Using mid-infrared spectroscopy to identify more fertile cows for insemination to sexed semen
Joanna E. Newton
A Agriculture Victoria, AgriBio, Centre for AgriBioscience, Bundoora, Vic. 3083, Australia.
B School of Applied Systems Biology, La Trobe University, Bundoora, Vic. 3083, Australia.
Abstract
Broader applications of milk mid-infrared spectral data could add value to milk-recording data. One such application is to rank cows on the probability of conception to first service (MFERT) which could help prioritise cows for insemination with dairy sexed semen (SS).
This study compared the use of MFERT estimates against two other approaches, to (1) identify most and least fertile dairy cows and (2) prioritise cows predicted to be most fertile for first service insemination with SS.
Mid-infrared spectral data from first herd test after calving was used to generate 13 379 MFERT predictions for 76 cohorts. Reproduction records were used to calculate reproductive parameters, calf numbers and net benefit, i.e. calf values minus mating costs, for two breeding programs. Breeding program 1 used SS and conventional dairy semen, while Breeding program 2 used SS, conventional dairy and beef semen. Three semen-allocation approaches were compared, namely, allocation via MFERT, calving date (CDATE) or assignment via random number generator (RANDOM).
MFERT significantly outperformed (1) RANDOM in identifying cows most and least likely to calf after first insemination (P < 0.05), and (2) both CDATE and RANDOM in identifying cows most and least likely to calf overall (P < 0.05). This resulted in up to 1.5 and 4.5 more dairy heifer calves, in Breeding programs 1 and 2 respectively, and up to six fewer dairy-beef calves in Breeding program 2. Differences in net benefit among semen-allocation approaches were modest, although generally favoured MFERT. Few significant differences between MFERT and CDATE were found. However, significant net benefit differences among all three semen-allocation approaches were seen in Breeding program 2.
MFERT outperformed CDATE and RANDOM in identifying most and least fertile cows. Realised net benefits of semen allocation by MFERT over other approaches were modest. Given the impact of semen type and dairy-beef calf prices value proposition will vary.
Our study confirmed that MFERT can add value to milk recording data by identifying the most and least fertile cows. As MFERT value is sensitive to individual farm parameters, incorporation alongside other fertility parameters into a decision support tool is desirable.
Keywords: artificial insemination, breeding program, dairy-beef, dairy breeding, herd recording, mid-infrared spectroscopy, milk recording, reproduction.
Introduction
Milk recording is routinely practiced on Australian dairy farms, with resulting data being used to support objective management decisions such as culling and ending a cow’s lactation (Newton et al. 2020). Increasingly, milk-sampling laboratories are installing mid-infrared spectroscopy (MIR) equipment which provides opportunities to extract more information from a milk sample, with minimal additional labour inputs on farm. Novel MIR-derived phenotypes have been reported in literature for cow health, milk composition, environmental footprint and fertility (De Marchi et al. 2014; Grelet et al. 2021). Achieving high prediction accuracy for novel MIR-derived phenotypes and demonstrating that they have on-farm applications could increase the value proposition for herd recording. This may help reverse declining participation in herd recording.
As fertility is of high importance to farmers (Martin-Collado et al. 2015), novel MIR-derived fertility phenotypes are likely to gain traction on farm if sufficient accuracy can be achieved. Recently, Ho and Pryce (2020) developed a model using on-farm data, including MIR collected in early lactation, to rank cows on the probability of conceiving at first insemination (MFERT). They have achieved a prediction accuracy up to 76%. In our previous study (Newton et al. 2021), when comparing the top and bottom 20% of cows ranked on Ho and Pryce (2020)’s MFERT, we found, on average, a 16 percentage point difference in first-service conception rates across 76 herd-year–calving season cohorts.
Being able to identify cows that are both more or less likely to conceive at first service could have applications in breeding decisions, particularly in supporting targeted use of sexed semen (SS). Reported benefits of the use of female dairy SS in dairy herds include: (1) increased numbers of female replacements; (2) increased genetic gain; (3) improved market opportunities for calves; and (4) improved calf and cow welfare outcomes (Holden and Butler 2018). Initial SS conception rates were only ~70–80% of those of conventional semen (Butler et al. 2014). This has posed a barrier and tainted adoption of SS, as the economic consequences of a reduction in fertility quickly outweigh the benefits of SS (Holden and Butler 2018). However, more recent studies (Izzo 2015; Noonan et al. 2016) have illustrated that comparable SS conception rates are possible in well managed herds. Growing industry confidence in SS is reflected in a rapid increase in SS sales. In 2021, SS was 22% of dairy semen sales in Australia, compared with 10% in 2019 and 5% in 2015 (NHIA 2022). Another barrier to adoption is the high price of SS compared with conventional semen, often being double the price per straw (Genetics Australia 2021; Walsh et al. 2021). Therefore, prioritising the use of SS on more fertile cows is of great interest to farmers. Prioritisation strategies previously used for targeted semen allocation include early calving cows with high body condition score (Butler et al. 2014), 2–4-year-old cows with no peri-parturient disease and heifers who have reached 60–65% of mature cow bodyweight (Izzo 2015).
One approach currently used is to allocate SS to the earliest calving cows. Cows calving later in the calving season are less likely to conceive in the subsequent mating period (Dennis et al. 2018). SS is most often used for first-service inseminations (DeJarnette et al. 2009; Healy et al. 2013) or early in the breeding season (Hutchinson et al. 2013). Therefore, MFERT could be an alternative to calving date for targeted allocation of SS. The aim of this study was, first, to compare the use of MFERT estimates to existing approaches for identifying most and least fertile cows within a herd; and second, to determine whether MFERT estimates can improve the allocation of dairy SS compared with existing approaches. The following three different fertility-ranking approaches were compared: (1) MFERT; (2) earliest to latest calving date; and (3) assignment via random number generator. Two breeding programs were explored: using SS and conventional dairy semen only or using SS, conventional dairy semen and beef semen. Other variables that were considered include a decrease in conception rates when SS was used, the proportion of cows that received each type of semen, the price of beef semen and price of dairy-beef calves.
Materials and methods
Data
Historical records including lactation and reproductive parameters were obtained from DataGene (https://www.datagene.com.au/) for 36 Australian commercial dairy herds located in Victoria, Tasmania and New South Wales from 2016 to 2019 inclusive. This is a 1 year extension on the data previously described in Ho and Pryce (2020). Milk MIR spectral data from first herd test after calving captured on NexGen Series FTS Combi machines (Bentley Instruments) was obtained directly from Hico (Maffra, Victoria, Australia), TasHerd (Hadspen, Tasmania, Australia) and DairyExpress (Armidale, New South Wales, Australia) for these herds. Artificial insemination and subsequent calving records were used to determine pregnancy status and calculate mating outcomes; conceived to first-service insemination, conceived to second, third, fourth or nth insemination, pregnant or not pregnant at the end of the mating season and number of semen straws used.
The likelihood of conceiving to first-service insemination (i.e. MFERT) was derived for each cow by using a prediction model developed using partial least squares discriminant analysis as described in Ho and Pryce (2020). Briefly, the model (Model 3 in Ho and Pryce (2020)) included milk spectra, age at calving, days in milk at herd test, and milk yield and somatic cell count. For model training, cows were classified as having ‘good’, ‘average’ or ‘poor’ fertility, which meant that they conceived to first insemination, conceived after two or more inseminations or did not conceive but were inseminated more than once, or did not conceive within the mating season and had only one insemination respectively. As training prediction models in a population of cows with ‘extreme’ phenotypes can improve accuracy (Ho et al. 2019), only good- and poor-fertility cows were used in the training population. This means that cows that did not conceive and had multiple inseminations were not used in model development. To independently obtain MFERT, a procedure similar to external validation was used. Specifically, as there were 61 herd-years of data, we predicted the probability of conception to first service of cows (ranging from 0 to 1) in each herd-year in turn by applying a model that was trained using data from the other 60 herd-years. The process continued until all 61 herd-years had been predicted.
A complete record for the purposes of this study included the following: MFERT, calving dates immediately before and after MFERT record, insemination records of subsequent mating period (insemination dates and number of inseminations) and mating outcomes (described above). The final dataset for this study had 13 379 complete records on 11 369 cows (some cows had records from multiple years). An initial analysis undertaken across 61 herd-year contemporary groups identified several herds operating split-calving systems. Data were manually subdivided into 76 herd-year–calving-season contemporary groups, hereafter referred to as cohorts. The average cohort size was 176.0 ± 13.4 cows, although it ranged from 32 to 517 cows. Overall average calving rate for the mating period was 65% ± 2%.
Fertility-ranking strategies
Three different strategies were used to rank cows within a cohort on the basis of the predicted fertility and then classify cows into high, medium and low expected-fertility subgroups. The proportion of cows within a cohort classified as high or low expected fertility was varied in 5% increments from 5% to 50%. In the MFERT strategy, a high and low subgroup from each cohort was selected on the basis of the highest and lowest MFERT respectively, with the balance of cows being classified into the medium subgroup. Similarly, in the calving-date strategy, hereafter referred to as CDATE, cows were classified on the basis of earliest (high-fertility subgroup) and latest (low-fertility subgroup) calving date, with the balanced being classified into the medium subgroup. In the random allocation strategy, hereafter referred to as RANDOM, cows within a cohort were assigned a dummy rank using a random number generator and then split into high-, medium- and low-fertility subgroups using this arbitrary ranking. Results presented for the random semen-allocation strategy are the average of 100 replicates for each cohort. The realised reproductive performance of each subgroup of cows was compared across the three ranking strategies.
