Modification of the Rothermel model parameters – the rate of surface fire spread of Pinus koraiensis needles under no-wind and various slope conditions
Daotong Geng A , Guang Yang A * , Jibin Ning A , Ang Li B * , Zhaoguo Li A , Shangjiong Ma A , Xinyu Wang A and Hongzhou Yu
A
B
Abstract
The prediction accuracy for the rate of surface fire spread varies in different regions; thus, increasing the prediction accuracy for local fuel types to reduce the destructive consequences of fire is critically needed.
The objective of this study is to improve the Rothermel model’s accuracy in predicting the ROS for surface fuel burning in planted forests of Pinus koraiensis in the eastern mountains of north-east China.
Fuel beds with various fuel loads and moisture content was constructed on a laboratory burning bed, 276 combustion experiments were performed under multiple slope conditions, and the ROS data from the combustion experiments were used to modify the related parameters in the Rothermel model.
The surface fire spread rate in Pinus koraiensis plantations was directly predicted using the Rothermel model but had significant errors. The Rothermel model after modification predicted the following: MRE = 25.09%, MAE = 0.46 m min−1, and R2 = 0.80.
The prediction accuracy of the Rothermel model was greatly enhanced through parameter tuning based on in-lab combustion experiments
This study provides a method for the local application of the Rothermel model in China and helps with forest fire fighting and management in China.
Keywords: fuel loads, fuel moisture, modified parameters, Pinus koraiensis, ROS, Rothermel model, slope, surface fire.
Introduction
The frequency and intensity of forest fires have increased significantly in recent years due to human activity and global climate change (Bowman et al. 2017; Xu et al. 2020; Yu et al. 2020; Abram et al. 2021), with significant harm to human health and safety, wildlife, biodiversity, and ecosystems and substantial effects on the environment, economy, and society (Driscoll et al. 2010; Johnston et al. 2012; Dittrich and McCallum 2020; Filkov et al. 2020; Koopmans et al. 2020; Vaiciulyte et al. 2021). Forest fire behaviour characteristics refer to all characteristics and actions exhibited by forest fires, including fuel ignition, flame growth, fire spread, and extinction. The fire source, fuel, weather, and slope combination influence these characteristics and behaviours according to the spatial location of occurrence (Canadian Interagency Forest Fire Center 2003; Benali et al. 2016; Finney et al. 2021). Forest fires can be divided into underground fires, surface fires, and canopy fires (Xue et al. 2022). Low-intensity surface fires can become specific fire behaviours, such as high-energy crown fires, under conditions promoting fire spread. Large tracts of forest can be burned by quickly spreading, high-energy fires in a short amount of time (Manzello 2020). The primary type of forest burning and stage of most forest fires are surface fires. The rate of spread is the most critical indicator of the behavioural characteristics of forest fires. The rate of surface fire spread (ROS) needs to be accurately estimated to reduce the severe effects of forest fires (Gould and Sullivan 2020).
Fire specialists have developed various models to forecast the rate of surface fires that have spread over the past 100 years. Four categories of predictive models were developed and sorted based on how they were created: (1) physical; (2) quasi-physical; (3) empirical; and (4) quasi-empirical (Weber 1991a, 1991b; Sullivan 2009a, 2009b). The physical model considers the physical and chemical changes in combustion and heat transfer processes. In contrast, the quasi-physical model is based solely on heat transmission and considers only the physical processes. The physical and quasi-physical models have the drawback of requiring the input of numerous parameters, and the majority of these parameters cannot be measured at the fire site. These models also have high computational, data, and resource requirements. Therefore, the physical and quasi-physical models are not usually used as tools for forest fire management. The empirical model uses data from local forest fires, prescribed burning, and laboratory combustion experiments, as well as a model created by statistical analysis of the rate of fire spread under various fuel, weather, and slope conditions, completely disregarding the physical and chemical changes that occur during fire spread; while the quasi-empirical model serves as the foundation for many globally used fire spread prediction systems, is framed in the physical conservation of energy, incorporates data from natural fires and laboratory combustion experiments, and uses statistical methods to integrate physical elements with historical fire data organically. However, regardless of the prediction model employed, the effectiveness of the model in its prediction of the fire spread rate is highly important (Cruz and Alexander 2013). Fire scholars have studied the accuracy of fire spread prediction models and found that the model’s applicability, the internal accuracy of the model relationships, and the dependability of the input data are the leading causes of model prediction errors (Albini 1976a; Keane and Reeves 2011; Alexander and Cruz 2013). Model applicability is defined as the degree to which the model accurately predicts fire behaviour (primarily ROS). All current fire spread prediction models simulate wildfires, and the fire behaviour predicted by these models differs somewhat from the actual fire behaviour of wildfires. The Rothermel model used to forecast fire behaviour in the northern Rocky Mountains, USA contained 18 key assumptions (Rothermel 1991) . These include the notions that the fuel mixture is continuous and homogenous, there are no distances between fuels, and ash from flying fires is not considered. In this instance, significant error in ROS prediction could occur. The fire spread prediction error is strongly affected by the internal accuracy of the model relationships and the dependability of the input data (Anderson 1982; Salvador et al. 2001; Fernandes 2009). This primarily occurs because the model parameters (fuel density, moisture, weather, and terrain conditions) are typically fixed and measured in a laboratory or on controlled fires. Additionally, erroneous observations, imprecise fuel estimates, or irrational assumptions can result in significant model prediction errors during actual wildfires.
The Rothermel model is the most popular quasi-empirical model (Alibini 1976a; Keane and Reeves 2011; Alexander and Cruz 2013). The work of Fons (1946) and Byram (1966) is based on the equation described by Frandsen (1971) for thermal balance. Information from Australian grassland fires and laboratory combustion experiments was used to match fire behaviour to measured input variables. Since then, the Rothermel model has undergone modifications and extensions from associated academics, and it now serves as the foundation for the BehavePlus (Andrews 2007) and FARSITE (Finney 1998). Even though the Rothermel model is currently widely used, it still has certain limitations when predicting the spread rate for various fuel types, slopes, and wind speeds (Jimenez et al. 2008; Thompson and Calkin 2011). To simplify the calculations, Rothermel (1972) treated the fuel as a homogeneous bed over a small area and a short period. Nonetheless, in a laboratory setting with a reproducible fuel bed and stable environmental conditions, Catchpole et al. (1993, 1998) reported up to 20% unexplained variation in ROS. The Rothermel model has different abilities for different combustible plant types, with moderate prediction errors for herbaceous and shrub spread rates and more significant prediction errors for harvesting trails and forest understorey combustible spread rates, according to the review of 29 published papers assessing the accuracy of the Rothermel model by Cruz et al. (2018a). Benali et al. (2016) used FARSITE to forecast eight wildfires in Portugal. They discovered that variables including fuel type, weather, and the location of fire initiation, significantly impacted how accurately FARSITE predicted the rate of fire spread. Dupuy et al. (2011) used the Rothermel model to predict fires under different bed widths and slope conditions and reported that the predicted values were 30% lower than the observed values when the bed width was 1 m at a slope of 30°. Andrews et al. (2013, 2018) reported that the addition of wind limitations to the Rothermel model and the use of the same slope parameters for all combustibles could significantly affect the prediction accuracy.
