle in Western Australia align with the assertion made by Godrich et al. (2022) that supermarkets in Western Australia were able to meet consumer demand for red meat during the COVID period. In addition, the relatively long production time of cattle means that the industry is less agile in being able to respond to short-term changes in demand. Thus, the changes that occurred were gradual rather than abrupt.
The findings of this study are consistent with the reported cascading effects of the COVID pandemic on food chain activities (Murphy et al. 2023). The impact of on-farm decisions cascades downstream in the supply chain (Edwards-Jones 2006). However, farmers’ decisions are heterogeneous, and understanding the nature of triggers underlying these decisions is an important initial step for sensemaking (Martin-Clouaire 2017). Ogier et al. (2023) established that the COVID pandemic had both negative and positive impacts (in terms of price). The decrease in cattle slaughtered at the abattoir level corresponded with increases in processor cattle price and retail price. Thus, the complaints by consumers about retailers increasing prices during the COVID period (Godrich et al. 2022) can be linked to general inflation and the shortage created by the decreased supply due to reduced processing activities.
In response to increased prices, consumers’ purchasing behaviour changed during the COVID period, with reports of food insecurity and increased purchases of unhealthy foods (Grieger et al. 2022). Vo et al. (2023) further highlighted significant short-term disruptions in consumption behaviour during the COVID period, followed by a return to pre-COVID levels when restrictions were lifted. When increasing consumer demand puts pressure on supply from the production sector, the price increases and triggers a change in the supply patterns. Thus, the demand and price dynamics may have posed a moderating effect on farm-level beef cattle trade decisions.
The Australian government’s containment of the COVID-19 pandemic via the lockdown, border closure, and social distance restrictions resulted in the unintended consequence of labour shortages (Lewis et al. 2023; Ogier et al. 2023), which in turn impacted the labour cost. The findings of this study corroborate the reported increase in the price of lean meat during the COVID period (Lee et al. 2021) due to increased demand. Hence, the increased demand was somewhat desirable from the processor and retailer perspectives.
Limitations of the study
The selection of the pre-COVID and COVID periods in this study is a crucial determinant of this study’s findings. For instance, an iteration of results that included data earlier than 2014 for the pre-COVID period showed a significant increase in beef cattle sold during the COVID period rather than the insignificant change reported in this study. Therefore, the findings in this study provide a relatively short-term change perspective instead of a long-term change perspective of on-farm activities. Furthermore, the study focused on the impact of rainfall as the only climatic confounding factor. Other factors like evapotranspiration were not included because the data available did not extend beyond 2017. Moreover, the effect of natural disasters like bushfires and cyclones that occurred within the COVID period, which have been identified as contributing factors to supply shortage (Lewis et al. 2023), were not captured
Moreover, due to data unavailability, the significant changes in the activities beyond the farmgate as represented by the changes in the beef cattle slaughtered were not localised at the intrastate/territory level in this study. An intrastate/territory analysis of the changes that occurred beyond the farmgate would offer more insights into the cascading effect of changes that occurred at the farm level. An extension of this study would be to assess if similar results were recorded in other livestock with relatively shorter lifecycles and fully integrated operations, e.g. poultry and pigs.
Conclusions
This study focused on examining the changes in beef cattle movement and trade before and during the COVID period in Australia. The findings highlight a general increase in beef cattle movement and trade in the NT where no lockdown measures were implemented. There were recorded increases in the proportion of beef cattle herds sold in three states/territory including the NT, Victoria (Vic), and South Australia (SA) during the COVID period. The changes in the beef cattle sold were not statistically significant nationwide. However, there were significant increases in the live cattle export from Vic, despite being the state that implemented the longest lockdown. In general, there was no significant change in the beef cattle movement (transfers off and onto farms) nationwide despite the increases recorded in NT, SA, and WA. Moreover, the findings showed that rainfall patterns did not have a significant impact on cattle trade but did impact cattle movement. Additionally, the findings showed that the Chinese export bans impacted cattle sales only in Queensland.
The findings showed that the significant shortage in the supply chain can be linked to the significant decrease in the number of cattle slaughtered during the COVID period nationwide, leading to an increase in retail prices. According to Sutherland et al. (2012), farmers’ decisions often follow a path dependency characterised by minor incremental changes until a trigger event prompts a significant shift. In line with this perspective, the findings of this present study indicate that most regions across the country did not experience significant changes in farm-level decisions regarding beef cattle movement and trade during the COVID period. Instead, there were minor adjustments made to address the growing demand. Primarily, regions that witnessed significant changes in farm-level decisions were already characterised by a high frequency of cattle movements even before the COVID-19 pandemic. Thus, the findings suggest that the COVID-19 pandemic did not provide a strong trigger to cause changes in the farm-level decisions on beef cattle movement and trade in Australia.
