Impact of heat stress on dairy cow rumination, milking frequency, milk yield and quality in a pasture-based automatic milking system
S. Talukder



A School of Agriculture, Food and Ecosystem Sciences, Faculty of Science, The University of Melbourne, Parkville, Vic. 3010, Australia.
B Sydney Institute of Agriculture, Sydney School of Veterinary Science, The University of Sydney, Camden, NSW 2570, Australia.
C School of Agriculture, Food and Ecosystem Sciences, Faculty of Science, The University of Melbourne, Dookie College, Vic. 3647, Australia.
Abstract
Heat stress is an increasing concern for the Australian dairy industry.
This study aimed to evaluate the effect of temperature–humidity index (THI) on rumination time (RT), milk yield and quality, and milking frequency in a pasture-based voluntary-movement automatic milking system (AMS).
Data were collected from the University of Melbourne Dookie College AMS farm for 3 years (June 2016 to March 2019). Daily RT was collected through the transponder collar (Qwes-HR, Lely). Climatic data (maximum ambient temperature and relative humidity) were obtained from the Dookie Meteorological station to calculate daily maximum THI (THImax).
Daily milk yield increased with a rising THImax to 65, then declined after THImax 65. Milking frequency was highest at THImax 90, followed by a steady decline afterwards. Rumination time was maximum at mid-range THImax and declined for high and low values.
The findings of this study clearly indicated that under pasture-based voluntary-movement AMS, high THI resulted in a drop in the milk yield, milking frequency and RT.
With the provision of automation of data collection from AMS, further study with mathematical modelling describing the daily patterns and thresholds in conjunction with the different heat stress levels can be useful for assessing animal welfare and to mitigate heat stress and seek alternative management strategies.
Keywords: automatic milking systems, heat stress, milk quality, milking frequency, pasture, rumination, somatic cell count, temperature humidity index.
Introduction
The dairy industry will be increasingly affected by heat exposure due to climate change, which causes a very hot summer season with slight cooling during night hours (Nidumolu et al. 2014; Müschner-Siemens et al. 2020). In addition, pasture-based dairy systems in Australia, particularly in the southern part, which has a Mediterranean climate, will likely face increasing challenges as the number of hot days and duration of heat events are predicted to increase (Horton et al. 2016; Hendricks et al. 2022). It is projected that Australia will experience annually an additional 31–42 heat-stress days per year by 2050 (Nidumolu et al. 2014). Besides rising environmental temperatures, the selection of cows for the improved genetic potential for milk yield makes dairy cows less tolerant to heat stress (Osei-Amponsah et al. 2020). The combination of internal heat load and environmental conditions can prevent heat dissipation, creating a state of metabolic heat stress (Maia et al. 2020). Heat stress in dairy cows causes a drop in milk yield (Ouellet et al. 2019; Herbut et al. 2021) and milk composition (protein and fat; Bouraoui et al. 2002), rumination (Soriani et al. 2013), reproductive signals (Hansen 2007), and an increase in somatic cell count (SCC; Negri et al. 2021).
Worldwide, dairy cows are kept in various production and housing systems ranging from pasture-based systems to fully indoor systems (Lambertz et al. 2014). About 75% of the dairy farms in Australia are pasture-based systems (Garcia et al. 2013). Additionally, pasture-based automatic milking systems (AMS) are becoming more popular, in which grazing is organised in such a way that voluntary movement of cows to the milking facility is rewarded by providing a new pasture allocation after milking.
The voluntary movement and motivation of cows to the milking facility under pasture-based AMS might change depending on the weather. During hot weather, cows are often less active as a common behavioural adaptation (West et al. 2003), since their body temperature increases in response to locomotion (Schütz et al. 2011). A decline in milking frequency (MF) during and soon after (overnight) a heat event in pasture-based AMS was observed (Wildridge et al. 2018). Speroni et al. (2006) also noted that the milk yield of cows in an indoor AMS (with reduced MF) had declined more rapidly than the milk yield of a herd that was simultaneously batch-milked in an indoor conventional milking system (CMS). In addition, during summer in CMS, cows are cooled using water and shade in the milking facility and waiting yard after collection for milking. While in AMS, the presence of cows at the milking facility 24 h a day, with only a small to moderate percentage of the herd is present at any one time, making challenges to use cooling strategies at the milking facility (Wildridge et al. 2017).
Considering the increasing popularity of pasture-based AMS in Australia, it is worthwhile to explore the impact of climate variables on dairy cows’ milk yield, milk quality and welfare under pasture-based AMS. The majority of the previous heat-stress studies were conducted in free-stall barn CMS (Soriani et al. 2013; Abeni and Galli 2017; Moretti et al. 2017; Ramón-Moragues et al. 2021), in an induced heat-stress climate chamber (Bernabucci et al. 2010; Garner et al. 2017; Maia et al. 2020), or the impacts of heat stress under summer conditions were modelled using historical climates (Nidumolu et al. 2010; Ji et al. 2020). Several earlier studies (Soriani et al. 2013; Maia et al. 2020; Müschner-Siemens et al. 2020) measured the impact of heat stress by using temperature–humidity index (THI), which combines ambient temperature and relative humidity into a single value. Maia et al. (2020) and Ramón-Moragues et al. (2021) used different thresholds of THI for heat-stress conditions to measure the impact on milk yield and rumination. Rumination is an indicator of welfare (Bar and Solomon 2010), which includes the process of regurgitation, remastication, salivation, and swallowing of ingesta to reduce the particle size of feedstuffs and enhance fibre digestion (Erina et al. 2013). Cattle spend 25–80 min ruminating per kilogram of roughage consumed (Sjaastad et al. 2003), and healthy matured dairy cows ruminate for 7 h/day (range: 2.5–10.5 h/day; Beauchemin 2018).