Numerous researchers have employed mathematical techniques to quantify the uncertainty in the Rothermel model’s input parameters to increase the model’s precision in predicting spreading rates and simplify the model. Bachmann and Allgöwer (2002) analysed the Rothermel model using a first-order Taylor series and reported that the uncertainty in the input parameters had very significant impacts on the prediction error. Salvador et al. (2001) performed global sensitivity and scale effects analyses on the Rothermel model, the fuel low-heating value, particle density, and mineral content had negligible effects on the model prediction error. In contrast, all other input variables significantly impacted the prediction error. Using sensitivity inverse augmented sampling, Jimenez et al. (2008) researched how the uncertainty of the Rothermel model’s parameters affects model prediction results. Although it is computationally possible to use mathematical techniques to calculate the impact of uncertainty in the Rothermel model’s input parameters, Andrews (2014) suggested that progress in the model should be made in terms of fuel properties, fuel moisture content, and improvements to the model using data from natural fires, in-lab combustion experiments, and other sources, to improve the prediction accuracy of the model for different fire scenarios. Based on these findings, several the Rothermel model parameters were improved in this work by utilising data on the fire spread rate collected from combustion tests in a laboratory combustion bed to increase the applicability of the Rothermel model.
Planted forests are an essential component of forest ecosystems. China currently holds the top spot globally, with 69.33 million hm2 of planted forests (State Forestry Administration 2014). The structure of Chinese plantation forests is simple, the main silvicultural species are mostly pure coniferous forests rich in oil and grease, the surface fuel is the most homogeneous bed, the ability to resist natural disasters is low, and a massive risk of forest fire is present (Ning et al. 2023). Pinus koraiensis is a vital plantation tree with high timber value in northern China. However, because of its high withering volume and large amount of oil it contains, forest fires can cause significant losses (Zhang and Sun 2020). Some parameters in the Rothermel model were modified using the P. koraiensis ROS measured in the laboratory to increase the prediction accuracy of the Rothermel model in predicting the ROS for surface fuel in P. koraiensis plantation forests. Our findings could serve as basic data for fire researchers, a basis for further research into the science of forest fires, and a tool for firefighters in assessing the severity of a fire and ensuring public safety. It also provides valuable information for developing China’s specific fire behaviour prediction system.
Materials and methods
The study area is the Maoershan Experimental Forestry Farm of Northeast Forestry University, Shangzhi city, Heilongjiang Province, China (45°14′–45°29′N, 127°29′–127°44′E); this is a branch of the Changbai Mountain System and has an average elevation of approximately 300 m. The area is mainly composed of low-altitude hilly and gently sloping terrain with 85% forest cover. Influenced by the Eurasian continental monsoon climate, the region has a temperate climate with an average annual temperature of 2.8°C and an average annual precipitation of approximately 723.8 mm. The existing vegetation is a natural secondary forest and plantation formed by anthropogenic disturbance of the broadleaf Pinus koraiensis forest, which is the top zone vegetation, and the main tree species are P. koraiensis, Quercus mongolica, Betula platyphylla, Larix gmelinii and Juglans mandshurica.
In August 2022, 12 standard plantation plots (30 m × 30 m) of P. koraiensis were set up in the experimental forest. Each tree in the plots were examined to determine diameter at breast height (DBH), tree height, and crown width. Five 1-m × 1-m quadrats were established (four in corners and one in the centre) in each subplot for the fuel load survey (the basic information of the sample site is in Table 1). Many P. koraiensis apoplastic leaves were collected in the forest by destructive sampling and returned to the laboratory for ventilation and preservation in preparation for the burning experiments.
Stand information | Maximum value | Minimum value | Mean value | s.d. | |
---|---|---|---|---|---|
Diameter at breast high (cm) | 21.8 | 15.3 | 18.9 | 2.3 | |
Tree height (m) | 14.6 | 9.7 | 12.2 | 1.3 | |
Crown length (m) | 8.6 | 4.6 | 6.5 | 1.4 | |
Crown width (m) | 2.9 | 1.7 | 2.2 | 0.4 | |
Density | 1633.0 | 650.0 | 1064.8 | 329.5 | |
Fuel loads (kg m−2) | 1.5 | 0.5 | 1.0 | 0.3 |
Combustion experiments
The Forest Fire Behaviour Laboratory of Northeast Forestry University is semi-open, 20 m long, 10 m wide, and 8 m high, with a roof equipped with passive exhaust devices. The combustion experiments were conducted in a 4-m long and 1.7-m wide variable slope burning bed with a slope variation range of 0°–40° and a 4-m long, 1.3-m wide area available for combustion (Fig. 1).
(a) Diagram of the experimental device and (b) experimental process (fuel load, 1.2 kg m−2; slope angle, 30°; FMC, 10%).
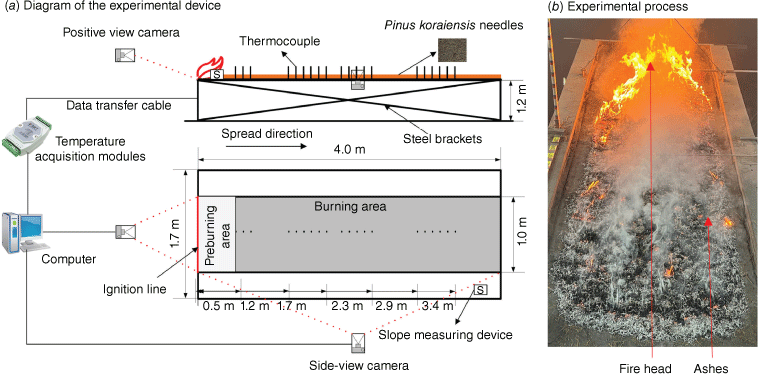
The combustion experiments were carried out in north-eastern China during the fall fire season from September to October 2022 to better meet the needs of forest fire science research. The absolute dry mass of the fuel per unit area is called the fuel load. The fuel load in this experiment was set to 0.4, 0.8, 1.2 and 1.6 kg m−2, with four levels.
Pre-experiments indicated that when the fuel moisture content of P. koraiensis fuel was 20%, open flame combustion of the fuel was difficult to sustain, and a smouldering phenomenon occurred. The Rothermel (1972) was established for the open flame condition of fuel. The fuel moisture content was set to 5, 10, and 15%, for a total of three levels, to maintain the fuel in the open flame combustion condition. The dry fuel weight and moisture needed for various fuel loads and moisture contents were calculated using the fuel moisture content calculation formula (Ning et al. 2022). The quantitative moisture was then quickly and evenly sprayed onto the fuel surface using a spray bottle and then immediately transferred to a sealed box for 24 h to allow the moisture to be fully absorbed. The preparation for the fuel’s moisture content was finished at that point. However, during the experiments, the fuel still absorbed a small amount of water from the air while configuring the fuel moisture content. Moreover, a small amount of unabsorbed water was present on the inner surface of the sealed box; thus, the wet weight of the fuel was determined before each experiment to calculate the actual fuel moisture content (Table 2). The actual fuel moisture content data were utilised for Rothermel model prediction, while the preset fuel moisture content was used for graphical plotting in the text for description.
Preset moisture content (%) | Maximum value | Minimum value | Mean value | s.d. | Percentiles | |||
---|---|---|---|---|---|---|---|---|
25% | 50% | 75% | ||||||
5 | 7.99 | 4.42 | 5.52 | 0.48 | 5.27 | 5.47 | 5.67 | |
10 | 11.80 | 8.40 | 10.33 | 0.52 | 10.09 | 10.36 | 10.59 | |
15 | 18.01 | 14.11 | 15.34 | 0.58 | 15.05 | 15.22 | 15.56 |
The slope setting of this study was set according to actual forest fires. Considering that the study area has mostly low hills and gently sloping terrain, the slope generally does not exceed 35°; thus, the slopes were set to 0°, 10°, 20°, 30°, and 35° for a total of five levels.
