Using stable-isotope analysis to assess recent diet and habitat use of stranded green turtles (Chelonia mydas)
Joshua J. Glen
A
B
C
Abstract
Gaining a better understanding of the foraging ecology of green turtles (Chelonia mydas) in different regions can improve the effectiveness of conservation and management strategies for this species. New South Wales (NSW), in eastern Australia, has been identified as a region requiring improved information on foraging ecology and habitat use by green turtles.
The aim of this study was to use stable-isotope analyses to investigate diet composition and foraging ecology of green turtles found stranded along the NSW coastline from 2018 to 2021.
This study measured δ13C, δ15N, and δ34S in macrophyte (mangrove, seagrass, and algae) samples collected along the NSW coastline, between March and June 2021, and epidermis tissue from green turtles that stranded in the same region between 2018 and 2021. In addition, stable-isotope values for animals known, or expected, to be consumed by green turtles were sourced from the literature. Macrophyte stable-isotope values were mapped to display spatial variations, then combined with literature-sourced animal matter and measured epidermis isotope values into stable-isotope mixing models. Mixing models were used to determine diet structure for each stranded green turtle, and their similarity to regional macrophyte isotope values to infer potential foraging locations.
High variation was present in δ13C, δ15N, and δ34S values of macrophyte sources along the NSW coastline, limiting the potential to infer the recent foraging locations of stranded green turtles. High δ15N values in green turtle epidermis samples indicated that most sampled turtles were feeding two to three trophic levels above primary producers within algal-based food webs. Mixing models suggested that these food webs were predominantly supported by algae (mean ± s. d. = 51.2 ± 6.1%) and animal material (31.4 ± 4.7%), with mangrove (13.4 ± 2.0%) and seagrass (4.0 ± 2.5%) representing much smaller proportions of overall diet composition.
The results showed that green turtles stranded along the NSW coastline feed primarily in algae-dominated food webs, with evidence of omnivory. These findings suggest that sampled individuals display diet compositions alternative to those of their Queensland counterparts, who feed primarily in seagrass-dominated habitats. Management and conservation programs in NSW should focus on limiting anthropogenic disturbances in algae-dominated habitats.
This novel finding demonstrated the importance of algae-dominated habitats for green turtle conservation and management in south-eastern Australia. The generated isoscapes are useful in examining isotopic variations in macrophytes along the NSW coastline.
Keywords: δ13C/δ15N/δ34S, green turtle, marine turtle, New South Wales, SIMMR.
Introduction
Insights into the foraging ecology and habitat use of threatened species can provide valuable knowledge for management and conservation programs for the species and their habitats. Green turtle, Chelonia mydas, is listed by the International Union for the Conservation of Nature (IUCN) as endangered globally (Seminoff 2004), with subpopulation assessments ranging from least concern to vulnerable (IUCN 2021). Global trends in green turtle populations have been monitored since the late 19th century (Jeremy et al. 2001), and regional population declines have been attributed to increased anthropogenic activities (Hays et al. 2024), including injuries and death caused by fishing and boating activities (Wallace et al. 2010; Flint et al. 2015; Monteiro et al. 2016; Cheng et al. 2019), coastal developments affecting nesting and foraging habitats (Verutes et al. 2014; Fuentes et al. 2016; Fuentes et al. 2020), and climate change affecting sex ratios and habitat quality (Wolff et al. 2018). Green turtles show strong fidelity to their home foraging grounds (Seminoff et al. 2002; Lamont et al. 2015), and can travel many kilometres to breeding grounds (Shimada et al. 2020). It is therefore important to investigate sea turtle ecology regionally, particularly in less-well studied regions, and those at distributional limits, which may display unique elements of ecology, such as habitat use and foraging.
Marine turtle strandings are common throughout their ranges, and include dead turtles found washed up on beaches or floating in oceans and seas, as well as turtles that are still alive, but in poor health. Strandings can be caused by multiple factors, including ingestion of plastics and other synthetic materials (Witzell and Teas 1994), entanglements in fisheries and shark-control nets (Flint et al. 2015), boat-strikes, coastal development (Türkozan et al. 2013; Cheng et al. 2019) and natural causes (e.g. poor health, nutrition, disease, cold stunning) (Servis et al. 2015; Flint et al. 2017). Sometimes the cause of stranding is obvious (e.g. boat strike or net entanglement wounds), whereas disease, ingestion of foreign material and poor nutrition can often be more cryptic. Marine turtles can strand in locations far removed from where the impact has occurred (Koch et al. 2013), making it difficult to ascertain the cause of stranding, or to identify the habitats that were prominently used by marine turtles prior to stranding. Understanding the habitat use and foraging ecology of marine turtles prior to stranding can therefore provide important insights into the types and locations of threats affecting particular marine turtle populations, and what regions and habitats require conservation and management focus.
Stable-isotope analysis (SIA) can be a useful tool for retrospectively investigating the relationship between stranded green turtles, their diet composition, and location prior to stranding (Pearson et al. 2017; Haywood et al. 2019). In ecological applications, SIA uses a suite of elemental isotopes measured within tissue samples, adjusting for metabolic processes through trophic discrimination (Peterson and Fry 1987; Fry 2006). The principle of SIA is that the tissue of consumers reflects the isotope values of diet sources, which can be traced down to the baseline isotope value of primary producers within the relevant food web (Fry 2006). Ratios of carbon isotopes (δ13C) undergo minimal trophic enrichment and, so, are used to trace the source of carbon production via photosynthesis (Peterson and Fry 1987; Fry 2006). Similarly, δ34S undergoes negligible fractionation from source to consumer, and is particularly useful in marine turtle studies by distinguishing sources of marine sulfate (algae) from terrestrial sulfate (seagrass, mangroves) (Cardona et al. 2009; Bradshaw et al. 2017). Ratios of nitrogen isotopes (δ15N values) are enriched through metabolic processes, resulting in predictable increases with each trophic level, allowing for the estimation of consumer trophic position (Deniro and Epstein 1981; Hobson, 1999; Fry 2006). SIA data can provide insight into diet changes through space and time, because of different rates of assimilation in different tissue types.
Isotopic values often differ with latitude, alongside changes in temperature, salinity and photosynthetic potential, which are known drivers of isotopic variation (Rubenstein and Hobson 2004; Fry 2006). The capacity of SIA to accurately estimate the location of stranded green turtle foraging activity relies on there being low variation in baseline isotope values within foraging locations, and high variation among locations. Where true, the isotope values of a consumer can be used to place an animal in time and space, when tissue values correlate to the stable-isotope values of diet sources within a certain location (Fry 2006). Maps showing the geographic variation in isotope values (isoscapes) allow for visual comparison between observed and expected isotope values to place animals in space and time (Torniainen et al. 2017). The use of isoscapes in marine turtle ecology was successfully implemented in the northern hemisphere by Ceriani et al. (2014), who found latitudinal variations in loggerhead turtle (Caretta caretta) δ13C and δ15N values derived from epidermis samples. However, in some cases, factors such as oceanic currents, temperature regimes and river discharges can prevent regionally unique isoscapes from being established, limiting the ability to assign foraging locations of sea turtles on the basis of their isotope values (Jones and Seminoff 2013; Coffee et al. 2020).
SIA of stranded marine turtles has also enabled researchers to infer diet prior to stranding in more detail (Cardona et al. 2009; Turner-Tomaszewicz et al. 2018). Epidermis is the most commonly used tissue in sea turtle isotope analyses because this allows researchers to validate diet composition with greater confidence than by using blood samples, which may be more suggestive of opportunistic sampling immediately prior to stranding. Vélez-Rubio et al. (2015) found that SIA conducted on epidermis tissue of stranded green turtles in the south-western Atlantic indicated an omnivorous diet composition up to several months prior to stranding. Williams et al. (2014) similarly found that green turtles that died during cold-stunning events had consistently been feeding on seagrasses, tunicates, and soft-bodied invertebrates prior to stranding. Understanding the diet composition of green turtles prior to stranding can provide important information about how diet choice is related to stranding risk and identify habitats of conservation priority.
In Australia, knowledge of green turtle foraging ecology is being continually developed, as traditional notions of herbivory within mature resident green turtles has been challenged (Bjorndal, 1997; Arthur et al. 2008, 2009; Prior et al. 2015). Furthermore, the need for improved understanding extends to understudied regions, such as New South Wales (NSW), where management of green turtle populations, including stranding events, could benefit from improved understanding of diet and habitat use. The primary aim of this study was to use stable-isotope analyses of tissues from stranded green turtles and their potential diet items along the NSW coastline, to better understand the diet and habitat use of stranded green turtles in NSW. The development of the first δ13C, δ15N, and δ34S isoscapes for sea turtles and diet items in NSW was incorporated to better understand diet choices and feeding locations prior to stranding. These data will provide a baseline for future research into the foraging ecology of green turtles in NSW and allow inference relating to potential threats in different areas and the drivers behind stranding events.
