Identification of differential duodenal gene expression levels and microbiota abundance correlated with differences in energy utilisation in chickens
Barbara M. Konsak A B D G , Dragana Stanley A D F G , Volker R. Haring A , Mark S. Geier B C D , Robert J. Hughes B C D , Gordon S. Howarth B , Tamsyn M. Crowley A D E and Robert J. Moore A D HA Australian Animal Health Laboratory, CSIRO Animal, Food and Health Sciences, Geelong, Vic. 3220, Australia.
B School of Animal and Veterinary Sciences, University of Adelaide, Roseworthy, SA 5371, Australia.
C South Australian Research and Development Institute, Pig and Poultry Production Institute, Nutrition Research Laboratory, Roseworthy, SA 5371, Australia.
D Poultry Cooperative Research Centre, PO Box U242, University of New England, Armidale, NSW 2315, Australia.
E School of Medicine, Deakin University, Geelong, Vic. 3220, Australia.
F Central Queensland University, Higher Education Division, Bruce Highway, Rockhampton, Qld, 4702.
G Made equal contributions: co-first authorship.
H Corresponding author. Email: rob.moore@csiro.au
Animal Production Science 53(12) 1269-1275 https://doi.org/10.1071/AN12426
Submitted: 13 December 2012 Accepted: 2 August 2013 Published: 1 October 2013
Journal Compilation © CSIRO Publishing 2013 Open Access CC BY-NC-ND
Abstract
Among the terrestrial production animals, chickens are the most efficient users of energy. Apparent metabolisable energy (AME) is a measure of energy utilisation efficiency representing the difference between energy consumed and energy lost via the excreta. There are significant differences in the energy utilisation capability of individual birds that have a similar genetic background and are raised under identical conditions. It would be of benefit to poultry producers if the basis of these differences could be understood and the differences minimised. We analysed duodenal gene expression and microbiota differences in birds with different energy utilisation efficiencies. Using microarray analysis, significant differences were found in duodenal gene expression between high- and low-AME birds, indicating that level of cell turnover may distinguish different groups of birds. High-throughput sequencing of bacterial 16S rRNA genes indicated that duodenal microbiota was dominated by Lactobacillus species and two operational taxonomic units, identified as lactobacilli species, were found to be more abundant (P < 0.05) in low-AME birds. The present study has identified gene expression and microbiota properties that correlate with differences in AME; further studies will be required to investigate the causal relationships.
Introduction
Feed conversion efficiency is a key consideration in efforts to supply the growing global population with high-quality protein. Chicken meat accounts for approximately one-third of the world’s meat consumption, with annual production of over 88 million tonnes (FAO 2011). Price competitiveness, mostly due to lower production costs than with other meat sources, has increased the demand for chicken meat (Henry and Rothwell 1995). This competitiveness has been enabled through genetic and nutritional advances in feed utilisation and production efficiency. Havenstein et al. (2003) highlighted these improvements by demonstrating that modern Ross 308 broilers were approximately four times larger, required three times less food to reach target weight, and had a 3-fold difference in feed conversion when compared with a 1950s strain of chicken. Knowledge and tools to facilitate further improvements will contribute to global food security. In a typical broiler-production system, all birds have similar genetics and are raised together under similar environmental conditions, with access to identical feed and water supplies. Despite these consistencies, growth performance of individual birds can be quite variable. Consistent bird performance, together with flock uniformity, is highly desired by the chicken processors and is a good indicator of the effectiveness of the production process (Toudic 2006). Poor flock uniformity results in management problems, delayed growth, poor feed conversion ratios and rejected carcasses (Toudic 2006). Improvements in flock uniformity could increase the proportion of birds that reach their target weight within a given time, and therefore reduce numbers of birds falling outside the size standards set for automated processing-plant equipment (Bedford 2001), and benefit production efficiency (Mulder et al. 2009).
Given the importance of bird performance and flock uniformity, extensive research has been undertaken investigating the effects of various dietary regimens on bird performance. The outcomes and effects have been measured and quantified in various ways such as weight gain, time to market weight and feed conversion ratio. Apparent metabolisable energy (AME) represents the efficiency of energy intake and measures the difference between energy consumed, via the feed, and energy lost, via the excreta (Farrell 1999). AME is directly influenced by chicken breed, sex, health and also nutrient availability and digestibility, and can be manipulated by nutrient composition and additives (Owens et al. 2008). Previous studies have identified considerable differences in AME values among birds fed an identical diet (Hughes and Choct 1997). In a flock of the same breed, fed an identical diet, some birds are able to extract the available nutrients more efficiently, while others are less efficient and subsequently lose a greater proportion of energy via the excreta (Hughes 2003, 2004). In poultry, as in human research, knowledge of the mechanisms underlying variation in efficient utilisation of available energy at a gene expression and microbiota level is sparse.