Before the start of the combustion experiment, the treated P. koraiensis fuel was uniformly sprinkled on the burning bed in a manner that simulated falling under pine needles. The fuel bed dimensions were 4 m × 1 m. The fuel depth is a crucial input parameter for the Rothermel model, and there is an inevitable error in the fuel depth for each layer due to factors such as the fuel’s gravity and moisture content. The fuel depth was measured four times after each lay-up was finished, and the average value was calculated (Table 3). A portable weather station (Kestrel 4500) was used to record the air temperature and relative humidity before the start of each combustion experiment, and the burning bed was then adjusted to the required slope. A volume of 15 mL of anhydrous ethanol was sprayed into the ignition tank (1 m × 1 cm) to ignite the fuel before the experiment started. In this study, 60 (four fuel loads × three fuel moisture contents × five slopes) combinations of various fuel loads, fuel moisture contents, and slopes were used. For the 0°, 10°, 20°, and 30° slope combinations, five replicate were used. For the 35° slope combinations, three replications were used. This totals 276 combustion experiments (Table 4). An infra-red thermometer was used to confirm that the burning bed had cooled to room temperature before the commencement of each experiment to prevent the impact of the excess heat from affecting the next experiment.
Fuel load (kg m−2) | Maximum value | Minimum value | Mean value | s.d. | Percentiles | |||
---|---|---|---|---|---|---|---|---|
25% | 50% | 75% | ||||||
0.4 | 3.00 | 2.03 | 2.51 | 0.29 | 2.25 | 2.50 | 2.73 | |
0.8 | 5.53 | 3.57 | 4.50 | 0.41 | 4.19 | 4.42 | 4.87 | |
1.2 | 7.20 | 5.30 | 6.14 | 0.43 | 5.80 | 6.15 | 6.47 | |
1.6 | 9.17 | 6.97 | 7.86 | 0.51 | 7.46 | 7.80 | 8.33 |
Symbol | Parameter | Pinus koraiensis fall of leaf | |
---|---|---|---|
h | Low heat content (kJ kg−1) | 17,854A | |
M x | The moisture content of extinction (fraction) | 0.3B | |
σ | Surface-area-to-volume ratio (m−2 m−3) | 6864.3A | |
ρ p | Oven-dry particle density (kg m−3) | 396.4A | |
S T | Total mineral content (fraction) | 0.0311A | |
S e | Effective mineral content (fraction) | 0.01B |
The Rothermel model is a model of a firefront in a ‘seemingly steady state’ situation. In the process of upslope fire spread, the rate of fire spread first increases and then stabilises. After the pre-experiment, a 0.5-m long precombustion area was determined. When the fire heads passed through the precombustion area, the fire spread rate reached the ‘seemingly steady state,’ and at this time, the measurement of the fire spread rate was started (Li et al. 2021). To measure the rate of fire spread, 20 thermocouples are placed along the centreline of the combustion bed at intervals of 0.1, 0.5 m from the igniting end; each thermocouple position was 0.5, 0.6, 0.7, 1.2, 1.3, 1.4, 1.5, 1.6, 1.7, 1.9, 2.0, 2.1, 2.2, 2.3, 2.9, 3.0, 3.1, 3.2, 3.3, 3.4 m. The time for each thermocouple’s temperature to reach 254°C was extracted, and this was called the igniting point for P. koraiensis. The thermocouple temperature–time image is fitted to the position time image at this point, and the slope of this fit indicates the rate of fire spread under the conditions of this experimental setup (Liu et al. 2014) (Fig. 2).
Rothermel model parameter modification
The Rothermel model is used in this section to predict the ROS under different fuel loads, fuel moisture contents, and slope conditions. Rothermel (1972) derived the prediction equations for the spreading rate under no-wind and no-slope conditions based on fuel characteristics and different fuel moisture contents. Dimensionless wind and slope equations were included to add the effects of wind and slope into the model in order to create the primary form of the Rothermel model, which Albini (1976b) subsequently modified. In this study, because the effect of wind on the rate of fire spread was not considered, the Rothermel model for the no-wind condition is as:
where R0 is the no-wind and no-slope rate of spread, IR is the reaction intensity, ξ is the propagating flux ratio, ρb is the bulk density, ε is the effective heating number, and Qig is the heat of preignition.
where R is the rate of spread, and ϕS is the slope factor.
where Γ′ is the optimum reaction velocity, wn is the net fuel load, h is the low heat content, ηM is the moisture damping coefficient, and ηS is the mineral damping coefficient.
where is the maximum reaction velocity, β is the packing ratio, and βop is the optimum packing ratio.
where σ is the surface-area-to-volume ratio.
where ρp is the over-dry particle density.
where w0 is the over-dry fuel load, and δ is the fuel bed depth.
where ST is the total mineral content.
where rM is Mf/Mx (max = 1.0).
where Mf is the fuel moisture content, and Mx is the dead fuel moisture content during extinction.
where Se is the effective mineral content.
The values of the input parameters of the Rothermel model are in Table 4.
The Rothermel model predicts a significant discrepancy between the ROS and observed ROS and has different applicability for various fuel types. The input parameters in the Rothermel model include three types: (1) fuel particle (h, ST, SE, and ρp); (2) fuel array (σ, w0, δ, and Mx); and (3) environmental (Mf, ϕS, and U). The leading cause of the prediction inaccuracy is the variation in fuel array settings. The fuel array influences the fuel heat transfer process, where the primary fuel array parameter is. Since the environment has a different impact on ROS for various fuels, the fuel array and environment-related parameters are chosen for modification in this paper.
The process of Rothermel model formulation has five main stages: (1) proposing a conceptual physical model framework; (2) proposing homogeneous fuel ROS prediction equations under windless conditions on flat land; (3) introducing dimensionless wind and slope parameters; (4) extending to nonhomogeneous fuel ROS prediction; and (5) practical application in the field. Therefore, the parameters of the fuel array and environment under no-wind and no-slope conditions are initially modified, the parameters with the best modification effect are compared and selected and then the optimal parameters are incorporated into the model for slope parameter modification.
The parameters related to σ in the fuel array of the Rothermel model under no-wind and no-slope conditions include ε, A, βop, , ξ and IR, and the Mf parameter is selected for correction in the environment. To improve the running efficiency of the MATLAB program, the parameters unrelated to the modification of the parameters are integrated and only the parameters to be modified are included.
As an example of correcting the IR parameter, Eqns 4–17 are first substituted into Eqn 1 to produce:
Converting the coefficients in Eqn 18 into that parameters to be modified yields Eqn 19:
Eqn 19 is the formula for modifying the IR parameter. Here, Y1 is the observed ROS under no-wind and no-slope conditions, X1 is the product of all Rothermel model parameters except IR, Z is σ, C is β, M1 is rM, M2 is (rM)2, M3 is (rM)3, K is Se, and a1, b1, c1, d1, e1, f1, g1, h1, i1, j1, k1, and l1 are the coefficients to be modified.
Similarly, the modification equations for each of the other parameters are:
Modification ε:
where X2 is the product of all the Rothermel model parameters except ε and a2 is the coefficient to be modified.
Modification A:
where X3 is the product of all the Rothermel model parameters except A and B are the β/βop, and a3 and b3 are the coefficients to be modified.
Modification βop:
where X4 is the product of all the Rothermel model parameters except βop and a4 and b4 are the coefficients to be modified.
Modification :
where X5 is the product of all the Rothermel model parameters except , and a5, b5, and c5 the coefficients to be modified.
Modification ξ
where X6 is the product of all the Rothermel model parameters except ξ and a6, b6, c6, e6, and f6 are the coefficients to be modified.
Modification ηM
where X7 is the product of all the Rothermel model parameters except ηM and a7, b7, and c7 are the coefficients to be modified.
The mean relative error (MRE), mean absolute error (MAE), root mean square error (RMSE), coefficient of determination (R square, R2), and the R2 value of the predicted ROS and observed ROS of the Rothermel model were calculated after the parameter modification under no-wind and no-slope conditions was finished. The parameter with the best modification effect was chosen and introduced into the Rothermel model for slope coefficient modification. The correction formula for the slope coefficient ϕs is:
where X8 is the product of all Rothermel model parameters except ϕS; a8, b8, and c8 are the coefficients to be modified; and T is the tanϕ.