Methods
To ensure good resolution within the δ13C, δ15N, and δ34S isoscapes of the sampled green turtle diet sources, 11 geographically separate sites were chosen along ~1100 km of the NSW coastline (Fig. 1). In addition, green turtle tissue samples were collected from multiple management and conservation organisations responsible for cataloguing stranded green turtles along the NSW coastline. In total, 35 green turtle tissue samples were available from turtles that were stranded between 2018 and 2021, spanning 18 stranding locations, which were assigned to the following three major stranding regions: northern coast (n = 9), mid-northern coast (n = 18), and central coast (n = 8).
Green turtle diet sampling locations, and stranding locations from which green turtle tissue samples were provided. Blue markers indicate sampling locations for seagrass and algae. Yellow markers indicate sampling locations for mangroves. Triangles denote locations of stranding for sampled green turtles from 2018 to 2021, with triangle size indicating the number of stranding events (n). Dashed line indicates regional boundaries.
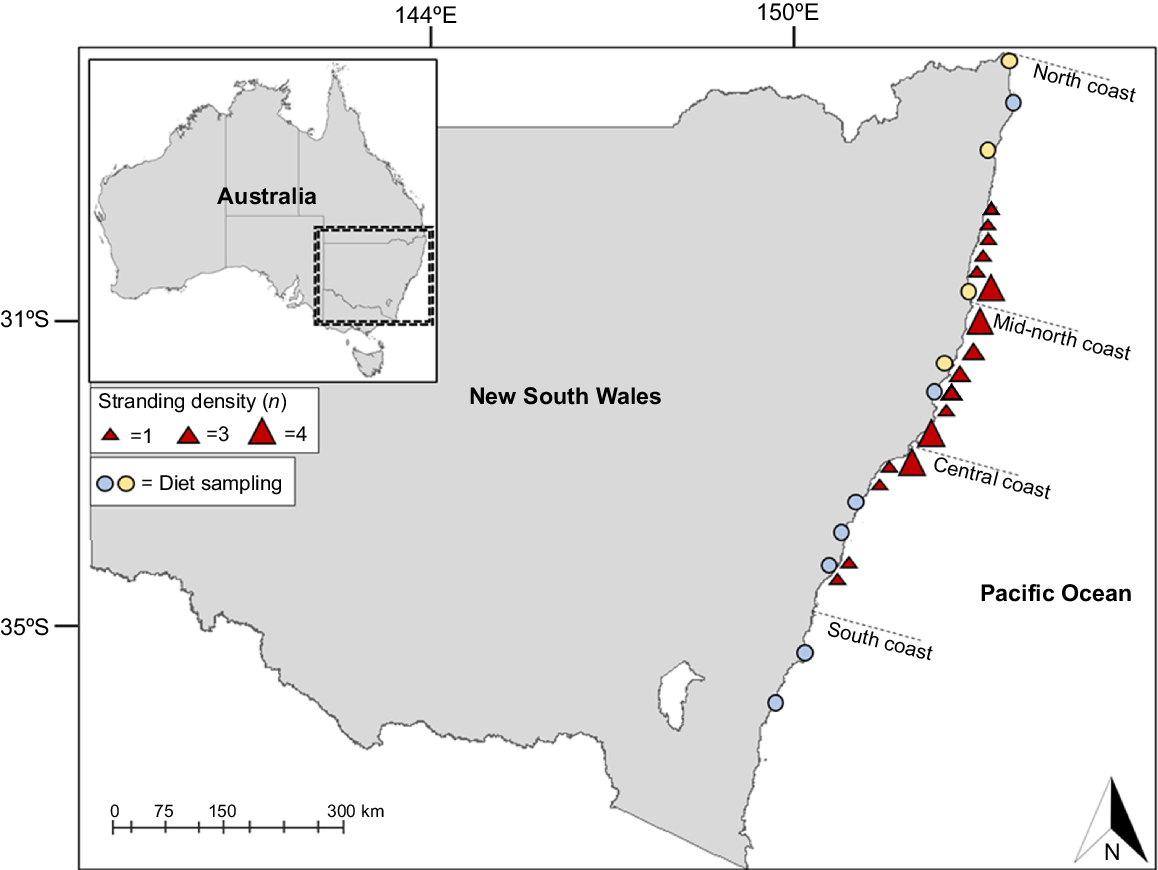
Green turtle diet-source sampling
Green turtle diet sources (seagrass, algae, and mangrove) were collected from 11 locations along the NSW coastline (Fig. 1), with three to five coastal or estuary sites (5 km2 radius) within each location selected for sampling, on the basis of accessibility and depth (<3 m). Habitat selection included intertidal and subtidal seagrass meadows, coastal rocky headlands, mangrove stands, and areas adjacent to artificial formations such as sea walls and boat ramps, representing typical foraging habitats for green turtles (Bjorndal 1997; Limpus 2009). Diet source selection was confined to taxa known to be consumed by green turtles within Australian waters (Arthur et al. 2008; Prior et al. 2015), with known distributions in NSW. Seagrass and algae were collected during southern hemisphere autumn months (2021), and commonly ingested mangrove species were sampled during winter months of the same year (Fig. 1). Mangrove sampling was restricted to the northern NSW coastline in 2021 because local COVID-19 restrictions at the time prevented further sampling. All algal groups (Rhodophyta, Chlorophyta, Phaeophyta) were combined, because there was no significant difference between isotopic values (one-way ANOVA, δ13C: degrees of freedom (d.f.) = 2, 57, F = 2.954, P = 0.06; δ15N: d.f. = 2, 57, F = 0.008, P = 0.99; and δ34S: d.f. = 2, 57, F = 8.351, P = 0.63). The inclusion of locally collected animal diet items was not possible in this study because of COVID-19 restrictions preventing access to sampling locations. However, δ13C, δ15N, and δ34S values for Ctenophora, Cephalopoda, and Crustacea (collectively termed as ‘animal material’), all known to be consumed by green turtles, were obtained from existing literature (Hatase et al. 2002, 2006; Shimada et al. 2014; Coffee et al. 2020 and associated data).
Turtle tissue sampling
Epidermis and full-thickness tissue samples were taken from the skin webbing on the posterior margins of the hind or fore flipper by using a 4 mm biopsy punch. A circular cut was made rapidly by rotating the tool while pressing down. The resulting disk of tissue was removed using forceps. In some cases, a small triangular notch of a similar size was removed with a sterile scalpel. The curved carapace length (CCL) of each turtle was measured between the nuchal notch and posterior tip of the carapace (Limpus et al. 1994). In total, 32 sampled turtles were considered juveniles (CCL 38–64 cm), two samples were considered subadults (CCL 65–86 cm) and one sample had no CCL data, but was reported as being within juvenile/subadult age class (Day et al. 2024). Owing to the opportunistic sampling associated with the stranded green turtles used in this study, it is possible that isotope values may have been affected by rates of decomposition during the days prior to sampling. In temperatures exceeding 20°C, bacterial decomposition is known to increase both δ13C and δ15N values after 3–4 days, with a significant enrichment after 14 days (Burrows et al. 2014; Keenan and DeBruyn 2019). However, Payo-Payo et al. (2013) illustrated that δ13C, δ15N values of loggerhead turtle epidermis did not significantly change over 62 days of decomposition. Of the 35 turtles sampled during this study, 29 were sampled from live animals, while under veterinary care, or during a post-mortem within 24 h from time of death. For the remaining samples, the timeframe for sampling stranded turtles found deceased is generally within 1 week because of limitations conducting post-mortems on decomposed carcasses. In addition, the storage of these samples was either frozen or in ethanol (>70%), likely inhibiting the enrichment of isotope values resulting from decomposition, because freezing and ethanol are known to greatly hinder bacterial growth (Ingram 1989; Dyrda et al. 2019).