In the majority of nutrition studies, key components of a diet are varied and the differential response of animal or human subjects is measured to determine the effect of dietary change. In contrast, few studies have investigated the basis of differential responses of an outbred population to a single constant diet. Such work is important because it investigates the role of the individual and its underlying biology in determining the efficiency with which nutrients are taken up and assimilated. Within a population, there will be a distribution in the value of any continuous parameter that is measured; from crude measures, such as weight gain, to measures of much finer detail, such as expression level of a particular gene in a specific tissue or the abundance of particular microbes within the gut. We have previously reported that differences can be detected in the caecal microbiota of chickens with high and low AME values from a single homogenously treated flock (Stanley et al. 2013). We were then interested to determine potential differences in small-intestinal gene-expression patterns and microbiota in these birds. If significant correlations could be found, it may contribute to the understanding of the causes of variable bird performance.
Materials and methods
Birds and diet
Male Cobb 500 broilers (Baiada Hatchery, Willaston, South Australia) were raised on wood shavings in a floor-rearing pen in a temperature-controlled room until the commencement of the AME study period. All birds were given ad libitum access to a broiler grower diet previously described in Stanley et al. (2012) that met or exceeded National Research Council guidelines for broiler chickens (NRC 1994). The feed contained 44.4% of wheat, 17% soybean meal, 15% barley, 10% canola meal, 5% peas, 3.2% meat meal, 3% tallow, 1% limestone, 0.5% vitamin mix, and traces of salt, lysine HCl, DL-methionine and threonine. All procedures were approved by the Animal Ethics Committees of the University of Adelaide and the Department of Primary Industries and Resources South Australia.
Apparent metabolisable energy study
At 13 days post-hatch, 96 chickens were transferred in pairs to 48 metabolism cages located in a temperature-controlled room initially kept between 25°C and 27°C. Birds were placed in pairs for an initial acclimation period to minimise stress associated with isolation. Birds continued to have free access to food and water before, and during, the experimental period. On Day 15, birds were placed individually in 96 metabolism cages.
AME values were determined in a classical 7-day energy-balance study, commencing when birds were 15 days of age. Bodyweight was recorded at the beginning and end of the 7-day period. The first 3 days enabled the chickens to adapt to solitary confinement in the metabolism cages. During the following 4 days, all excreta were collected daily and dried at 80°C. Feed intake was recorded during the adaptation and collection phases of the study period.
On Day 22, all birds were weighed and retained in individual cages until Day 25. On Day 25 post-hatch, birds were killed by cervical dislocation. Tissue (1-cm segment) from the midpoint of the duodenum was collected, rinsed in phosphate-buffered saline and stored in a 5-mL tube containing 2 mL of RNAlater (Ambion, Austin, TX, USA). The adjacent section was used to collect mucosal scrapings for microbiota analysis. During collection, samples were stored on ice and then frozen (−20°C) for later processing to extract nucleic acids.
Gross energy (GE) values of feed were measured with a Parr isoperibol bomb calorimeter (Parr Instrument, Moline, IL, USA). Gross energy values of dried excreta were also measured and AME values (in MJ/kg DM) were calculated as follows:
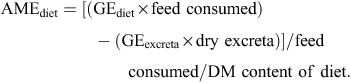
Microarray sample preparation
Total RNA was isolated from each duodenal sample by using the Meridian total RNA isolation kit (Cartagen Molecular, San Carlos, CA, USA) according to the manufacturer’s instructions. The quantity of RNA and 260 : 280 and 260 : 230 ratios were determined using a NanoDrop ND-1000 spectrophotometer (Thermo Fisher Scientific, Wilmington, DE, USA) and RNA was subsequently stored at −80°C. RNA samples that had a 260 : 280 ratio above 1.8 were used for the study. Total RNA (3 μg) was reverse-transcribed into cDNA by using oligo dT20, as per the Invitrogen Superscript III First Strand Synthesis System protocol (Invitrogen, Carlsbad, CA, USA). The cDNA labelling with Cyanine 3, hybridisation and washing was performed following NimbleGen Arrays User’s Guide protocol without modifications (http://www.nimblegen.com/downloads/support/05434505001_NG_Expression_UGuide_v6p0.pdf, verified 21 August 2013).