Data processing and analysis
A multifactor ANOVA was used to analyse the effects of fuel load, fuel moisture content, and slope, and their interactions on the ROS. The parameters in the Rothermel model are modified using the least squares method written in MATLAB R2018b (Eqns 19–26). Least squares are a mathematical modification technique for finding the best functional match of data by minimising the sum of squares of errors; this method can easily find the unknown data and reduce the sum of squares of errors between these data and the actual data. In the adjustment calculation, the number of equations listed is always less than the number of unknowns contained in the equation, which can be solved under the least squares criterion to obtain a set of unique solutions. Therefore, the least squares method is widely used in error estimation, uncertainty analysis, and model parameter solutions. The model-modification effect was evaluated using the MAE, MRE, RMSE, and R2.
Results and discussions
Observed ROS
The rate of spread is one of the crucial indicators of fire behaviour and affected by a combination of factors. In this study, the ROS are influenced by the slope, fuel load, and fuel moisture content involved, which is similar to the basic principles of surface fire ROS. The three control variables of fuel load, fuel moisture content, and slope and their interactions all significantly affected the ROS (P < 0.001) (Table 5). In this study, the maximum average ROS was 2.96 m min−1 at a fuel load of 1.6 kg m−2 and the minimum average ROS was 0.10 m min−1 at a fuel load of 0.4 kg m−2 (Table 6, Fig. 3). Overall, the ROS increased with increasing fuel load, which was similar to results of most studies. This occurred because an increase in fuel load directly improved the effective fuel load involved in combustion, added the heat released from the fuel during combustion, increased the flame length (Cruz et al. 2018b), and increased the heat release rate from the flame (Tihay et al. 2012), thereby enhancing the radiative preheating of the flame to the unburned fuel ahead (Tihay et al. 2014). However, in this study, the ROS decreased with increasing fuel load under certain fuel moisture content and slope conditions, such as fuel load = 1.2 kg m−2, FMC = 5%, and slope = 30°. This can be explained by the following: under certain combinations of fuel moisture content and slope conditions, a threshold value existed between the fuel load and ROS. Below this value, the ROS increased with increasing fuel load; above this value, with increasing fuel load, the combustible bed became thicker, more heat was needed to ignite the lower flammable fuel, and the proportion of heat needed to ignite the forward fuel described, as shown by a decreasing ROS. Some scholars have also decreased the above phenomenon. McCaw et al. (2012) found that although the ROS increased as the fuel load increased, the ROS was more significantly linked with the different parameters. Lozano et al. (2008) investigated the relationship between fuel load and ROS using particle image velocimetry (PIV). They found that as the fuel load increased, the fuel affected the oxygen supplied to the combustion zone, leading to a decrease in ROS. By conducting laboratory studies, Rothermel (1972) reported that the relationship between ROS and fuel loading depended on the fuel bed’s bulk density and the fuel particle surface-area-to-volume ratio. For fine surface fuels, if the bulk density was lower than the optimum packing ratio, the ROS increased with increasing fuel loading; however, if the bulk density was higher than the optimum packing ratio, the ROS decreased with increasing fuel loading. Based on the experimental results of this study, the optimum packing ratio could vary depending on the slope and fuel moisture content. Consequently, the ROS decreased with increasing fuel load.
Effect factors | df | Mean square | F-value | P-value | |
---|---|---|---|---|---|
Fuel load | 3 | 2.40 | 148.85 | <0.001 | |
FMC | 2 | 4.54 | 281.31 | <0.001 | |
Slope | 4 | 10.47 | 649.46 | <0.001 | |
Fuel load × FMC | 6 | 0.20 | 12.61 | <0.001 | |
Fuel load × slope | 12 | 0.36 | 22.18 | <0.001 | |
FMC × slope | 8 | 0.67 | 41.29 | <0.001 |
Fuel load (kg m−2) | Minimum | Maximum | Mean | s.d. | Percentiles | |||
---|---|---|---|---|---|---|---|---|
25% | 50% | 75% | ||||||
0.4 | 0.10 | 1.09 | 0.36 | 0.24 | 0.18 | 0.26 | 0.46 | |
0.8 | 0.14 | 1.99 | 0.51 | 0.41 | 0.23 | 0.33 | 0.67 | |
1.2 | 0.16 | 2.51 | 0.68 | 0.56 | 0.27 | 0.39 | 1.01 | |
1.6 | 0.19 | 2.96 | 0.74 | 0.63 | 0.29 | 0.48 | 1.07 |
Rate of spread variation under different conditions. (a–c) Observed ROS. (d–f) Rothermel model predicted ROS. (g–i) Predicted ROS after modifying the Rothermel model parameters.
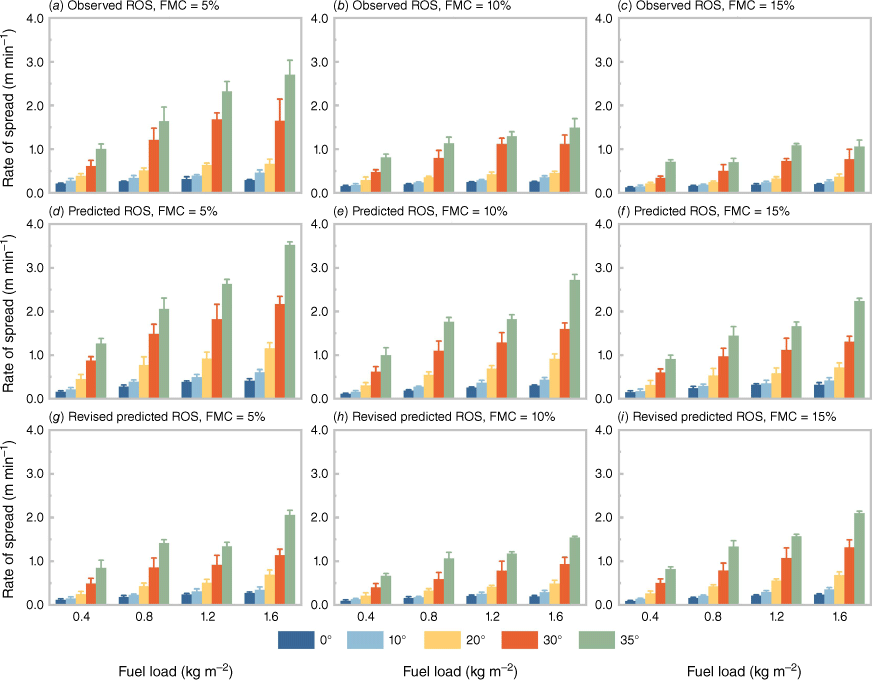
During upslope fires, the ROS increases with slope. In this study, as the slope increased from 10° to 20°, and from 20° to 30°, the ROS increased by 0.51 and 1.18 times, respectively. The heat transmission between the fuel bed varied as the slope increased, and the mode shift occurred at a slope of approximately 20°. Dupuy and Maréchal (2011) found that radiative heat transfer between fuel beds dominated the mechanism of fire spread heat transfer influence for slope conditions between 0° and 20°. However, at 20° or higher slopes, convective heating significantly increased, but radiative heat transfer ceased to grow or even slightly decreased. Thus, convective heating was the primary component affecting the increase in ROS under high slope conditions. To precisely estimate the ratio of radiation and convection at various slopes and determine the critical slope of the heat transfer change, smaller slope gradients should be used in subsequent investigations of the influence of the slope on the ROS.
