Genetic and genomic relationship between methane production measured in breath and fatty acid content in milk samples from Danish Holsteins
J. Lassen A C , N. A. Poulsen B , M. K. Larsen B and A. J. Buitenhuis AA Centre for Quantitative Genetic and Genomics, Department of Molecular Biology and Genetics, Faculty of Science and Technology, Aarhus University, PO Box 50, DK-8830 Tjele, Denmark.
B Aarhus University, Department of Food Science, Blichers Allé 20, PO Box 50, DK-8830 Tjele, Denmark.
C Corresponding author. Email: jan.lassen@mbg.au.dk
Animal Production Science 56(3) 298-303 https://doi.org/10.1071/AN15489
Submitted: 27 August 2015 Accepted: 15 November 2015 Published: 9 February 2016
Journal Compilation © CSIRO Publishing 2016 Open Access CC BY-NC-ND
Abstract
In this study the objective was to estimate the genetic and genomic relationship between methane-related traits and milk fatty acid profiles. This was done using two different estimation procedures: a single nucleotide polymorphism-based genomic relationship matrix and a classical pedigree-based relationship matrix. Data was generated on three Danish Holstein herds and a total of 339 cows were available for the study. Methane phenotypes were generated in milking robots during milking over a weekly period and the milk phenotypes were quantified from milk from one milking. Genetic and genomic parameters were estimated using a mixed linear model. Results showed that heritability estimates were comparable between models, but the standard error was lower for genomic heritabilities compared with genetic heritabilities. Genetic as well as genomic correlations were highly variable and had high standard errors, reflecting a similar pattern as for the heritability estimates with lower standard errors for the genomic correlations compared with the pedigree-based genetic correlations. Many of the correlations though had a magnitude that makes further studies on larger datasets worthwhile. The results indicate that genotypes are highly valuable in studies where limited number of phenotypes can be recorded. Also it shows that there is some significant genetic association between methane in the breath of the cow and milk fatty acids profiles.
Additional keywords: correlations, dairy cattle, fatty acids, heritability, methane.
Introduction
Methane (CH4) is a potent greenhouse gas that is ~25 as active as carbon dioxide. Dairy cows emit CH4 while digesting and their contribution to the worldwide emission of greenhouse gas is substantial. Several mitigation strategies have been suggested: feeding, vaccination, management but also genetic selection (Cottle et al. 2011). In order to select for a given trait, the trait must show genetic or genomic variability and a substantial amount of data from individual cows are needed.
Several studies have worked on developing methods for large-scale phenotyping of individual CH4 measurements (Garnsworthy et al. 2012; Lassen et al. 2012). These methods focus on CH4 recordings while cows are being milked in automatic milking systems. However, even with this effort, it will still be very time consuming and difficult to make direct individual CH4 measurements on all cows or the majority of cows in a population to make genetic selection possible. Therefore, indicators are needed, if CH4 emission from dairy cattle should be decreased. It has been shown that feed intake can be used to predict CH4 emission (de Haas et al. 2011). It is even harder to imagine that feed intake data rather than CH4 data should be available on the majority of the cows in a population.
A stoichiometric relationship between CH4 and ruminal acetate, propionate, and butyrate was proposed by Demeyer and Van Nevel (1975). These volatile fatty acids, which are primarily formed in the rumen act as precursors for the de novo synthesis of milk fatty acids in the mammary tissue. Thus, milk fatty acid data has been used to predict CH4 emission in several studies (Dijkstra et al. 2011; Dehareng et al. 2012). Additionally, if one would like to implement CH4 in the breeding goal it is important to know the correlation to other traits. Kandel et al. (2013) showed positive genetic correlations between milk mid-infrared spectre (MIR) CH4 in g/day and energy-corrected milk (ECM), fat yield, and protein yield (Kandel et al. 2013). This means that a decrease of CH4 should have negative impacts on milk, fat and protein yields. Due to the relative small datasets with CH4 measures standard errors are usually high. Recently it was shown that single nucleotide polymorphism (SNP) information can be used to estimate the heritability in small datasets of ~400 animals (Krag et al. 2013a, 2013b).