Microarray design
A custom designed NimbleGen 12x135K chicken high-density multiplex microarray was used in this experiment (NimbleGen design #10309). The microarray contained a set of 65 849 long oligonucleotides (60–75 nt) based on chicken UniGene sequence families. Each gene was represented by at least two independently designed probes and each probe was present in duplicate on the array. Each fabricated glass slide contained 12 identical arrays that were independently hybridised. More information on the platform can be found on the NCBI Gene Expression Omnibus under platform Accession number GPL17190 and dataset number GSE47213.
Microarray analysis
NimbleScan Software Version 2.5.26 (Roche NimbleGen) was used to extract the microarray data. The data were then analysed using Bioconductor (Gentleman et al. 2004) and several other packages, including (1) arrayQualityMetrics (Kauffmann et al. 2009) for quality control, (2) preprocessCore (Bolstad 2010) for background correction and normalising and (3) GeneFilter (Gentleman et al. 2009) for removal of low-intensity features. Limma (Smyth 2004) was initially used to identify differentially expressed genes. So as to minimise false positives, the GeneSelector package was utilised, as described by Boulesteix and Slawski (2009). Briefly, selection of differential genes can be highly influenced by the statistical method used, and the overlap of genes selected using the same significance value can be quite low. GeneSelector ranks genes on the basis of how well they perform in a selected number of statistical tests and minimises the number of false positives. Ranked lists, based on ordinary Student’s t-test, significance analysis of microarrays (Chu et al. 2000), limma parametric empirical Bayes (eBayes) testing (Smyth 2004) and fold difference were first generated for each of the criteria. Then the Markov chain model within the GeneSelector package was used to aggregate the ranked lists of all criteria into a list of genes that are significant, independent of the method used. Gene ontology (GO) analysis and data visualisation and exploration were performed using the database for annotation, visualisation and integrated discovery (Huang et al. 2009).
Microbial-DNA preparation
Twenty birds, including those with the 10 highest and 10 lowest AME values, were selected for microbiota analysis by 16S rRNA gene sequencing. Mucosal scrapings from the duodenal section of the small intestine were taken from each bird and diluted 1 : 4 with phosphate buffered saline. Total DNA was isolated using the method of Yu and Morrison (2004). Briefly, cells were lysed using lysis buffer (500 mM NaCl, 50 mM Tris–HCl pH8.0, 50 mM EDTA and 4% sodium dodecyl sulfate) and sterile zirconium beads, and by homogenising (Precellys 24 tissue homogeniser; Bertin Technologies, Montigny-le-Bretonneux, France) at the maximum instrument speed of 6500g, 6 × 10 s. Nucleic acid pellets were resuspended in Tris–EDTA buffer and digested with DNase-free RNase and proteinaseK to remove RNA and protein and purified using QIAamp columns (Qiagen, Chadstone, Vic., Australia). Quantity and quality of DNA were measured on a NanoDrop ND-1000 spectrophotometer (Thermo Fisher Scientific).
Polymerase chain reaction (PCR) amplification of 16S rRNA gene sequences
DNA was amplified using Bio-Rad iProof DNA polymerase. Each PCR reaction contained 2 µL of template DNA, 20 µL of iProof 2X master mix (containing buffer, nucleotides and iProof enzyme), 2 µL of each primer (final concentration 0.5 µM), and 14 µL of water. The primers used were selected to amplify the V1–V3 region of the 16S rRNA gene (forward primer (Lane 1991), 5′ AGAGTTTGATCCTGG 3′; reverse primer, a truncated version of W31 (Snell-Castro et al. 2005), 5′ TTACCGCGGCTGCT 3′) and contained additional sequences for the pyrosequencing process, including sequence barcodes (Roche/454 extended barcode set, 454 Life Sciences, Branford, CT, USA) that allowed each sample to be identified from the pools used for sequencing. Amplification of products was performed in an Eppendorf Mastercycler (Eppendorf South Pacific, North Ryde, NSW, Australia) using the following conditions: 98°C for 60 s then 25 cycles of 98°C for 5 s, 40°C for 30 s, 72°C for 30 s, elongation at 72°C for 10 min, then held at 5°C. The yield of PCR amplification of each sample was assessed by running 10 µL of the PCR mix on a 1.2% agarose gel.
High throughput sequencing and analysis of 16S rRNA gene amplicons
The amplified 16S rRNA gene samples from each bird were pooled using equivalent amounts of each PCR product. The pooled sample was sequenced using the Roche/454 FLX Genome Sequencer (454 Life Sciences) according to the manufacturer’s instructions. Sff files were split into fasta and qual files using PyroBayes (Quinlan et al. 2008) and data were analysed using Mothur v1.12 (Schloss et al. 2009), Qiime v1.0.0 software (Caporaso et al. 2010b) and ARB (Ludwig et al. 2004).