Breeding programs
The three cow-ranking strategies described above were then used to overlay two breeding programs onto the historical MFERT, insemination and calving records for the 76 cohorts. For each breeding program, a cow’s assigned fertility subgroup (high, medium or low expected fertility) determined the type/s of semen she was allocated, as summarised in Table 1. In Breeding program 1 (BP1), all high-fertility cows receive SS at first-service insemination and conventional dairy semen for all other inseminations and all medium- and low-fertility cows receive conventional dairy semen only. The same occurs for high- and medium-fertility cows in Breeding program 2 (BP2), however, low-fertility cows receive conventional beef semen for all inseminations. In the baseline breeding program, all cows were mated to conventional dairy semen for all insemination events. Individual cow genetic merit was not considered in semen-allocation strategies.
Breeding program | Types of semen used | Semen allocation within fertility subgroup | |||
---|---|---|---|---|---|
High | Medium | Low | |||
Baseline | Conventional dairy only | Conventional dairy | Conventional dairy | Conventional dairy | |
BP1 | Sexed dairy and conventional dairy | Sexed dairy first AIA and conventional dairy remaining AI | Conventional dairy | Conventional dairy | |
BP2 | Sexed dairy, conventional dairy and beef | Sexed dairy first AIA and conventional dairy remaining AI | Conventional dairy | Beef |
AFirst artificial-insemination event.
Net-benefit calculations
The net benefit was calculated for each cow using calf values, semen straw costs and other associated mating costs plus an adjustment to account for changes in first-service conception rates in cows that received SS, further described below. All values are reported in Australian dollars. The value of different types of calf, costs of different semen straws and other mating costs ($19.36) are described in Table 2. The final model for net benefit ($/cow) for a cohort can be described as
where no. cows is the number of cows in a cohort, $calf is the value of calves born, $totsemen is the total semen costs, $totAIcost is the total cost of labour and materials associated with breeding, and $SSA is an adjustment to account for changes to first-service conception rates in cows that received SS. Each cow’s assigned fertility subgroup and pregnancy status at the end of mating period influenced $calf, $totsemen and $SSA. The value of $calf for cows in the high-fertility subgroup was also influenced by pregnancy status after first-service insemination and if a drop in SS first-service conception rates occurred. All calculations were conducted within a cohort, with across-cohort averages and standard errors reported here. For the random semen-allocation strategy, the average of 100 replicates was first calculated within each cohort and this average was used to calculate across-cohort averages.
Parameter | Value | |
---|---|---|
Sexed-semen purity (female calves) (%) | 0.93 | |
Conventional-semen price ($/straw) | 20 | |
Sexed-semen price ($/straw) | 50 | |
Calf from sexed-semen insemination ($/hd)A | 259.55 | |
Calf from conventional-semen insemination ($/hd)B | 164.67 | |
Cost of artificial-insemination event ($/insemination) | 19.36 | |
Cost of failed insemination with sexed semen ($/failed insemination)C | 143.09 | |
Proportion cows classified as high fertilityD | 5–50 | |
Proportion cows classified as low fertility | 5–50 | |
Decrease in conception rates with sexed semen (%) | 0, 5, 10 | |
Beef-semen price ($/straw) | 10, 15, 20 | |
Value of dairy-beef calves ($/head) | 100, 200, 250 |
AAssuming sexed-semen purity of 93%, dairy heifer calf price of $275 and male dairy calf price of $54.34.
BAssuming a 50:50 sex ratio of calves.
CCalculated assuming that a failed insemination delays calving by 21 days, affecting subsequent lactation performance, likelihood of surviving to the next lactation, and incurrs additional insemination and semen costs. Source: Byrne et al. (2016); DataGene (2020).
DThis changes the proportion of cows that receive SS at first-service insemination.
Decision trees were used to derive $calf, $totsemen, $totAIcost and $SSA for each cow. Decisions were derived from historical records for mating outcomes and total number of inseminations received and assigned fertility subgroup. Breeding program also influenced $calf and $totsemen. Table 3 shows all combinations of $calf, $totsemen and $totAIcost used. Fig. 1 provides an example decision tree, showing how $calf was assigned. For example, a cow classified as high fertility that conceived after two inseminations would have a $calf of $164.67 as she conceived at second service to conventional semen. Her $totsemen would be $70 = $50 + $20 × (2 − 1), $totAIcost $38.72 = $19.36 × 2 and $SSA = 0.
Fertility subgroup | $totsemenA | $totAIcostB | $SSAC | $calfD | |||||
---|---|---|---|---|---|---|---|---|---|
Preg first AI | Not Preg first AI | Preg first AI | Not Preg first AI | Preg first AI | Preg later AI | Not pregnant | |||
Baseline breeding program | |||||||||
All cows | $20.00 | $20.00 × n | $19.36 × n | NA | NA | $164.67 | $164.67 | $0 | |
Breeding program 1 | |||||||||
High | $50.00 | $50.00 + $20.00 (n − 1) | $19.36 × n | $SSA | $0 | $calfhigh | $164.67 | $0 | |
Medium | $20.00 | $20.00 × n | $19.36 × n | NA | NA | $164.67 | $164.67 | $0 | |
Low | $20.00 | $20.00 × n | $19.36 × n | NA | NA | $164.67 | $164.67 | $0 | |
Breeding program 2 | |||||||||
High | $50.00 | $50 + $20.00 (n − 1) | $19.36 × n | $SSA | NA | $calfhigh | $164.67 | $0 | |
Medium | $20.00 | $20.00 × n | $19.36 × n | NA | NA | $164.67 | $164.67 | $0 | |
Low | $semenBS | $semenBS × n | $19.36 × n | NA | NA | $calfBS | $calfBS | $0 |
Each cow’s values were allocated on the basis of fertility subgroup (high, medium or low), the total number (n) of artificial inseminations (AI) she received, whether she was pregnant after first artificial insemination (Preg first AI) or not (Not Preg first AI), or fell pregnant during the mating period (Preg later AI) or not.
A$semenBS cost of beef semen is varied as described in Table 2.
BCost of an individual mating is $19.36 as described in Table 2.
CSexed-semen adjustment, $SSA, values of $0, $7.15 and $14.31 were used for no change, a 5% and a 10% decrease in conception rate achieved to sexed semen.
D$calfhigh, the value of calves from matings to sexed semen is provided in Table 3 as it is affected by the conception rate achieved to sexed semen. $calfBS, the value of dairy-beef calves was varied, as described in Table 2.
Decision tree illustrating how pregnancy status at the end of mating period, fertility subgroup, conceiving to first artificial insemination (first AI), the decrease in first-service conception rate (CR_1) with sexed semen (SS) affects the calf value ($calf) each cow is assigned. Green, orange and blue shading aligns to high-, medium- and low-fertility subgroups. The value of a calf from a low-fertility subgroup is also determined by whether Breeding program 1 (BP1) or 2 (BP2) is being modelled and the assumed dairy-beef calf price.