Stable-isotope analyses
Green turtle epidermis and potential diet samples were lightly scrubbed and rinsed with distilled water to remove attached debris and epiphytes. Diet samples were stored frozen (−18°C) for preservation post-sampling (Barrow et al. 2008; Burrows et al. 2014). Epidermis samples were either frozen, or stored in 70% ethanol, then frozen, in line with methodology set out by Barrow et al. (2008). The effect of lipid extraction on the isotope values of epidermis values has been examined in previous literature, and due to the low lipid composition of sea turtle epidermis, lipid extraction is often considered unnecessary for this sample type (Post et al. 2007; Vander Zanden et al. 2012; Bergamo et al. 2016; Turner Tomaszewicz et al. 2017). Therefore, in this study, stable-isotope analyses were performed on non-lipid extracted samples. Acidification of samples to remove inorganic carbonate was also not performed, because this is known to alter both δ15N and δ34S values in plant, algae and animal samples (Connolly and Schlacher 2013), and inorganic carbonate was expected to be low in all samples. Diet samples were oven-dried for 24–48 h and ground to a fine consistency by using a mortar and pestle (Arthur et al. 2008, 2009; Reich et al. 2008). Standardised sample sizes (12–15 mg for plant and algae items; 6–10 mg for epidermis) were then placed into tin capsules, pelletised, and placed into an elemental analyser for combustion. Samples were analysed for carbon, nitrogen and sulfur stable isotopes in an IsoPrime isotope ratio mass spectrometer (with N2 and CO2 gases chromatographically separated) at the Stable Isotope Laboratory at Griffith University, Nathan Campus, Queensland (Qld), Australia. Elemental analysis provided δ13C, δ15N, and δ34S isotope values as a relative deviation (‰) from conventional standards, and expressed in delta (δ) notation in parts per thousand, as follows:
where Rsample is the ratio of heavy to light isotopes in the sample, and Rstandard is the isotope ratio of the corresponding international standard, Pee-Dee Belemnite for carbon, atmospheric air for nitrogen, and Canyon Diablo Troilite for sulfur. Laboratory-calibrated glycine and spinach (for diet sources), and bovine liver (for epidermis samples) were used during each run for control purposes. Standard deviation of the mean glycine, spinach and bovine liver samples confirmed the precision of analysis for diet and epidermis samples respectively.
For the turtle epidermis samples, a series of one-way ANOVAs (α = 0.05) was used to test the statistical differences in δ13C, δ15N, and δ34S values among turtle stranding locations (central, mid-north, north). Macrophyte stable-isotope values were used to generate isoscapes (see below). In addition, for each macrophyte (seagrass, algae, mangrove), statistical differences in δ13C, δ15N, and δ34S among sampling locations were tested using a series of one-way ANOVAs (α = 0.05). The statistical differences in overall δ13C, δ15N, and δ34S values among macrophytes were also tested using a series of one-way ANOVAs (α = 0.05).
Generating isoscapes
Spatial analyses for generating elemental isoscapes for green turtle diet sources were run in R (ver. 4.1.1; R Core Team 2021). Three sets of elemental isoscapes for δ13C, δ15N, and δ34S were generated for three major green turtle diet sources, namely, seagrass (n = 90), algae (n = 63), and mangrove (n = 21). Isoscapes were generated using spatial and mapping packages in R, with data from each sampling location, including specific geocoordinate locations associated with sampled foraging areas. A 20 km wide ‘buffer zone’ raster layer was constructed to map coastal geographic isotopic variations to enhance visualisation of isotopic trends at a large scale, and with spatial resolution set to 500 × 500-m pixels to account for sufficient levels of detail, given the amount of, and distance among, sample data values.
Each isoscape was modelled using a gaussian generalised additive mixed model (GAMM), with an identity-link function (Wood 2017) fit by restricted maximum likelihood by using the R package mgcv (Wood 2003). These models included a one-dimensional gaussian process (kriging) for Northing, and a random intercept for Species that accounted for isotopic differences among the sampled diet groups (e.g. Zostera muelleri for ‘seagrass’). Site-fold cross-validation (CV) was used to compare each model (excluding those for mangroves, which had insufficient data) to two other candidate models; a model with an additional random intercept for Site, and model that used a thin plate spline for Northing instead of the gaussian process. This investigation showed that our model outperformed the other two models at predicting interpolated data in four of six trials, on the basis of the root mean-square error. Gaussian processes that allowed for different levels of complexity were fit to ensure that the models adequately captured spatial relationships without overfitting the data. For each of the final models, the assumptions of normality and heteroscedasticity were investigated visually using Q–Q plots and standardised residual plots respectively, which showed no major concerns with the specification of these models. For each isotope value within each of the diet groups, there was a strong linear correspondence between values predicted by the models, and observed values from the sampling dataset, indicating that the models fit the data relatively well.
Following model selection and validation, the models were used to spatially interpolate areas among sampling locations, which allowed for the construction of isoscape maps along the entire NSW coastline. Further, GAMM models were restricted to predictions within the isotopic range of sampled diet sources, leading to only realistic values being predicted in the isoscapes.
Stable-isotope mixing models
The relative contribution of seagrass, macroalgae, mangrove and animal material was assessed for sampled turtles within and among all three stranding regions, with the availability of each diet source being assumed as continuously available to all study turtles. All statistical analyses were conducted using R software (ver. 4.1.1; R Core Team 2021). Isotope discrimination factors for green turtle epidermis were 2.3 ± 0.3‰ for δ13C and 4.1 ± 0.4‰ for δ15N (Turner Tomaszewicz et al. 2017), and 0‰ for δ34S (Raoult et al. 2024), and were applied to account for turtle-diet isotopic discrimination (Fry 2006). Stable-isotope mixing models for diet-source contributions were run in simmr (ver. 0.5.1.217; stable-isotope analysis in R; Govan and Parnell 2024), by using the Bayesian framework. The stable-isotope mixing models in R (SIMMR) utilise iterations of Markov-chain Monte Carlo algorithms for probability distributions (Moore and Semmens 2008). The model produced probability-density distributions for potential proportions of diet sources (Parnell et al. 2010). Normal distributions of the source data were confirmed, and the mean values for diet sources were examined for normality and homogeneity of variance, and were incorporated into the model to estimate the most probable contribution of each food source to the overall diet of each turtle.
Ethics statement
This research involved the collection and analysis of epidermis tissue derived from deceased and live Chelonia mydas, washed up along the NSW coastline. Live samples were collected under AEC 5c/08/19 and deceased sampled were collected under Taronga’s opportunistic sample request number R17B242. Macrophytes were collected under Scientific Collection Permit No. P20/0028-1.0 and Marine Park Permit MEAA20/294. Stranded turtles were processed and stored by local conservation organisations listed in the Acknowledgements, with samples transferred to the corresponding author.
Results
Regional trends in δ13C, δ15N, and δ34S values of macrophytes
This study produced the first known isoscapes for primary producers relevant to green turtle diets along the NSW coastline. The δ13C, δ15N, and δ34S isoscapes for seagrass (Fig. 2) and algae (Fig. 3) did not show distinct latitudinal trends. Conversely, the mangrove isoscapes displayed latitudinal variation within the range of sampling; isoscape for δ13C (Fig. 4a) exhibited progressive enrichment trending south, whereas δ15N (Fig. 4b) and δ34S (Fig. 4c) enrichment was most prominent heading north. For algae, δ13C and δ34S showed the highest variability along the coastline, with high levels of enrichment around the central coast (Fig. 3a, c). Both δ13C and δ34S values for algal samples were significantly different among sampling locations (ANOVA; δ13C: d.f. = 5, 57, F = 5.899, P = 1.84 × 10−4; δ34S: d.f. = 5, 57, F = 4.855, P = 9.09 × 10−4). δ15N for algae was not significantly different among locations (ANOVA; δ15N: d.f. = 5, 57, F = 2.212, P = 0.06). δ13C, δ15N, and δ34S values for seagrass were also highly variable and significantly different among sampling locations (ANOVA; δ13C: d.f. = 7, 82, F = 11.15, P = 7.91 × 10−10; δ15N: d.f. = 7, 82, F = 2.275, P = 0.03; δ34S: d.f. = 7, 82, F = 2.212, P = 0.04). For mangroves, significant differences among sampling locations were observed for δ15N values (ANOVA; δ15N: d.f. = 3, 17, F = 5.651, P = 7.12 × 10−2), but not for δ13C or δ34S (ANOVA; δ13C: d.f. = 3, 17, F = 1.708, P = 0.20; δ34S: d.f. = 3, 17, F = 0.609, P = 0.60).
Isoscapes of (a) δ13C, (b) δ15N, and (c) δ34S derived from seagrass samples across New South Wales and based on cross-validation of observed and predicted values. Black dots indicate sampling locations. All isotope values are in ‰.