This methodology has successfully been applied to fatty acid concentrations in milk fat with moderate estimates of heritability ranging from 0.10 for C18:1 trans-11 to 0.34 for C8:0 and C10:0 (Krag et al. 2013b). They showed that the SNP markers capture the population structure well and that SNP markers could be used as an alternative to traditional pedigree-based methods. Use of the genomic relationship matrix will decrease the standard error of the estimates as the true genetic relationship between animals is utilised in a better way than when a traditional pedigree is applied.
The aim of this study was to estimate the genetic and genomic heritability for CH4-related traits and fatty acid concentrations in milk fat as well as to estimate the genetic and genomic correlation between CH4-related traits and fatty acids in milk. This is done on a limited dataset but can give an indication of how milk fatty acids and CH4 emission relates genetically in dairy cattle.
Materials and methods
Data were generated on three commercial farms in Denmark. A total of 339 Holstein cows were available for this study. Several phenotypes were generated or extracted for the analysis (Table 1). Methane measuring equipment was installed in milking robots using a portable Fourier transformed infrared spectre measuring device making a registration of CH4 and carbon dioxide every 5 s (Lassen et al. 2012). This data was merged with traffic data from each milking robot so that each cow got a phenotype for each visit in the robot. The equipment was installed in each milking robot for 7 days. From each visit, bodyweight and milk yield were also recorded by the milking robot. From these records a weekly mean of bodyweight and milk production was calculated. This information was used to estimate daily CH4 production in grams per day (Madsen et al. 2010). Methane in g/day (CH4_GRAMS) is calculated based on heat-producing units (HPU), which is equal to (5.6*liveweight0.75 + 22*FPCM + 1.6*10−5*days carried calf). For each HPU a cow produces 180 g of carbon dioxide per h. CH4_GRAMSw is thereby CH4_RATIO*180*24*HPU. Also CH4 per kg of ECM (CH4_MILK) and the ratio between CH4 and carbon dioxide CH4_RATIO were used as CH4-related traits. Overall fat and protein percentages in milk were derived from the national milk recording system based on the nearest milking record from the week where CH4 measurements were taken. Also milk samples were taken to measure milk fatty acid content in the milk. This was done by gas chromatography, essentially as described by Larsen et al. (2013).
![]() |
The pedigree file contained 8049 animals and was traced back as far as possible in the national cattle database.
Genotyping
In total 339 DH cows were genotyped with the BovineSNP50 beadchip (http://www.illumina.com/Documents/products/datasheets/datasheet_bovine_snp5O.pdf, verified 18 November 2015). Genomic DNA was extracted from ear tissue. The platform used was an Illumina Infinium II Multisample assay device. SNP chips were scanned using iScan and analysed using Beadstudio software version 3.1. The quality parameters used for the selection of SNP in the GWAS were minimum call rates of 80% for individuals and 95% for loci. Marker loci with minor allele frequencies (MAF) below 1% were excluded. The quality of the markers was assessed using the GenCall data analysis software of Illumina. Individuals with average GenCall scores below 0.65 were excluded following Teo et al. (2007). The SNP positions were based on the Bos taurus genome assembly (Btau_4.0) (Liu et al. 2009). In total 39 121 SNP markers were used.
Calculation of the G-matrix
The calculation of the genomic relationship matrix has been described in detail by Buitenhuis et al. (2011). For each chromosome, a genomic relationship matrix as described by the first method presented in VanRaden (2008) was calculated as follows: Let M be a matrix with dimensions of the number of individuals (n) by the number of loci (m) that specifies which marker alleles each individual inherited. The elements of M were set to –1, 0, 1 for the homozygote, heterozygote and the other homozygote, respectively. The diagonals of M’M counts the number of homozygous loci for each individual and off diagonals measure the number of alleles shared by relatives. Let the frequency of the second allele at locus i be pi, and let P contain the allele frequencies, such that column i of P equals 2(pi – 0.5). Subtraction of P from M gives Z, which is needed to set the expected mean value to 0. The genomic relationship matrix G was then calculated as ZZʹ/[2∑pi(1 – pi)] (VanRaden 2008).
