A method for implementing methane breeding values in Australian dairy cattle
C. M. Richardson


A Agriculture Victoria Research, AgriBio, 5 Ring Road, Bundoora, Vic. 3083 Australia.
B School of Applied Systems Biology, La Trobe University, Bundoora, Vic. 3083, Australia.
C AbacusBio Limited, PO Box 5585, Dunedin 9058, New Zealand.
D Corresponding author. Email: caeli.richardson@agriculture.vic.gov.au
Animal Production Science - https://doi.org/10.1071/AN21055
Submitted: 5 February 2021 Accepted: 21 April 2021 Published online: 2 August 2021
Journal Compilation © CSIRO 2021 Open Access CC BY-NC-ND
Abstract
Context: There has been a lot of interest in recent years in developing estimated breeding values (EBVs) to reduce methane emissions from the livestock sector. However, while a major limitation is the availability of high-quality methane phenotypes measured on individual animals required to develop these EBVs, it has been recognised that selecting for improved efficiency of milk production, longevity, feed efficiency and fertility may be an effective strategy to genetically reduce methane emissions in dairy cows.
Aim: Applying carbon dioxide equivalents (CO2-eq) weights to these EBVs, we hypothesise that it is possible to develop a genetic tool to reduce greenhouse-gas emissions (GHG).
Methods: We calculated the effect of an EBV unit change in each trait in the Balanced Performance Index on CO2-eq emissions per cow per year. The estimated environmental weights were used to calculate a prototype index of CO2-eq emissions. The final set of EBVs selected for inclusion in the GHG subindex were milk volume, fat yield and protein yield, survival and feed saved, as these traits had an independent effect on emissions. Feed saved is the Australian feed efficiency trait. A further modification was to include a direct methane trait in the GHG subindex, which is a more direct genomic evaluation of methane estimated from measured methane data, calculated as the difference between actual and predicted emissions, for example, a residual methane EBV.
Key results: The accuracy of the GHG subindex (excluding residual methane EBV) is ~0.50, calculated as the correlation between the index and gross methane (using 3-day mean gross methane phenotypes corrected for fixed effects, such as batch and parity and adjusting for the heritability). The addition of the residual methane EBV had a minimal effect with a correlation of 0.99 between the indexes. This was likely to be due to limited availability of methane phenotypes, resulting in residual methane EBVs with low reliabilities.
Conclusions: We expect that as more methane data becomes available and the accuracy of the residual methane trait increases, the two GHG subindexes will become differentiated. When the GHG subindex estimates are applied to bull EBVs, it can be seen that selecting for bulls that are low emitters of GHG can be achieved with a small compromise in the BPI of ~20 BPI units (standard deviation of BPI = 100).
Implications: Therefore, selection for more sustainable dairy cattle, both economic and environmental, may be promptly implemented until sufficient data are collected on methane.
Keywords: methane emission, sustainability, selection index, index weights.
Introduction
It is recognised that within dairy systems, the largest source of greenhouse gas (GHG) emissions is from enteric fermentation and methane (CH4) production (g/day). Over 30 years (between 1980 and 2010), Moate et al. (2016) estimated that production of enteric CH4 has been almost static (185 000 t in 1980 versus 182 000 t in 2010). At the same time, milk production has increased, so that the intensity of CH4 emissions (i.e. emissions per unit of product, in this case, milk) has declined considerably by 40%, from ~33.6 g CH4/kg milk to 19.9 g CH4/kg milk. Since milk production traits are a large part of the breeding goal, it follows that the reduction in GHG has been, in part, the result of genetic improvement in efficiency and dilution of emissions per litre of milk produced.
The Australian national breeding objective, known as the Balanced Performance Index (BPI), includes traits that contribute to cow profitability, farmer preferences and desired gains (Byrne et al. 2016). The BPI encompasses biological traits associated with milk production, longevity, fertility, feed efficiency and health. Feed-efficiency breeding values, known as feed saved, include genomic breeding values for residual feed intake and breeding values for maintenance requirements based on predicted cow bodyweight (Pryce et al. 2015). Thus, by selecting for production traits and feed saved simultaneously, it is anticipated that gross efficiency will improve in Australian dairy cows (Byrne et al. 2016).