In conclusion, this study’s findings suggest that the measures implemented during the COVID period, such as the institution of biosecurity bubbles, the facilitation of free movements of animal transport workers and veterinarians in Australia and the diversion of beef cattle export to the domestic market may have been effective in normalising farm-level operations across the country. Hence, these measures could serve as an elevator for the supply chain preparedness for future disruptions not related to disease transmission in animals.
Data availability
The data that support this study are available in the article and accompanying online supplementary material.
References
ABARES (2023) Farm Data Portal Beta v1.21. Australian Bureau of Agricultural and Resource Economics and Sciences. Available at https://www.agriculture.gov.au/abares/data/farm-data-portal#data-definitions-concepts-and-methods [accessed 11 July 2023]
Baptista J, Blache D, Cox-Witton K, Craddock N, Dalziel T, De Graaff N, Fernandes J, Green R, Jenkins H, Kahn S, Kelly D, Lauber M, Maloney SK, Peachey B, Rodger I, Skuse J, Tilbrook AJ, Walker FR, Wall K, Zito S (2021) Impact of the COVID-19 pandemic on the welfare of animals in Australia. Frontiers in Veterinary Science 7, 621843.
| Crossref | Google Scholar | PubMed |
Bennett CJ, Christian M, Phan S, McCabe M, Cornish K, Kleve S (2022) Food insecurity during COVID-19: an Australian university experience. Health & Social Care in the Community 30(6), e5401-e5411.
| Crossref | Google Scholar |
BoM (2024) Climate data. Australian Bureau of Meteorology. Available at http://www.bom.gov.au/cgi-bin/climate/change/timeseries.cgi?graph=rranom&area=wa&season=0112&ave_yr=A
Bowen MK, Chudleigh F (2021) Achieving drought resilience in the grazing lands of northern Australia: preparing, responding and recovering. The Rangeland Journal 43(3), 67-76.
| Crossref | Google Scholar |
Bowen MK, Chudleigh F, Sallur NM, Sommerfield J (2022) Opportunities to build resilience of beef cattle properties in the mulga lands of south-western Queensland, Australia. The Rangeland Journal 44(2), 115-128.
| Crossref | Google Scholar |
Brennan ML, Christley RM (2012) Biosecurity on cattle farms: a study in north-west England. PLoS ONE 7(1), e28139.
| Crossref | Google Scholar | PubMed |
Bucci N (2020) Meatworks and coronavirus: the ‘domino effect’ from Victoria’s abattoirs pushing COVID-19 case numbers higher. ABC News 26 July. Available at https://www.abc.net.au/news/2020-07-26/coronavirus-covid-19-meatworks-abattoirs-victoria/12490178.
| Google Scholar |
Christianson MK, Barton MA (2021) Sensemaking in the time of COVID-19. Journal of Management Studies 58(2), 572-576.
| Crossref | Google Scholar |
Cobon DH, Stone G, Carter J, McKeon G, Zhang B, Heidemann H (2020) Native pastures and beef cattle show a spatially variable response to a changing climate in Queensland, Australia. European Journal of Agronomy 114, 126002.
| Crossref | Google Scholar |
Cowan T, Wheeler MC, Alves O, Narsey S, de Burgh-Day C, Griffiths M, Jarvis C, Cobon DH, Hawcroft MK (2019) Forecasting the extreme rainfall, low temperatures, and strong winds associated with the northern Queensland floods of February 2019. Weather and Climate Extremes 26, 100232.
| Crossref | Google Scholar |
Edwards-Jones G (2006) Modelling farmer decision-making: concepts, progress and challenges. Animal Science 82(6), 783-790.
| Crossref | Google Scholar |
Godde C, Dizyee K, Ash A, Thornton P, Sloat L, Roura E, Henderson B, Herrero M (2019) Climate change and variability impacts on grazing herds: insights from a system dynamics approach for semi-arid Australian rangelands. Global Change Biology 25(9), 3091-3109.
| Crossref | Google Scholar |
Godrich SL, Lo J, Kent K, Macau F, Devine A (2022) A mixed-methods study to determine the impact of COVID-19 on food security, food access and supply in regional Australia for consumers and food supply stakeholders. Nutrition Journal 21, 17.