Statistical analyses
The data was analysed using equivalent models for the different traits but with two different types of relationship structures. First approach was a standard pedigree-based approach where the inverse of the animal model A matrix is set up. In the second approach a SNP-based genomic relationship matrix was set up.
The following linear animal model was used to infer genetic parameters for CH4_GRAMS.

Where yijklm is the dependent phenotype CH4_GRAMS, μ is the overall intercept, herdi is a fixed effect of the herd-id where cows were measured, monthj is a fixed effect, robot*herd is the robot by herd interaction, lactk is a fixed effect of the lactation number at recording and dimL is the days in milk at recording. Days in milk was modelled with a linear regression and an exponential Wilmink term to take changes in early lactation into account. This fits a lactation curve to the data (Wilmink 1987). The β’s are fixed regression coefficients. am is the random animal effects and eijklm is the random residual effect.
The following linear animal model was used to infer genetic parameters for CH4_MILK, ECM and weight.

Where y is the dependent phenotype CH4_MILK, ECM or weight, μ is the overall intercept, herd is the herd-id where cows were measured, month* year is the month by year interaction, lact is the lactation number at recording and dim is the days in milk at recording. Days in milk was modelled with a linear regression and a Wilmink term to take changes in early lactation into account. The β’s are fixed regression coefficients; a’s are the random animal effects and e is the random residual effect.
The random effects for all models are assumed to be independently and normally distributed with means of zero. G0 is a matrix containing the additive genetic variance. A is a matrix with the additive genetic relationship of all animals. Pe0 is a matrix containing the permanent environmental variance for CH4_RATIO and R0 is a matrix with the residual variance. I is the identity matrix containing as many rows and columns as there are records for each trait.
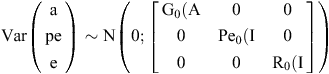
Variance and covariance components are estimated using the AI-REML procedure in DMU (Madsen and Jensen 2014). Correlations were estimated using a bi-variate model between CH4_RATIO, CH4_GRAMS, CH4_MILK, ECM, weight of all milk fatty acids and standard errors were estimated using a Taylor series approximation.
Results and discussion
Overall mean, standard deviation, minimum and maximum values for all the traits analysed are presented in Table 1. The milk data are generally in agreement with the values obtained in another study on Danish Holstein (Krag et al. 2013b). The average CH4 production was 395 g per day and the CH4 production in grams per kilo ECM was 11.04. This is in agreement with other studies on large-scale CH4 recordings in dairy cattle (Lassen et al. 2012). Genetic and genomic heritabilities are shown in Table 2. For most milk fatty acids heritability estimates were moderate to low (0.00–0.6). For the three CH4 traits the heritability was ~0.2 ranging from 0.09 to 0.26. Heritabilities estimated using either the SNP-based genomic relationship matrix or the pedigree-based relationship matrix are in agreement, but in most cases, standard error of the estimates are lower for the SNP-based genomic relationship matrix (mean standard error of 0.13) compared with the classical pedigree-based relationship matrix (mean standard error of 0.14) though not significant. Heritability estimates for C18 fatty acids are comparable to estimates found in Krag et al. (2013a), whereas the estimates for the de novo fatty acids are somewhat lower and estimates for C14:1 and C16:1 and to some extent C15:0 and C17:0 are higher. The difference in heritability estimates in the study by Krag et al. (2013a) could be due to a more stringent selection of cows, where daughter groups of limited size were achieved, whereas in this study, all animals were analysed without any restrictions on relationship. However, data recording was restricted to three farms, which means a small number of sires were used. This means that the cows in the current dataset might be more related compared with the cows in the Krag et al. (2013a) dataset.