There has been a lot of interest in recent years in developing estimated breeding values (EBVs) to reduce GHG from the livestock sector. However, a major limitation has been the quantity of high-quality CH4 phenotypes measured on individual cows required to develop these EBVs. Currently, most published reliabilities are <10% (Manzanilla-Pech et al. 2021), which is insufficient for implementation of genomic prediction. However, it has been recognised that selecting for improved efficiency of milk production, longevity and fertility has had a beneficial effect on GHG emissions (Løvendahl et al. 2018). Consequently, it might be possible to develop an index that includes the contribution of these traits to GHG emissions, either separately, or in addition to CH4 emissions. Breeders with an interest in selecting for reduced GHG emissions, without greatly affecting profitability, can select for high-BPI and low-GHG emitters.
An approach that could be suitable is to estimate the increment in CO2-eq per unit change in EBVs that are current selection objectives, while accounting for interactions among traits, thereby calculating trait-specific environmental coefficients that are independent, such as those in Amer et al. (2018) and Richardson et al. (2021a) and that, for selection index purposes, are superior to non-independent coefficients, such as those calculated by Bell et al. (2013) and Pryce and Bell (2017).
The aim of this research was to (1) develop a GHG subindex using the gross CO2-eq values derived by Richardson et al. (2021a) as subindex weights for the EBVs most strongly associated with GHG emissions (milk, fat and protein yield, longevity, and feed saved), (2) modify the GHG subindex to include residual CH4 EBVs, as defined by Richardson et al. (2021b), from measured CH4 data phenotypically corrected for energy-corrected milk and (3) validate the GHG subindex with CH4 phenotypes corrected for fixed effects. Finally, we explored the effect of current selection objectives on CH4 by regressing the derived subindexes for GHG on BPI and Health Weighted Index (HWI).
Materials and methods
Previously calculated CH4 coefficients (kg CO2-eq/unit change in trait) were used as weights and applied to EBVs commonly used in selection and most strongly associated with emissions to derive two possible subindexes aimed to rank the environmental impact of individual animal on the basis of their genetic merit. The first index includes traits that are currently included in the national breeding objective and known to have a direct environmental impact, including production traits, survival and feed efficiency. The second includes these index traits as well as a direct CH4 trait. The two subindexes were correlated with current national breeding indexes (BPI and HWI), as well as additional traits of interest.
Australian national breeding objective
Currently, there are two main indexes used for ranking dairy cattle in Australia. The BPI includes traits pertaining to production (milk, fat, protein), fertility, survival, health and feed efficiency. The HWI, which was developed using the same traits as in the BPI, places additional emphasis on traits related to health and efficiency, such as fertility and feed saved. Details of the index development are given in Byrne et al. (2016). DataGene (Melbourne, Vic., Australia) calculates the BPI and HWI routinely for all cows and bulls included in national genetic evaluations.
Phenotypic and genetic cow data
In total, 1712 individual cow CH4 measurements were obtained from 464 cows measured over a 5-day period from 12 experimental batches across 5 years from 2013 to 2017. Cows were located at the Ellinbank SmartFarm (Melbourne, Vic., Australia) and milked twice per day. Measurements for CH4 (g/day) were performed using the SF6 tracer method previously described by Deighton et al. (2014). Records for CH4 were averaged to obtain one observation per animal to account for day-to-day variation (Moate et al. 2016). Animals were between 68 and 187 days in milk at the start of measurement and across parities 1–9. Genotypes for the 464 cows used in this study were provided by DataGene Ltd. After editing by excluding genotype calls with a GenTrain score <0.6 using the methods described by Erbe et al. (2012), 47 162 single nucleotide polymorphism (SNP) markers were available for genomic analysis. EBVs for the 464 animals used in this study were estimated as part of the routine genetic evaluation service of DataGene Ltd and included milk, fat, protein, survival and feed saved, which are traits directly used in GHG subindex development.
Genetic bull data
Genotypes for the 3412 registered Holstein bulls used in this study were provided by DataGene Ltd, with processing and genotyping methods being consistent with the cow dataset. Bulls were born between 2010 and 2015. The EBVs used in this analysis included milk volume, milk fat, milk protein, survival and feed saved, as well as other traits of interest such as heat tolerance and liveweight and were accessed from the April 2020 official genetic evaluation run.