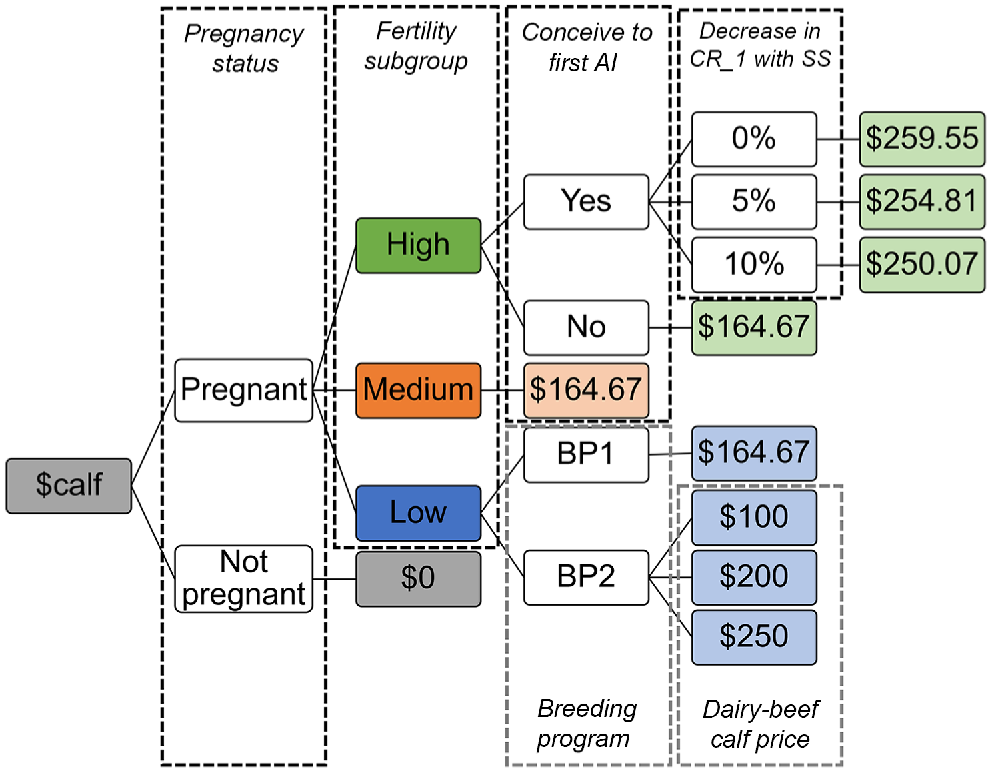
To account for the potential reduction in first-service conception rates when using SS, we modelled values representing first-service conception rates equivalent to, 5%, and 10% lower than each cohort’s reported first-service conception rates. The impact of a decrease in first-service conception rate from SS use is relevant only to the subgroup of high-fertility cows who conceived to first insemination. As this study uses historical records where insemination outcomes are already known, the cost of a decrease in first-service conception rate was applied proportionally to each cow in this subgroup, as SSdrop. For this subgroup of cows $calf, denoted as $calfhigh was therefore calculated as
where SSdrop is the reduction in first-service conception rate (0, 5% or 10%) and $calfSS and $calfCS are the values of calves born from SS and conventional semen, being $259.55 and $164.67 respectively (Table 2). The same proportional approach was used to calculate a SS adjustment, $SSA, as
where $SSpenalty ($143.09) is the estimated cost of a 21-day delay to conception (Table 2). This value incorporates the impact a 21-day to conception has on performance in the next lactation as well as additional mating and semen costs incurred due to a failed insemination in a cow that would have otherwise conceived at first service (Byrne et al. 2016; DataGene 2020). Calculated $SSA was $0, $7.15 and $14.31 for SSdrop of 0, 5% and 10% respectively. For all other cows, $SSA is not applicable ($0).
As well as comparing three semen-allocation strategies across two different breeding programs, key factors expected to influence results were varied. This included varying the proportion of cows within a cohort classified as high or low fertility from 5% to 50%. Therefore, the proportion of cows receiving SS at first insemination (BP1 and BP2) and beef semen (BP2 only) varied. SS conception rates equivalent to, 5% lower, and 10% lower than conventional dairy semen first-service conception rates were also considered. In BP2, three beef semen straw costs ($10, $15 and $20) and three dairy-beef calf prices ($100, $200, $250) were also considered (Table 2).
Different fertility-ranking approaches from the same cohort were treated as paired samples in statistical analyses. Preliminary analysis using a Shapiro–Wilk’s test and Q–Q plots found that the distribution of differences in paired samples was not normally distributed. Non-parametric testing was, therefore, conducted using the Wilcoxon signed rank test with continuity correction in R (R Development Core Team 2017). Differences in calving rate from first service insemination, overall calving rate and number of AIs among high-, medium- and low-fertility subgroups across the three fertility-ranking strategies were tested. From the breeding program modelling, differences in the number of dairy heifers, number of dairy-beef calves and net benefit were tested between each pairwise combination of MFERT, CDATE, and RANDOM.
Results
Historical reproductive performance
Calving rate from first service insemination, overall calving rate and number of artificial insemination events among cows allocated to high-, medium- and low-fertility subgroups using MFERT and CDATE are shown in Fig. 2a–c. Reproductive parameters of cows allocated to high-, medium- and low-fertility subgroups by using RANDOM did not differ from the cohort average. A single line representing cohort average is, therefore, shown in Fig. 2a–c, with the full results for RANDOM in Supplementary material Table S1.
Comparison of (a) calving rate from first artificial insemination, (b) overall calving rate, and (c) number of artificial-insemination events among high-, medium- and low-fertility subsets of cows, where subsets were defined on the basis of highest or lowest probability of conceiving to first service (MFERT) or earliest to latest calving date (CDATE). Overall cohort averages (average) are displayed as a black dotted line.
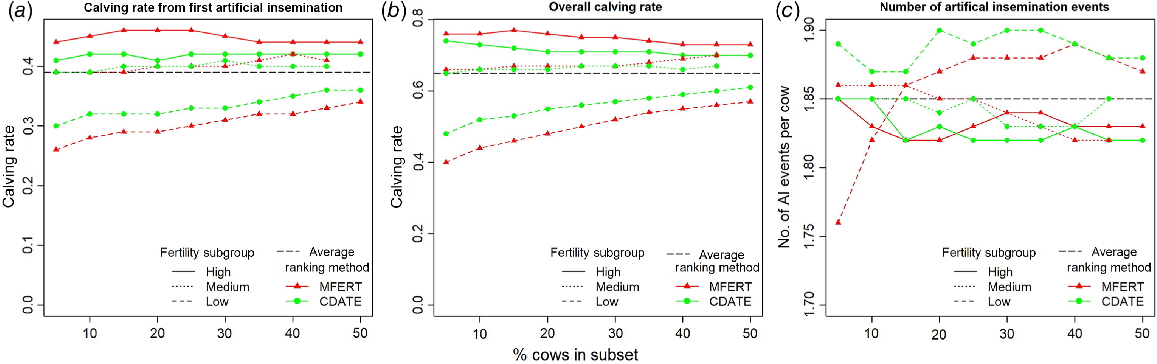
Calving rate from first insemination in cows classified as high fertility using MFERT (high-fertility MFERT cows) was between 4.8 and 6.9 percentage points higher than in cows classified as high fertility using RANDOM (high-fertility RANDOM cows). This difference was significant (P < 0.05), regardless of the proportion of cows in the subgroup (Table S1). Calving rate from first insemination in high-fertility MFERT cows was 2.4 to 5.7 percentage points higher than in high-fertility CDATE cows (Fig. 2a). This difference was significant (P < 0.05) only when comparing the 50% subgroup (Table S1). Calving rate from first insemination in low-fertility MFERT and low-fertility CDATE cows was significantly (P < 0.05) lower than that in low-fertility RANDOM cows for all subgroups tested. Calving rate to first insemination was between 2.4 and 5.3 percentage points lower, and 4.8 to 12.2 percentage points lower, in low-fertility CDATE and low-fertility MFERT cows respectively, than in low-fertility RANDOM cows. Calving rate to first insemination differed significantly only between low-fertility MFERT and low-fertility CDATE cows when 40% or more cows were assigned to the low-fertility subgroup.
Regardless of the proportion of cows in low-fertility subgroup, overall calving rate in low-fertility MFERT cows was significantly (P < 0.05) lower than in low-fertility RANDOM, or low-fertility CDATE cows (Fig. 2b, Table S1). Overall calving rate in low-fertility MFERT cows was between 3.9 and 11.94 percentage points lower, and 7.8–24.7 percentage points lower than in low-fertility CDATE cows and low-fertility RANDOM cows, respectively. Overall calving rate in high-fertility MFERT cows was significantly (P < 0.05) higher than that in high-fertility RANDOM cows, regardless of the proportion of the herd classified (Table S1). The same trend was observed comparing high-fertility MFERT and high-fertility CDATE cows. This difference was significant (P < 0.05) only when 15% or more of the cows were in the high-fertility subgroup. No significant differences were found in overall calving rate or calving rate from first insemination between medium-fertility MFERT and medium-fertility CDATE cows. Medium-fertility MFERT and medium-fertility CDATE cows had significantly (P < 0.05) higher overall calving rate and calving rate from first insemination than did medium-fertility RANDOM cows.
There was a trend for low-fertility CDATE cows to have more artificial inseminations than for other fertility subgroups or classification approaches (Fig. 2c). No statistical differences in the number of artificial insemination events were found. The only exception was that the bottom 5% of cows classified as low fertility by MFERT had significantly fewer (P < 0.05) artificial inseminations than did those classified as low RANDOM cows (Table S1).
Breeding program modelling results
In BP1, allocating SS for first insemination to cows with the highest MFERT or earliest calving date (CDATE) resulted in significantly (P < 0.05) more dairy heifer calves than did RANDOM (Table 4). This was seen regardless of whether a reduction in first-service conception rates occurred with SS. While a trend was seen for SS allocation by MFERT to result in more dairy heifers than SS allocation by CDATE, this difference was not statistically significant in BP1. In BP1, use of SS increased the number of heifer calves compared with using only conventional semen, regardless of semen-allocation strategy. When 50% of cows received SS at first service, up to 16.4, 15.9 and 14.9 additional heifer calves were expected (from average cohort of 176 cows) for MFERT, CDATE and RANDOM allocation strategies respectively, compared with using conventional dairy semen only.