![]() |
High heritabilities for C14:1 and C16:1 suggest that the desaturase activity underlying these fatty acids, to a large extent, is genetically regulated, which might be related to variation within the stearoyl-CoA desaturase 1 gene. However, in both studies the fatty acid heritability estimates are hardly significant due to relatively high standard errors. The differences in heritabilities for C14:1 and C16:1 between the pedigree-based and the SNP-based analysis is though probably largely due to randomness. In another study of 1800 Dutch Holstein cows intra-herd heritabilities were estimated for 14 milk fatty acids and estimates were somewhat higher than in this study ranging from 0.24 for C18:0 to 0.72 for C10:0 (Bouwman et al. 2011). In a UK study of 2408 Holstein cows heritability estimates for 16 milk fatty acids ranged from 0.00 for C18:1, trans-11 to 0.28 for C14:1, cis-9 (Garnsworthy et al. 2010). The magnitude of the heritabilities found in the present study seems to be closer to those found by Garnsworthy et al. (2010) than those found by Bouwman et al. (2011). This can be due to the relaxed selection of animals based on the whole herd in the present study as well as in the study by Garnsworthy et al. (2010).
Genetic and genomic correlations were highly variable between methods and estimates are all over the parameter space (Table 3). Also in some cases, there was problem with obtaining convergence and thereby reasonable estimates. This was especially true for some of the C18 fatty acids where standard errors of the estimates sometimes were even higher than 1. As for the heritability estimates, the standard errors were higher for the pedigree-based estimates (mean standard error of 0.56, 0.61 and 0.66 for correlations with CH4_GRAMS, CH4_MILK and CH4_RATIO, respectively), compared with estimates using the SNP-based genomic relationship matrix (mean standard error of 0.44, 0.43 and 0.63 for correlations with, CH4_GRAMS, CH4_MILK and CH4_RATIO, respectively). The de novo fatty acids in particular seem to be significantly negatively correlated CH4_MILK, when using both methods, whereas C13:0, C15:0 and partly C17:0 had significantly positive genomic correlations with all CH4 traits. The uneven saturated fatty acids are generated by the microbiota in the rumen and the content of these fatty acids in milk has previously been associated with the molar proportion of volatile fatty acids in the rumen (Vlaeminck et al. 2006). The standard errors of the estimates shown in this study are substantial, and it might not be possible to come to the same results on another similar study in another population of animals. SNP-based genomic relationship matrices to estimate heritability were shown to provide smaller standard error on the estimates compared with classical pedigree-based heritabilities. This is in line with the results of Krag et al. (2013a), who showed that when measures are hard or expensive to obtain reliable heritability estimates can be obtain on datasets containing ~400 animals with registrations and genotypes on traits with heritabilities higher than 0.15. In this study, we are right on the border of these thresholds for many of the traits and therefore also several heritabilities are not significantly different from 0. This is similarly the case for the genetic and the genomic correlations between the CH4 traits and the milk component traits. There is a positive effect on the standard error of the estimates to use a SNP-based genomic relationship matrix rather than a classical pedigree-based relationship matrix. But again the data collected in this study is on the border of the data that is needed to provide significant genetic or genomic correlations even though the results show that the standard error decreases with use of DNA information. Therefore, it can be beneficial to use the resources on getting genotypes rather than getting more expensive phenotypes.