Residual CH4 breeding value
Richardson et al. (2021b) investigated nine definitions of residual CH4 traits and concluded that CH4 production corrected for energy-corrected milk is an appropriate selection candidate to reduce environmental impact without severely affecting other traits, such as production, health and fertility. Using this definition of residual CH4 EBVs, we calculated genomic breeding values for bulls (or cows) that were not part of the reference dataset, by multiplying the vector of SNP effects with the genotype matrix of bulls. These SNP effects were derived using a process to back-calculate SNP effects from direct genomic values (DGVs) of cows in the reference population. In brief, using DGVs of CH4 production corrected for energy-corrected milk of 464 Ellinbank cow reference population, 41 276 SNP effects were estimated as:

where Z is the genotype matrix (464 individuals × 41276 SNP), and a vector of descaled DGVs with a mean of 0 estimated using genomic best linear unbiased prediction (VanRaden 2008). The prediction equations of SNP effects were then used to predict residual CH4 DGVs of the 3412 Holstein bulls.
Methane coefficients
Methane coefficients were previously calculated by Richardson et al. (2021a) on the basis of the approach used by Amer et al. (2018) and adapted to calculate the effect of a unit change in milk, fat, protein, feed saved, and survival traits on CO2-eq emissions per cow and per kilogram of protein equivalents. Protein equivalents are a weighted aggregate of the product outputs from milk protein, milk fat, and milk volume weighted on the component value ratio relative to protein. Briefly, this method estimates the change in total emissions and product output caused by a 1 unit change in each index trait, resulting from either a direct emissions trait (CH4 yield), changes in herd structure (fewer replacements), or the dilution effect of higher yields (milk production) and proliferation (more offspring/dam). The traits used in the current study were determined to have an independent effect on emissions, with the addition of fertility (Richardson et al. 2021a). However, as fertility is a primary reason for culling, the environmental impact of fertility is largely accounted for by the survival EBV, with minimal additional effects applying to extended lactations observed in seasonal calving systems (Workie et al. 2019; Richardson et al. 2021a). Therefore, only the survival CH4 coefficient is considered in the index. The model was used in the current study to dynamically represent an Australian dairy herd and assess effects of changes in traits. The effects of a unit change of a trait on GHG emissions are shown in Table 1. For example, a unit change in milk protein is estimated to be associated with a GHG emission of 1.97 kg of CO2-eq (3.70 kg DM/kg protein yield × 0.532 kg CO2eq / kg DM = 1.97 kg CO2eq/kg protein yield).
![]() |
GHG index
Indexes for CO2-eq emissions per cow per year were calculated by multiplying the EBVs of each trait in the breeding objective by the CH4 coefficient (i.e. effect of a single unit change of the trait on CO2-eq emissions). The sum of this is the total CO2-eq index. EBVs selected for this study included milk, fat, protein, feed saved and survival (Amer et al. 2018; Richardson et al. 2021a). Gross CH4 coefficients were applied to trait EBVs to calculate the GHG index as follows:
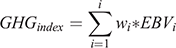
where wi is the CH4 coefficient (gross or intensity) of the ith trait and EBVi is the estimated breeding value of the ith trait (milk, fat, protein, feed saved or fertility).
GHG index with residual CH4 production
An additional index was developed that extends the GHG index described above through the addition of the EBV of residual CH4 and was calculated as follows:

where EBVRMP is the residual CH4 EBV and GHGindex is described above.
Correlations
The GHG indexes were correlated with the Australian national selection indexes, as well as with index traits currently estimated through the national genetic evaluation services (DataGene Ltd). Correlations were estimated using the cor.test() function in R.4.0.4 statistical programming (R Core Team 2013).
Validation of GHG index using CH4 phenotypes
The GHGindex subindex was validated using a 12-fold cross-validation, where the correlation between GHGindex and CH4 phenotypes for each experimental batch was independently estimated, that is, batch by batch. EBVs for index traits were provided by DataGene Ltd for milk, fat, protein, survival and feed saved on the 464 Ellinbank cows used in developing the residual CH4 trait. For the 464 cows, first GHGindex was calculated and then the values were correlated with SF6 CH4 phenotypes. As described by Su et al. (2012), to calculate the predicted accuracies of the true breeding values, instead of the EBVs, the correlations between the GHG subindex and SF6 CH4 phenotypes was divided by the square root of the heritability of residual CH4 production (h2 = 0.21; Richardson et al. 2021b).
The size of the dataset was insufficient to conduct a validation analysis for the GHG+index. This is because all data on 464 Ellinbank cows were required for development of the genomic prediction equation to estimate EBVRMP, so an independent dataset was not available.