Pre-processing undertaken in Mothur included qual file processing (average quality of 25) and removal of Pintail detected chimeric sequences. The fasta file, with chimeras removed, was then used for further analysis in Qiime v1.5 software using the suggested Qiime default settings. Further quality filtering selected sequences between 300 and 600 nucleotides, allowed a maximum homopolymer run of six nucleotides and no ambiguous bases. In total, 176 819 sequences remained after quality trimming, with a mean length of 565 nucleotides and mean sample size of 8840 sequences. A filter was applied to eliminate operational taxonomic units (OTUs) represented with less than 10 sequences or present in fewer than five samples. This resulted in the identification of 152 OTUs using 97% sequence similarity. The phylogeny of representative sequences from the OTUs was assessed by comparison to the EzTaxon (Chun et al. 2007) database. The statistical tools used to assess microbiota differences between high- and low-AME birds were used from within Qiime and are detailed with the results.
Results
Microarray
Birds were selected for microarray analysis on the basis of AME values; a Student’s t-test indicated that the six highest and six lowest AME-ranked birds were significantly (P = 7.82e–4) different. Feed conversion ratio, bodyweight gain and feed intake were not significantly different between high- and low-AME birds.
Poor hybridisation results with the sample from the 6th lowest AME bird meant that it had to be excluded from the analysis, leaving six arrays for high and five for low birds. The Genefilter package was used to remove features that had intensity values near background in at least 8 of the 10 arrays. eBayes testing (Fig. S1, available on the journal website as Supplementary Material for this paper) reported that 428 genes were more highly expressed in the high-AME birds and 431 genes were more highly expressed in the low-AME birds (P < 0.01, over 2-fold difference). Only 20 genes were more highly expressed in high- and 26 in low-AME samples with higher than a 3-fold difference. The highest-fold difference across all genes was 3.8-fold increase in the low-AME birds for the unannotated Unigene Gga.24220 (Table S1, available on the journal website as Supplementary Material to this paper). The GeneSelector package was used to identify genes recognised as differentially expressed across a range of statistical analysis methods. The overlap of significance using the different tests is presented in Fig. 1. This resulted in more than 2-fold higher expression of 360 genes in low-AME birds (Table S2, available on the journal website as Supplementary Material to this paper) and 325 genes in high-AME birds (Table S3, available on the journal website as Supplementary Material to this paper), with the lowest ranked gene scoring P-values of 0.0043, 0.0102 and 0.0012 for eBayes, significance analysis of microarrays and Student’s t-test, respectively (Table S1).
![]() |
The database for annotation, visualisation and integrated discovery was used to identify GO categories statistically more abundant in differentially expressed genes than expected by chance. A major issue in analysing chicken expression data is the low level of annotation for the chicken genome. Among the genes more highly expressed in low-AME birds, only 35% had functional annotations. On the basis of the annotations available, this group of genes was enriched in GO categories involved mainly in regulation of transcription and basic cell maintenance. GO clusters and categories are given in Tables S2–S3. The genes more highly expressed in high-AME birds were similarly poorly annotated and only 38.4% had useful functional annotations. GO analysis of the annotated genes indicated enrichment only of general cell maintenance-related GO categories. For both groups of genes, the lowest P-value for any GO category was ~0.003; however, the lowest Bonferoni corrected P-values were very high, 0.37 and 0.71, when inspecting genes more highly expressed in low- and high-AME birds, respectively. The 43 genes that had the highest expression differences, between 3 and 3.8, were mostly unannotated.
Microbiota analysis
Birds were selected for microbiota analysis on the basis of AME values; a Student’s t-test indicated that the 10 highest and 10 lowest AME-ranked birds were significantly (P = 7.74e–6) different. These groups included all the birds used for the microarray analysis, plus four additional birds in each class, added to increase statistical power of the analysis. Inspection of α-diversity plots indicated that depth of sequencing gave good sample coverage and showed very low diversity and strong dominance in the microbial community of the duodenum, with statistically significant differences in several α-diversity measures (Fig. S2, available on the journal website as Supplementary Material to this paper). Relatively low diversity compared with other sections of the chicken gut is supported by the total dominance of members of the Firmicutes phylum (99.37% of total sequences), Lactobacillaceae (99.21%) dominance on a family level and Lactobacillus (99.07%) dominance on a genus level. The 27 OTUs with abundance higher than 0.1% of total sequences represented 97% of all sequences identified in the duodenal samples and were identified as Lactobacillus salivarius (47.4%), L. crispatus (34.8%), L. reuteri (11.7%), L. johnsonii (2.3%) and L. taiwanensis (0.7%). These were classified with more than 95% sequence similarity to type strains catalogued in the EzTaxon database (Table S4, available on the journal website as Supplementary Material to this paper). Representative sequences have been uploaded to the European Nucleotide Archive (Accession numbers HG314125–HG314153).