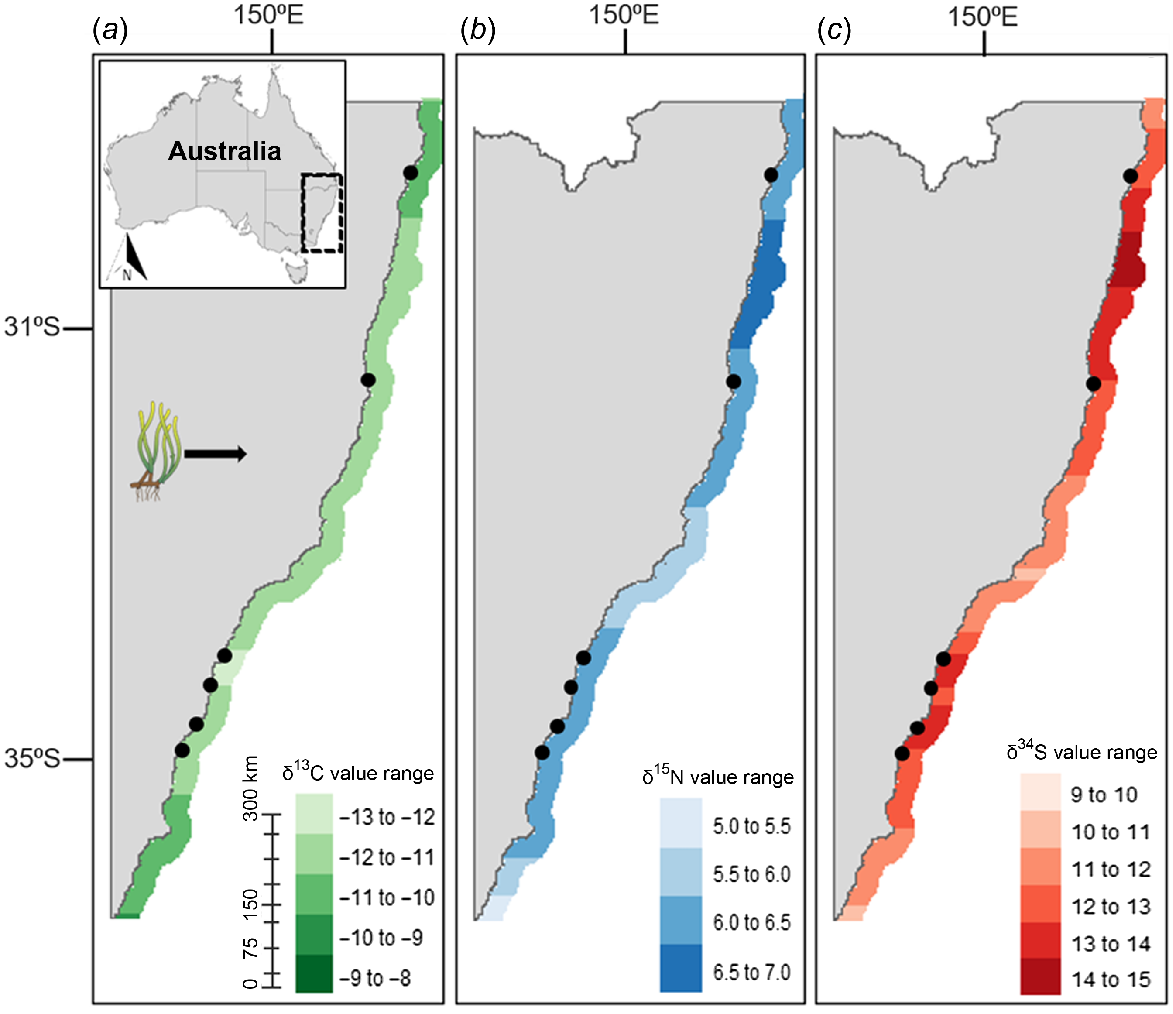
Comparing δ13C, δ15N, and δ34S values among macrophytes
The δ13C values of seagrass were highest, −10.67 ± 1.57‰, followed by macroalgae, −15.20 ± 2.20‰, mangroves, −28.50 ± 1.37‰ (Table 1, Fig. 5a, c). δ13C was significantly different among diet groups (ANOVA; d.f. = 2, 165, F = 837.3, P = 1.76 × 10−4). Mangrove had the lowest δ15N value, 5.07 ± 2.12‰, followed by seagrass, 6.03 ± 1.56‰, and macroalgae, 6.98 ± 1.26‰ (Table 1, Fig. 5a, b). δ15N was also significantly different among diet groups (ANOVA; d.f. = 2, 165, F = 14.29, P = 1.89 × 10−6). Mangrove had the lowest δ34S values (4.40 ± 5.04‰), whereas seagrass (13.15 ± 4.36‰), and macroalgae (16.80 ± 4.12‰) were much higher (Table 1, Fig. 5b, c). Algae and seagrass had similar δ34S values; however, the difference between seagrass and macroalgae δ34S values was statistically significant (ANOVA; δ34S: d.f. = 1, 37, F = 29.077, P = 3.27 × 10−7).
Diet source | δ13C (‰) | δ15N (‰) | δ34S (‰) | σ δ13C (‰) | σ δ15N (‰) | σ δ34S (‰) | |
---|---|---|---|---|---|---|---|
Seagrass | −10.67 | 6.03 | 13.14 | 1.57 | 1.56 | 4.36 | |
Algae | −15.20 | 6.98 | 16.80 | 2.20 | 1.26 | 4.12 | |
Mangrove | −28.50 | 5.07 | 4.40 | 1.37 | 2.12 | 5.04 | |
Animal material | −16.59 | 13.47 | 17.37 | 1.90 | 1.05 | 1.25 |
Cluster plots of isotope values δ15N against δ13C (a), δ34S against δ15N (b), and δ34S against δ13C (c) of green turtle diet sources (large circles), and green turtle epidermis samples (small diamonds; n = 36) sampled from various locations along the New South Wales coastline. Diet sources have been adjusted for trophic enrichment fractionation in green turtle epidermis (Turner Tomaszewicz et al. 2017). Error bars represent standard deviations. Animal material data were sourced from existing literature (Hatase et al. 2002, 2006; Shimada et al. 2014).
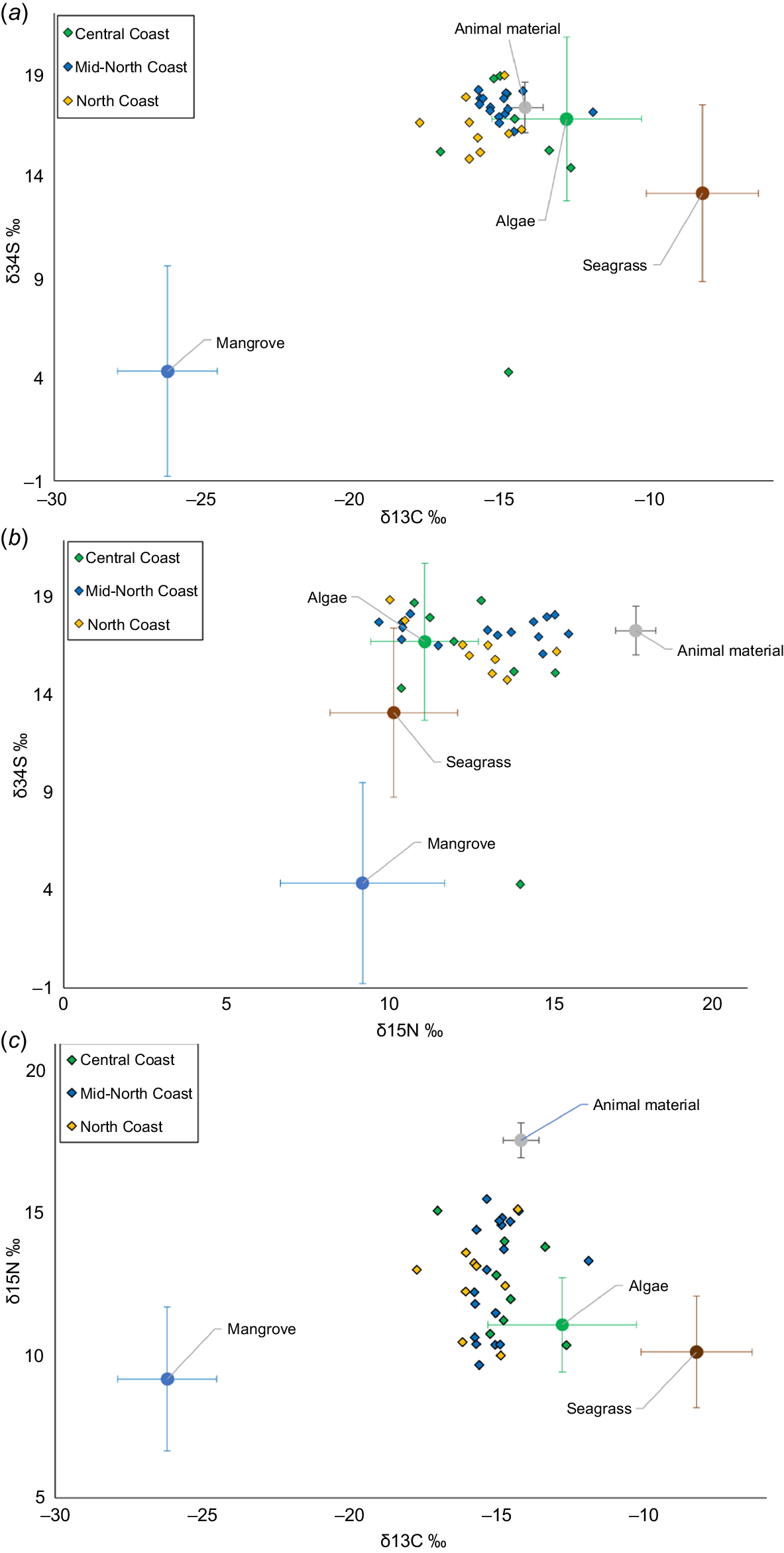
Comparing δ13C, δ15N, and δ34S values in green turtle epidermis
The mean δ13C, δ15N and δ34S values for green-turtle epidermis samples were not significantly different among locations (ANOVA; δ13C: d.f. = 2, 32, F = 2.480, P = 0.11; δ15N: d.f. = 2, 32, F = 0.109, P = 0.90; δ34S: d.f. = 2, 32, F = 02.104, P = 0.14). Data for all turtles were therefore pooled, and the values (mean ± s.d.) of δ13C, δ15N and δ34S were 15.31 ± 1.23‰, 12.84 ± 1.77‰, and 16.53 ± 2.53‰ respectively.