Results
GHG index values
The GHGindex subindex was applied to 3412 Holstein bulls and had a mean value of 38.22 kg CO2-eq, with a standard deviation of 76.39. When residual CH4 was incorporated into the subindex, GHG+index, the standard deviation was 76.62 with a mean of 37.65 kg CO2-eq.
Correlation between indexes
Correlations between the GHG subindexes, BPI and HWI are presented in Table 2. The two GHG-based indexes, with and without the RMP EBV, were highly correlated (0.99). The BPI was uncorrelated with the GHGindex subindex and GHG+index subindex, with correlations of close to zero at –0.03 and –0.02 respectively. Favourable correlations were estimated between HWI and both GHG-based subindexes, with correlations of –0.35 with GHGindex and –0.36 with GHG+index. These trends were consistent within the top 300 BPI bulls, where the average BPI of the top 30 BPI bulls was 333. Considering only bulls whose GHG index value was below zero, the average BPI for the top 30 BPI bulls decreased to 312, representing a low 21-point difference (Fig. 1).
![]() |
![]() |
Correlation with index traits
Correlations between the GHG indexes and selected EBVs are presented in Table 2. The GHGindex and GHG+index subindexes had strong favourable correlations to feed saved (–0.71 and –0.70 respectively), fertility (–0.52 and –0.51) and survival (–0.43 and –0.42), as well as to heat tolerance (–0.45 and –0.46). Low to moderate favourable correlations were observed between the indexes and mastitis resistance (–0.29 and –0.28) and RMP (0.01 and 0.07). The traits with the largest unfavourable correlation with both indexes were fat (0.66), protein (0.57) and liveweight (0.56). Milk, milking temperament, likability and overall type had low to moderately unfavourable correlations of 0.30, 0.17, 0.16 and 0.14 respectively.
Validation
When GHG index values were correlated with SF6 CH4 data (Fig. 2, Table 2), the average correlation of the 12 validation batches was 0.23 (ranging 0.07–0.41). This gives the GHGindex subindex an accuracy of ~0.50, assuming a moderate heritability of 0.21 (Richardson et al. 2021b).
![]() |
Discussion
We have developed two GHG-based subindexes that can be used to select for reduced emissions. The advantage of our approach is that it utilises the EBVs of traits currently estimated through the national genetic evaluation service and therefore does not require a direct CH4 trait. Although we apply the method to Holsteins in the present paper, it can be adapted to derive weights for other dairy breeds, such as Jerseys and crossbreds, although the method should be validated in these breeds before implementation. This approach enables emission mitigation strategies through genetics to be implemented immediately, with the option of including a CH4 trait when available. Our results showed that there is little to no advantage in including the current residual CH4 trait, although this is expected to change with a higher-reliability residual CH4 trait. It is expected that a residual CH4 trait may be available in the future (Manzanilla-Pech et al. 2021; Richardson et al. 2021b). However, this is still a significant challenge, as CH4 phenotypes are expensive and laborious to measure and combining international datasets is challenging, with multiple measuring apparatus and techniques being used in data CH4 collection internationally, typically on a single breed (University of Guelph 2016).
Relationship between GHG indexes
The high correlation between the two calculated subindexes, GHGindex and GHG+index, showed that the RMP trait has a small effect on the index. This was expected, as the RMP trait has a low accuracy and a standard deviation of 5.0, whereas, for example, the standard deviation of the survival component of the GHG subindex was 28.36. However, as the RMP EBVs become more accurate, it is expected that the effect of the RMP traits within the index will increase proportional to the standard deviation and that the two GHG subindexes will further differentiate. We assumed that because the GHG subindexes and residual CH4 EBV are both measured in CO2-eq and that the index components can be added together to calculate GHGindex+. However, further analysis is required to optimise the residual CH4 EBVs within GHGindex+. Conceptually, the inclusion of RMP traits in the subindex is important (Knapp et al. 2014). The GHG subindex index will result in more environmentally efficient animals; however, selection on a direct CH4 trait will capture the variation in CH4 that exists between animals, resulting in a further reduction in CH4 emissions. Further improvements to the GHG index and an increased availability of CH4 phenotypes may help increase the accuracy of the index, and thereby the proportion of between-animal genetic variance captured by the index that can be utilised for selection. Although the effect of the RMP impact is minimal, these results have shown that we can use genetic selection to begin reducing emissions immediately. The GHGindex method offers an option to include a sustainability component in the national breeding objective, with minimal compromises to advances in BPI and profit. In dairy systems where it is not possible to obtain accurate CH4 phenotypes, the GHGindex offers a valuable alternative that can be implemented simply and immediately, as it utilises EBVs currently available through the national genetic services.