In this study, the ROS decreased with increasing fuel moisture content. Studies related to this topic have demonstrated that when the fuel moisture content increases, the ignition energy needed to ignite the fuel also increases. This occurs because when the fuel is ignited, the water is heated to the boiling point and fully vaporised before it reaches the ignition temperature. Once the fuel is ignited, as the fire spreads, the water in the adjacent fuel needs to be evaporated, and the latent heat of vaporisation of the water absorbs the heat released as the fuel burns, resulting in a lower ROS (Simard 1968). Moreover, a reduced ROS results from the water in the fuel being released into the air as water vapour; this reduces the oxygen concentration and the heat generated by the flame (Rothermel and Anderson 1966; Catchpole et al. 1998; Mendes-Lopes et al. 1998). In future experiments, we plan to study the effects of various variables on ROS at greater depths by using smaller slopes, fuels with higher moisture content, and fuel load gradients.
Modification results for the Rothermel model parameters
Under no-wind and no-slope conditions, the fuel array parameters of the Rothermel model were modified. The improvement in model prediction accuracy varied depending on the variations in parameter modification. The results show that the MAE, MRE, and RMSE for the modified IR parameters are the lowest compared to those for the modified other parameters, 0.123 m min−1, 17.39%, and 0.19 m min−1, respectively, with R2 = 0.67 (Fig. 4). Compared with those of the original Rothermel model, the MAE, MRE, and RMSE for the predicted ROS under no-wind and no-slope conditions were reduced by 0.04 m min−1, 3.85% and 0.04 m min−1, respectively. Compared with the original Rothermel model, the equation for the modified IR parameters is (shown in Eqn 27):
The most significant improvement in forecast accuracy is associated with the adjusted IR parameters, indicating that the IR is the critical factor influencing the Rothermel model’s prediction. Modifying the ηM parameter alone is only second to correct the IR, which suggests that ηM has the most excellent effect on the IR and an essential effect on the Rothermel model. Rothermel developed the empirical formula IR from laboratory measurements of various fuel types and environmental variables. The IR includes five parameters: (1) modification reaction density; (2) net fuel load; (3) low heat content; (4) moisture damping coefficient; and (5) mineral damping coefficient. As a result, when modifying the Rothermel model, more than just one model parameter need to be considered. Additionally, the combined influence of several factors on the model’s prediction results should be considered, and the IR should be re-evaluated for various fuels.
Parameter correction results under no slope and no wind conditions. (a) Uncorrected, (b) modified the parameter ε, (c) modified the parameter ηM, (d) modified the parameter , (e) modified the parameter A, (f) modified the parameter βop, (g) modified the parameter ξ, and (h) modified the parameter IR.
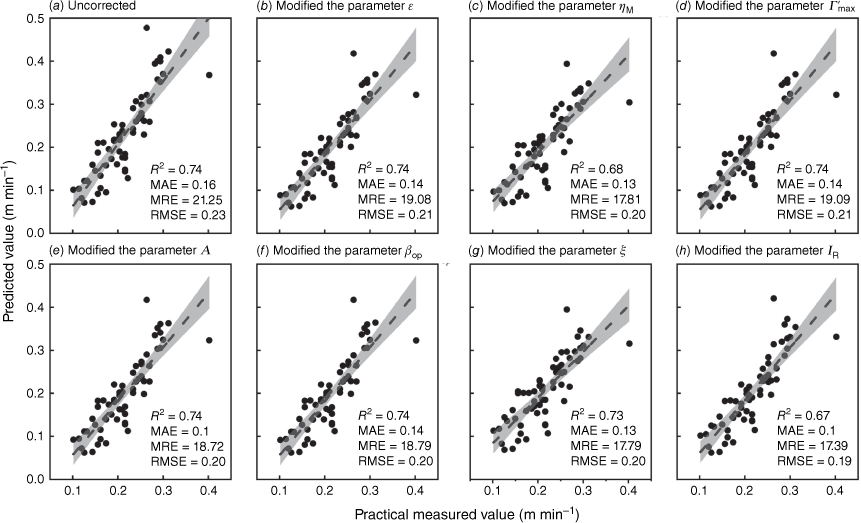
In this section, the modified IR parameter is brought into the Rothermel model to modify the slope parameter ϕs, and the modification of Eqn 25 yields:
The modification results are incorporated into the Rothermel model, and Fig. 5 depicts the model’s predicted ROS and errors. The model predicts ROS with an MAE = 0.46 m min−1, an MRE = 25.09%, an RMSE = 0.77 m min−1, and an R2 = 0.80 after improving the slope parameter.
Rothermel model predicted ROS fit to the observed ROS. (a) Uncorrected and (b) corrected IR and ϕs.
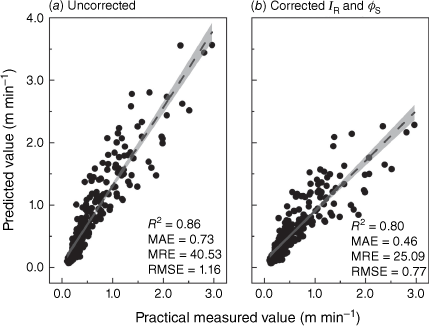
The accuracy of the Rothermel model predicted ROS on the surface of the P. koraiensis plantation forest significantly increased after modification of the slope parameters; the MRE of the model predicted ROS decreased by 18.64%, and the MAE decreased by 0.33 m min−1. This occurred because actual ROS data was used for modification, and related studies have shown that it can improve the model’s accuracy for ROS prediction by using real ROS data for model parameter modification. From the errors that the original Rothermel model projected after slope and modified parameters, the general trend is consistent with original model. But the most significant error value is shown at the 30° condition (MRE = 31.82%, MAE = 0.42 m min−1), which might be because the calculation for the slope parameter only takes into account two variables: (1) the fuel compression ratio; and (2) the tangent function of the slope. The change trend in physical mechanisms cannot be sufficiently described as the increase in the fire spread rate with slope. Instead, a thorough understanding of the fuel preheating mechanism is needed to develop accurate model predictions (Yuan et al. 2020). Therefore, physical equations need to be added to the slope parameter to enhance model prediction in further investigations.
Rothermel model prediction accuracy evaluation
The MAE and MRE of the predicted surface fuel ROS vs the observed ROS for P. koraiensis plantation forests with various combinations of fuel load, fuel moisture content, and slope in Fig. 6, with each bar representing the mean value of the prediction error at various levels of influencing factors. The data were obtained using the Rothermel model directly and after modification of the model’s parameters. The MAE ranged from 0.03 to 4.04 m min−1, and the MRE ranged from 4.28 to 105.59% with direct use of the Rothermel model. The Rothermel model predicted an MRE in the 4.40–53.99% range and an MAE in the 0.03–2.16 m min−1 range after modification of the IR and ϕs parameters. After the parameters were modified, the prediction accuracy of the Rothermel model significantly increased compared to that of the original Rothermel model.
Rothermel model predicted the MRE and MAE of the ROS under different conditions. (a–c) MRE for predicting the ROS concentration using the original Rothermel model parameters under different conditions. (d–f) MRE for predicting ROS levels using the modified Rothermel model parameters under different conditions. (g–i) MAE for predicting the ROS concentration using the original Rothermel model parameters under different conditions. (j–l) MAE for predicting the ROS concentration using the modified Rothermel model parameters under different conditions.