Stable-isotope mixing model analysis
A stable-isotope mixing model, representing feasible contributions of each food source to all sampled green-turtle diets over ~3 months prior to stranding indicated that macroalgae were the most prominent diet source (mean ± s.d. = 51.2 ± 6.1%) (Fig. 6). Dietary proportions of animal material were also a significant contribution to the diets of sampled green turtles (31.4 ± 4.7%), with mangrove (13.4 ± 2.0%) and seagrass (4.0 ± 2.5%) representing trivial proportions of overall diet composition. Further, the 2.5% and 97.5% credible intervals for macroalgae, animal matter, mangrove and seagrass were 38.1–62.3%, 22.5–41.1%, 9.5–17.3%, and 0.8–10.4% respectively. The wide ranges in credible intervals indicate that the dietary proportions of this model are quite uncertain. In addition, owing to COVID-19, mangrove sampling was limited to northern NSW only, and mangrove results within the mixing model may not reflect isotopic variations further south into NSW. For this study, stable-isotope values of sampled northern NSW mangroves were used to analyse the entire coastline, given the relatively low variation in mangrove stable-isotope values observed along the central and southern Qld coastlines (Prior et al. 2015). A high (negative) correlation between macroalgae and animal material (correlation = 85.5%) indicated that the combined (Fig. 6) and regional models (Supplementary Figs S1–S3) had trouble discerning between the two sources. This was expected, because the two sources lie closest together in δ13C, δ15N, and δ34S isotope values. Similar contributions from each diet source were observed within each stranding region (Figs S1–S3), with subtle changes made to the contribution of seagrass and mangrove material to overall diet contribution. The ecological relevance of the regional model findings should be interpreted with caution, because model uncertainty increases with low and uneven sample sizes.
Posterior distributions plot of the relative proportions of each diet source to the overall diet composition of sampled green turtles stranded along the NSW coastline, based on average δ13C, δ15N, and δ34S values of sampled diet items, animal material sourced from literature (see Methods: Green turtle diet-source sampling), and epidermis tissue from stranded green turtles, adjusting for trophic enrichment (Turner Tomaszewicz et al. 2017).
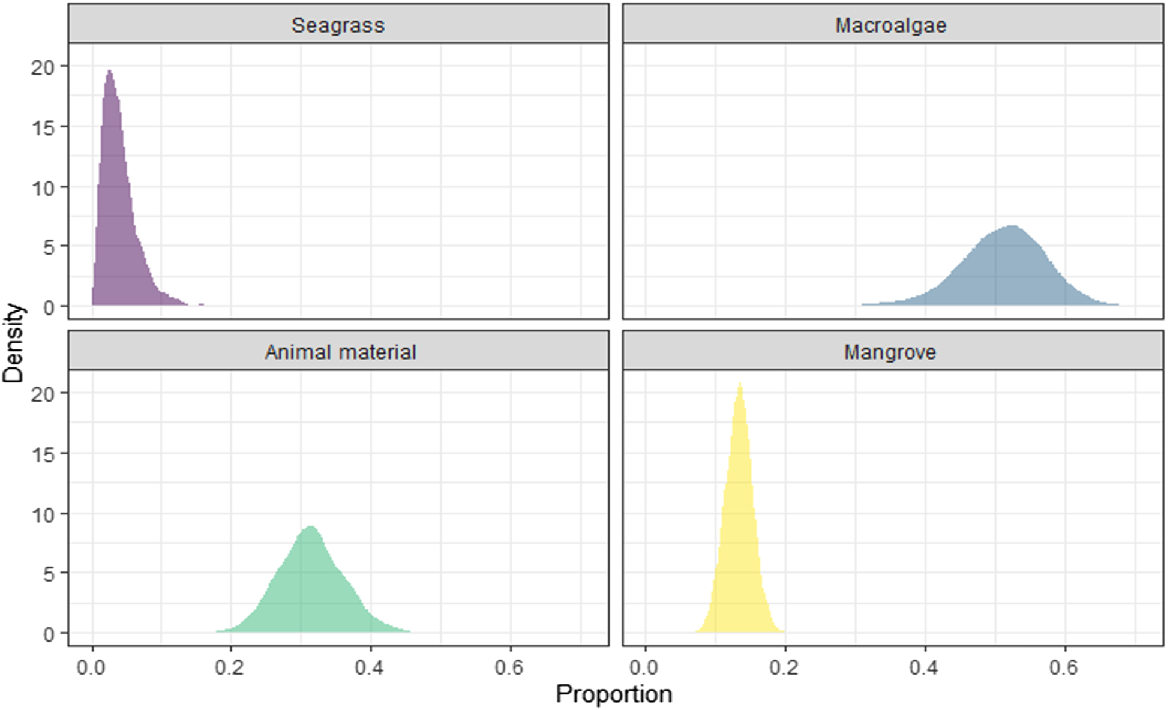
Discussion
This study has improved the understanding of the foraging ecology of green turtles prior to stranding in NSW. Isotope analyses of seagrass, mangroves, algae, and green turtle epidermis tissue, in combination with literature data on animal food sources, showed that a large proportion of sampled stranded turtles in NSW appear to be feeding at higher than expected trophic levels, and in food webs supported primarily by algae. Geographic variation in plant and algal isotopes did not demonstrate linear trends along latitudinal gradients, with high variation among locations for each diet source also found along the NSW coastline. This made it possible to make inferences about the proportional contribution of seagrass, mangrove, algae, and animal diet sources to the diet composition of stranded green turtles, but not possible to ascertain foraging locations from these analyses alone.
Green turtle diet isoscapes
The isotope values for green turtle diet sources in this study were comparable to those in recent research in Qld, Australia by Coffee et al. (2020), who showed that within-location variation was too high, and between location variation too low to infer habitat use of green turtles along the coastline. The variation and trends in the δ13C, δ15N, and δ34S isoscapes generated in this study were insufficient to infer the foraging locations that sampled green turtles inhabited prior to stranding along the NSW coastline. Minimal systematic latitudinal variation was coupled with high variation among locations for each diet source, and for δ13C, δ15N, and δ34S values. Variability in baseline isotope values can be affected by bottom-up processes, top-down processes, and anthropogenic influence (Peterson and Fry 1987), all of which require further research to better understand the influence each has on isotope values observed in NSW, and to estimate isotopic variation among different trophic groups (Ceriani et al. 2014; Coffee et al. 2020).
The high variation exhibited by isotope values within specific regions in this study may be associated with terrestrial run-off regimes within NSW, and influenced further by dynamic oceanic currents and weather patterns along the eastern Australian coastline (Rubenstein and Hobson 2004). Green turtle diet δ13C values in the northern Atlantic are influenced by increased nutrient cycling and turnover driven by the Gulf Stream, which is facilitated by the equatorial Loop Current of the Gulf of Mexico (Lee and Mellor 2003). These factors facilitate δ13C depletion in diet sources with an ascending latitude (Graham et al. 2010), allowing local isoscapes to be useful in assigning turtles to foraging locations (Ceriani et al. 2014). However, within Australian waters the southward-flowing East Australian Current extends from the South Equatorial Current, and converges with temperate currents within the geographical extent of NSW (Church 1987). This convergence of temperate and tropical currents could explain the high variation and lack of predictable trends in δ13C and δ15N values observed along the NSW coastline. In marine environments, fixation, denitrification, and sediment deposition, resulting from river outflows, influence values of δ15N (Fry 2006) and δ34S (Oakes and Connolly 2004) in marine sources, which may be further altered by increased nutrient and chemical deposition (Hobson 2019). Quantifying the influence of these factors along the NSW coastline would be beneficial to ascertain how these drivers affect regional isotopic variation of primary producers.
Although the foraging locations for stranded green turtles in NSW could not be determined in this study, the analyses are informative for environmental management practices. Mapping δ13C, δ15N and δ34S values at the base of marine food webs can be useful in identifying the effects of oceanic currents on photosynthetic biomass accumulation, and locating high levels of nutrient input (δ15N) over large distances (Durante et al. 2021). Sources of land-based run-off and areas of eutrophication can be hotspots of primary production, possibly resulting from anthropogenic inputs or weather events (Oczkowski et al. 2014), which can drive high nutrient concentrations and enriched δ15N values around river and estuary systems (Voss et al. 2000), alongside increased variation in δ34S values (Oakes and Connolly 2004). The information contained in the isoscapes presented in this study could therefore direct local management to areas increasingly affected by high nutrient outflow, especially if stemming from anthropogenic influence.