Relationships between GHG indexes and current indexes
The relationships between the GHG indexes (GHGindex and GHG+index) and the current national selection indexes (BPI and HWI) are favourable. The strong favourable correlations between HWI and GHG subindexes support the hypothesis that selection for improved efficiency and survival is a viable mitigation option, as previous studies have identified (Wall et al. 2010; Pryce and Haile-Mariam 2020). Although the HWI and BPI are strongly correlated (0.93), some re-ranking does occur between the indexes. The HWI places greater selection emphasis on health and functional traits, such as 5% on feed saved and ~40% on fertility and survival. Comparatively, the relative index weight in BPI for feed saved, and fertility and survival are 2.5% and 30% respectively (DataGene). The difference in comparative weighting also explains the differences in correlation with the GHG subindexes. Both the GHG subindexes and HWI place additional weight on survival and efficiency traits. However, the GHG subindexes also penalise higher production (milk, fat and protein). Selection on the HWI would results in favourable gains in the GHG subindexes. For the BPI, the correlations with the GHG subindexes were negligible (–0.02). However, in the top 50 bulls, 10 ranked negative on the GHG subindexes and a considerable number of bulls within the top 300 rank negative or close to 0 (Fig. 1). When considering the HWI, the GHG subindexes have a favourable negative correlation (–0.36) meaning that the higher the HWI, the less GHGs are emitted. As expected, on the basis of the favourable correlation between HWI and GHG, all but one of the top HWI Holstein bulls (within the top 300 BPI bulls) had a negative GHG subindex value, with one bull that was positive having a value very close to zero. This presents an opportunity to select for bulls that are profitable and also low GHG emitters.
Correlations between GHG indexes and index traits
As expected, the GHG subindexes are strongly correlated with the traits used in the index development, as well as with traits independent of index development that are suspected to have mitigation properties. Fertility was not included in the GHG subindexes as the CH4 coefficient previously calculated only accounted for a portion of the effects of fertility, with all effects related to a reduction in replacements being accounted for through the survival EBV (Richardson et al. 2021a). However, there is a strong favourable effect between the GHG subindexes and fertility. This large favourable effect is also seen with heat tolerance (Nguyen et al. 2017), a trait defined as a rate of decline in milk, fat and protein yields per unit increase in temperature–humidity index. Heat tolerance is not included in the BPI and has a strong negative correlation with production traits, similar to the GHG subindexes. This suggests that selection only for a lower GHG subindex would result in decreases in production. However, by combining the GHG subindex with HWI or BPI, we can ensure that both genetic gain in profitable traits and decreases in gross emissions are achieved. Historically, unfavourable correlations among traits have been overcome by simultaneous inclusion in selection indexes, such as with fat and protein yield, although genetic gain is seen at a slower rate (Miglior et al. 2005). Type and production traits are also unfavourably correlated with the GHG subindexes, whereas more functional traits such as calving ease and mastitis resistance are favourably correlated. This suggests that transitioning some selection emphasis from type and production traits is favourable for environmental impact, and as Miglior et al. (2017) suggested, the selection for robust animals is vital for the future sustainability of the dairy industry.
Validation
The validation methods of this research were limited by the small dataset size, as only the GHGindex subindex could be compared with the SF6 CH4 data. However, as the GHGindex and GHG+index subindexes were highly related, it is expected that a similar accuracy may be obtained for the GHG+index subindex. With an increase in dataset size to where a cross-validation may be conducted without jeopardising the accuracy of the RMP EBV or validity of the analysis, a validation may be also conducted on the GHG+index subindex with a higher confidence. The reliability of the GHGindex is sufficient for including the subindex in selection strategies. However, the accuracy of the GHGindex to select for direct CH4 is low. This is primarily due to the small dataset, as obtaining accurate CH4 phenotypes on a large number of individual animals is challenging. In Australia, CH4 phenotypes are currently measured on a reference population of 464 females, which is considerably lower than the suggested 5000-cow female reference population required for a moderately heritable trait to reach the same level of reliability (0.25) as the GHG subindex (González-Recio et al. 2014).