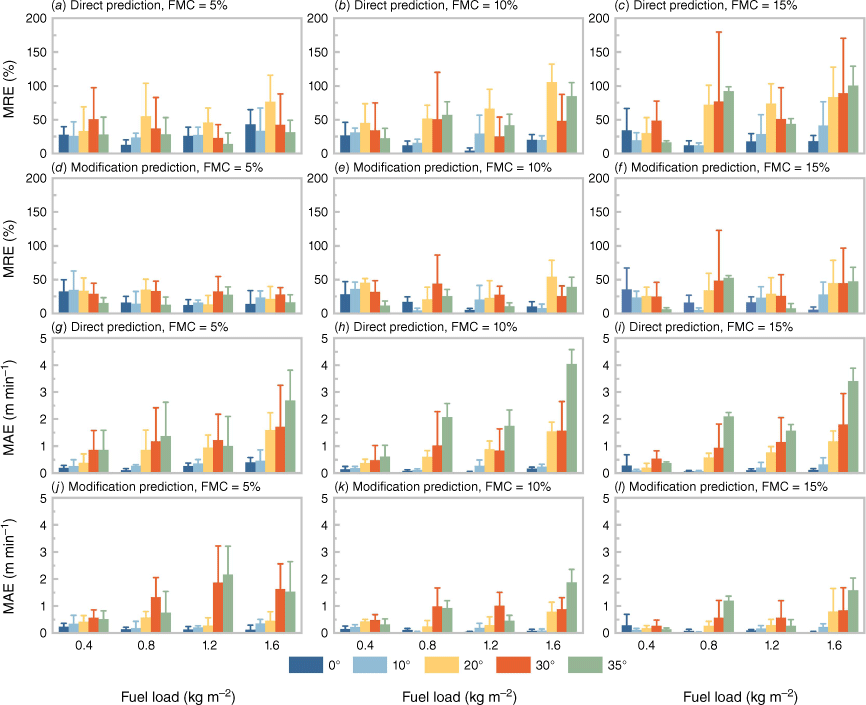
When using the Rothermel model directly to predict the ROS, the relationship between fuel loads and prediction error increased, then decreased, and then continued to increase, with the lowest MRE of 31.60% occurring at 0.4 kg m−2 and the highest MRE of 55.87% occurring at 1.6 kg m−2. After modifying the model parameters, the MRE showed an initial decreasing trend and then an increasing trend, with the lowest error of 19.32% occurring at 1.2 kg m−2. With fuel loads of 0.4, 0.8, 1.2, and 1.6 kg m−2, the MAE decreased by 0.08, 0.27, 0.22, and 0.66 m min−1, respectively, while the MRE decreased by 4.08, 15.32, 15.19, and 28.54%, respectively. Because the internal structure of the fuel bed changed due to a change in fuel loads, the heat transfer mode of the fuel during the combustion process also changed, resulting in poor prediction accuracy for the Rothermel model under different fuel load conditions. The Rothermel model forecasts the same fuel type with varying degrees of accuracy for various fuel load scenarios. Benali et al. (2016) found that the uncertainties in fuel model assignments and parameters significantly impacted the prediction of the ROS and that small changes in fuel structure could result in large changes in the predicted or observed ROS. Caution is advised when using the Rothermel model to predict ROS for various fuel loads. The prediction error caused by the change in loading is somewhat altered by the Rothermel model based on combustion experiments with modifications IR and ϕs. The prediction accuracy greatly improved (Fig. 5).
As shown by the fuel moisture content and model prediction errors (Fig. 6), the Rothermel model directly predicts higher ROS levels than the observed ROS, and the MRE increases with increasing fuel moisture content, with the lowest MRE of 34.26% occurring at 5% fuel moisture content and the highest at 15% occurring at 48.08%. This occurs because: (1) the Rothermel model’s direct application predicts a more excellent ROS than the observed ROS, indicating that the model underestimates the impact of moisture content on ROS, and this, increases the predictive error of the model (Storey et al. 2021); and (2) the fuel-related component of the Rothermel model is called ηM, where ηM comprises the variables Mf and Mx. The range of Mx for various kinds of pine needles is between 28 and 40% (Rothermel 1972). The fixed value of 30% provided in the Rothermel model is the Mx employed in this study; this value should differ from the actual Mx of P. koraiensis surface fuel. The modified parameters of the Rothermel model predicted that the MRE of ROS would increase with increasing moisture content, decrease by 15.79%, and decrease by 0.30 m min−1 compared to those of the original model.
In terms of the slope and model prediction errors, the MRE of ROS prediction directly using the Rothermel model increased and then decreased with increasing slope, with a mean value of 40.64%, the smallest MRE of 21.24% at 0°, and the lowest MRE of 61.44% at 20°. There may be three reasons for this difference. First, in the Rothermel (1972), only combustion experiments were carried out at 25, 50, and 75%, with slope conditions of 14.32°, 28.64°, and 42.96°, and this large range of slope gradient settings were insufficient to represent the ROS variations at all slopes. Second, in addition, Andrews’ (2018) summary of the Rothermel model slope parameters revealed that different fuel types corresponded to different slope parameters; therefore, using a fixed slope parameter to predict different fuel types led to significant errors. Third, the Rothermel model incorporates the effect of slope on the ROS into the model by adding dimensionless slope coefficients without considering the shift in heat transfer during the increase in slope. The combined impact of these three factors increases the significance of the Rothermel model prediction inaccuracy under slope circumstances. After the parameters are modified, the Rothermel model predicts that the MRE of the ROS increases and then decreases with increasing slope, with the lowest value of 17.39% occurring at 0° and the highest value of 32.87% occurring at 30°, for a mean value of 24.85%. The slope parameter is a dimensionless factor added to the Rothermel model through the empirical formula, which has no practical physical meaning. The original Rothermel model overestimates the impacts of the compression ratio and slope factors on the ROS, leading to significant model projections when combined with the modified slope formula.
Overall, compared with the original model, the modified Rothermel model yielded a 15.22% decrease in the MRE, a 0.27 m min−1 decrease in the MAE, a 0.39 m min−1 decrease in the RMSE, and a R2 = 0.80. These findings demonstrated the significant improvement in the prediction accuracy of the Rothermel model after the parameters were modified.
The prediction accuracy of the Rothermel model increased with model prediction MRE = 25.09% after some model parameters were modified based on the real ROS acquired from inlab combustion tests. Cruz and Alexander (2013) used ROS data from 1278 fires to analyse the applicability of 49 fire spread models. They found that a model prediction error of 35% was considered a reasonable model performance criterion. As a result, the modified Rothermel model in this study could be used for ROS prediction of surface fires in P. koraiensis plantations.
To increase the precision of the model in forecasting the ROS of surface fires in P. koraiensis plantations, we modified several Rothermel model parameters using ROS data from laboratory combustion experiments. We performed a preliminary study on the connection between the influencing elements and the model’s forecast accuracy. Due to the experimental setup, this study only displayed indoor combustion tests for a single combustible material under varied fuel loads, fuel moisture content, and slope circumstances. This study can provide fundamental information for the ensuing fire database despite variations in actual field fires. In addition, predicting spatial and temporal changes in fire behaviour and protecting lives of firefighters are crucial. Moreover, understanding fire behaviour, predicting spatial and temporal changes in fire behaviour, providing guidelines for conducting local fire risk assessments and developing fire management systems are essential.
Conclusion
In this paper, the ability of the Rothermel model to predict surface fire ROS in P. koraiensis plantations under different fuel loads (0.4, 0.8, 1.2, and 1.6 kg m−2), fuel moisture contents (5, 10, and 15%), and slopes (0°, 10°, 20°, 30°, and 35°) was investigated through laboratory combustion experiments. The model’s accuracy was evaluated, and the model parameters were modified based on laboratory-acquired ROS data. We conclude that the effects of variations in fuel load, fuel moisture content, and slope on ROS from a single factor are consistent with our current knowledge of combustion. Despite this, numerous factors interact to cause the opposite trend in ROS. The prediction accuracy of predicting ROS directly using the Rothermel model is poor. The accuracy of the Rothermel model in predicting ROS can be significantly improved by correcting the Rothermel model parameters with the ROS data obtained in the laboratory. In subsequent work, the prediction accuracy of the Rothermel model will be improved by merging data from controlled fires and actual forest fires.