Stable-isotope mixing model analyses
The stable-isotope values of the epidermis samples from green turtles that had stranded along the NSW coastline were comparable to isotope values of stranded green turtles in the eastern Pacific Ocean (Turner-Tomaszewicz et al. 2018), as well as the North (Williams et al. 2014) and South Atlantic Ocean (Vélez-Rubio et al. 2015). Stable-isotope analysis within these studies stated that stranded green turtles were likely to be feeding both on benthic sources, and in the epipelagic zone in coastal waters (Turner-Tomaszewicz et al. 2018), primarily on neritic animal sources such as jellyfish and cephalopods (Vélez-Rubio et al. 2015), and tunicates (Williams et al. 2014). In Australia, similarities appear between stable-isotope values of green turtles further north in Queensland and this study. Stable-isotope analysis from Prior et al. (2015) exhibited non-trivial amounts of neritic animal matter in the diet compositions of sampled green turtles. Similarly, findings from this current study demonstrated high δ15N values that indicate feeding at higher trophic levels, rather than on primary producers themselves. Our analyses indicated that algae are the primary diet source at the base of the food web for the sampled stranded turtles in NSW, with most individuals likely to be feeding on algivores, or consumers of algivores. These findings support recent evidence of green turtle omnivory (Esteban et al. 2020), which has challenged the long-standing belief that green turtles are obligate herbivores after recruiting to coastal foraging areas (Cardona et al. 2009; Piovano et al. 2020).
Our improved understanding of the diet composition of stranded green turtles presented in this study can direct efforts to manage important sea turtle habitats along the NSW coastline. The simmr results indicate a strong reliance on algal dominated food webs, in comparison to seagrass- and mangrove-supported systems. Long-term observational studies and stable-isotope research in Qld, and elsewhere, have established seagrass as a prominent diet source for neritic green turtles (Limpus et al. 1994; Arthur et al. 2009; Prior et al. 2015). Large bays and estuary systems in Queensland facilitate large seagrass meadow areas. Conversely, seagrass is less prevalent in NSW, because habitat suitability is more ideal for algae species, notably kelp, which features more prominently (Kennelly and Underwood 1993). The algae-dominated diet of green turtles foraging in NSW therefore illustrates the plasticity of green turtle foraging ecology and the ability of green turtles to adapt their diet to available food sources (Figgener et al. 2019; Esteban et al. 2020). NSW hosts convergences of temperate and tropical currents, potentially altering green turtle diet preferences, but the likelihood of passive drift, disease, and food availability should also be investigated in future studies. These findings point to protecting habitats that both support algal production and give refuge to green turtles, as opposed to the focus on protecting seagrass meadows, the dominant food source for green turtles in Qld (Limpus 2009).
Consideration of study parameters and model caveats in future studies
The isoscapes presented in this study are constrained by the limited quantity of source data and, as such, utilize the most workable model parameters to accurately interpolate data at the displayed scale. This resulted in displayed patterns not exhibiting the highest possible detail because of confounding effects and limited degrees of freedom used to construct the model parameters. Hence, the predictions made among sampling locations, particularly across large distances, are associated with a higher degree of error. In the case of the mangrove isoscapes (Fig. 3), latitudinal variation can be seen for δ13C, δ15N, and δ34S values. This could imply an increase in photosynthetic biomass from enriched δ13C, and reductions in nutrient outflow as interpreted by depleted δ15N, and δ34S values to the south (Peterson and Fry 1987). This provides a baseline understanding, but the isoscapes can be improved by filling in the gaps, especially in regions where source data are not available yet. Another caveat to consider would be the validation of results if stranded turtle foraging behaviour could be reliably inferred within the sampling regions. The additional sampling of healthy turtles along the NSW coastline would assist in the validation of results.
Mixing-model results are limited in interpretation because of similar isotope values for sampled diet sources, unknown stranding locations limiting direct comparisons to sampled diet sources in relevant regions, and model uncertainty stemming from low sampling efforts. The model indicated algae as a major contributor to the food web in which these stranded turtles were feeding, although the strong negative correlation between algae and animal material indicated that mixing-model uncertainty was high when discerning between algae and animal proportions. Model uncertainty may increase estimates of source proportions and alter interpretations (Fry 2006). A mixing-model analysis with more source data (n) and incorporation of stomach contents analysis would oppose the influence of model uncertainty (Piovano et al. 2020). Because of the nature of stranding, the ability to directly analyse diet sources and stranded turtles from similar regions is not reliable, because deceased turtles can drift for many kilometres before washing ashore (Koch et al. 2013). Future research can explore this avenue further by sampling turtles of known foraging location. Finally, model results can be reinforced by the sampling of live and healthy individuals (e.g. tissue sampling and stomach lavage) caught in areas of increased stranding incidence, as a direct indication of diet proportion indications (Vélez-Rubio et al. 2016).
Conclusions
This study used stable-isotope analyses to assess the relationship among diet, stranding, and foraging locations of green turtles in NSW. In contrast to the northern hemisphere, insufficient systematic latitudinal variation and high variation among locations within sampled macrophyte items along the NSW coastline made inferring foraging location of stranded green turtles unreliable. However, it appears that green turtles that stranded in NSW, fed predominantly within algae-based food webs, with high proportions of animal matter in their diet. This may be due to the known flexibility of green turtle foraging ecology (Figgener et al. 2019; Esteban et al. 2020), illustrating an adaption to more available algal food sources in NSW. Whether healthy, free-ranging populations of green turtles in NSW are also foraging primarily on algae requires further investigation. Local management could benefit from closer scrutiny of algae-dominated habitats in areas proximal to green turtle stranding events in NSW, because the novel findings can inform habitat management and the identification of biologically important sites for the species (e.g. the release of rehabilitated green turtles into algae-dominated habitats to increase long-term survivability). Future studies should incorporate the effects of climate change on the structure and health of algae-dominated habitats along the NSW coastline. The plant and algae isoscapes also provide a practical baseline for future assessments of geological cycles along the NSW coastline and have relevance in identifying areas of anthropogenic influence and how they interact with nearby coastal dynamics.
Data availability
The datasets and supplementary materials generated for this study are available upon request to the corresponding author. A pre-print version of this paper is available at: https://www.researchsquare.com/article/rs-2335761/v1.
Declaration of funding
This research, including field logistics, equipment, and analysis, was funded by both the Taronga Conservation Society and the Australian Rivers Institute (Griffith University). Thanks go to the Wettenhall Environment Trust and James N. Kirby Foundation for contributions to funding.
Author contributions
Authors listed on this paper all contributed to either the data collection, analysis, interpretation and the writing and editing of this paper.
Acknowledgements
This research was supported by Taronga Conservation Society Australia who hold all relevant ethics and field permits related to this research. Special thanks to Bronwyn Palmer, Kaylee Dodd, Safaira Osborn, and Talia Murray, for assistance with field and laboratory work, and Vanessa Fry and Rad Bak at the Stable Isotope Laboratory, Griffith University, Nathan Campus. Thanks to Australian Turtle and Seabird Rescue; Irukandji Shark and Ray Centre; Taronga Wildlife Hospital; Dolphin Marine Conservation Park; Australian Registry for Wildlife Health; Australian Museum for turtle tissue curation and collection.
References
Arthur KE, Boyle MC, Limpus CJ (2008) Ontogenetic changes in diet and habitat use in green sea turtle (Chelonia mydas) life history. Marine Ecology Progress Series 362, 303-311.
| Crossref | Google Scholar |
Arthur KE, McMahon KM, Limpus CJ, Dennison WC (2009) Feeding ecology of green turtles (Chelonia mydas) from Shoalwater Bay, Australia. Marine Turtle Newsletter 123, 6-12.
| Google Scholar |
Barrow LM, Bjorndal KA, Reich KJ (2008) Effects of preservation method on stable carbon and nitrogen isotope values. Physiological and Biochemical Zoology 81(5), 688-693.
| Crossref | Google Scholar | PubMed |
Bergamo TF, Botta S, Copertino M (2016) Lipid extraction in stable isotope analyses of juvenile sea turtle skin and muscle. Aquatic Biology 25, 1-6.
| Crossref | Google Scholar |
Bradshaw PJ, Broderick AC, Carreras C, Inger R, Fuller W, Snape R, Stokes KL, Godley BJ (2017) Satellite tracking and stable isotope analysis highlight differential recruitment among foraging areas in green turtles. Marine Ecology Progress Series 582, 201-214.
| Crossref | Google Scholar |
Burrows DG, Reichert WL, Bradley Hanson M (2014) Effects of decomposition and storage conditions on the δ13C and δ15N isotope values of killer whale (Orcinus orca) skin and blubber tissues. Marine Mammal Science 30(2), 747-762.