% to sexed semen | Semen-allocation strategyA | |||
---|---|---|---|---|
RANDOM | MFERT | CDATE | ||
0 | 58.8 (5.2) | – | – | |
No change in conception rate with sexed semen | ||||
10 | 61.8 (5.4)a | 62.2 (5.4)b | 62.0 (5.4)b | |
20 | 64.7 (5.7)a | 65.5 (5.7)b | 65.2 (5.7)b | |
30 | 67.7 (5.9)a | 68.7 (6.0)b | 68.5 (6.0)b | |
40 | 70.7 (6.2)a | 71.8 (6.2)b | 71.5 (6.2)b | |
50 | 73.7 (6.4)a | 75.2 (6.5)b | 74.7 (6.5)b | |
5% decrease in conception rate with sexed semen | ||||
10 | 61.6 (5.4)a | 62.0 (5.4)b | 61.8 (5.4)b | |
20 | 64.4 (5.7)a | 65.1 (5.7) b | 64.8 (5.7)b | |
30 | 67.3 (5.9)a | 68.2 (5.9)b | 68.0 (5.9)b | |
40 | 70.1 (6.1)a | 71.2 (6.2)b | 70.9 (6.2)b | |
50 | 72.9 (6.4)a | 74.3 (6.5)b | 73.9 (6.4)b | |
10% decrease in conception rate with sexed semen | ||||
10 | 61.5 (5.4)a | 61.8 (5.4)b | 61.7 (5.4)b | |
20 | 64.1 (5.6)a | 64.8 (5.6)b | 64.5 (5.6)b | |
30 | 66.8 (5.9)a | 67.7 (5.9)b | 67.5 (5.9)b | |
40 | 69.5 (6.1)a | 70.5 (6.1)b | 70.3 (6.1)b | |
50 | 72.2 (6.3)a | 73.5 (6.4)b | 73.1 (6.3)b |
ADiffering letters within a row indicate that results are significantly (P < 0.05) different from one another in Wilcoxon signed-rank test.
In BP2, regardless of the proportion of the cohort was mated to SS and beef semen, allocation using MFERT always resulted in significantly (P < 0.05) more heifer calves and fewer dairy-beef calves than allocation using CDATE or RANDOM (Fig. 3, statistical data not reported). Up to 6.0 fewer dairy-beef calves and 4.5 more dairy heifer calves were produced in the MFERT scenarios than in RANDOM. The biggest difference comparing allocation via MFERT and CDATE was 2.1 fewer dairy-beef and 1.4 more dairy heifer calves.
Number of dairy heifers and dairy-beef calves in Breeding program 2 (targeted usage of sexed semen for first insemination and beef semen for all inseminations in a variable proportion of cows within a cohort with the balance of the cohort mated to conventional dairy semen) where three strategies were used for ranking cows to allocate semen, i.e. probability of conceiving to first service (MFERT), earliest to latest calving date (CDATE) or randomly (RANDOM), assuming (a) no change, (b) a 5% decrease or (c) a 10% decrease in sexed-semen conception rates relative to conventional semen conception rates. The averages across 76 cohorts with an average cohort size of 176 ± 13 cows are presented.
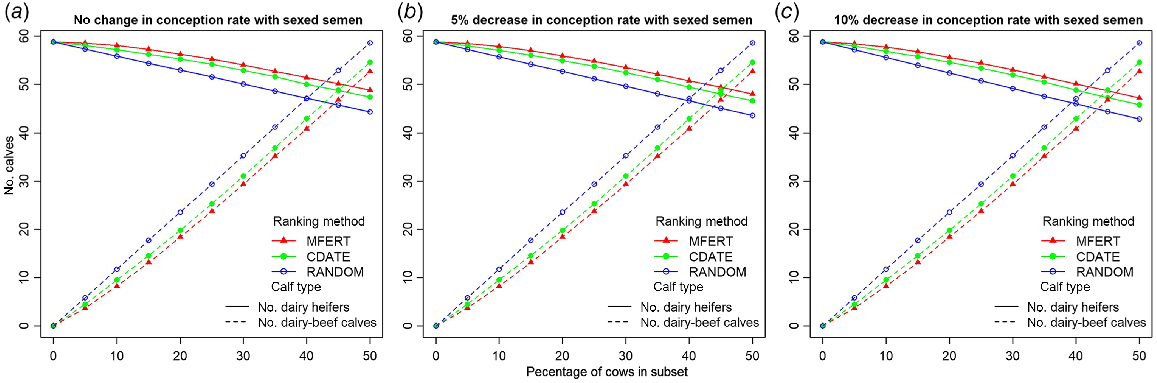
Although SS first-service conception rates influenced heifer calf numbers, they did not greatly influence the magnitude of differences observed among semen-allocation strategies in both BP1 and BP2. For example, in BP1, if 40% of cows were allocated SS at first insemination using MFERT, this resulted in 0.31, 0.30 or 0.28 more heifers than did using CDATE for 0%, 5% and 10% decrease in first-service conception rates respectively. Similarly, allocating SS using MFERT resulted in 1.49, 1.41 and 1.34 more heifers than did using RANDOM for SS first-service conception rates decreases of 0%, 5% and 10% respectively.
Allocating SS by MFERT had the highest net benefit ($/cow), followed by CDATE then RANDOM, regardless of SS first-service conception rates or the proportion of the herd mated to SS in BP1 (Fig. 4). The advantage of SS allocation by MFERT compared with RANDOM ranged from $0.20 to $2.29/cow and was significant (P < 0.05) for all subgroups tested (Table S2). Allocating SS by MFERT had a net benefit between $0.11 and $0.97/cow greater than did allocation by CDATE, although it was significant (P < 0.05) only when 45% or 50% of cows received SS at first service.
Net benefit ($/cow) in Breeding program 1 (using sexed semen at first service and conventional dairy semen for subsequent inseminations in a variable (5–50%) proportion of cows within a cohort, with the balance of the cohort mated to conventional dairy semen.) where three strategies were used for ranking cows to allocate semen, probability of conceiving to first service (MFERT), earliest to latest calving date (CDATE) or randomly (RANDOM), assuming (a) no change, (b) a 5% decrease or, (c) a 10% decrease in sexed-semen conception rates relative to conventional semen conception rates. A baseline scenario (Baseline) where all cows are mated to conventional semen is shown as a black dotted line.
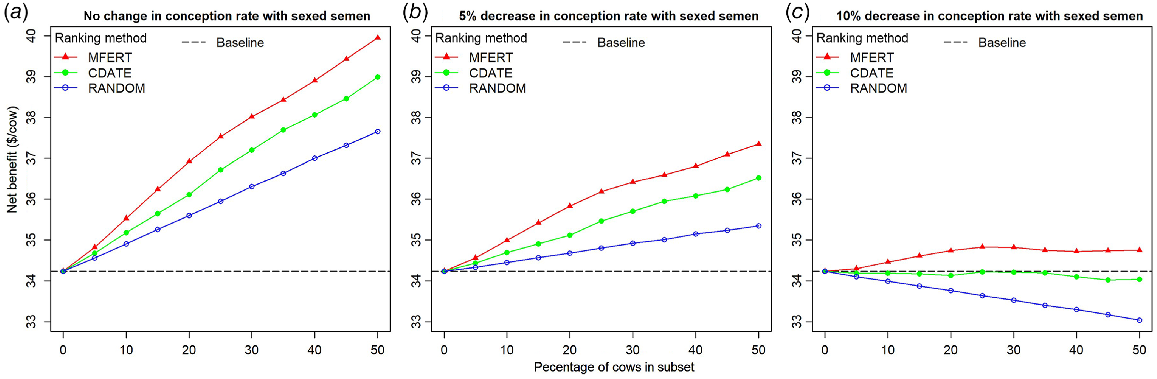
When SS first-service conception rate decrease was 0% or 5%, net benefit of BP1 was greater than net benefit of using conventional dairy semen only for all three semen-allocation strategies (Fig. 4a and b). When SS first-service conception rate decreased by 10%, MFERT was the only semen-allocation strategy where BP1 had a higher net benefit than using conventional dairy semen only (Fig. 4c). Here net benefit of semen allocation by MFERT ranged from $34.30 ± $3.59 to $34.75 ± $3.97 for MFERT strategies, compared with $34.24 ± $3.57 using conventional dairy semen only.
Not all variables tested in BP2 had large impacts on net benefit; therefore Figs 5, 6, 7 present a summary of results, while Tables S3–S5 contain all results, including statistical testing. Value of dairy-beef calves influenced which semen-allocation strategy had the highest net benefit in BP2 as well as whether the net benefit was greater than when using conventional dairy semen only (Figs 5–7). In BP2, when dairy-beef calf price was $100, the net benefit was highest when semen was allocated using MFERT, followed by CDATE, then RANDOM (Fig. 5). These differences were significant (P < 0.05) for all beef semen prices, variations to SS first-service conception rate or subgroups being considered (Table S3). The exception to this was the difference between MFERT and CDATE at a beef semen price of $10 and 30% subgroup. The net benefit was from $0.32 to $2.12/cow greater when comparing semen allocation by MFERT with that by CDATE, and between $0.96 and $4.94/cow higher when comparing MFERT with RANDOM (Fig. 5). However, net benefit from BP2 was usually lower than net benefit from using only conventional dairy semen when dairy-beef calf price was $100 (Fig. 5). The only time BP2 net benefit exceeded that from using conventional dairy semen only was when SS first-service conception-rate decrease was 0%, beef semen $10/straw and MFERT was used to select 25% or fewer cows for insemination to SS and beef semen (Fig. 5a). Under these circumstances, highest net benefit from allocation by MFERT was $34.53 ± 3.50 compared with $33.28 ± 3.50 and $31.71 ± 3.55 for CDATE and RANDOM respectively, and $34.24 ± 3.57 when using conventional dairy semen only.