Two Australian pasture-based AMS studies (Wildridge et al. 2018; Osei-Amponsah et al. 2020) reported a drop in milk yield of 14% from low (≤72) to high (≥83) THI (Osei-Amponsah et al. 2020) and a 0.15 kg decrease per THI unit increase (Wildridge et al. 2018). The latter two studies were conducted only during the summer heat events of 1 year. To the best of our knowledge, limited studies have reported on the association of THI on milk yield, rumination and milk quality under pasture-based AMS in a single study by using a more extended dataset. Therefore, this study considered 3-year herd data to evaluate the effect of THI on daily milk yield, protein and fat concentration, and somatic cell count (SCC), MF and rumination time (RT). It was hypothesised that high THI would adversely affect milk yield, MF, RT and SCC.
Materials and methods
Farm, and animals’ data
Field measurements were performed from The University of Melbourne AMS dairy farm in Dookie (Victoria, Australia), located on latitude 36.4°S and longitude 145.7°E. The farm region has a Mediterranean climate, with an average annual rainfall of 550 mm. As described in the previous paper (Cullen et al. 2021), the AMS consisted of three Lely Astronaut robotic milking machines (Astronaut milking machine; Lely Industries NV, Maasland, Netherlands). The dairy farm has a pasture-based production system with an AMS and voluntary cow movement where cows move from the paddock to the dairy individually or in small groups on a voluntary basis. A ‘three-way’ grazing system was implemented where the pasture area was divided into three zones, and the cows were allowed access to a new paddock approximately every 8 h (Cullen et al. 2021). The herd consumed a diet consisting of grazed pasture, pasture silage/hay and concentrates fed during milking. Diet can vary according to the season, with an average of 50% of the diet from grazed pasture, 30% from concentrate, 20% from hay and silage. The farm’s total area was 86 ha, with 41 ha of border-check irrigated pasture and 14 ha of rainfed pasture used as grazing paddocks by the milking herd. Depending on the paddock allocated to the grazing herd, cows had to walk from 100 m to 1 km from the paddock to the AMS. The dairy has three fans, with an attached water mister set up for cooling in the undercover area of the dairy. These are controlled by a thermostat and turn on automatically if the temperature goes above 30°C (in the undercover), generally between October to March. There are two paddocks that have complete shade and extra water troughs that the cows have access after 11 am. The herd consisted of up to 158 Holstein–Friesian cows in milk at any one time. The farm had a split calving pattern, with approximately two-thirds of the cows calved between late winter and early spring, and one-third in summer/autumn. Minimum and maximum MFs were 1 and 6 respectively.
Daily milk yield in kilograms, milk quality (milk fat and protein concentration, somatic cell count), and cow (days in milk, lactation number, liveweight in kilograms, concentrate intake based on milk production) data were collected automatically by the robotic milking machine (Lely AMS), identifying individual cows via radio-frequency identification (RFID) ear tags. Additionally, all cows were fitted with a transponder collar (Qwes-HR, Lely). As validated by Schirmann et al. (2009) and described in the previous study (Talukder et al. 2015), these collars consisted of an accelerometer to monitor one daily RT (h/cow), with data downloaded automatically to the support software on a computer located at the dairy. Data for all lactating cows during the study period from June 2016 to March 2019 were included for analysis. Across the study, the average milk yield was 29 kg/day, MF was 2.4/day, days in milk was 181 days, liveweight was 662 kg and parity was 3.
Climate data
Climatic data, including daily minimum, average and maximum temperatures and relative humidity at maximum temperature were obtained from the Dookie weather meteorological station (http://weatherplus.ikcaldwell.com.au/) for the study period. The weather information included 15-min interval records of temperature (°C) and relative humidity (%). The THI was calculated for each 15-min climate record, and then the daily maximum THI (THImax) was identified in Microsoft Excel (Microsoft Corporation, Redmond, WA, USA). THI was calculated using the formula (Kelly and Bond 1971)
where Tmax is the maximum dry-bulb temperature (°C), and RH is the relative humidity at the maximum temperature of the air (%).
Maximum daily THI has been used in this study since milk yield, for instance, is more sensitive to the extreme values of the THImax than to the daily average THI (Brügemann et al. 2012). The climate data were used to calculate the THI from the Day 0, −1, −2, and −3 relative to the milking data, to assess the lag responses of the THI on outcome variables. To evaluate the severity of heat stress, THI was classified into the following four categories; No stress (THImax < 72), Mild stress (72 ≤ THImax < 78), Moderate stress (78 ≤ THImax < 82), and Severe stress (THImax ≥ 82) on the basis of Dairy Australia (2020) website.