| Crossref | Google Scholar |
Cardona L, Aguilar A, Pazos L (2009) Delayed ontogenic dietary shift and high levels of omnivory in green turtles (Chelonia mydas) from the NW coast of Africa. Marine Biology 156(7), 1487-1495.
| Crossref | Google Scholar |
Ceriani SA, Roth JD, Sasso CR, McClellan CM, James MC, Haas HL, Smolowitz RJ, Evans DR, Addison DS, Bagley DA, Ehrhart LM, Weishampel JF (2014) Modeling and mapping isotopic patterns in the Northwest Atlantic derived from loggerhead sea turtles. Ecosphere 5(9), 1-24.
| Crossref | Google Scholar |
Cheng IJ, Wang HY, Hsieh WY, Chan YT (2019) Twenty-three years of sea turtle stranding/bycatch research in Taiwan. Zoological Studies 58, e44.
| Crossref | Google Scholar |
Church JA (1987) East Australian current adjacent to the Great Barrier Reef. Marine and Freshwater Research 38(6), 671-683.
| Crossref | Google Scholar |
Coffee OI, Booth DT, Thia JA, Limpus CJ (2020) When isotopes fail: importance of satellite telemetry and multi-site validation when estimating the foraging grounds of migratory species. Marine Ecology Progress Series 633, 197-206.
| Crossref | Google Scholar |
Connolly RM, Schlacher TA (2013) Sample acidification significantly alters stable isotope ratios of sulfur in aquatic plants and animals. Marine Ecology Progress Series 493, 1-8.
| Crossref | Google Scholar |
Day J, Hall J, Rose K, Vinette Herrin K, March D, Pitt O, Fitzsimmons NN, Hall L, Marshall K, Iredall S, Meagher P (2024) Mixed stock analysis identifies natal origins of green turtles at foraging grounds in southeastern Australia. Frontiers in Marine Science 11, 1346932.
| Crossref | Google Scholar |
Deniro MJ, Epstein S (1981) Influence of diet on the distribution of nitrogen isotopes in animals. Geochimica et Cosmochimica Acta 45(3), 341-351.
| Crossref | Google Scholar |
Durante LM, Smith RO, Kolodzey S, McMullin RM, Salmond NH, Schlieman CD, O’Connell-Milne SA, Frew RD, Van Hale R, Wing SR (2021) Oceanographic transport along frontal zones forms carbon, nitrogen, and oxygen isoscapes on the east coast of New Zealand: implications for ecological studies. Continental Shelf Research 216, 104368.
| Crossref | Google Scholar |
Dyrda G, Boniewska-Bernacka E, Man D, Barchiewicz K, Słota R (2019) The effect of organic solvents on selected microorganisms and model liposome membrane. Molecular Biology Reports 46(3), 3225-3232.
| Crossref | Google Scholar | PubMed |
Esteban N, Mortimer JA, Stokes HJ, Laloë J-O, Unsworth RKF, Hays GC (2020) A global review of green turtle diet: sea surface temperature as a potential driver of omnivory levels. Marine Biology 167, 183.
| Crossref | Google Scholar |
Figgener C, Bernardo J, Plotkin PT (2019) Beyond trophic morphology: stable isotopes reveal ubiquitous versatility in marine turtle trophic ecology. Biological Reviews 94(6), 1947-1973.
| Crossref | Google Scholar | PubMed |
Flint J, Flint M, Limpus CJ, Mills PC (2015) Trends in marine turtle strandings along the east Queensland, Australia coast, between 1996 and 2013. Journal of Marine Biology 2015, 848923.
| Crossref | Google Scholar |
Flint J, Flint M, Limpus CJ, Mills PC (2017) The impact of environmental factors on marine turtle stranding rates. PLoS ONE 12(8), e0182548.
| Crossref | Google Scholar | PubMed |
Fuentes MMPB, Gredzens C, Bateman BL, Boettcher R, Ceriani SA, Godfrey MH, Helmers D, Ingram DK, Kamrowski RL, Pate M, Pressey RL, Radeloff VC (2016) Conservation hotspots for marine turtle nesting in the United States based on coastal development. Ecological Applications 26(8), 2708-2719.
| Crossref | Google Scholar |
Fuentes MMPB, Allstadt AJ, Ceriani SA, Godfrey MH, Gredzens C, Helmers D, Ingram D, Pate M, Radeloff VC, Shaver DJ, Wildermann N, Taylor L, Bateman BL (2020) Potential adaptability of marine turtles to climate change may be hindered by coastal development in the USA. Regional Environmental Change 20(3), 104.
| Crossref | Google Scholar |
Govan E, Parnell A (2024) SIMMR: A Stable Isotope Mixing Model. Available at https://CRAN.R-project.org/package=simmr
Graham BS, Koch PL, Newsome SD, McMahon KW, Aurioles D (2010) Using isoscapes to trace the movements and foraging behavior of top predators in oceanic ecosystems. In ‘Isoscapes’. (Eds J West, G Bowen, T Dawson, K Tu) pp. 299–318. (Springer: Dordrecht, Netherlands) 10.1007/978-90-481-3354-3_14
Hatase H, Takai N, Matsuzawa Y, Sakamoto W, Omuta K, Goto K, Arai N, Fujiwara T (2002) Size-related differences in feeding habitat use of adult female loggerhead turtles Caretta caretta around Japan determined by stable isotope analyses and satellite telemetry. Marine Ecology Progress Series 233, 273-281.
| Crossref | Google Scholar |
Hatase H, Sato K, Yamaguchi M, Takahashi K, Tsukamoto K (2006) Individual variation in feeding habitat use by adult female green sea turtles (Chelonia mydas): are they obligately neritic herbivores? Oecologia 149(1), 52-64.
| Crossref | Google Scholar | PubMed |
Hays GC, Schofield G, Papazekou M, Chatzimentor A, Katsanevakis S, Mazaris AD (2024) A pulse check for trends in sea turtle numbers across the globe. iScience 27(3), 109071.
| Crossref | Google Scholar | PubMed |
Haywood JC, Fuller WJ, Godley BJ, Shutler JD, Widdicombe S, Broderick AC (2019) Global review and inventory: how stable isotopes are helping us understand ecology and inform conservation of marine turtles. Marine Ecology Progress Series 613, 217-245.
| Crossref | Google Scholar |
Hobson KA (1999) Tracing origins and migration of wildlife using stable isotopes: a review. Oecologia 120(3), 314-326.
| Crossref | Google Scholar | PubMed |
Ingram LO (1989) Ethanol tolerance in bacteria. Critical Reviews in Biotechnology 9(4), 305-319.
| Crossref | Google Scholar |
IUCN (2021) The IUCN Red List of Threatened Species. Version 2021-1. Available at https://www.iucnredlist.org [Accessed 30 November 2021]
Jeremy JBC, Kirby MX, Berger WH, Bjorndal KA, Botsford LW, Bourque BJ, Bradbury RH, Cooke R, Erlandson J, Estes JA, Hughes TP, Kidwell S, Lange CB, Lenihan HS, Pandolfi JM, Peterson CH, Steneck RS, Tegner MJ, Warner RR (2001) Historical overfishing and the recent collapse of coastal ecosystems. Science 293(5530), 629-637.
| Crossref | Google Scholar |
Keenan SW, DeBruyn JM (2019) Changes to vertebrate tissue stable isotope (δ15N) composition during decomposition. Scientific Reports 9(1), 9929.
| Crossref | Google Scholar | PubMed |
Kennelly SJ, Underwood AJ (1993) Geographic consistencies of effects of experimental physical disturbance on understorey species in sublittoral kelp forests in central New South Wales. Journal of Experimental Marine Biology and Ecology 168(1), 35-58.
| Crossref | Google Scholar |
Koch V, Peckham H, Mancini A, Eguchi T (2013) Estimating at-sea mortality of marine turtles from stranding frequencies and drifter experiments. PLoS ONE 8(2), e56776.
| Crossref | Google Scholar | PubMed |
Lamont MM, Fujisaki I, Stephens BS, Hackett C (2015) Home range and habitat use of juvenile green turtles (Chelonia mydas) in the northern Gulf of Mexico. Animal Biotelemetry 3(1), 53.
| Crossref | Google Scholar |
Lee H-C, Mellor GL (2003) Numerical simulation of the gulf stream system: the loop current and the deep circulation. Journal of Geophysical Research: Oceans 108(C2), 3043.
| Crossref | Google Scholar |
Limpus CJ, Couper P, Read MA (1994) The green turtle, Chelonia mydas, in Queensland: population structure in a warm temperature feeding area. Memoirs of the Queensland Museum 35, 139-154.