Net benefit ($/cow) in Breeding program 2 (targeted usage of sexed semen for first insemination and beef semen for all inseminations in a variable proportion of cows within a cohort, with the balance of the cohort mated to conventional dairy semen), assuming dairy-beef calf values of $100 and beef semen costs ($semen) of (a, b) $10/straw or (c, d) $15/straw. Three strategies were used for ranking cows to allocate semen, the probability of conceiving to first service (MFERT), earliest to latest calving date (CDATE) or randomly (RANDOM), assuming (a, c) no decrease, or (b, d) a 10% decrease in sexed-semen conception rates relative to conventional semen conception rates. A baseline scenario (Baseline) where all cows are mated to conventional semen is shown as a black dotted line.
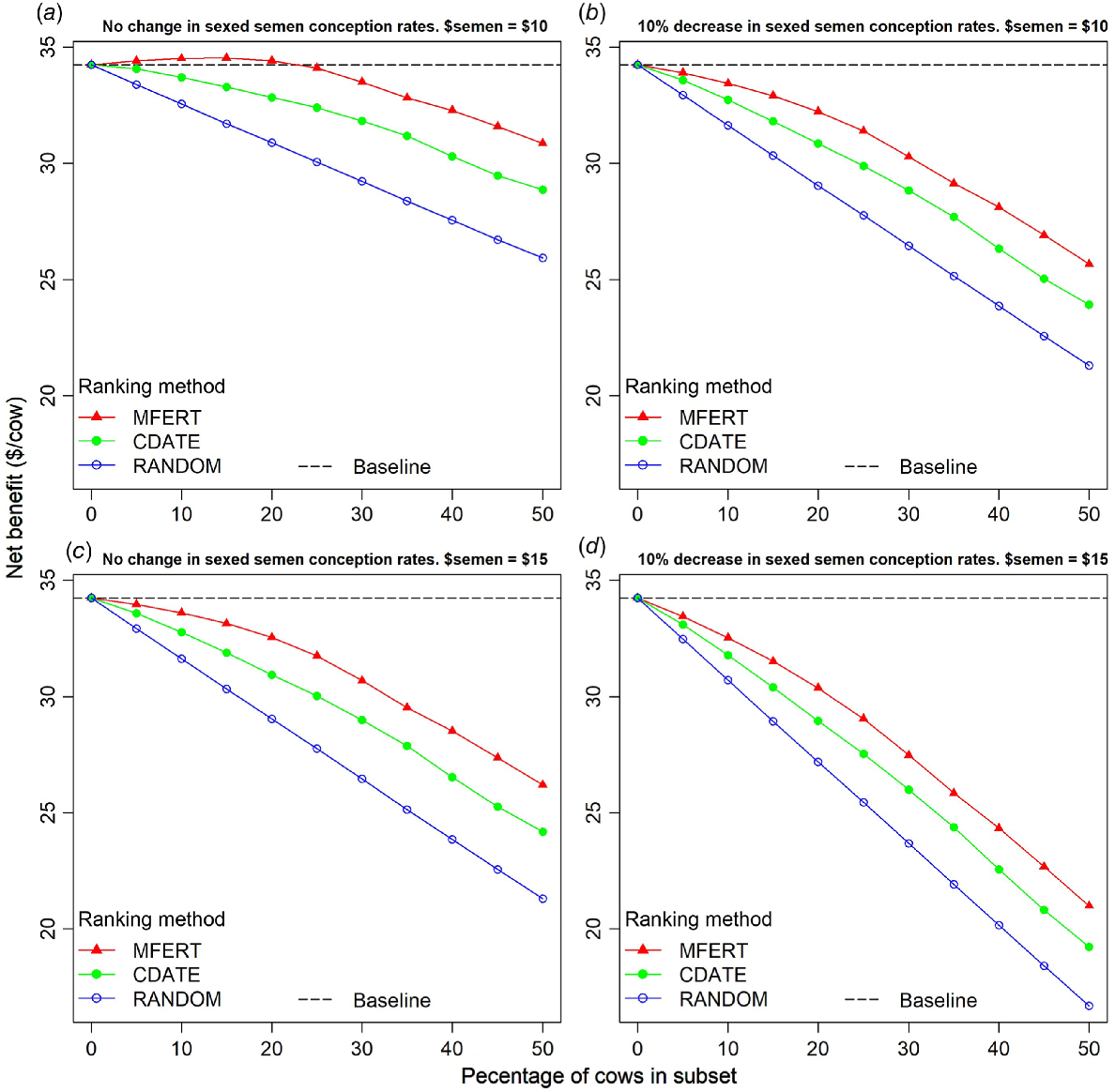
Net benefit ($/cow) in Breeding program 2 (targeted usage of sexed semen for first insemination and beef semen for all inseminations in a variable proportion of cows within a cohort, with the balance of the cohort mated to conventional dairy semen), assuming dairy-beef calf values of $200, beef semen costs ($semen) of (a, b) $10/straw or (c, d) $15/straw. Three strategies were used for ranking cows to allocate semen, the probability of conceiving to first service (MFERT), earliest to latest calving date (CDATE) or randomly (RANDOM), assuming (a, c) no decrease or (b, d) a 10% decrease in sexed-semen conception rates relative to conventional semen conception rates. A baseline scenario (Baseline) where all cows are mated to conventional semen is shown as a black dotted line.
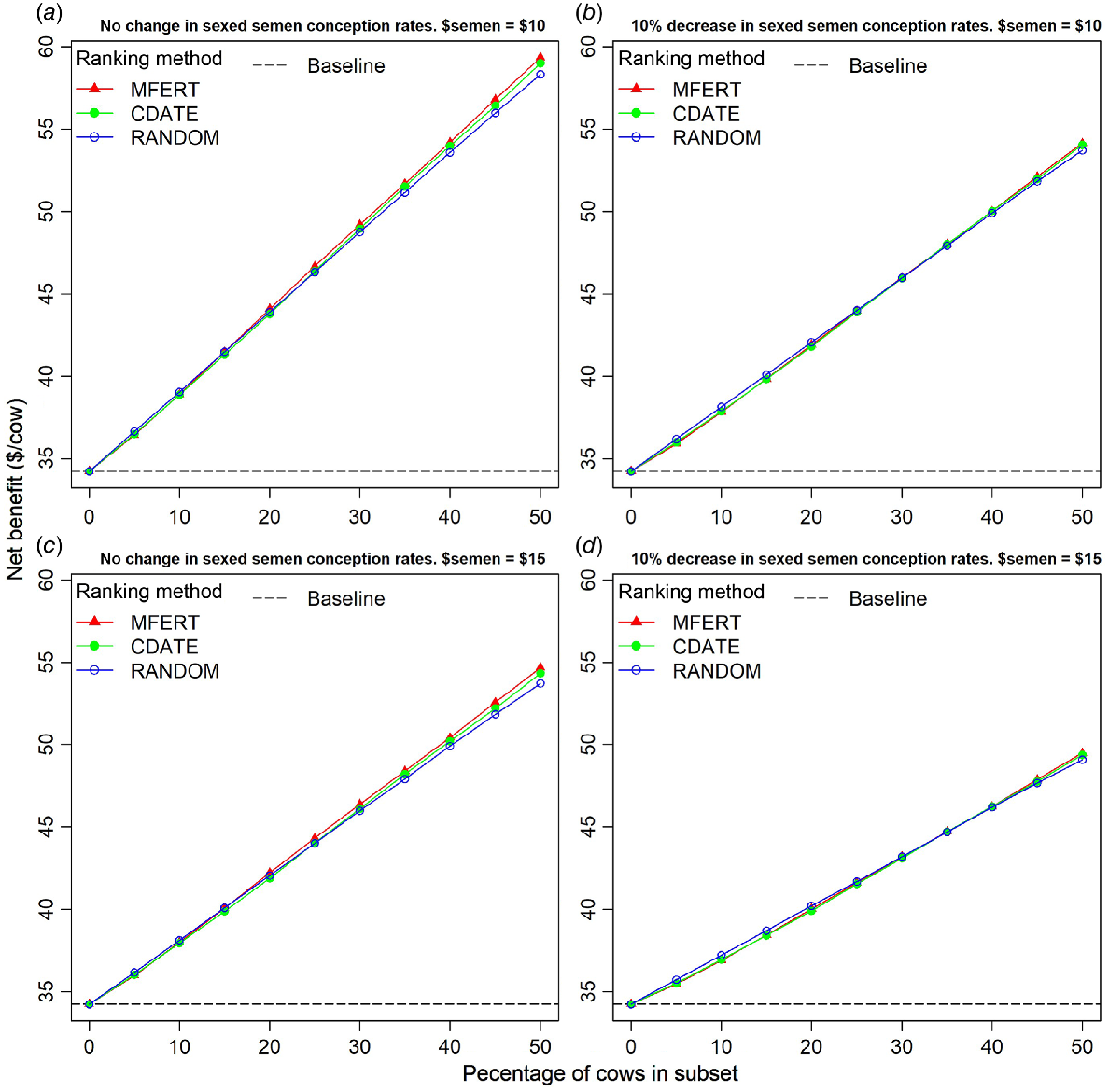
Net benefit ($/cow) in Breeding program 2 (targeted usage of sexed semen for first insemination and beef semen for all inseminations in a variable proportion of cows within a cohort, with the balance of the cohort mated to conventional dairy semen), assuming a dairy-beef calf value of $250 and beef semen ($semen) cost of $10/straw. Three strategies were used for ranking cows to allocate semen, the probability of conceiving to first service (MFERT), earliest to latest calving date (CDATE) or randomly (RANDOM), assuming (a) no decrease or (b) a 10% decrease in sexed-semen conception rates relative to conventional semen conception rates. A baseline scenario (Baseline) where all cows are mated to conventional semen is shown as a dotted line.