A principal coordinate analysis plot (Fig. 2) based on Weighted UniFrac results showed the relationship among samples and suggested two disparate groupings within the high-AME group. ANOVA between high- and low-AME birds detected only two OTUs differentially abundant between the two groups. The two differential OTUs (Table S5, available on the journal website as Supplementary Material to this paper); OTU6 (P-value 0.02) and OTU11 (P-value 0.04) were 2- and 2.5-fold more abundant, respectively, in low-AME birds. Qiime RDP classifier identified both OTUs as Lactobacillus. To further classify, sequences were submitted to EzTaxon and in separate analysis placed in the ARB Greengenes database tree (Fig. 3); EzTaxon placed OTU6 as closest to L. salivarius (EzTaxon similarity to Type strain ATCC 11741(T) was 95.85%) and OTU11 closest to L. crispatus (EzTaxon similarity to Type strain DSM 20584(T) was 96.15%). In ARB, we compared the sequences to all culturable Lactobacillus strains and placed them, with 100% sequence similarity, to L. crispatus str. LAB32 (OTU 11) and L. salivarius str. C22 (OTU6).
![]() |
![]() |
Discussion
In the present study, birds were raised under identical conditions with access to the same feed, water and environmental conditions. As previous studies have reported that AME is influenced by many factors (Owens et al. 2008), in the present study, we investigated the additional factors of the duodenal microbiota and duodenal gene expression to determine whether correlations could be found with the efficiency with which birds retained energy from food. The multi-test analysis performed within the GeneSelector package indicated differential expression of several thousand genes in the duodenum between the high- and low-AME birds. The expression differences were subtle, with only a few hundred genes with fold changes of more than 2 and only 46 genes with differential expression levels over 3-fold. The paucity of functional annotations currently available for the chicken genome meant that only limited information was available for the differentially expressed genes. The only gene ontology groups that were over-represented among the greater than 2-fold differentially expressed genes were those relating to regulation of transcription and basic cell maintenance. This suggested that the basis for differential gene expression in the birds with high AME values is related to a higher rate of cell turnover and renewal within the duodenum rather than differential expression of genes encoding digestive functions such as digestive enzymes or nutrient transporters. It is of course possible that there are genes that are directly involved in nutrient and energy capture represented in the greater than 60% of differentially expressed genes for which no useful functional annotation was available.
The observed correlations between differential expression of some genes and the efficiency with which chickens can retain energy provide the impetus for further studies of the causal links between gene expression and bird performance. In further studies, it would be interesting to determine whether the genes found to be differentially expressed in the duodenum are also differentially expressed in other sections of the GIT, or whether other genes are of importance in other sections of the gut. Given that the differences between high- and low-AME birds appeared to be related to general cell turnover, it is perhaps likely that this would be a common feature along the entire length of the digestive tract. One motivation in carrying out this research was to determine whether there are ways in which birds could be manipulated or selected to be as efficient as possible at retaining energy. If there is more than just a correlation, but rather a causal link between differences in gene expression and energy efficiency, then it will be important to understand the basis of the subtle underlying differences in gene expression. Are the differences in gene expression established by the genetics of the birds or are they directly influenced by the gut environment, for example, the exact composition of feed or the microbiota that establishes in the gut?
In the current study, the duodenum-associated microbiota was dominated by lactobacilli, comprising up to 99% of the sequences in some birds. Statistical analysis revealed two OTUs, OTU6 and OTU11, both members of the Lactobacillus family, to be more abundant in low-AME birds. They were identified as most closely related to L. salivarius and L. crispatus, respectively, on the basis of homology to characterised strains represented in the database. Other OTUs identified as closely related to L. salivarius and L. crispatus were not identified as differentially abundant between high- and low-AME birds. Previously, Torok et al. (2008) reported correlations between the composition of ileal and caecal microbiota and AME values. Accordingly, it appears that the microbiota across the small intestine and the caecum may influence or be influenced by the efficiency of energy retention.