| Google Scholar |
Monteiro DS, Estima SC, Gandra TBR, Silva AP, Bugoni L, Swimmer Y, Seminoff JA, Secchi ER (2016) Long-term spatial and temporal patterns of sea turtle strandings in southern Brazil. Marine Biology 163(12), 247.
| Crossref | Google Scholar |
Moore JW, Semmens BX (2008) Incorporating uncertainty and prior information into stable isotope mixing models. Ecology Letters 11(5), 470-480.
| Crossref | Google Scholar | PubMed |
Oakes JM, Connolly RM (2004) Causes of sulfur isotope variability in the seagrass, Zostera capricorni. Journal of Experimental Marine Biology and Ecology 302(2), 153-164.
| Crossref | Google Scholar |
Oczkowski A, Markham E, Hanson A, Wigand C (2014) Carbon stable isotopes as indicators of coastal eutrophication. Ecological Applications 24(3), 457-466.
| Crossref | Google Scholar | PubMed |
Parnell AC, Inger R, Bearhop S, Jackson AL (2010) Source partitioning using stable isotopes: coping with too much variation. PLoS ONE 5(3), e9672.
| Crossref | Google Scholar | PubMed |
Payo-Payo A, Ruiz B, Cardona L, Borrell A (2013) Effect of tissue decomposition on stable isotope signatures of striped dolphins Stenella coeruleoalba and loggerhead sea turtles Caretta caretta. Aquatic Biology 18(2), 141-147.
| Crossref | Google Scholar |
Pearson RM, van de Merwe JP, Limpus CJ, Connolly RM (2017) Realignment of sea turtle isotope studies needed to match conservation priorities. Marine Ecology Progress Series 583, 259-271.
| Crossref | Google Scholar |
Peterson BJ, Fry B (1987) Stable isotopes in ecosystem studies. Annual Review of Ecology and Systematics 18(1), 293-320.
| Crossref | Google Scholar |
Piovano S, Lemons GE, Ciriyawa A, Batibasaga A, Seminoff JA (2020) Diet and recruitment of green turtles in Fiji, South Pacific, inferred from in-water capture and stable isotope analysis. Marine Ecology Progress Series 640, 201-213.
| Crossref | Google Scholar |
Post DM, Layman CA, Arrington DA, Takimoto G, Quattrochi J, Montaña CG (2007) Getting to the fat of the matter: models, methods and assumptions for dealing with lipids in stable isotope analyses. Oecologia 152(1), 179-189.
| Crossref | Google Scholar | PubMed |
Prior B, Booth DT, Limpus CJ (2015) Investigating diet and diet switching in green turtles (Chelonia mydas). Australian Journal of Zoology 63(6), 365-375.
| Crossref | Google Scholar |
R Core Team (2021) R: a language and environment for statistical computing. R Foundation for Statistical Computing, Vienna, Austria. Available at https://www.R-project.org/
Raoult V, Phillips AA, Nelson J, Niella Y, Skinner C, Tilcock MB, Burke PJ, Szpak P, James WR, Harrod C (2024) Why aquatic scientists should use sulfur stable isotope ratios (34S) more often. Chemosphere 355, 141816.
| Crossref | Google Scholar | PubMed |
Reich KJ, Bjorndal KA, Martinez Del Rio C (2008) Effects of growth and tissue type on the kinetics of 13C and 15N incorporation in a rapidly growing ectotherm. Oecologia 155(4), 651-663.
| Crossref | Google Scholar | PubMed |
Rubenstein DR, Hobson KA (2004) From birds to butterflies: animal movement patterns and stable isotopes. Trends in Ecology & Evolution 19(5), 256-263.
| Crossref | Google Scholar | PubMed |
Seminoff JA (2004) Chelonia mydas. The IUCN Red List of Threatened Species 2004: e.T4615A11037468. Available at http://doi.org/10.2305/IUCN.UK.2004.RLTS.T4615A11037468.en [Accessed 4 March 2022]
Seminoff JA, Resendiz A, Nichols WJ (2002) Home range of green turtles Chelonia mydas at a coastal foraging area in the Gulf of California, Mexico. Marine Ecology Progress Series 242, 253-265.
| Crossref | Google Scholar |
Servis JA, Lovewell G, Tucker AD (2015) Diet analysis of subadult Kemp’s ridley (Lepidochelys kempii) turtles from west-central Florida. Chelonian Conservation and Biology 14(2), 173-181.
| Crossref | Google Scholar |
Shimada T, Aoki S, Kameda K, Hazel J, Reich K, Kamezaki N (2014) Site fidelity, ontogenetic shift and diet composition of green turtles Chelonia mydas in Japan inferred from stable isotope analysis. Endangered Species Research 25(2), 151-164.
| Crossref | Google Scholar |
Shimada T, Limpus CJ, Hamann M, Bell I, Esteban N, Groom R, Hays GC (2020) Fidelity to foraging sites after long migrations. Journal of Animal Ecology 89(4), 1008-1016.
| Crossref | Google Scholar | PubMed |
Torniainen J, Lensu A, Vuorinen PJ, Sonninen E, Keinänen M, Jones RI, Patterson WP, Kiljunen M (2017) Oxygen and carbon isoscapes for the Baltic Sea: testing their applicability in fish migration studies. Ecology and Evolution 7(7), 2255-2267.
| Crossref | Google Scholar | PubMed |
Türkozan O, Özdilek ŞY, Ergene S, Uçar AH, Sönmez B, Yılmaz C, Kaçar Y, Aymak C (2013) Strandings of loggerhead (Caretta caretta) and green (Chelonia mydas) sea turtles along the eastern Mediterranean coast of Turkey. The Herpetological Journal 23(1), 11-15.
| Google Scholar |
Turner Tomaszewicz CN, Seminoff JA, Price M, Kurle CM (2017) Stable isotope discrimination factors and between-tissue isotope comparisons for bone and skin from captive and wild green sea turtles (Chelonia mydas). Rapid Communications in Mass Spectrometry 31(22), 1903-1914.
| Crossref | Google Scholar | PubMed |
Turner-Tomaszewicz CN, Seminoff JA, Avens L, Goshe LR, Rguez-Baron JM, Peckham SH, Kurle CM (2018) Expanding the coastal forager paradigm: long-term pelagic habitat use by green turtles Chelonia mydas in the eastern Pacific Ocean. Marine Ecology Progress Series 587, 217-234.
| Crossref | Google Scholar |
Vander Zanden HB, Bjorndal KA, Mustin W, Ponciano JM, Bolten AB (2012) Inherent variation in stable isotope values and discrimination factors in two life stages of green turtles. Physiological and Biochemical Zoology 85(5), 431-441.
| Crossref | Google Scholar | PubMed |
Vélez-Rubio GM, Tomás J, Míguez-Lozano R, Xavier JC, Martinez-Souza G, Carranza A (2015) New insights in Southwestern Atlantic Ocean Oegopsid squid distribution based on juvenile green turtle (Chelonia mydas) diet analysis. Marine Biodiversity 45(4), 701-709.
| Crossref | Google Scholar |
Vélez-Rubio GM, Cardona L, López-Mendilaharsu M, Martínez Souza G, Carranza A, González-Paredes D, Tomás J (2016) Ontogenetic dietary changes of green turtles (Chelonia mydas) in the temperate southwestern Atlantic. Marine Biology 163(3), 57.
| Crossref | Google Scholar |
Verutes GM, Huang C, Estrella RR, Loyd K (2014) Exploring scenarios of light pollution from coastal development reaching sea turtle nesting beaches near Cabo Pulmo, Mexico. Global Ecology and Conservation 2, 170-180.
| Crossref | Google Scholar |
Voss M, Larsen B, Leivuori M, Vallius H (2000) Stable isotope signals of eutrophication in Baltic Sea sediments. Journal of Marine Systems 25(3), 287-298.
| Crossref | Google Scholar |
Wallace BP, Lewison RL, McDonald SL, McDonald RK, Kot CY, Kelez S, Bjorkland RK, Finkbeiner EM, Helmbrecht S, Crowder LB (2010) Global patterns of marine turtle bycatch. Conservation Letters 3(3), 131-142.
| Crossref | Google Scholar |
Williams NC, Bjorndal KA, Lamont MM, Carthy RR (2014) Winter diets of immature green turtles (Chelonia mydas) on a northern feeding ground: Integrating stomach contents and stable isotope analyses. Estuaries and Coasts 37(4), 986-994.
| Crossref | Google Scholar |
Wolff NH, Mumby PJ, Devlin M, Anthony KRN (2018) Vulnerability of the Great Barrier Reef to climate change and local pressures. Global Change Biology 24(5), 1978-1991.
| Crossref | Google Scholar | PubMed |
Wood SN (2003) Thin-Plate regression splines. Journal of the Royal Statistical Society (B) 65(1), 95-114.
| Google Scholar |
Wood SN (2017) ‘Generalized additive models: an introduction with R,’ 2nd edn. (Chapman and Hall/CRC: New York, NY, USA) 10.1201/9781315370279