Nomenclature
Symbol | Parameters |
R0 | No-wind and no-slope rate of spread (m min−1) |
IR | Reaction intensity (kW m−2) |
ξ | Propagating flux ratio |
ρb | Bulk density |
ε | Effective heating number |
Qig | Heat of preignition (kJ kg−1) |
R | Rate of spread (m min−1) |
ϕS | Slope factor |
Γ′ | Optimum reaction velocity (min−1) |
wn | Net fuel load (kg m−2) |
h | Low heat content (kJ kg−1) |
ηM | Moisture damping coefficient |
ηS | Mineral damping coefficient |
Maximum reaction velocity (min−1) | |
β | Packing ratio |
βop | Optimum Packing ratio |
A | – |
σ | Surface-area-to-volume ratio (m−1) |
ρp | Over-dry particle density (kg m−3) |
w0 | Over-dry fuel load (kg m−2) |
δ | Fuel bed depth (m) |
ST | Total mineral content |
rM | Mf/Mx (max = 1.0) |
a1, a2, b2, a3, b3, a4, b4, c4, a5, b5, c5, d5, e5, f5, a6, b6, c6, d6, e6, f6, g6, h6, i6, j6, k6, l6, a7, b7, c7, a8, b8, c8 | The coefficients to be modified |
Mf | Fuel moisture content |
Mx | Dead fuel moisture content of extinction |
Se | Effective mineral content |
ϕ | Slope (°) |
ϕS | Slope factor |
Y1 | Observed ROS under no-wind and no-slope conditions (m min−1) |
X1 | Product of all Rothermel model parameters except IR |
X2 | Product of all Rothermel model parameters except ε |
X3 | Product of all Rothermel model parameters except A |
X4 | Product of all Rothermel model parameters except βop |
X5 | Product of all Rothermel model parameters except |
X6 | Product of all Rothermel model parameters except ξ |
M2 | (rM)2 |
M3 | (rM)3 |
X7 | Product of all Rothermel model parameters except ηM |
Y2 | Observed ROS under no-wind condition (m min−1) |
X8 | Product of all Rothermel model parameters except ϕs |
T | tan ϕ |
Declaration of funding
This work was funded by the National Key Research and Development Program of China (2022YFC3003104), National Natural Science Foundation of China (32371881), Fundamental Research Funds for the Central Universities (2572023CT01-01) and High-level/excellent doctoral talents introduction scientific research start-up project of Inner Mongolia Agricultural University under Grant (NDYB2021-7).
Author contributions
Daotong Geng: Writing – original draft, Methodology, Investigation, Data curation, Formal analysis, Conceptualisation. Guang Yang: Methodology, Writing – review & editing, Funding acquisition, Project administration, Conceptualisation. Jibin Ning: Methodology, Writing – review & editing. Ang Li: Writing – review & editing. Zhaoguo Li: Investigation. Shangjiong Ma: Investigation. Xinyu Wang: Investigation. Hongzhou Yu: Software, Formal analysis.
References
Abram NJ, Henley BJ, Sen Gupta A, Lippmann TJR, Clarke H, Dowdy AJ, Sharples JJ, Nolan RH, Zhang T, Wooster MJ, Wurtzel JB, Meissner KJ, Pitman AJ, Ukkola AM, Murphy BP, Tapper NJ, Boer MM (2021) Connections of climate change and variability to large and extreme forest fires in southeast Australia. Communications Earth & Environment 2, 1-17.
| Crossref | Google Scholar |
Alexander ME, Cruz MG (2013) Limitations on the accuracy of model predictions of wildland fire behaviour: a state-of-the-knowledge overview. Forestry Chronicle 89, 370-381.
| Crossref | Google Scholar |
Andrews PL (2007) BehavePlus fire modeling system: past, present, and future. In ‘Proceedings of 7th Symposium on Fire and Forest Meteorological Society’, 23–25. October 2007, Bar Harbor, ME. Paper J2.1. (American Meteorological Society: Boston, MA, USA) Available at https://ams.confex.com/ams/pdfpapers/126669.pdf
Andrews PL (2014) Current status and future needs of the BehavePlus Fire Modeling System. International Journal of Wildland Fire 23, 21-33.
| Crossref | Google Scholar |
Andrews PL, Cruz MG, Rothermel RC (2013) Examination of the wind speed limit function in the Rothermel surface fire spread model. International Journal of Wildland Fire 22, 959-969.
| Crossref | Google Scholar |
Bachmann A, Allgöwer B (2002) Uncertainty propagation in wildland fire behaviour modelling. International Journal of Geographical Information Science 16, 115-127.
| Crossref | Google Scholar |
Benali A, Ervilha AR, Sá AC, Fernandes PM, Pinto RM, Trigo RM, Pereira JMC (2016) Deciphering the impact of uncertainty on the accuracy of large wildfire spread simulations. Science of the Total Environment 569–570, 73-85.
| Crossref | Google Scholar | PubMed |
Bowman DMJS, Williamson GJ, Abatzoglou JT, Kolden CA, Cochrane MA, Smith AMS (2017) Human exposure and sensitivity to globally extreme wildfire events. Nature Ecology & Evolution 1, 0058.
| Crossref | Google Scholar | PubMed |
Byram GM (1966) Scaling laws for modeling mass fires. Pyrodynamics 4, 271-284.
| Google Scholar |
Canadian Interagency Forest Fire Center (2003) ‘Glossary of Forest Fire Management Terms.’ 61 p. (Winnipge, MB, Canada) Available at http://bcwildfire.ca/MediaRoom/Backgrounders/2003_Fire_Glossary.pdf2003
Catchpole EA, Catchpole WR, Rothermel RC (1993) Fire behavior experiments in mixed fuel complexes. International Journal of Wildland Fire 3, 45-57.
| Crossref | Google Scholar |
Catchpole WR, Catchpole EA, Butler BW, Rothermel RC, Latham DJ (1998) Rate of spread of free-burning fires in woody fuels in a wind tunnel. Combustion Science & Technology 131, 1-37.
| Crossref | Google Scholar |
Cruz MG, Alexander ME (2013) Uncertainty associated with model predictions of surface and crown fire rates of spread. Environmental Modelling & Software 47, 16-28.
| Crossref | Google Scholar |
Cruz MG, Alexander ME, Sullivan AL, Gould JS, Kilinc M (2018a) Assessing improvements in models used to operationally predict wildland fire rate of spread. Environmental Modelling & Software 105, 54-63.
| Crossref | Google Scholar |
Cruz MG, Sullivan AL, Gould JS, Hurley RJ, Plucinski MP (2018b) Got to burn to learn: the effect of fuel load on grassland fire behaviour and its management implications. International Journal of Wildland Fire 27, 727-741.
| Crossref | Google Scholar |
Dittrich R, McCallum S (2020) How to measure the economic health cost of wildfires – A systematic review of the literature for northern America. International Journal of Wildland Fire 29, 961-973.
| Crossref | Google Scholar |
Driscoll DA, Lindenmayer DB, Bennett AF, Bode M, Bradstock RA, Cary GJ, Clarke MF, Dexter N, Fensham R, Friend G, Gill M, James S, Kay G, Keith DA, MacGregor C, Russell-Smith J, Salt D, Watson JEM, Williams RJ, York A (2010) Fire management for biodiversity conservation: key research questions and our capacity to answer them. Biological Conservation 143, 1928-1939.
| Crossref | Google Scholar |
Dupuy JL, Maréchal J (2011) Slope effect on laboratory fire spread: contribution of radiation and convection to fuel bed preheating. International Journal of Wildland Fire 20, 289-307.