Practical decision-making
The GHG subindex may be used in practice to allow farmers to make environmentally conscious breeding decisions. The developed GHGindex subindex has many opportunities for implementation, with minimal compromise to profit (Fig. 1). Within the BPI, the GHGindex subindex could be included as an additional subindex and, although HWI is already favourably correlated with the GHG subindexes, inclusion of the GHGindex subindex in HWI would put additional weight on traits based on their environmental impact (i.e. favouring longevity and efficiency). Additionally, these weights may be used in culling decisions to differentiate between two animals of similar genetic merit, as the higher emitter may be removed from the herd or breeding stock. These GHGindex subindex values may be used on a magnitude or range basis. For example, the GHGindex subindex may be used to sort cows into high-, medium-, low- and negative-emitter ranges. While using only bulls or animals with negative GHGindex subindex values may not be possible at this time as this would result in increased inbreeding, farmers could use this range ranking system as a way of selecting for lower-emitting cows, leading towards a more environmentally conscious industry.
Future index weights
The traits within the GHG based subindexes are weighted based on their environmental impact. This weighting scheme is an alternative to the traditional economic index, which weights traits on the basis of profit. Alternative weighting options have been suggested, including social impact and farmer preference (Nielsen and Amer 2007). This has been implemented in the current Australian Breeding Objective through the HWI and previously through the (now discontinued) Type Weighted Index (Byrne et al. 2016). Using survey approaches described by Martin-Collado et al. (2015), weights were assigned to traits on the basis of the priority and preferences of farmers, which grouped farmers into three sections (profit based, health based, and type based). Multi-source weighting allows the weights within an index to be developed on the basis of a subset of conditions. Those conditions may be economic, social, environmental or preference based, with the final weight applied to a trait being an aggregate of these weights. This should be considered when including the GHGindex subindex in the national selection indexes (BPI and HWI) and not just as a supplementary selection decision tool. The GHG subindexes offer an additional option for weighting traits, on the basis of environmental impact. In theory, the GHG indexes are effectively weighting the traits twice. First, on the basis of economic analysis, and second, on the basis of environmental impact. However, in practice we are adjusting the index weight so that it is no longer purely economic based, but instead an aggregate weight of environmental and economic factors. This method may be especially useful to develop breeding strategies for traits with low economic values in breeds where expensive and laborious phenotypes, such as CH4, are not currently collected. Ideally, further breed-specific validations should be conducted, but as the majority of data has been collected on Holsteins, validation in other breeds is not possible at this time. As interest to include traits with low direct economic value in national selection indexes grows, further investigation is required to determine optimal inclusion methods.
Conclusions
This research has proposed a GHG subindex using EBVs currently estimated through the national genetic service and previously derived index weights that describe the change in CO2-eq associated with a unit change in each trait. The final set of EBVs selected for inclusion in the GHG subindex were milk, fat and protein yield, survival and feed saved as these traits had an independent effect on emissions. A further modification was to include a direct CH4 trait in the GHG subindex, which was a RMP EBV. The two GHG subindexes were highly correlated, suggesting the RMP had a minimal impact on the GHG index, which was expected due to the low accuracy of the RMP EBV. The GHG subindexes had high reliabilities; however, the accuracy of the subindexes to predict CH4 was reasonably low. The correlations between the GHG subindexes and current national selection indexes (HWI and BPI) suggest that selection strategies using HWI are expected to reduce emissions, whereas selection on BPI results in negligible changes in either GHG subindex. Type and production traits were unfavourably correlated with the GHG based subindexes, whereas traits associated with longevity, health, fertility and efficiency were favourable correlated. The GHG subindex may be included in the national selection index or used independently in practice by farmers to make environmentally conscious breeding and culling decisions, with minimal compromise to profit. Therefore, as the GHG subindex does not necessarily require a direct CH4 trait to lower the environmental impact of dairy cattle, selection for more sustainable dairy cattle may be promptly implemented until sufficient data are collected on CH4.
Conflicts of interest
The authors declare no conflicts of interest.
Acknowledgements
We thank DairyBio, jointly funded by Dairy Australia (Melbourne, Australia), The Gardiner Foundation (Melbourne, Australia) and Agriculture Victoria (Melbourne, Australia) for funding this project and C. M. Richardson’s PhD project. Special thanks go to colleagues from Agriculture Victoria Research and AbacusBio Ltd for useful discussions helped shape the thinking in this paper.