Statistical analyses
The effects of various explanatory variables on RT, SCC (analysed as log10SCC), milk yield, milk protein (%), milk fat (%) and protein:fat ratio were analysed by a set of linear mixed models, incorporating smoothing splines to allow for possible non-linear effects. The form of each model was
where y is the particular response variable, and days in milk (DIM), maximum temperature–humidity index (THImax), concentrate feed intake (Feed) and liveweight (Weight) are covariate (linear) fixed effects with associated regression coefficients β1, …, β4, with non-linear effects of these four covariates specified as splines, s(DIM), s(THImax), s(Feed) and s(Weight) as random effects. Parity is a fixed-effect categorical variable. As there were multiple observations on each animal (indexed as i), random effects were specified as Animali (random intercept) and also random linear terms for each animal (b1i, …, b4i). Further animal-specific spline terms were specified, as indicated by the si(DIM), si(THImax), si(Feed) and si(Weight) terms. Consequently, the models allow for each animal to have their own individual response to each of the explanatory variables. The term β0 is the overall intercept, and ε is the random error. The models were fitted using ASReml-R (Butler et al. 2017). In ASReml-R, it is necessary for a non-linear term to be specified as both as a fixed effect (linear) and random effect (spline). Significance of fixed effects was assessed using Wald F-tests and random-effect variance components significance was tested using likelihood ratio chi-squared tests. For MF, a Poisson generalised linear mixed model was fitted to the count data by using ASReml-R, allowing for under-dispersion, with the same fixed and random effect terms as for the linear mixed models. Model-based predicted means were visualised using the ggplot2 package in R (Wickham 2016).
Results
During the study period, the farm’s maximum temperature, relative humidity at maximum temperature, and THImax ranged from 6.5°C to 45°C, 12.5% to 97.8% and 43.6 to 113 respectively. For all the response variables analysed, there were strong associations with the explanatory variables, as examined by the linear and non-linear trends (Table 1). However, in only one instance (effect of liveweight on log10SCC), no non-linearity was detected (P = 0.995), and the (negative) linear trend itself was only marginal (P = 0.053).
Explanatory variable | Milk yield | Rumination | Milking frequency | ||||
---|---|---|---|---|---|---|---|
Linear | Non-linear | Linear | Non-linear | Linear | Non-linear | ||
DIM | <0.001 | <0.001 | <0.001 | 0.0009 | 0.607 | <0.001 | |
THImax | <0.001 | <0.001 | <0.001 | <0.001 | 0.001 | <0.001 | |
Concentrate feed | <0.001 | <0.001 | 0.004 | <0.001 | <0.001 | <0.001 | |
Weight | <0.001 | <0.001 | <0.001 | <0.001 | 0.032 | <0.001 | |
Parity | <0.001 | <0.001 | <0.001 |
Explanatory variable | log10SCC | Milk fat % | Milk protein % | ||||
---|---|---|---|---|---|---|---|
Linear | Non-linear | Linear | Non-linear | Linear | Non-linear | ||
DIM | 0.003 | <0.001 | <0.001 | <0.001 | 0.116 | <0.001 | |
THImax | <0.001 | <0.001 | <0.001 | <0.001 | 0.421 | <0.001 | |
Concentrate feed | 0.042 | 0.001 | 0.001 | <0.001 | 0.002 | <0.001 | |
Weight | 0.053 | 0.995 | 0.076 | 0.002 | <0.001 | 0.398 | |
Parity | <0.001 | <0.001 |
Explanatory variable | Milk fat:protein | ||
---|---|---|---|
Linear | Non-linear | ||
DIM | <0.001 | <0.001 | |
THImax | <0.001 | <0.001 | |
Concentrate feed | 0.024 | <0.001 | |
Weight | <0.001 | 0.010 | |
Parity | <0.001 |
Values shown are P-values from the fitted linear mixed models. ‘Linear’ indicates significance of the overall linear trend for each of the explanatory variables based on Wald F-tests. ‘Non-linear’ indicates significance of the non0linear departure around the linear trend, based on likelihood ratio chi-squared tests.
The THI data showed a significant association with daily milk yield. Daily milk yield increased with a rising THImax to 65, was fairly constant until 85, followed by a decline afterwards (Fig. 1). Rumination time was maximum at mid-range THImax and declined for high and low values, particularly showing a decreasing trend after THImax 92. Milking frequency increased with THImax, reaching a maximum at THImax 90, followed by a steady decline afterwards. There was a steady decrease in log10SCC between THImax 56 and 106 (Fig. 1). An overall decline of milk fat% with an increasing THImax values was observed, except a rise from THImax 72 to 85. Milk protein percentage declined until THImax 72, followed by a sharp rise afterwards, except for the downward trend between THImax 85 and 95. Milk fat and protein ratio initially declined with an increasing THImax, with little change up to 90, but a decline beyond. The lag response of THImax tested at different intervals (from Day 0, −1, −2, and −3 relative to the milking data) on RT, milk yield, milk quality and log10SCC showed overall similar patterns.
Plots of the model-based mean milk yield (a), rumination time (b), milking frequency (c), log10SCC (d) and milk composition (e, f, g) for each of the five explanatory variables. For the four covariates (quantitative explanatory variables), the model-based mean response is the blue line, and the grey shading represents ±1 s.e. around the means. For ‘THImax’, three vertical lines are drawn at 72, 78 and 82, marking the boundaries between no, mild, moderate and high thermal stress. For parity, model-based means are shown, with error bars being ±1 s.e.