![]() |
Using MIR to predict other phenotypes is indeed appealing. The phenotypic and genetic variability of CH4 production (g/day) and CH4 intensity (g/kg ECM) has been predicted by MIR. However, such estimates based on predicted CH4 are heavily smoothed and are based on traits that already have high heritability, so they are expected to have high heritability themselves. In 2013, Kandel et al. estimated genetic parameters of MIR predicted CH4 traits by using single trait random regressions test-day models from 679 444 test-day records collected from Holstein cows in their first three lactations. The calculated heritability values were ~0.10 for CH4 in g/day (0.12, 0.10 and 0.09 for the 1st, 2nd, and 3rd parity, respectively). The heritability for CH4 intensity was slightly higher with values ~0.15 (0.18, 0.12, and 0.14 for cows in their first three parities). These results suggest a relatively low heritability of CH4 emission by dairy cows. Kandel et al. (2014) studied the consequences of selection for environmental impact traits in dairy cows using MIR data to predict CH4 emission and setting up a selection index that include MIR-based CH4 emission together with other traits of economic importance. These authors used CH4 intensity (g/kg of milk) and calculated approximate genetic correlations from estimated breeding values. Negative approximate genetic correlations were observed between CH4 intensity and milk yield (–0.67), fat yield (–0.13), protein yield (–0.46), longevity (–0.07), and average of conformation traits (–0.23). Positive approximate correlations were observed for fertility (0.31) and body condition score (0.27) in the study by Kandel et al. (2013). Based on these correlations and by putting a hypothetical 25% weight on CH4 intensity on the current Walloon genetic evaluation index and proportional reduction on other selection traits, the response to selection was a reduction of CH4 intensity by 24%, increase in milk yield by 30%, fat yield by 17%, protein yield by 29%, somatic cells score by –15%, longevity by 24%, fertility by –11%, body condition score by –13%, and conformation traits by 24%. These results suggest that a decrease of CH4 intensity could have a negative impact on the cow fertility but a positive effect on the longevity but it needs to be tested on independent data as well as on data where more CH4 observations are available.
Conclusion
Some milk fatty acids are genetically correlated with CH4 emission in dairy cattle. In this study it was mainly C13:0, C15:0 and C17:0 that showed significant genetic and genomic correlations with CH4 emission. Using a SNP-based genomic relationship matrix rather than pedigree-based relationship matrix provided estimates with lower standard errors. In small studies with limited sample size it is important to use animals that are genotyped to get more reliable estimates.
Acknowledgements
The study is financed by the Danish Milk Levy Foundation, The Danish Strategic Research Council the Danish Research Council for Independent Research | Technology and Production. Project number is 11–105913. The pedigree and data used to estimated fixed effects was provided by SEGES, Skejby, Denmark. The authors acknowledge the farmers that opened their farm and made this study possible.
References
Bouwman AC, Bovenhuis H, Visker MHPW, van Arendonk JAM (2011) Genome-wide association of milk fatty acids in Dutch dairy cattle. BMC Genetics 12, 43| Genome-wide association of milk fatty acids in Dutch dairy cattle.Crossref | GoogleScholarGoogle Scholar | 1:CAS:528:DC%2BC3MXmtlKks7k%3D&md5=713fba863960b107c03f02271140f765CAS |
Buitenhuis AJ, Sundekilde UK, Poulsen NA, Bertram HC, Larsen LB, Sørensen P (2011) Estimation of genetic parameters and detection of quantitative trait loci for metabolites in Danish Holstein milk. Journal of Dairy Science 2013, 3285–3295.
Cottle DJ, Nolan JV, Wiedemann SG (2011) Ruminant enteric methane mitigation: a review. Animal Production Science 51, 491–514.
| Ruminant enteric methane mitigation: a review.Crossref | GoogleScholarGoogle Scholar | 1:CAS:528:DC%2BC3MXntVGisLY%3D&md5=ca8409519db155f19af58311f9f4e1d4CAS |
de Haas Y, Windig JJ, Calus MPL, Dijkstra J, de Haan M, Bannink A, Veerkamp RF (2011) Genetic parameters for predicted methane production and potential for reducing enteric emissions through genomic selection. Journal of Dairy Science 94, 6122–6134.