| Crossref | Google Scholar |
Dupuy JL, Maréchal J, Portier D, Valette JC (2011) The effects of slope and fuel bed width on laboratory fire behaviour. International Journal of Wildland Fire 20, 272-288.
| Crossref | Google Scholar |
Fernandes PM (2009) Combining forest structure data and fuel modelling to classify fire hazard in Portugal. Annals of Forest Science 66, 415.
| Crossref | Google Scholar |
Filkov AI, Ngo T, Matthews S, Telfer S, Penman TD (2020) Impact of Australia’s catastrophic 2019/20 bushfire season on communities and environment. Retrospective analysis and current trends. Journal of Safety Science and Resilience 1, 44-56.
| Crossref | Google Scholar |
Fons WL (1946) Analysis of fire spread in light forest fuels. Journal of Agricultural Research 72, 93-121.
| Google Scholar |
Frandsen WH (1971) Fire spread through porous fuels from the conservation of energy. Combustion & Flame 16, 9-16.
| Crossref | Google Scholar |
Gould JS, Sullivan AL (2020) Two methods for calculating wildland fire rate of forward spread. International Journal of Wildland Fire 29, 272-281.
| Crossref | Google Scholar |
Jimenez E, Hussaini MY, Goodrick S (2008) Quantifying parametric uncertainty in the Rothermel model. International Journal of Wildland Fire 17, 638-649.
| Crossref | Google Scholar |
Johnston FH, Henderson SB, Chen Y, Randerson JT, Marlier M, Defries RS, Kinney P, Bowman DM, Brauer M (2012) Estimated global mortality attributable to smoke from landscape fires. Environment Health Perspectives 120, 695-701.
| Crossref | Google Scholar | PubMed |
Keane RE, Reeves M (2011) Use of expert knowledge to develop fuel maps for wildland fire management. In ‘Expert Knowledge and Its Application in Landscape Ecology’. (Eds AH Perera, C Drew, C Johnson, et al.) pp. 211–228. (Springer) 10.1007/978-1-s4614-1034-8_11
Koopmans E, Fyfe T, Eadie M, Pelletier CA (2020) Exploring prevention and mitigation strategies to reduce the health impacts of occupational exposure to wildfires for wildland firefighters and related personnel: protocol of a scoping study. Systematic Reviews 9, 119.
| Crossref | Google Scholar | PubMed |
Li H, Liu N, Xie X, Zhang L, Yuan X, He Q, Viegas DX (2021) Effect of fuel bed width on upslope fire spread: an experimental study. Fire Technology 57, 1063-1076.
| Crossref | Google Scholar |
Liu N, Wu J, Chen H, Xie X, Zhang L, Yao B, Zhu J, Shan Y (2014) Effect of slope on spread of a linear flame front over a pine needle fuel bed: experiments and modelling. International Journal of Wildland Fire 23, 1087-1096.
| Crossref | Google Scholar |
Lozano J, Tachajapong W, Pan H, Swanson A, Kelley C, Princevac M, Mahalingam S (2008) Experimental investigation of the velocity field in a controlled wind-aided propagating fire using particle image velocimetry. Fire Safety Science 9, 255-266.
| Crossref | Google Scholar |
McCaw WL, Gould JS, Cheney NP, Ellis P, Anderson WR (2012) Changes in behaviour of fire in dry eucalypt forest as fuel increases with age. Forest Ecology and Management 271, 170-181.
| Crossref | Google Scholar |
Mendes-Lopes J, Ventura J, Amaral J (1998) Rate of spread and flame characteristics in a bed of pine needles. In ‘Proceedings of the III International Conference on Forest Fire Research – 14th Conference on Fire and Forest Meteorology’. Vol. 1, 16–20 November 1998, Luso, Portugal. (Ed. DX Viegas) pp. 497–511.
Ning J, Yang G, Liu X, Geng D, Wang L, Li Z, Zhang Y, Di X, Sun L, Yu H (2022) Effect of fire spread, flame characteristic, fire intensity on particulate matter 2.5 released from surface fuel combustion of Pinus koraiensis plantation – A laboratory simulation study. Environment International 166, 107352.
| Crossref | Google Scholar | PubMed |
Ning J, Yang G, Zhang Y, Geng D, Wang L, Liu X, Li Z, Yu H, Zhang J, Di X (2023) Smoke exposure levels prediction following laboratory combustion of Pinus koraiensis plantation surface fuel. Science of the Total Environment 881, 163402.
| Crossref | Google Scholar | PubMed |
Salvador R, Piñol J, Tarantola S, Pla E (2001) Global sensitivity analysis and scale effects of a fire propagation model used over Mediterranean shrublands. Ecological Modelling 136, 175-189.
| Crossref | Google Scholar |
Storey MA, Bedward M, Price OF, Bradstock RA, Sharples JJ (2021) Derivation of a Bayesian fire spread model using large-scale wildfire observations. Environmental Modelling & Software 144, 105127.
| Crossref | Google Scholar |
Sullivan AL (2009a) Wildland surface fire spread modelling, 1990–2007. 1: Physical and quasi-physical models. International Journal of Wildland Fire 18, 349-368.
| Crossref | Google Scholar |
Sullivan AL (2009b) Wildland surface fire spread modelling, 1990–2007. 2: Empirical and quasi-empirical models. International Journal of Wildland Fire 18, 369-386.
| Crossref | Google Scholar |
Thompson MP, Calkin DE (2011) Uncertainty and risk in wildland fire management: a review. Journal of Environmental Management 92, 1895-1909.
| Crossref | Google Scholar | PubMed |
Tihay V, Morandini F, Santoni PA, Perez-Ramirez Y, Barboni T (2012) Study of the influence of fuel load and slope on a fire spreading across a bed of pine needles by using oxygen consumption calorimetry. Journal of Physics. Conference Series 395, 012075.
| Crossref | Google Scholar |
Tihay V, Morandini F, Santoni PA, Perez-Ramirez Y, Barboni T (2014) Combustion of forest litters under slope conditions: burning rate, heat release rate, convective and radiant fractions for different loads. Combustion and Flame 161, 3237-3248.
| Crossref | Google Scholar |
Vaiciulyte S, Hulse LM, Veeraswamy A, Galea ER (2021) Cross-cultural comparison of behavioural itinerary actions and times in wildfire evacuations. Safety Science 135, 105122.
| Crossref | Google Scholar |
Weber RO (1991a) Modelling fire spread through fuel beds. Progress in Energy and Combustion Science 17, 67-82.
| Crossref | Google Scholar |
Weber RO (1991b) Toward a comprehensive wildfire spread model. International Journal of Wildland Fire 1, 245-248.
| Crossref | Google Scholar |
Xu X, Jia G, Zhang X, Riley WJ, Xue Y (2020) Climate regime shift and forest loss amplify fire in Amazonian forests. Global Change Biology 26, 5874-5885.
| Crossref | Google Scholar | PubMed |
Xue Q, Lin H, Wang F (2022) FCDM: an improved forest fire classification and detection model based on YOLOv5. Forests 13, 2129.
| Crossref | Google Scholar |
Yu P, Xu R, Abramson MJ, Li S, Guo Y (2020) Bushfires in Australia: a serious health emergency under climate change. The Lancet Planetary Health 4, e7-e8.
| Crossref | Google Scholar | PubMed |
Yuan X, Liu N, Xie X, Viegas DX (2020) Physical model of wildland fire spread: parametric uncertainty analysis. Combustion and Flame 217, 285-293.
| Crossref | Google Scholar |
Zhang Y, Sun P (2020) Study on the diurnal dynamic changes and prediction models of the moisture contents of two litters. Forests 11, 95.
| Crossref | Google Scholar |