| Crossref | Google Scholar |
Grieger JA, Habibi N, O’Reilly SL, Harrison CL, Moran LJ, Vo H, Sabir S, Enticott J, Teede H, Siew Lim MND (2022) Psychological distress and its association with intake of sugar-sweetened beverages, discretionary foods, and alcohol in women during the COVID-19 pandemic in Australia. Nutrition 103, 111794.
| Crossref | Google Scholar |
Halter A (2024) Australian beef industry looks towards resuming China trade after COVID-19 bans. Available at https://www.abc.net.au/news/rural/2024-05-06/australian-beef-industry-looks-to-resume-trade-china-covid-bans/103794206
Hayden MT, Mattimoe R, Jack L (2021) Sensemaking and the influencing factors on farmer decision-making. Journal of Rural Studies 84, 31-44.
| Crossref | Google Scholar |
Hidano A, Gates MC (2019) Why sold, not culled? Analysing farm and animal characteristics associated with livestock selling practices. Preventive Veterinary Medicine 166, 65-77.
| Crossref | Google Scholar | PubMed |
Hidano A, Gates MC, Enticott G (2019) Farmers’ decision making on livestock trading practices: cowshed culture and behavioural triggers amongst New Zealand dairy farmers. Frontiers in Veterinary Science 6, 320.
| Crossref | Google Scholar | PubMed |
Hughes N, Lu M, Soh WY, Lawson K (2022) Modelling the effects of climate change on the profitability of Australian farms. Climatic Change 172(1), 12.
| Crossref | Google Scholar |
Iglesias RM, East IJ (2015) Cattle movement patterns in Australia: an analysis of the NLIS database 2008–2012. Australian Veterinary Journal 93(11), 394-403.
| Crossref | Google Scholar | PubMed |
Kalaba R, Tesfatsion L (1989) Time-varying linear regression via flexible least squares. Computers & Mathematics with Applications 17(8–9), 1215-1245.
| Crossref | Google Scholar |
Kent K, Murray S, Penrose B, Auckland S, Godrich S, Lester E, Visentin D (2022) Food insecure households faced greater challenges putting healthy food on the table during the COVID-19 pandemic in Australia. Appetite 169, 105815.
| Crossref | Google Scholar | PubMed |
Kibona CA, Yuejie Z (2021) Factors that influence market participation among traditional beef cattle farmers in the Meatu District of Simiyu Region, Tanzania. PLoS ONE 16(4), e0248576.
| Crossref | Google Scholar | PubMed |
Kleve S, Bennett CJ, Davidson ZE, Kellow NJ, McCaffrey TA, O’Reilly S, Enticott J, Moran LJ, Harrison CL, Teede H, Lim S (2021) Food insecurity prevalence, severity and determinants in Australian households during the COVID-19 pandemic from the perspective of women. Nutrients 13(12), 4262.
| Crossref | Google Scholar |
Lee AJ, Patay D, Herron L-M, Tan RC, Nicoll E, Fredericks B, Lewis M (2021) Affordability of heathy, equitable and more sustainable diets in low-income households in Brisbane before and during the COVID-19 pandemic. Nutrients 13(12), 4386.
| Crossref | Google Scholar | PubMed |
Lewis M, Herron L-M, Chatfield MD, Tan RC, Dale A, Nash S, Lee AJ (2023) Healthy food prices increased more than the prices of Unhealthy Options during the COVID-19 Pandemic and Concurrent Challenges to the Food System. International Journal of Environmental Research and Public Health 20(4), 3146.
| Crossref | Google Scholar | PubMed |
Louie S, Shi Y, Allman-Farinelli M (2022) The effects of the COVID-19 pandemic on food security in Australia: a scoping review. Nutrition & Dietetics 79(1), 28-47.
| Crossref | Google Scholar | PubMed |
Martin-Clouaire R (2017) Modelling operational decision-making in agriculture. Agricultural Sciences 8(7), 527-544.
| Crossref | Google Scholar |
Maye D, Chan KWR (2020) On-farm biosecurity in livestock production: farmer behaviour, cultural identities and practices of care. Emerging Topics in Life Sciences 4(5), 521-530.
| Crossref | Google Scholar | PubMed |
McKay FH, Bastian A, Lindberg R (2021) Exploring the response of the Victorian emergency and community food sector to the COVID-19 pandemic. Journal of Hunger & Environmental Nutrition 16(4), 447-461.
| Crossref | Google Scholar |
McKeon G, Stone G, Ahrens D, Carter J, Cobon D, Irvine S, Syktus J (2021) Queensland’s multi-year Wet and Dry periods: implications for grazing enterprises and pasture resources. The Rangeland Journal 43(3), 121-142.