| Genetic parameters for predicted methane production and potential for reducing enteric emissions through genomic selection.Crossref | GoogleScholarGoogle Scholar | 1:CAS:528:DC%2BC3MXhsFClsbvL&md5=ef0f4a4107c2103203bc10704fcba95cCAS |
Dehareng F, Delfosse C, Froidmont E, Soyeurt H, Martin C, Gengler N, Vanlierde A, Dardenne P (2012) Potential use of milk mid-infrared spectra to predict individual methane emission of dairy cows. Animal 6, 1694–1701.
| Potential use of milk mid-infrared spectra to predict individual methane emission of dairy cows.Crossref | GoogleScholarGoogle Scholar | 1:CAS:528:DC%2BC38Xht1Gis7zP&md5=4aa34f19d46bf8fcba0ccfb516a8a24eCAS | 23031566PubMed |
Demeyer DI, Van Nevel CJ (1975) Methanogenesis, an integrated part of carbohydrate fermentation and its control. In ‘Digestion and metabolism in the ruminant’. (Eds IW McDonald, ACI Warner) pp. 366–382. (The University of New England Publishing Unit: Armidale, NSW)
Dijkstra J, van Zijderveld SM, Apajalahti JA, Bannink A, Gerrits WJJ, Newbold JR, Perdok HB, Berends H (2011) Relationships between methane production and milk fatty acid profiles in dairy cattle. Animal Feed Science and Technology 166–167, 590–595.
| Relationships between methane production and milk fatty acid profiles in dairy cattle.Crossref | GoogleScholarGoogle Scholar |
Garnsworthy PC, Feng S, Lock AL, Royal MD (2010) Heritability of milk fatty acid composition and stearoyl-CoA desaturase indices in dairy cows. Journal of Dairy Science 93, 1743–1748.
| Heritability of milk fatty acid composition and stearoyl-CoA desaturase indices in dairy cows.Crossref | GoogleScholarGoogle Scholar | 1:CAS:528:DC%2BC3cXms1aqsrk%3D&md5=80ba94be128940008feea695981805cdCAS | 20338453PubMed |
Garnsworthy PC, Craigon J, Hernandez-Medrano JH, Saunders N (2012) Variation among individual dairy cows in methane measurements made on farm during milking. Journal of Dairy Science 95, 3181–3189.
| Variation among individual dairy cows in methane measurements made on farm during milking.Crossref | GoogleScholarGoogle Scholar | 1:CAS:528:DC%2BC38Xntlyksbk%3D&md5=6c6728c6d63911fc60254356b4774721CAS | 22612953PubMed |
Kandel PB, Vanrobays M-L, Vanlierde A, Dehareng F, Froidmont E, Dardenne P, Lewis E, Buckley F, Deighton MH, McParland S, Gengler N, Soyeurt H (2013) Genetic parameters for methane emissions predicted from milk mid-infrared spectra in dairy cows. Advances in Animal Biosciences 4, 279
Kandel PB, Vanderick S, Vanrobays M-L, Vanlierde A, Dehareng F, Froidmont E, Soyeurt H, Gengler N (2014) Consequences of selection for environmental impact traits in dairy cows. in 10th World Congress on Genetics Applied to Livestock Production (WCGALP), Vancouver, Canada.
Krag K, Janss LL, Shariati MM, Berg P, Buitenhuis AJ (2013a) SNP-based heritability estimation using a Bayesian approach. Animal 7, 531–539.
| SNP-based heritability estimation using a Bayesian approach.Crossref | GoogleScholarGoogle Scholar | 1:CAS:528:DC%2BC3sXjt1Wksbo%3D&md5=4bb2283275d039cad84df370843eab1bCAS | 23177174PubMed |
Krag K, Poulsen NA, Larsen MK, Larsen LB, Janss LL, Buitenhuis B (2013b) Genetic parameters for milk fatty acids in Danish Holstein cattle based on SNP markers using a Bayesian approach. BMC Genetics 14, 79
| Genetic parameters for milk fatty acids in Danish Holstein cattle based on SNP markers using a Bayesian approach.Crossref | GoogleScholarGoogle Scholar | 24024882PubMed |
Larsen MK, Kidmose U, Kristensen T, Beaumont P, Mortensen G (2013) Chemical composition and sensory quality of bovine milk as affected by type of forage and proportion of concentrate in the feed ration. Journal of the Science of Food and Agriculture 93, 93–99.