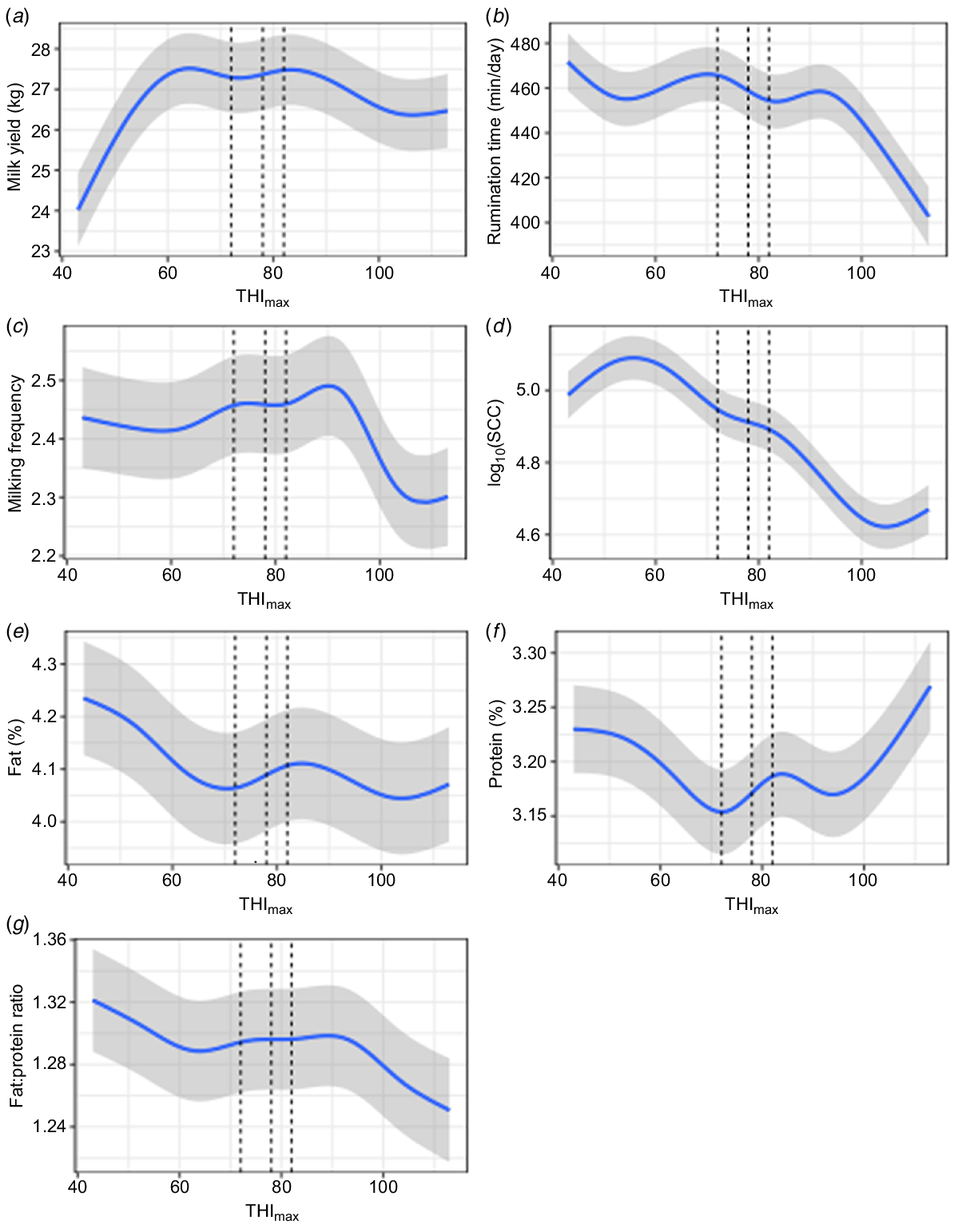
Discussion
Impact of THImax on milk yield and composition
The magnitude of milk yield losses in our study was lower than in other previous studies (Garner et al. 2017; Wildridge et al. 2018; Osei-Amponsah et al. 2020). In the first study (Garner et al. 2017), the cows were housed individually in climate-controlled respiration chambers during the experimental period and THI was calculated by a different equation, using hourly dry-bulb temperature and dew-point temperature. In the latter two studies (Wildridge et al. 2018; Osei-Amponsah et al. 2020), cows were under pasture-based systems and similar equations were used in THI calculation. There are couple of possible reasons for the lower differences in milk yield. First, in our study, during summer, the average night-time temperature was 10.5°C (range 5.5–17.9°C), which may be attributed to cool night-time temperatures allowing cows to dissipate heat gained during the day and resulting in moderate heat stress. Furthermore, the moderate effects of THI on milk yield could be the cooling strategies, for example, sprinklers/fan in the waiting yard, and providing shade on hot days by putting cows in paddocks with trees. The moderate effect on milk yield indicates that the current mitigating strategies in the study farm may be adequate to minimise heat stress during the summer season.
The effect of THI on milk protein% and fat% was not very clear in our study, with an indication of an overall decline in fat% with increasing values. Under pasture-based systems, an increase in milk fat% and protein% by 3% and 2% from low to high THI respectively, was reported (Osei-Amponsah et al. 2020). In the TMR feeding system (Nasr and El-Tarabany 2017), protein% was the lowest in the moderate THI, with no difference in low and high THI, while fat% was lower in the high THI than in the low THI. In the study of Osei-Amponsah et al. (2020), data were collected for one Australian summer season (December to February), while in our study, data were collected for three entire years, irrespective of the seasons. Therefore, the data collection duration might result in differences in the heat-stress effect on milk protein% and fat%.