References
Amer PR, Hely FS, Quinton CD, Cromie AR (2018) A methodology framework for weighting genetic traits that impact greenhouse gas emissions intensity into selection indexes. Animal 12, 5–11.| A methodology framework for weighting genetic traits that impact greenhouse gas emissions intensity into selection indexes.Crossref | GoogleScholarGoogle Scholar | 28693653PubMed |
Byrne TJ, Santos BFS, Amer PR, Martin-Collado D, Pryce JE, Axford M (2016) New breeding objectives and selection indices for the Australian dairy industry. Journal of Dairy Science 99, 8146–8167.
| New breeding objectives and selection indices for the Australian dairy industry.Crossref | GoogleScholarGoogle Scholar | 27522425PubMed |
Bell MJ, Eckard RJ, Haile-Mariam M, Pryce JE (2013) The effect of changing cow production and fitness traits on net income and greenhouse gas emissions from Australian dairy systems. Journal of Dairy Science 96, 7918–7931.
| The effect of changing cow production and fitness traits on net income and greenhouse gas emissions from Australian dairy systems.Crossref | GoogleScholarGoogle Scholar | 24140333PubMed |
Deighton MH, Williams SRO, Hannah MC, Eckard RJ, Boland TM, Wales WJ, Moat PJ (2014) A modified sulphur hexafluoride tracer technique enables accurate determination of enteric methane emissions from ruminants. Animal Feed Science and Technology 197, 47–63.
| A modified sulphur hexafluoride tracer technique enables accurate determination of enteric methane emissions from ruminants.Crossref | GoogleScholarGoogle Scholar |
Erbe M, Hayes BJ, Matukumalli LK, Goswami S, Bowman PJ, Reich M, Mason BA, Goddard ME (2012) Improving accuracy of genomic predictions within and between dairy cattle breeds with imputed high-density single nucleotide polymorphism panels. Journal of Dairy Science 95, 4114–4129.
| Improving accuracy of genomic predictions within and between dairy cattle breeds with imputed high-density single nucleotide polymorphism panels.Crossref | GoogleScholarGoogle Scholar | 22720968PubMed |
González-Recio O, Coffey MP, Pryce JE (2014) On the value of the phenotypes in the genomic era. Journal of Dairy Science 97, 7905–7915.
| On the value of the phenotypes in the genomic era.Crossref | GoogleScholarGoogle Scholar | 25453600PubMed |
Knapp JR, Laur GL, Vadas PA, Weiss WP, Tricarico JM (2014) Invited review: enteric methane in dairy cattle production: quantifying the opportunities and impact of reducing emissions. Journal of Dairy Science 97, 3231–3261.
| Invited review: enteric methane in dairy cattle production: quantifying the opportunities and impact of reducing emissions.Crossref | GoogleScholarGoogle Scholar | 24746124PubMed |
Løvendahl P, Difford GF, Li B, Chagunda MGG, Huhtanen P, Lidauer MH, Lassen J, Lund P (2018) Review: selecting for improved feed efficiency and reduced methane emissions in dairy cattle. Animal 12, s336–s349.
| Review: selecting for improved feed efficiency and reduced methane emissions in dairy cattle.Crossref | GoogleScholarGoogle Scholar | 30255826PubMed |
Manzanilla-Pech CIV, Løvendahl P, Mansan Gordo D, Difford GF, Pryce JE, Schenkel F, Wegmann S, Miglior F, Chud TC, Moate PJ, Williams SRO, Richardson CM, Stothard P, Lassen J (2021) Breeding for reduced methane emission and feed-efficient Holstein cows: an international response. Journal of Dairy Science 104, 8983–9001.
| Breeding for reduced methane emission and feed-efficient Holstein cows: an international response.Crossref | GoogleScholarGoogle Scholar | 34001361PubMed |
Martin-Collado D, Byrne T, Am P, Santos B, Axford M, Pryce J (2015) Analyzing the heterogeneity of farmers’ preferences for improvements in dairy cow traits using farmer typologies Journal of Dairy Science 98, 4148–4161.
| Analyzing the heterogeneity of farmers’ preferences for improvements in dairy cow traits using farmer typologiesCrossref | GoogleScholarGoogle Scholar | 25864048PubMed |
Miglior F, Muir BL, Van Doormaal BJ (2005) Selection indices in Holstein cattle of various countries Journal of Dairy Science 88, 1255–1263.
| Selection indices in Holstein cattle of various countriesCrossref | GoogleScholarGoogle Scholar | 15738259PubMed |
Miglior F, Fleming A, Malchiodi F, Brito LF, Martin P, Baes CF (2017) A 100-year review: identification and genetic selection of economically important traits in dairy cattle. Journal of Dairy Science 100, 10251–10271.