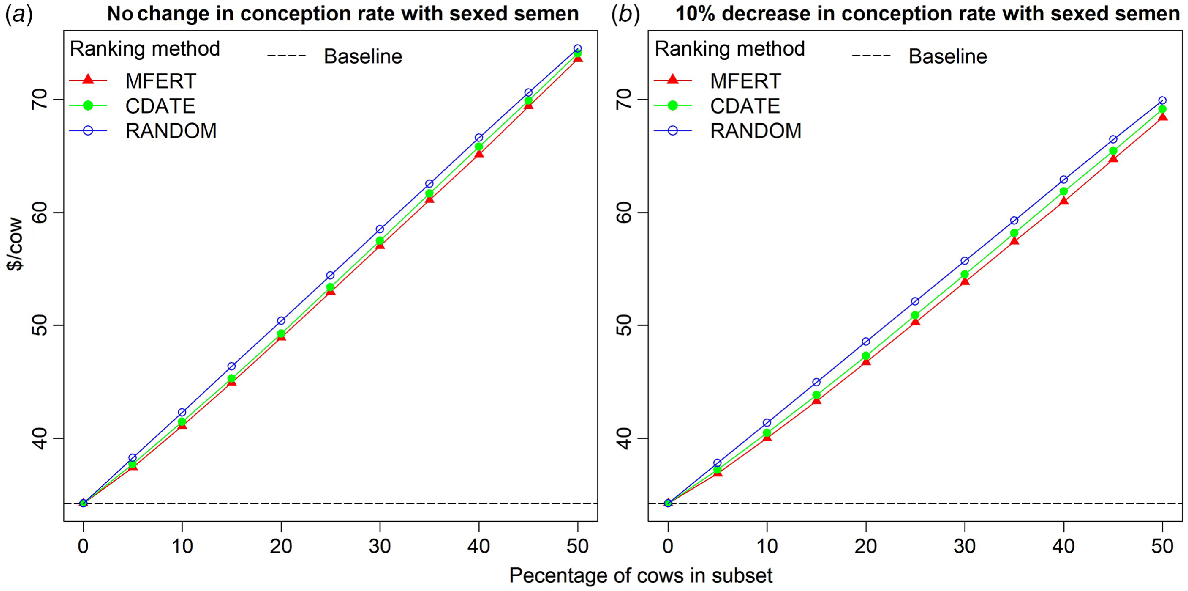
In BP2, when dairy-beef calf price was $200 or higher, net benefit was greater than when using conventional dairy semen only, across all three semen-allocation strategies (Figs 6, 7). When the dairy-beef calf price was $200, difference in net benefit among semen-allocation strategies was small and mostly non-significant, averaging $0.29/cow (Fig. 6, Table S4). The exception was when 50% of cohort was allocated SS at first insemination and the remaining 50% was allocated to beef semen. Here, net benefit of semen allocation by MFERT and CDATE was significantly (P < 0.05) greater than that by RANDOM, assuming no decrease, or 5% decrease in conception rate with SS (Table S4).
In contrast, when dairy-beef calf price was $250, RANDOM had significantly (P < 0.05) higher net benefit than did allocation by CDATE or MFERT for all three beef semen prices tested (Fig. 7, Table S5), a reversing of trends seen for all previous parameter combinations modelled. While MFERT had lower net benefit than did CDATE at a dairy-beef calf price of $250, this difference was significant only in a small number of cases (n = 6/45; Table S5). Again, observed differences were small; the net benefit was $0.22–$0.90/cow less comparing allocation by MFERT to CDATE, and between $0.81 and $2.10/cow less comparing allocation by MFERT to RANDOM. For all calf prices used, beef semen prices had a minimal effect on comparisons of net benefit across the different semen-allocation approaches.
Discussion
As SS is often more expensive than conventional semen, prioritising more fertile cows to mate to SS is of interest to farmers. Our study has shown that both the likelihood of conceiving to first service insemination (MFERT) and calving date (CDATE) outperformed a random number generator (RANDOM) in identifying subgroups of cows most and least likely to produce a calf from first service insemination. Further, MFERT outperformed both CDATE and RANDOM in identifying subgroups of cows most and least likely to produce a calf overall. Differences in calving rates among the three fertility-ranking strategies were greater in the low-fertility subgroups. When historical mating records were used to compare semen allocation using MFERT, CDATE or RANDOM for two different breeding programs incorporating the use of SS, the results generally favoured MFERT. Using MFERT and CDATE to identify high-fertility cows for first-service insemination to SS (BP1) resulted in more dairy heifers than did using RANDOM. When considering allocation of SS, conventional dairy semen and beef semen (BP2), using MFERT to allocate the highest-fertility cows to SS resulted in more dairy heifer calves than did allocating semen by RANDOM or CDATE. Conversely, when cows with lower fertility were assigned beef semen, semen allocation by MFERT had fewer dairy-beef calves than did allocation by CDATE or RANDOM. This was true regardless of the proportion of the cohort mated to SS and beef semen. These differences in calf numbers translated to small differences in net benefit between the three semen-allocation approaches. By offering an additional use for data routinely captured during herd recording, MFERT can add value to data routinely captured during herd recording. While MFERT can be used to prioritise the allocation of SS, the value proposition is sensitive to individual farm parameters.
Using MFERT to identify high- and low-fertility cows
MFERT was able to identify cows more likely to calve overall compared to both RANDOM and CDATE. MFERT was also able to identify cows more likely to calve after first service compared to RANDOM. However, MFERT arguably did a better job at identifying cows least likely to calve after first service insemination or overall. This is illustrated by the difference in calving rate from first insemination between cows classified using MFERT and those classified using RANDOM. When MFERT was used to identify high-fertility cows, calving rate from first service was 4.6–6.9 percentage points greater than that from using RANDOM. Whereas when MFERT was used to identify low-fertility cows, calving rate from first service was 4.8–12.2 percentage points lower than that from using RANDOM. This is supported by Ho et al. (2019) who reported a higher prediction accuracy in low-fertility cows. This also aligns with Lou et al. (2022) who trained a similar model to the one used in our study to predict the likelihood of conception to first insemination in a population of 5738 Chinese Holstein cows. Lou et al. (2022) reported that their model was better able to identify cows with a lower probability of conception, as evidenced by a higher specificity than sensitivity.
Impact of targeted semen allocation on calf numbers
Breeding program and fertility-ranking approach affected the number of dairy heifer calves and dairy-beef calves. Using MFERT to identify high-fertility cows for first-service insemination with SS resulted in more dairy heifer calves than did using RANDOM to identify high-fertility cows in both BP1 and BP2. The average maximum increase of 4.5 heifer calves/per 176 cows is numerically small. However, considerable variation existed among cohorts. An additional 19.4 heifer calves were observed in one of the larger cohorts (no. cows = 454) in this study when comparing semen allocation by MFERT and RANDOM in BP2. The same number of straws of SS is used to mate a given proportion of the herd (high-fertility cows) to SS. This means that the observed increase in heifer numbers is due to changing which cows receive SS, and for BP2 conventional semen, not the amount of SS used. When cows with the lowest fertility were assigned beef semen, semen allocation by MFERT had significantly fewer dairy -beef calves (up to 6.0 fewer calves), than did allocation by CDATE or RANDOM. The lower number of dairy-beef calves found in MFERT scenarios is not unexpected, given the bigger differences in reproductive performance of low-fertility cows. A flow-on effect of more effective identification of low-fertility cows for insemination with beef semen is that semen allocation by MFERT also resulted in significantly more dairy heifers than using CDATE did in BP2.