In our study, milk yield reached the plateau between THImax 60 and 65 and then declined after THImax 65, which is consistent with the threshold mentioned in previous studies (Dunshea et al. 2013; Carabaño et al. 2016; Islam et al. 2021). A lower threshold of between THImax 60 and 68 has been suggested as the thermal threshold for high-producing dairy cows (Dunshea et al. 2013; Carabaño et al. 2016; Islam et al. 2021). A THI value of 72 as the upper threshold of cattle thermal comfort has been suggested by earlier literature (Bohmanova et al. 2007). A previous Australian study (Garner et al. 2017), using cows in a climate chamber with a THI maintained at THI 74–84 for 4 days, observed a 63% decrease in milk yield. Under the Mediterranean climate, previous studies reported a reduction in daily milk yield/cow by 2% for THI values of >69 (Bouraoui et al. 2002), 1% for each THI unit increase above 68 (Bernabucci et al. 2010) and a decrease in milk yield of 21% when the THI increased from 68 to 78 (Bouraoui et al. 2002). THI threshold for reduced milk yield was 72 (approximately 25°C at 50% relative humidity; Igono et al. 1992), which decreased to 68 at about 22°C at 50% relative humidity 10 years later (Wildridge et al. 2018).
Impact of THImax on MF
It was hypothesised that MF would decline in response to high THImax. However, in this study, the decline in MF was not observed in mild or moderate THImax. Instead, MF dropped significantly only when THImax was severe (above 90) and there was a concurrent reduction in milk yield. Cooling strategies, trees as shade might minimise the effect of mild/moderate THI on MF. In this study, we observed a lower MF during the spring–summer than autumn–winter period (2.4 ± 0.01 vs 2.5 ± 0.07), similar to the results of a previous study (Speroni et al. 2006). In pasture-based systems cows walk voluntarily from grazing paddock to milking area, which can be negatively affected by the hot weather, thus affecting the overall milking frequency of the herd (Wildridge et al. 2018). Spörndly and Wredle (2004) observed the negative effect of longer walking distances on milk yield, MF and grazing time, with a further decline in grazing time when greater walking distances are combined with warmer weather (Spörndly and Wredle 2004; Wildridge et al. 2017). Therefore, a reduction in voluntary movement in hot season might be a challenge for AMS. To minimise the impact on milking frequency and, subsequently, on milk yield, fetching of cows to encourage additional milkings before and after particularly hot weather can be useful (Stockdale 2006).
Impact of THImax on RT
The findings of this study support the hypothesis that high THI would adversely affect RT, with a decrease in RT as the THI shifted from moderate (78 ≤ THImax < 82) to severe THI (THImax ≥ 82). This is similar to a previous study (Soriani et al. 2013) where there was a reduction in daily RT for every daily maximum THI unit above the critical THI thresholds of 76 and 77. Furthermore, a weak negative correlation (r = −0.22) between RT and THI was reported in a more recent study (Moretti et al. 2017). The reduction in RT with an increasing THI might be due to reduced feed intake, i.e. a physiological and metabolic response activator to deal with unideal environmental conditions (Moretti et al. 2017; Maia et al. 2020). While our data collection did not allow the study of rumination patterns, daily rumination of cows has been reported to shift towards night, with more than 60% daily rumination occurring at night to reduce their metabolic heat load (Soriani et al. 2013; Ramón-Moragues et al. 2021).
In this study, the range of RT was 390–480 min/day, which was consistent with the previously reported studies. In a thermal neutral zone, RT in adult cows might range between 276 and 624 min/day (Yang and Beauchemin 2006; Adin et al. 2009). An average RT in dairy cows without disease and stress was estimated to be 463 min/day in primiparous and 522 min/day in pluriparous cows (Soriani et al. 2012). A change in RT might be attributed to physiological and management factors such as feeding management, feed intake, physical characteristics of the diet, and individual variation among animals (Beauchemin 2018; Maia et al. 2020). Approximately 32% of the variation in daily RT could be explained by intakes of the dietary fractions, whereas 48% of the total variation in RT was accounted for by individual variation among cows (Byskov et al. 2015).
Impact of THImax on log10SCC
In the present study, a significant decline was observed in log10SCC with an increasing THImax, indicating a greater log10SCC in autumn and winter. Studies that investigated the effect of season on bulk tank SCC had mixed results, with a high incidence of mastitis in dairy cows during the summer (Olde Riekerink et al. 2007; Hogan and Smith 2012) or during the autumn and winter (El-Tahawy and El-Far 2010). The increased incidence of mastitis during the summer might be related to the effect that high temperatures and humidity have on cows’ susceptibility to infections, as well as to the increased number of pathogens or vectors to which the cows are exposed (Bertocchi et al. 2014). However, those studies were conducted in the northern hemisphere with a climatic zone different from that in this study area located in the southern hemisphere with a Mediterranean climate. There have been limited studies concerning the relationship between THI or other climatic variables and somatic cell or bacterial count of dairy cows in Australia, making it difficult to compare the results of our study. Previous pasture-based studies in New Zealand reported that the prevalence of environmental mastitis, particularly Streptococcus uberis, is high in pasture-based seasonal calving dairying countries (McDougall 2002) and a significant variation of S. uberis contamination among seasons, being highest in winter and lowest in summer (Clements et al. 2005; Lopez-Benavides et al. 2007).
About two-thirds of dairy cows in this study calve during late winter and early spring, when lower temperatures and solar radiation are typical of that time of the year. The observed high somatic cell count during winter in this study might be positively associated with bacterial survival at cooler temperatures and with management factors occurring during these times, such as grazing intensity, which generates longer pasture rotation lengths during the autumn and winter months (Macdonald and Penno 1998; Lopez-Benavides et al. 2007). The longer pasture rotation implies that cows on pasture may maintain a contamination cycle through the faeces (Olde Riekerink et al. 2007), indicating that the cow’s presence in the paddocks is an important factor for bacterial survival in paddocks (Lopez-Benavides et al. 2007). The temperature and relative humidity in THI 50–60 might be favourable for bacterial growth, particularly coliforms, and the growth of environmental streptococci is greatest during warm, wet weather (Hogan and Smith 2012). Solar radiation and drying during the summer months reduce bacterial contamination in these areas compared with rainy winter months (Lopez-Benavides et al. 2007), which might explain the greater SCC during autumn and winter in the present study. Further investigation of SCC in relation to season and THI is needed.