| A 100-year review: identification and genetic selection of economically important traits in dairy cattle.Crossref | GoogleScholarGoogle Scholar | 29153164PubMed |
Moate PJ, Deighton MH, Williams SRO, Pryce JE, Hayes BJ, Jacobs JL, Eckard RJ, Hannah MC, Wales WJ (2016) Reducing the carbon footprint of Australian milk production by mitigation of enteric methane emissions. Animal Production Science 56, 1017–1034.
| Reducing the carbon footprint of Australian milk production by mitigation of enteric methane emissions.Crossref | GoogleScholarGoogle Scholar |
Nielsen HM, Amer PR (2007) An approach to derive economic weights in breeding objectives using partial profile choice experiments. Animal 1, 1254–1262.
| An approach to derive economic weights in breeding objectives using partial profile choice experiments.Crossref | GoogleScholarGoogle Scholar | 22444881PubMed |
Nguyen TT, Bowman PJ, Haile-Mariam M, Nieuwhof GJ, Hayes BJ, Pryce JE (2017) Implementation of a breeding value for heat tolerance in Australian dairy cattle Journal of Dairy Science 100, 7362–7367.
| Implementation of a breeding value for heat tolerance in Australian dairy cattleCrossref | GoogleScholarGoogle Scholar | 28711268PubMed |
Pryce JE, Gonzalez-Recio O, Nieuwhof G, Wales WJ, Coffey MP, Hayes BJ, Goddard ME (2015) Hot topic: Definition and implementation of a breeding value for feed efficiency in dairy cows. Journal of Dairy Science 98, 7340–7350.
Pryce JE, Bell MJ (2017) The impact of genetic selection on greenhouse-gas emissions in Australian dairy cattle. Animal Production Science 57, 1451–1456.
| The impact of genetic selection on greenhouse-gas emissions in Australian dairy cattle.Crossref | GoogleScholarGoogle Scholar |
Pryce JE, Haile-Mariam M (2020) Symposium review: genomic selection for reducing environmental impact and adapting to climate change. Journal of Dairy Science 103, 5366–5375.
| Symposium review: genomic selection for reducing environmental impact and adapting to climate change.Crossref | GoogleScholarGoogle Scholar | 32331869PubMed |
R Core Team (2013) ‘R: a language and environment for statistical computing.’ (R Foundation for Statistical Computing: Vienna, Austria)
Richardson CM, Amer PR, Hely F, van den Berg I, Pryce JE (2021a) Estimating methane coefficients to predict the environmental impact of traits in the Australian dairy breeding program.
Richardson CM, Nguyen TTT, Abdelsayed M, Moate PJ, Williams SRO, Chud TCS, Schenkel FS, Goddard ME, van den Berg I, Cocks BG, Marett LC, Wales WJ, Pryce JE (2021b) Genetic parameters for methane emission traits in Australian dairy cows. Journal of Dairy Science 104, 539–549.
| Genetic parameters for methane emission traits in Australian dairy cows.Crossref | GoogleScholarGoogle Scholar | 33131823PubMed |
Su G, Christensen OF, Ostersen T, Henryon M, Lund MS (2012) Estimating additive and non-additive genetic variances and predicting genetic merits using genome-wide dense single nucleotide polymorphism markers. PLoS One 7, e45293
| Estimating additive and non-additive genetic variances and predicting genetic merits using genome-wide dense single nucleotide polymorphism markers.Crossref | GoogleScholarGoogle Scholar | 23056402PubMed |
University of Guelph (2016) Efficient dairy genome project (EDGP). Available at https://genomedairy.ualberta.ca [Verified 3 November 2020]
VanRaden PM (2008) Efficient methods to compute genomic predictions. Journal of Dairy Science 91, 4414–4423.
| Efficient methods to compute genomic predictions.Crossref | GoogleScholarGoogle Scholar | 18946147PubMed |
Wall E, Ludemann C, Jones H, Audsley E, Moran D, Roughsedge T, Amer PR (2010) The potential for reducing greenhouse gas emissions for sheep and cattle in the UK using genetic selection. Final report to DEFRA. DEFRA, London, UK.
Workie ZW, Gibson JP, van der Werf JHJ (2019) Age at culling and reasons of culling in Australian dairy cows. Proceedings of the Association for the Advancement of Animal Breeding and Genetics 23, 143–146.