Net benefit of targeted semen allocation
Differences in dairy heifer and dairy-beef calf numbers translated to differences in net benefit among the three semen allocation approaches in BP1 and BP2. Generally, this reflected favourably on MFERT, followed by CDATE then RANDOM. Net benefit differences between semen allocation by MFERT and CDATE were significant only for a limited range of parameters tested, for example, at a dairy-beef calf price of $100. The additional net benefit from semen allocation by MFERT decreased as dairy-beef calf prices increased, becoming negligible at dairy-beef calf prices of $200/head and negative at dairy-beef calf prices of $250/head. Although allocation by MFERT resulted in more heifer calves, the increased the value of dairy-beef calves was advantageous to CDATE and RANDOM strategies, which produced more dairy-beef calves. The financial advantage of using MFERT to identify more fertile cows for insemination with SS was effectively cancelled out by the fewer number of dairy-beef calves when they had a high value. Overall, the two breeding programs considered in this study had a higher net benefit than did using conventional dairy semen only. The exceptions to this were (a) in BP1 when SS first-service conception rate decreased by 10% and semen was allocated by RANDOM and by CDATE and (b) for majority of scenarios where dairy-beef calf price was $100/head. In a stochastic simulation study, which included a comparison of targeted allocation of SS to cows with a high predicted chance of successful conception to random allocation of SS, Ruelle et al. (2021) reported differences in farm profit (targeted minus random allocation) ranging from −$4.30 to $8.96 per cow. Scenarios where decreases in farm profit occurred with targeted allocated of SS were attributable to increased usage of SS in heifers, whereas our study considered only cows. In addition to considering heifers and not using MIR milk spectral data in the identification of high-fertility cows, another key difference is that Ruelle et al. (2021) set a target number of dairy female replacements. Despite these differences, the two studies are broadly in agreement that targeted allocated of SS to higher-fertility cows can add additional value to use of SS.
Although the use of milk MIR spectral data for fertility prediction is novel, other reproduction and mating decision support tools have previously been developed. These have focused on other aspects of breeding program design including, genetic gain (e.g. Gaynor et al. 2020), reducing inbreeding (e.g. Carthy et al. 2019), return on investment from genomic testing, and economic benefits of improving reproductive performance (e.g. Cabrera 2018). Previously Hempstalk et al. (2015) and Shahinfar et al. (2014, 2015) developed models to predict the probability of conceiving at a particular insemination. Using model inputs such as production, health and reproduction data and breeding values, Shahinfar et al. (2015) estimated a profit increase of between $0.44/cow and $5.21/cow when applying their model to support insemination decisions. The additional gain per cow, from making insemination decisions based on probability of conception, was similar to that in our study where the additional net benefit from using MFERT ranged from $0.11/cow to $4.94/cow. This may partly be attributed to the similar prediction accuracies between the two models (~0.76). However, the model of Shahinfar et al. (2015) uses health-data inputs that are not readily available on Australian dairy farms such as incidences of ketosis, lameness and retained placenta. There may, in fact, be additional benefits of combining MFERT with health data and this could be explored in countries where both these data exist. As the pool of MIR spectral data in Australia grows, we may expect additional increases in the accuracy of our model.
Reducing economic impact of low conception rates with SS usage
Historically, one of the barriers to adoption of SS is lower conception rates than with conventional dairy semen (Butler et al. 2014). This is in part because any decrease in reproductive performance has flow-on effects on farm profitability (Shalloo et al. 2014), reducing the benefits that the SS offers (Holden and Butler 2018). Therefore, SS is often recommended to be targeted for use on the most fertile cows (Butler et al. 2014). Doing so reduces the risk of poorer conception rates and subsequent economic impacts. Using MFERT or calving date to prioritise more fertile cows for insemination with SS at first insemination helps mitigate economic loses that arise if poorer SS conception rates occur. In our study, as anticipated, net benefit decreased when SS first-service conception rate decreased, regardless of semen-allocation strategy, or the combination of semen prices and calf values used. However, in BP1 when a 10% decrease in SS first-service conception rate was assumed, MFERT was the only semen-allocation approach that yielded a higher net benefit than did using conventional dairy semen only. This indicates the superior ability of MFERT to identify more fertile cows within a contemporary group, which could help counter the economic effects of poorer first-service conception rate with SS. However, some caution should be taken with this inference as the value of SS allocation by MFERT has not been tested in a whole-farm model. Another strategy for reducing the impact of low conception rates with SS is to use synchronisation (Ruelle et al. 2021); however, as our study used historical records, this approach could not be tried.
Limitations and justifications
In our study, differences in calf numbers and net benefit between SS-allocation approaches were small overall (maximum $4.94/cow), with standard errors of a magnitude similar to observed differences. The use of a net-benefit calculation with simple inputs means that all potential impacts on farm margins may not be accounted for. For example, it has been shown that the genetic merit of a beef sire mated to a dairy cow can adversely affect her milk production in the subsequent lactation, with this production loss potentially outweighing the financial benefit of a dairy-beef calf (Berry and Ring 2020). Our study used a flat price for each calf type. However, a dairy heifer born earlier in the calving season (i.e. from a first-service insemination) is arguably more valuable than one born later in the calving period. These animals have more time to reach target mating weights than later-born calves (Izzo 2015). Given the higher calving rate to first service of high-MFERT cows, allocation of SS by MFERT could also help concentrate the calving of heifer replacements in the beginning of the calving season. There are also potential management and animal-health benefits during calf rearing from having a tighter calving pattern (Izzo 2015). Given the large impact of dairy-beef calf prices on net benefit reported in this study, further sensitivity analyses of dairy heifer calf prices may be warranted. If the heifer calf value is greater than the $275 used here, net benefit differences could be greater than reported here.
In this study, we only varied the price of beef semen, not conventional semen or SS; however, this is unlikely to affect conclusions drawn from comparison of semen-allocation strategies. Although changing beef-semen price affected overall net benefit, it had minimal impact on the magnitude of differences in net benefit across semen-allocation strategies. We would expect a similar trend if conventional dairy-semen or SS prices were varied. This is because few differences in the average number of inseminations were seen amongst subsets of cows. Similar average number of total inseminations across subherds could be influenced by the definitions used in the MFERT prediction model. A poor-fertility cow was one who did not conceive within the mating season and had only one insemination (Ho and Pryce 2020). Also, the MFERT prediction developed by Ho et al. (2019) uses calving records instead of pregnancy records to determine successful conception. Therefore, cows that fell pregnant to first service and then aborted would be classified as poor fertility. When sufficient data exist, it could be helpful to examine abortion rates in high- or low-MFERT predicted fertility cows. While use of pregnancy-scanning records to record insemination outcomes is desirable and may lift model accuracy, sufficient data are not available in Australia.
We acknowledge that a systems approach is sometimes recommended for valuing tools (i.e. Cabrera 2018); however, such approaches require detailed data inputs, which are not always available, especially across diverse dairy farming systems as seen in Australia. The reporting of key reproductive parameters and the simple net-benefit approach used here allowed the calculations to be easily applied to a historical dataset of 76 herd–year–calving season cohorts from 36 farms across eastern Australia. The cohort size in this study varied from 32 to ~500 cows, illustrating that MFERT has applicability on a range of herd sizes. The need for a local reference population means that the model used to generate MFERT values here cannot be directly applied into international cow populations. The same principles could be used to develop local MFERT values, as has been undertaken in China (Lou et al. 2022).
On-farm application of MFERT
Over 100 years ago, herd recording revolutionised individual management of lactating cows, and still represents a good return on investment to most dairy businesses (Newton et al. 2020). Prediction of fertility is one of many potential applications of MIR data currently being explored (De Marchi et al. 2014). As MIR data can be captured during routine herd testing, each novel application of MIR milk spectral data does not need to offer large returns. Small returns from multiple novel applications collectively could add considerable value to herd test data. For an average Australian herd (no. cows = 300), additional benefit from using MFERT is expected to be ~$300–600/herd.year. The value proposition for using MFERT will be more attractive in larger herds. Although the value of using MFERT for targeted semen allocation was small in this study, improving fertility remains a priority for many dairy farmers (Martin-Collado et al. 2015). SS is recommended for use in well managed herds with high reproductive performance and established artificial insemination programs (Holden and Butler 2018; Walsh et al. 2021). These herds are likely to have already realised majority of economic benefits from improving reproductive efficiency in other areas. Tools supporting further incremental increases (i.e. $1–2/cow) in reproductive efficiency may gain traction with this cohort of farmers. Also, non-monetary considerations such as convenience, compatibility with existing systems, changed labour requirements and complexity also affect farmer uptake of precision dairy tools (Stone 2020). Due to the higher cost of SS and history of reduced conception rates, a tool perceived to reduce risks associated with SS usage may also be attractive.
Balancing decision making for short-term and long-term gains in breeding programs is another consideration in the adoption of MFERT on farm. This paper has focused on the short-term, with a goal of inseminating cows most likely to conceive to first-service insemination with SS within a single mating period. Over a longer timeframe, this may not be the most profitable breeding strategy. Increased herd genetic gain is one of the widely reported benefits of SS (Holden and Butler 2018). This can be achieved by increasing the number of heifer-selection candidates (as was undertaken in BP1) or through more efficiently producing higher-genetic merit herd replacements by identifying high genetic-merit cows for insemination with SS (Holden and Butler 2018). The economic benefits of the later strategy are realised over a longer timeframe. However, high genetic-merit cows may not always be the most likely to conceive at first-service insemination or have the highest MFERT values. It has previously been shown that including the daughter fertility genomic breeding value in the MFERT prediction model used here improves prediction accuracy by 2–3% (Ho et al. 2019). As fertility traits have low heritability (Berry et al. 2014), and management has a large influence on cow fertility, the modest increase in model accuracy is not unexpected. Although MFERT could be used as a standalone tool to prioritise allocation of high-value semen, a tool that reports MFERT alongside other fertility variables influencing reproductive success, such as calving dates and fertility breeding values, and other information used in breeding decisions, is likely to provide better value to farmers. Scope exists to further consider how a short-term decision support tool such as MFERT could be integrated with genetic information to better support longer-term-data driven breeding program design and the likely economic benefits of doing so. Given the ability of MFERT to identify cows least likely to conceive during the mating period, further exploration of this value proposition is warranted. As MFERT predictions are, in principle, available weeks before artificial insemination starts, management interventions may be possible.