Heat stress could cause a significant risk of developing contagious (or cow-associated) mastitis (Lambertz et al. 2014) caused by microorganisms such as Staphylococcus aureus, Streptococcus agalactiae, Arcanobacterium pyogenes and Mycoplasma spp. (Dairy Australia (2020). Cool cows: Strategies for managing heat stress in dairy cows. Dairy Australia 2020). Those microorganisms mostly live inside udders or on teat skin and spread from infected cows during milking by contaminated hands or milking machines (Dairy Australia 2020. Cool cows: Strategies for managing heat stress in dairy cows. Dairy Australia, 2020). The depressed immune function in heat-stressed cows during summer might increase the susceptibility of cows to contagious mastitis (Lacetera et al. 2005). In addition to the season, another important factor that could contribute to the effect of heat stress on SCC is the stage of lactation (Lambertz et al. 2014). As observed in our study, cows in early lactation had the lowest SCC, with an increasing trend in SCC afterwards with the progression of DIM, which is consistent with the results of a previous study (Abebe et al. 2016). Perhaps this could be linked to diapedesis of neutrophils into the mammary gland taking longer in recently calved cows (Radostits et al. 2007), and increased oxidative stress and reduced antioxidant defence mechanisms during early lactation (Sharma et al. 2011). In contrast, cows with five or more parities had a higher SCC than did cows with a parity four or less. The possible reason could be having pendulous cows in older cows with increased parities that expose the teat and udder to injury, and pathogens easily adhering to the teat and gain access to the gland tissue (Awale et al. 2012).
Conclusions
The findings of this study clearly indicated that under pasture-based voluntary-movement AMS, high THI resulted in a drop in the milk yield, MF and RT. Also, somatic cell count decreased with a high THI. Management strategies are needed to minimise heat stress and attain optimal animal productivity. With the provision of automation of data collection from AMS, further study with mathematical modelling describing the daily patterns and thresholds in conjunction with the different heat-stress levels can be useful to mitigate heat stress and seek alternative management strategies.
References
Abebe R, Hatiya H, Abera M, Megersa B, Asmare K (2016) Bovine mastitis: prevalence, risk factors and isolation of Staphylococcus aureus in dairy herds at Hawassa milk shed, South Ethiopia. BMC Veterinary Research 12, 270.
| Crossref | Google Scholar |
Abeni F, Galli A (2017) Monitoring cow activity and rumination time for an early detection of heat stress in dairy cow. International Journal of Biometeorology 61, 417-425.
| Crossref | Google Scholar |
Adin G, Solomon R, Nikbachat M, Zenou A, Yosef E, Brosh A, Shabtay A, Mabjeesh SJ, Halachmi I, Miron J (2009) Effect of feeding cows in early lactation with diets differing in roughage-neutral detergent fiber content on intake behavior, rumination, and milk production. Journal of Dairy Science 92, 3364-3373.
| Crossref | Google Scholar |
Awale MM, Dudhatra GB, Avinash K, Chauhan BN, Kamani DR (2012) Bovine mastitis: a threat to economy. Open Access Scientific Reports 1, 295.
| Google Scholar |
Bar D, Solomon R (2010) Rumination collars; what they tell us? In ‘Proceedings of the 1st North American conference, precision dairy management’, Toronto, Canada. Available at http://www.precisiondairy.com/proceedings/s11bar.pdf
Beauchemin KA (2018) Invited review: current perspectives on eating and rumination activity in dairy cows. Journal of Dairy Science 101, 4762-4784.
| Crossref | Google Scholar |
Bernabucci U, Lacetera N, Baumgard LH, Rhoads RP, Ronchi B, Nardone A (2010) Metabolic and hormonal acclimation to heat stress in domesticated ruminants. Animal 4, 1167-1183.
| Crossref | Google Scholar |
Bertocchi L, Vitali A, Lacetera N, Nardone A, Varisco G, Bernabucci U (2014) Seasonal variations in the composition of Holstein cow’s milk and temperature–humidity index relationship. Animal 8, 667-674.
| Crossref | Google Scholar |
Bohmanova J, Misztal I, Cole JB (2007) Temperature-humidity indices as indicators of milk production losses due to heat stress. Journal of Dairy Science 90, 1947-1956.
| Crossref | Google Scholar |
Bouraoui R, Lahmar M, Majdoub A, Djemali M, Belyea R (2002) The relationship of temperature-humidity index with milk production of dairy cows in a Mediterranean climate. Animal Research 51, 479-491.
| Crossref | Google Scholar |
Brügemann K, Gernand E, König von Borstel U, König S (2012) Defining and evaluating heat stress thresholds in different dairy cow production systems. Archives Animal Breeding 55, 13-24.
| Crossref | Google Scholar |
Byskov MV, Nadeau E, Johansson BEO, Nørgaard P (2015) Variations in automatically recorded rumination time as explained by variations in intake of dietary fractions and milk production, and between-cow variation. Journal of Dairy Science 98, 3926-3937.