AME, as a measure of an animal’s ability to efficiently extract energy from the feed, is an important consideration in directing the formulation of low-cost high-efficiency feed. Improving animal performance has long been a priority in agricultural research and has resulted in massive productivity improvements over several decades. The present study identified aspects of duodenal gene expression and microbiota that correlate with differences in the efficiency of energy retention. The data indicated correlations but could not infer any causation. As such, it is not yet apparent whether the manipulation of gene expression or microbiota composition could have beneficial impacts on AME; this awaits further experimental work.
Acknowledgements
This work was partly conducted within the Poultry CRC, established and supported under the Australian Government’s Cooperative Research Centres Program. Professor Gordon Howarth is supported by the Sally Birch Cancer Council Australia Senior Research Fellowship in Cancer Control. We thank D. Schultz, E. Daniels and K. Swanson from SARDI, for assistance with animal experimentation. Thanks go to Dr J. Wynn and Dr M. Adams for helpful suggestions regarding the manuscript. The data analysis was performed at the National Computational Infrastructure Specialised Facility in Bioinformatics (NCISF), hosted by a consortium led by the University of Queensland.
References
Bedford M (2001) Enzymes improve broiler body weight uniformity for increased profitability. World Poultry 17, 16–19.Bolstad, BM (2010) ‘preprocessCore: a collection of pre-processing functions. R package, version 1.20.0.’ Available at http://www.bioconductor.org/packages//2.10/bioc/html/preprocessCore.html [Verified 23 August 2013]
Boulesteix AL, Slawski M (2009) Stability and aggregation of ranked gene lists. Briefings in Bioinformatics 10, 556–568.
| Stability and aggregation of ranked gene lists.Crossref | GoogleScholarGoogle Scholar | 1:CAS:528:DC%2BD1MXhtVamu7bN&md5=f99b9c858e714b2f1a88aab652fc1fd8CAS | 19679825PubMed |
Caporaso JG, Bittinger K, Bushman FD, DeSantis TZ, Andersen GL, Knight R (2010a) PyNAST: a flexible tool for aligning sequences to a template alignment. Bioinformatics 26, 266–267.
| PyNAST: a flexible tool for aligning sequences to a template alignment.Crossref | GoogleScholarGoogle Scholar | 1:CAS:528:DC%2BC3cXntlGqug%3D%3D&md5=9b0864a16cf2e1f9d6c50796d3e652b4CAS | 19914921PubMed |
Caporaso JG, Kuczynski J, Stombaugh J, Bittinger K, Bushman FD, Costello EK, Fierer N, Pena AG, Goodrich JK, Gordon JI, Huttley GA, Kelley ST, Knights D, Koenig JE, Ley RE, Lozupone CA, McDonald D, Muegge BD, Pirrung M, Reeder J, Sevinsky JR, Turnbaugh PJ, Walters WA, Widmann J, Yatsunenko T, Zaneveld J, Knight R (2010b) Qiime allows analysis of high-throughput community sequencing data. Nature Methods 7, 335–336.
| Qiime allows analysis of high-throughput community sequencing data.Crossref | GoogleScholarGoogle Scholar | 1:CAS:528:DC%2BC3cXksFalurg%3D&md5=30357f63b4c045af34688e2e3cec0730CAS | 20383131PubMed |
Chu G, Goss V, Narasimhan B, Tibshirani R (2000) ‘SAM, significance analysis of microarrays. User guide and technical documentation.’ (Stanford University: Stanford, CA)
Chun J, Lee JH, Jung Y, Kim M, Kim S, Kim BK, Lim YW (2007) EzTaxon: a web-based tool for the identification of prokaryotes based on 16S ribosomal RNA gene sequences. International Journal of Systematic and Evolutionary Microbiology 57, 2259–2261.
| EzTaxon: a web-based tool for the identification of prokaryotes based on 16S ribosomal RNA gene sequences.Crossref | GoogleScholarGoogle Scholar | 1:CAS:528:DC%2BD2sXhsVanu73P&md5=54a3adbcdb09144d77ca442c761a4449CAS | 17911292PubMed |
FAO (2011) ‘World livestock 2011 – livestock in food security.’ (FAO: Rome) Available at http://www.fao.org/docrep/014/i2373e/i2373e.pdf [Verified 20 August 2013]
Farrell DJ (1999) In vivo and in vitro techniques for the assessment of the energy content of feed grains for poultry: a review. Australian Journal of Agricultural Research 50, 881–888.