Conclusions
Predicting the fertility of dairy cows from MIR milk spectral data adds value to herd test data. MFERT outperformed CDATE and RANDOM in identifying cows most and least likely to produce a calf overall. MFERT and CDATE significantly outperformed RANDOM in identifying cows most and least likely to produce a calf from first-service insemination. Targeted allocation of SS for first-service insemination to more fertile cows using MFERT or CDATE can increase the number of heifer calves born per straw of SS used, supporting more efficient SS usage than random allocation of semen. In the scenarios modelled in this study, the net benefit of using MFERT over CDATE or RANDOM for semen allocation was small, but generally favoured MFERT unless dairy-beef calf prices were comparatively expensive. MFERT therefore adds value to data captured during herd recording. A tool combining MFERT with other measures of cow fertility, including calving date, and genetic information, would facilitate more data-driven reproductive management decisions and breeding program design. Other applications of MFERT should also be considered.
Acknowledgements
The authors thank DataGene (Melbourne, Australia) for providing the data used in this study. TasHerd Pty Ltd (Hadspen, Tasmania, Australia), Hico Pty Ltd (Maffra, Victoria, Australia), and DairyExpress (Armidale, New South Wales, Australia) are gratefully acknowledged for collecting milk samples and providing MIR spectral data as are the dairy farmers who participated in this study. The authors also acknowledge Irene Van den Berg (Agriculture Victoria, Melbourne, Australia) for providing feedback on a draft manuscript.
References
Berry DP, Ring SC (2020) Short communication: the beef merit of the sire mated to a dairy female affects her subsequent performance. Journal of Dairy Science 103, 8241-8250.
| Crossref | Google Scholar |
Berry DP, Wall E, Pryce JE (2014) Genetics and genomics of reproductive performance in dairy and beef cattle. Animal 8, 105-121.
| Crossref | Google Scholar |
Butler ST, Hutchinson IA, Cromie AR, Shalloo L (2014) Applications and cost benefits of sexed semen in pasture-based dairy production systems. Animal 8, 165-172.
| Crossref | Google Scholar |
Byrne TJ, Santos BFS, Amer PR, Martin-Collado D, Pryce JE, Axford M (2016) New breeding objectives and selection indices for the Australian dairy industry. Journal of Dairy Science 99, 8146-8167.
| Crossref | Google Scholar |
Cabrera VE (2018) Invited review: Helping dairy farmers to improve economic performance utilizing data-driving decision support tools. Animal 12, 134-144.
| Crossref | Google Scholar |
Carthy TR, McCarthy J, Berry DP (2019) A mating advice system in dairy cattle incorporating genomic information. Journal of Dairy Science 102, 8210-8220.
| Crossref | Google Scholar |
De Marchi M, Toffanin V, Cassandro M, Penasa M (2014) Invited review: mid-infrared spectroscopy as phenotyping tool for milk traits. Journal of Dairy Science 97, 1171-1186.
| Crossref | Google Scholar |
DeJarnette JM, Nebel RL, Marshall CE (2009) Evaluating the success of sex-sorted semen in US dairy herds from on farm records. Theriogenology 71, 49-58.
| Crossref | Google Scholar |
Dennis NA, Stachowicz K, Visser B, Hely FS, Berg DK, Friggens NC, Amer PR, Meier S, Burke CR (2018) Combining genetic and physiological data to identify predictors of lifetime reproductive success and the effect of selection on these predictors on underlying fertility traits. Journal of Dairy Science 101, 3176-3192.
| Crossref | Google Scholar |
Genetics Australia (2021) Catalogues: 2021 April Dairy Sire Catalogue. Available at https://genaust.com.au/resource/catalogues [Accessed 14 July 2021]
Gaynor RC, Gorjanc G, Hickey JM (2020) AlphaSimR: an R package for breeding program simulations. G3 Genes|Genomes|Genetics [2] 11 1-5.
| Crossref | Google Scholar |
Grelet C, Dardenne P, Soyeurt H, Fernandez JA, Vanlierde A, Stevens F, Gengler N, Dehareng F (2021) Large-scale phenotyping in dairy sector using milk MIR spectra: key factors affecting the quality of predictions. Methods 186, 97-111.
| Crossref | Google Scholar |
Healy AA, House JK, Thomson PC (2013) Artificial insemination field data on the use of sexed and conventional semen in nulliparous Holstein heifers. Journal of Dairy Science 96, 1905-1914.
| Crossref | Google Scholar |
Hempstalk K, McParland S, Berry DP (2015) Machine learning algorithms for the prediction of conception success to a given insemination in lactating dairy cows. Journal of Dairy Science 98, 5262-5273.
| Crossref | Google Scholar |
Ho PN, Pryce JE (2020) Predicting the likelihood of conception to first insemination of dairy cows using milk mid-infrared spectroscopy. Journal of Dairy Science 103, 11535-11544.
| Crossref | Google Scholar |
Ho PN, Bonfatti V, Luke TDW, Pryce JE (2019) Classifying the fertility of dairy cows using milk mid-infrared spectroscopy. Journal of Dairy Science 102, 10460-10470.
| Crossref | Google Scholar |
Holden SA, Butler ST (2018) Review: Applications and benefits of sexed semen in dairy and beef herds. Animal 12, s97-s103.
| Crossref | Google Scholar |
Hutchinson IA, Shalloo L, Butler ST (2013) Expanding the dairy herd in pasture-based systems: the role of sexed semen use in virgin heifers and lactating cows. Journal of Dairy Science 96, 6742-6752.
| Crossref | Google Scholar |
Lou W, Shi R, Ducro B, van der Linden A, Mulder HA, Oosting SJ, Liu L, Wang Y (2022) Classifying the likelihood of conception in dairy cow with milk mid-infrared spectra before the first insemination. In ‘World Congress on Genetics Applied to Livestock Production, Rotterdam, Netherlands, 3–8 July 2022.’ (Wageningen University & Research) Available at https://www.wageningenacademic.com/pb-assets/wagen/WCGALP2022/04_002.pdf
Martin-Collado D, Byrne TJ, Amer PR, Santos BFS, Axford M, Pryce JE (2015) Analyzing the heterogeneity of farmers’ preferences for improvements in dairy cow traits using farmer typologies. Journal of Dairy Science 98, 4148-4161.
| Crossref | Google Scholar |
Newton JE, Nettle R, Pryce JE (2020) Farming smarter with big data: Insights from the case of Australia’s national dairy herd milk recording scheme. Agricultural Systems 181, 102811.
| Crossref | Google Scholar |
Newton JE, Ho PN, Pryce JE (2021) Using mid-infrared spectroscopy predictions of fertility to optimise semen allocation in dairy herds. Proceedings of the Association for the Advancement of Animal Breeding and Genetics 24, 263-266 Available at http://www.aaabg.org/aaabghome/AAABG24papers/66Newton24263.pdf.
| Google Scholar |
Noonan EJ, Kelly JC, Beggs DS (2016) Factors associated with fertility of nulliparous dairy heifers following a 10-day fixed-time artificial insemination program with sex-sorted and conventional semen. Australian Veterinary Journal 94, 145-148.
| Crossref | Google Scholar |
Ruelle E, Shalloo L, Butler ST (2021) Economic impact of different strategies to use sex-sorted sperm for reproductive management in seasonal-calving, pasture-based dairy herds. Journal of Dairy Science 104, 11747-11758.
| Crossref | Google Scholar |
Shahinfar S, Page D, Guenther J, Cabrera V, Fricke P, Weigel K (2014) Prediction of insemination outcomes in Holstein dairycattle using alternative machine learning algorithms. Journal of Dairy Science 97, 731-742.
| Crossref | Google Scholar |
Shahinfar S, Guenther JN, David Page D, Kalantari AS, Cabrera VE, Fricke PM, Weigel KA (2015) Optimization of reproductive management programs using lift chart analysis and cost-sensitive evaluation of classification errors. Journal of Dairy Science 98, 3717-3728.
| Crossref | Google Scholar |
Shalloo L, Cromie A, McHugh N (2014) Effect of fertility on the economics of pasture-based dairy systems. Animal 8, 222-231.
| Crossref | Google Scholar |
Stone AE (2020) Symposium review: The most important factors affecting adoption of precision dairy monitoring technologies. Journal of Dairy Science 103, 5740-5745.
| Crossref | Google Scholar |
Walsh DP, Fahey AG, Mulligan FJ, Wallace M (2021) Effects of herd fertility on the economics of sexed semen in a high-producing, pasture-based dairy production system. Journal of Dairy Science 104, 3181-3196.
| Crossref | Google Scholar |