| Chemical composition and sensory quality of bovine milk as affected by type of forage and proportion of concentrate in the feed ration.Crossref | GoogleScholarGoogle Scholar | 1:CAS:528:DC%2BC38XnslKrsb8%3D&md5=74ee9a7478365d3183e5cc9fc389ff7bCAS | 22653610PubMed |
Lassen J, Lovendahl P, Madsen J (2012) Accuracy of noninvasive breath methane measurements using Fourier transform infrared methods on individual cows. Journal of Dairy Science 95, 890–898.
| Accuracy of noninvasive breath methane measurements using Fourier transform infrared methods on individual cows.Crossref | GoogleScholarGoogle Scholar | 1:CAS:528:DC%2BC38XhsFSmtro%3D&md5=a4f0c121293cbf9d8edcda64f1596e60CAS | 22281353PubMed |
Liu Y, Qin X, Song XZ, Jiang H, Shen Y, Durbin KJ, Lien S, Kent MP, Sodeland M, Ren Y, Zhang L, Sodergren E, Havlak P, Worley KC, Weinstock GM, Gibbs RA (2009) Bos taurus genome assembly. BMC Genomics 10, 180
| Bos taurus genome assembly.Crossref | GoogleScholarGoogle Scholar | 19393050PubMed |
Madsen P, Jensen J (2014) ‘A user’s guide to DMU, version 6, release 5.2.’ (Faculty of Agricultural Sciences, Aarhus University: Denmark) Available at http://dmu.agrsci.dk/DMU/Doc/Current/dmuv6_guide.5.2.pdf [Verified 24 November 2015]
Madsen J, Bjerg BS, Hvelplund T, Weisbjerg MR, Lund P (2010) Methane and carbon dioxide ratio in excreted air for quantification of the methane production from ruminants. Livestock Science 129, 223–227.
| Methane and carbon dioxide ratio in excreted air for quantification of the methane production from ruminants.Crossref | GoogleScholarGoogle Scholar |
Teo YY, Inouye M, Small KS, Gwilliam R, Deloukas P, Kwiatkowski DP, Clark TG (2007) A genotype calling algorithm for the Illumina BeadArray platform. Bioinformatics 23, 2741–2746.
| A genotype calling algorithm for the Illumina BeadArray platform.Crossref | GoogleScholarGoogle Scholar | 1:CAS:528:DC%2BD2sXht1KmtLvN&md5=cb49ecf818c79c9ff217e424570d39a3CAS | 17846035PubMed |
VanRaden PM (2008) Efficient methods to compute genomic predictions. Journal of Dairy Science 91, 4414–4423.
| Efficient methods to compute genomic predictions.Crossref | GoogleScholarGoogle Scholar | 1:CAS:528:DC%2BD1cXhtlajtLzO&md5=5f5d518ecbd76e274a573e8a260c7914CAS | 18946147PubMed |
Vlaeminck B, Fievez V, Tamminga S, Dewhurst RJ, van Vuuren A, de Brabander D, Demeyer D (2006) Milk odd- and branched-chain fatty acids in relation to the rumen fermentation pattern. Journal of Dairy Science 89, 3954–3964.
| Milk odd- and branched-chain fatty acids in relation to the rumen fermentation pattern.Crossref | GoogleScholarGoogle Scholar | 1:CAS:528:DC%2BD28XhtVWgsb%2FL&md5=156d3aadfd337d5d5e1e86b8652c214dCAS | 16960070PubMed |
Wilmink JBM (1987) Adjustment of lactation yield for age at calving in relation to level of production. Livestock Production Science 16, 321–334.
| Adjustment of lactation yield for age at calving in relation to level of production.Crossref | GoogleScholarGoogle Scholar |