| In vivo and in vitro techniques for the assessment of the energy content of feed grains for poultry: a review.Crossref | GoogleScholarGoogle Scholar |
Gentleman RC, Carey VJ, Bates DM, Bolstad B, Dettling M, Dudoit S, Ellis B, Gautier L, Ge Y, Gentry J, Hornik K, Hothorn T, Huber W, Iacus S, Irizarry R, Leisch F, Li C, Maechler M, Rossini AJ, Sawitzki G, Smith C, Smyth G, Tierney L, Yang JY, Zhang J (2004) Bioconductor: open software development for computational biology and bioinformatics. Genome Biology 5, R80
| Bioconductor: open software development for computational biology and bioinformatics.Crossref | GoogleScholarGoogle Scholar | 15461798PubMed |
Gentleman RC, Carey VJ, Huber W, Hahne F (2009) ‘Genefilter: methods for filtering genes from microarray experiments. R Package Version 1.24.2., Bioconductor 2.6.’ Available at http://bioc.ism.ac.jp/2.6/bioc/html/genefilter.html. [Verified 20 August 2013]
Havenstein G, Ferket P, Qureshi M (2003) Growth, livability, and feed conversion of 1957 versus 2001 broilers when fed representative 1957 and 2001 broiler diets. Poultry Science 82, 1500–1508.
Henry R, Rothwell G (1995) ‘The world poultry industry. IFC Global agribusiness series.’ (World Bank: Washington DC)
Huang DW, Sherman BT, Lempicki RA (2009) Systematic and integrative analysis of large gene lists using DAVID bioinformatics resources. Nature Protocols 4, 44–57.
| Systematic and integrative analysis of large gene lists using DAVID bioinformatics resources.Crossref | GoogleScholarGoogle Scholar | 1:CAS:528:DC%2BD1cXhsFCkurnI&md5=8fa4b4df3f538c76cbc23c3e24cfd22aCAS |
Hughes R (2003 ) Sex and the single chicken. Australian Poultry Science Symposium 15 172 –176
Hughes R (2004 ) The rate of passage of digesta influences energy metabolism in broiler chickens. Australian Poultry Science Symposium 16 63 –66
Hughes R Choct M (1997 ) Low-ME wheat or low-ME chickens? Highly variable responses by birds on the same low-ME wheat diet. Australian Poultry Science Symposium 9 138 –141
Kauffmann A, Gentleman R, Huber W (2009) arrayQualityMetrics – a bioconductor package for quality assessment of microarray data. Bioinformatics 25, 415–416.
| arrayQualityMetrics – a bioconductor package for quality assessment of microarray data.Crossref | GoogleScholarGoogle Scholar | 1:CAS:528:DC%2BD1MXhtlOlsrw%3D&md5=61d363b00e0eb8316f536b969ab0fe8dCAS | 19106121PubMed |
Lane DJ (1991) 16S and 23S rRNA sequencing. In ‘Nucleic acid techniques in bacterial systematics’. (Eds E Stackebrandt, M Goodfellow) pp. 115–175. (Wiley: Chichester, UK)
Ludwig W, Strunk O, Westram R, Richter L, Meier H, Yadhukumar , Buchner A, Lai T, Steppi S, Jobb G, Forster W, Brettske I, Gerber S, Ginhart AW, Gross O, Grumann S, Hermann S, Jost R, Konig A, Liss T, Lussmann R, May M, Nonhoff B, Reichel B, Strehlow R, Stamatakis A, Stuckmann N, Vilbig A, Lenke M, Ludwig T, Bode A, Schleifer KH (2004) ARB: a software environment for sequence data. Nucleic Acids Research 32, 1363–1371.
| ARB: a software environment for sequence data.Crossref | GoogleScholarGoogle Scholar | 1:CAS:528:DC%2BD2cXhvFSqu7k%3D&md5=b35f94c7f3f34d214786c7ab8782b4d6CAS | 14985472PubMed |
Mulder HA, Hill WG, Vereijken A, Veerkamp RF (2009) Estimation of genetic variation in residual variance in female and male broiler chickens. Animal 3, 1673–1680.
| Estimation of genetic variation in residual variance in female and male broiler chickens.Crossref | GoogleScholarGoogle Scholar | 1:STN:280:DC%2BC38vptVynsg%3D%3D&md5=ccfe88917f7c10f21ed103eebbb4e983CAS | 22443551PubMed |
NRC (1994) ‘Nutrient requirements of poultry.’ (National Academic Press: Washington, DC)
Owens B, Tucker L, Collins M, McCracken K (2008) Effects of different feed additives alone or in combination on broiler performance, gut microflora and ileal histology. British Poultry Science 49, 202–212.