| Crossref | Google Scholar |
Meat and Livestock Australia (2020) COVID-19 crisis sees surge in domestic retail demand. Available at https://www.mla.com.au/prices-markets/market-news/2020/covid-19-crisis-sees-surge-in-domestic-demand/ [accessed 21 September 2020]
Mihrshahi S, Dharmayani PNA, Amin J, Bhatti A, Chau JY, Ronto R, Turnip D, Taylor M (2022) Higher prevalence of food insecurity and psychological distress among international university students during the COVID-19 pandemic: an australian perspective. International Journal of Environmental Research and Public Health 19(21), 14101.
| Crossref | Google Scholar | PubMed |
MLA (2023) Price and Market Database. Meat and Livestock Authority. Available at https://www.mla.com.au/prices-markets/slaughter/ [accessed 11 July 2023]
Montana G, Triantafyllopoulos K, Tsagaris T (2009) Flexible least squares for temporal data mining and statistical arbitrage. Expert Systems with Applications 36(2), 2819-2830.
| Crossref | Google Scholar |
Murato Y, Hayama Y, Kondo S, Sawai K, Yamaguchi E, Yamamoto T (2023) Region-wise analysis of beef cow movements in Japan. Frontiers in Veterinary Science 10, 1012978.
| Crossref | Google Scholar | PubMed |
Murphy M, Carey R, Alexandra L (2023) Building the resilience of agri-food systems to compounding shocks and stresses: a case study from Melbourne, Australia. Frontiers in Sustainable Food Systems 7, 1130978.
| Crossref | Google Scholar |
Ogier EM, Smith DC, Breen S, Gardner C, Gaughan DJ, Gorfine HK, Hobday AJ, Moltschaniwskyj N, Murphy R, Saunders T, Steer M, Woodhams J (2023) Initial impacts of the COVID-19 pandemic on Australian fisheries production, research organisations and assessment: shocks, responses and implications for decision support and resilience. Reviews in Fish Biology and Fisheries 33(2), 513-534.
| Crossref | Google Scholar | PubMed |
Rahmani E, Khatami M, Stephens E (2024) Using probabilistic machine learning methods to improve beef cattle price modelling and promote beef production efficiency and sustainability in Canada. Sustainability 16(5), 1789.
| Crossref | Google Scholar |
Snow V, Rodriguez D, Dynes R, Kaye-Blake W, Mallawaarachchi T, Zydenbos S, Cong L, Obadovic I, Agnew R, Amery N, Bell L, Benson C, Clinton P, Fernanda Dreccer M, Dunningham A, Gleeson M, Harrison M, Hayward A, Holzworth D, Johnstone P, Meinke H, Mitter N, Mugera A, Pannell D, Silva LFP, Roura E, Siddharth P, Siddique KHM, Stevens D (2021) Resilience achieved via multiple compensating subsystems: the immediate impacts of COVID-19 control measures on the agri-food systems of Australia and New Zealand. Agricultural Systems 187, 103025.
| Crossref | Google Scholar |
Steele SG, Toribio JALML, Mor SM (2021) Global health security must embrace a One Health approach: contributions and experiences of veterinarians during the COVID-19 response in Australia. One Health 13, 100314.
| Crossref | Google Scholar | PubMed |
Sutherland L-A, Burton RJF, Ingram J, Blackstock K, Slee B, Gotts N (2012) Triggering change: towards a conceptualisation of major change processes in farm decision-making. Journal of Environmental Management 104, 142-151.
| Crossref | Google Scholar | PubMed |
Tomkins NW, O’Reagain PJ, Swain D, Bishop-Hurley G, Charmley E (2009) Determining the effect of stocking rate on the spatial distribution of cattle for the subtropical savannas. The Rangeland Journal 31(3), 267-276.
| Crossref | Google Scholar |
Vo LH, Martinus K, Smith B (2023) A demand systems approach to understanding medium-term post-pandemic consumption trends. Economic Papers: A Journal of Applied Economics and Policy 42, 183-199.
| Crossref | Google Scholar |
Whelan J, Brown AD, Coller L, Strugnell C, Allender S, Alston L, Hayward J, Brimblecombe J, Bell C (2021) The impact of COVID-19 on rural food supply and demand in Australia: utilising group model building to identify retailer and customer perspectives. Nutrients 13(2), 417.
| Crossref | Google Scholar | PubMed |
Windsor PA (2021) Progress with livestock welfare in extensive production systems: lessons from Australia. Frontiers in Veterinary Science 8, 674482.
| Crossref | Google Scholar |