| Crossref | Google Scholar |
Carabaño MJ, Logar B, Bormann J, Minet J, Vanrobays M-L, Díaz C, Tychon B, Gengler N, Hammami H (2016) Modeling heat stress under different environmental conditions. Journal of Dairy Science 99, 3798-3814.
| Crossref | Google Scholar |
Clements ACA, Pfeiffer DU, Hayes D (2005) Bayesian spatio-temporal modelling of national milk-recording data of seasonal-calving New Zealand dairy herds. Preventive Veterinary Medicine 71, 183-196.
| Crossref | Google Scholar |
Cullen BR, Weng H-M, Talukder S, Cheng L (2021) Cow milking order and its influence on milk production in a pasture-based automatic milking system. Animal Production Science 61, 306-312.
| Crossref | Google Scholar |
Dunshea FR, Leury BJ, Fahri F, DiGiacomo K, Hung A, Chauhan S, Clarke IJ, Collier R, Little S, Baumgard L, Gaughan JB (2013) Amelioration of thermal stress impacts in dairy cows. Animal Production Science 53, 965-975.
| Crossref | Google Scholar |
El-Tahawy AS, El-Far AH (2010) Influences of somatic cell count on milk composition and dairy farm profitability. International Journal of Dairy Technology 63, 463-469.
| Crossref | Google Scholar |
Erina S, Cziszter LT, Acatincăi S, Baul S, Tripon I, Gavojdian D, Răducan GG, Buzamăt G (2013) Research on rumination time according to administration order of forages in Romanian Black and White cows. Animal Science and Biotechnologies 46, 302-305.
| Google Scholar |
Garcia SC, Clark CEF, Kerrisk KL, Islam MR, Fariña SR, Evans J (2013) Gaps and variability in pasture utilisation in Australian pasture-based dairy systems. In ‘Proceedings of the 22nd international grassland congress’, University of Kentucky (Eds DL Michalk, GD Millar, WB Badgery, KM Broadfoot) pp. 1709–1716. (New South Wales Department of Primary Industry, Orange, NSW, Australia)
Garner JB, Douglas M, Williams SRO, Wales WJ, Marett LC, DiGiacomo K, Leury BJ, Hayes BJ (2017) Responses of dairy cows to short-term heat stress in controlled-climate chambers. Animal Production Science 57, 1233-1241.
| Crossref | Google Scholar |
Hansen PJ (2007) Exploitation of genetic and physiological determinants of embryonic resistance to elevated temperature to improve embryonic survival in dairy cattle during heat stress. Theriogenology 68, S242-S249.
| Crossref | Google Scholar |
Hendricks J, Mills KE, Sirovica LV, Sundermann L, Bolton SE, von Keyserlingk MAG (2022) Public perceptions of potential adaptations for mitigating heat stress on Australian dairy farms. Journal of Dairy Science 105, 5893-5908.
| Crossref | Google Scholar |
Herbut P, Hoffmann G, Angrecka S, Godyń D, Vieira FMC, Adamczyk K, Kupczyński R (2021) The effects of heat stress on the behaviour of dairy cows – a review. Annals of Animal Science 21, 385-402.
| Crossref | Google Scholar |
Hogan J, Smith KL (2012) Managing environmental mastitis. Veterinary Clinics of North America: Food Animal Practice 28, 217-224.
| Crossref | Google Scholar |
Horton RM, Mankin JS, Lesk C, Coffel E, Raymond C (2016) A review of recent advances in research on extreme heat events. Current Climate Change Reports 2, 242-259.
| Crossref | Google Scholar |
Igono MO, Bjotvedt G, Sanford-Crane HT (1992) Environmental profile and critical temperature effects on milk production of Holstein cows in desert climate. International Journal of Biometeorology 36, 77-87.
| Crossref | Google Scholar |
Islam MA, Lomax S, Doughty A, Islam MR, Jay O, Thomson P, Clark C (2021) Automated monitoring of cattle heat stress and its mitigation. Frontiers in Animal Science 2, 737213.
| Crossref | Google Scholar |
Ji B, Banhazi T, Ghahramani A, Bowtell L, Wang C, Li B (2020) Modelling of heat stress in a robotic dairy farm. Part 3: rumination and milking performance. Biosystems Engineering 199, 58-72.
| Crossref | Google Scholar |
Lacetera N, Bernabucci U, Scalia D, Ronchi B, Kuzminsky G, Nardone A (2005) Lymphocyte functions in dairy cows in hot environment. International Journal of Biometeorology 50, 105-110.
| Crossref | Google Scholar |
Lambertz C, Sanker C, Gauly M (2014) Climatic effects on milk production traits and somatic cell score in lactating Holstein-Friesian cows in different housing systems. Journal of Dairy Science 97, 319-329.
| Crossref | Google Scholar |
Lopez-Benavides MG, Williamson JH, Pullinger GD, Lacy-Hulbert SJ, Cursons RT, Leigh JA (2007) Field observations on the variation of Streptococcus uberis populations in a pasture-based dairy farm. Journal of Dairy Science 90, 5558-5566.
| Crossref | Google Scholar |
Maia GG, Siqueira LGB, Vasconcelos COdP, Tomich TR, Camargo LSdA, Rodrigues JPP, de Menezes RA, Gonçalves LC, Teixeira BF, Grando RdO, Nogueira LAG, Pereira LGR (2020) Effects of heat stress on rumination activity in Holstein-Gyr dry cows. Livestock Science 239, 104092.