| Effects of different feed additives alone or in combination on broiler performance, gut microflora and ileal histology.Crossref | GoogleScholarGoogle Scholar | 1:CAS:528:DC%2BD1cXksFaqurk%3D&md5=40f8464345892393bdd99115ad926133CAS | 18409095PubMed |
Quinlan AR, Stewart DA, Stromberg MP, Marth GT (2008) Pyrobayes: an improved base caller for SNP discovery in pyrosequences. Nature Methods 5, 179–181.
| Pyrobayes: an improved base caller for SNP discovery in pyrosequences.Crossref | GoogleScholarGoogle Scholar | 1:CAS:528:DC%2BD1cXhsV2ksLo%3D&md5=5a4c66e47f773b29a2e87142e02bc04aCAS | 18193056PubMed |
Schloss PD, Westcott SL, Ryabin T, Hall JR, Hartmann M, Hollister EB, Lesniewski RA, Oakley BB, Parks DH, Robinson CJ, Sahl JW, Stres B, Thallinger GG, Van Horn DJ, Weber CF (2009) Introducing mothur: open-source, platform-independent, community-supported software for describing and comparing microbial communities. Applied and Environmental Microbiology 75, 7537–7541.
| Introducing mothur: open-source, platform-independent, community-supported software for describing and comparing microbial communities.Crossref | GoogleScholarGoogle Scholar | 1:CAS:528:DC%2BC3cXis1yltw%3D%3D&md5=b5fe3ac0c05235e886f325af78b7d4e1CAS | 19801464PubMed |
Smyth GK (2004) Linear models and empirical bayes methods for assessing differential expression in microarray experiments. Statistical Applications in Genetics and Molecular Biology 3, Article3
| Linear models and empirical bayes methods for assessing differential expression in microarray experiments.Crossref | GoogleScholarGoogle Scholar | 16646809PubMed |
Snell-Castro R, Godon JJ, Delgenes JP, Dabert P (2005) Characterisation of the microbial diversity in a pig manure storage pit using small subunit rDNA sequence analysis. FEMS Microbiology Ecology 52, 229–242.
| Characterisation of the microbial diversity in a pig manure storage pit using small subunit rDNA sequence analysis.Crossref | GoogleScholarGoogle Scholar | 1:CAS:528:DC%2BD2MXisVCrtbk%3D&md5=f40f80c00d94db3d3f541721338ae87bCAS | 16329909PubMed |
Stamatakis A, Hoover P, Rougemont J (2008) A rapid bootstrap algorithm for the RAxML web servers. Systems Biology 57, 758–771.
| A rapid bootstrap algorithm for the RAxML web servers.Crossref | GoogleScholarGoogle Scholar |
Stanley D, Denman SE, Hughes RJ, Geier MS, Crowley TM, Chen H, Haring VR, Moore RJ (2012) Intestinal microbiota associated with differential feed conversion efficiency in chickens. Applied Microbiology and Biotechnology 96, 1361–1369.
| Intestinal microbiota associated with differential feed conversion efficiency in chickens.Crossref | GoogleScholarGoogle Scholar | 1:CAS:528:DC%2BC38Xhs1ektL7P&md5=7922fe153cb425bb8500d4e3eb85ed82CAS | 22249719PubMed |
Stanley D, Geyer MS, Denman SE, Haring VR, Crowley TM, Hughes RJ, Moore RJ (2013) Identification of chicken intestinal microbiota correlated with the efficiency of energy extraction from feed. Veterinary Microbiology 164, 85–92.
| Identification of chicken intestinal microbiota correlated with the efficiency of energy extraction from feed.Crossref | GoogleScholarGoogle Scholar | 23434185PubMed |
Torok VA, Ophel-Keller K, Loo M, Hughes RJ (2008) Application of methods for identifying broiler chicken gut bacterial species linked with increased energy metabolism. Applied and Environmental Microbiology 74, 783–791.
| Application of methods for identifying broiler chicken gut bacterial species linked with increased energy metabolism.Crossref | GoogleScholarGoogle Scholar | 1:CAS:528:DC%2BD1cXhvVWjsr0%3D&md5=9ad23b085c83cf2927e07355256cfe35CAS | 18065621PubMed |
Toudic C (2006) ‘Evaluating uniformity in broilers – factors affecting variation. Hubbard Technical Bulletin.’ Available at http://www.hubbardbreeders.com/bulletins/index.php?id=28. [Verified 30 May 2013]
Yu Z, Morrison M (2004) Improved extraction of PCR-quality community DNA from digesta and fecal samples. BioTechniques 36, 808–812.