| Crossref | Google Scholar |
McDougall S (2002) Bovine mastitis: epidemiology, treatment and control. New Zealand Veterinary Journal 50, 81-84.
| Crossref | Google Scholar |
Moretti R, Biffani S, Chessa S, Bozzi R (2017) Heat stress effects on Holstein dairy cows’ rumination. Animal 11, 2320-2325.
| Crossref | Google Scholar |
Müschner-Siemens T, Hoffmann G, Ammon C, Amon T (2020) Daily rumination time of lactating dairy cows under heat stress conditions. Journal of Thermal Biology 88, 102484.
| Crossref | Google Scholar |
Nasr MAF, El-Tarabany MS (2017) Impact of three THI levels on somatic cell count, milk yield and composition of multiparous Holstein cows in a subtropical region. Journal of Thermal Biology 64, 73-77.
| Crossref | Google Scholar |
Negri R, Daltro DdS, Cobuci JA (2021) Heat stress effects on somatic cell score of Holstein cattle in tropical environment. Livestock Science 247, 104480.
| Crossref | Google Scholar |
Nidumolu U, Crimp S, Gobbett D, Laing A, Howden M, Little S (2014) Spatio-temporal modelling of heat stress and climate change implications for the Murray dairy region, Australia. International Journal of Biometeorology 58, 1095-1108.
| Crossref | Google Scholar |
Olde Riekerink RGM, Barkema HW, Stryhn H (2007) The effect of season on somatic cell count and the incidence of clinical mastitis. Journal of Dairy Science 90, 1704-1715.
| Crossref | Google Scholar |
Osei-Amponsah R, Dunshea FR, Leury BJ, Cheng L, Cullen B, Joy A, Abhijith A, Zhang MH, Chauhan SS (2020) Heat stress impacts on lactating cows grazing australian summer pastures on an automatic robotic dairy. Animals 10, 869.
| Crossref | Google Scholar |
Ouellet V, Cabrera VE, Fadul-Pacheco L, Charbonneau É (2019) The relationship between the number of consecutive days with heat stress and milk production of Holstein dairy cows raised in a humid continental climate. Journal of Dairy Science 102, 8537-8545.
| Crossref | Google Scholar |
Ramón-Moragues A, Carulla P, Mínguez C, Villagrá A, Estellés F (2021) Dairy cows activity under heat stress: a case study in Spain. Animals 11 2305.
| Crossref | Google Scholar |
Schirmann K, von Keyserlingk MAG, Weary DM, Veira DM, Heuwieser W (2009) Technical note: Validation of a system for monitoring rumination in dairy cows. Journal of Dairy Science 92, 6052-6055.
| Crossref | Google Scholar |
Schütz KE, Rogers AR, Cox NR, Webster JR, Tucker CB (2011) Dairy cattle prefer shade over sprinklers: Effects on behavior and physiology. Journal of Dairy Science 94, 273-283.
| Crossref | Google Scholar |
Sharma N, Singh NK, Singh OP, Pandey V, Verma PK (2011) Oxidative stress and antioxidant status during transition period in dairy cows. Asian–Australasian Journal of Animal Science 24, 479-484.
| Crossref | Google Scholar |
Soriani N, Trevisi E, Calamari L (2012) Relationships between rumination time, metabolic conditions, and health status in dairy cows during the transition period. Journal of Animal Science 90, 4544-4554.
| Crossref | Google Scholar |
Soriani N, Panella G, Calamari L (2013) Rumination time during the summer season and its relationships with metabolic conditions and milk production. Journal of Dairy Science 96, 5082-5094.
| Crossref | Google Scholar |
Speroni M, Pirlo G, Lolli S (2006) Effect of automatic milking systems on milk yield in a hot environment. Journal of Dairy Science 89, 4687-4693.
| Crossref | Google Scholar |
Spörndly E, Wredle E (2004) Automatic milking and grazing – effects of distance to pasture and level of supplements on milk yield and cow behavior. Journal of Dairy Science 87, 1702-1712.
| Crossref | Google Scholar |
Stockdale CR (2006) Influence of milking frequency on the productivity of dairy cows. Australian Journal of Experimental Agriculture 46, 965-997.
| Crossref | Google Scholar |
Talukder S, Thomson PC, Kerrisk KL, Clark CEF, Celi P (2015) Evaluation of infrared thermography body temperature and collar-mounted accelerometer and acoustic technology for predicting time of ovulation of cows in a pasture-based system. Theriogenology 83, 739-748.
| Crossref | Google Scholar |
West JW, Mullinix BG, Bernard JK (2003) Effects of hot, humid weather on milk temperature, dry matter intake, and milk yield of lactating dairy cows. Journal of Dairy Science 86, 232-242.
| Crossref | Google Scholar |
Wildridge AM, Garcia SC, Thomson PC, Jongman EC, Clark CEF, Kerrisk KL (2017) The impact of a shaded pre-milking yard on a pasture-based automatic milking system. Animal Production Science 57, 1219-1225.
| Crossref | Google Scholar |
Wildridge AM, Thomson PC, Garcia SC, John AJ, Jongman EC, Clark CEF, Kerrisk KL (2018) Short communication: the effect of temperature-humidity index on milk yield and milking frequency of dairy cows in pasture-based automatic milking systems. Journal of Dairy Science 101, 4479-4482.
| Crossref | Google Scholar |
Yang WZ, Beauchemin KA (2006) Effects of physically effective fiber on chewing activity and ruminal ph of dairy cows fed diets based on barley silage. Journal of Dairy Science 89, 217-228.
| Crossref | Google Scholar |