Stronger together: different community science platforms all contribute to wildlife research
Lucas Rodriguez Forti A B , Ana Marta P. R. da Silva Passetti B , Talita Oliveira C , Kauane Freitas C , Guilherme de Freitas Costa C , Juan Victor de Lima Maia C , Arthur Queiros C , Maria Alice Dantas Ferreira Lopes C and Judit K. Szabo
A
B
C
D
Abstract
Engaging the general public can increase spatio-temporal coverage of wildlife monitoring. Given the potentially substantial costs, we need to evaluate the contribution of known and planned initiatives and confirm whether multiple platforms increase the efficiency of data collection. As observer behaviour affects data quantity and quality, users of specialised and generalist platforms are expected to behave differently, resulting in more connected networks for specialised and higher nestedness for generalist platforms. Specialist observers are expected to contribute a balanced ratio of rare and common species, whereas non-specialist contribution will depend more on species detectability.
We aim to evaluate whether the combined contribution of observers from different platforms can improve the quality of occurrence and distribution data of 218 endemic Atlantic Forest bird species in Brazil. We also describe and compare observer-bird species interaction networks to illustrate observer behaviour on different platforms.
On the basis of data from five community science platforms in Brazil, namely, eBird, WikiAves, Biofaces, iNaturalist and Táxeus, we compared the spatial distribution of bird observations, the number of observers, the presence of the same observers on various platforms, bird species coverage, and the proportion of duplicate observations within and among platforms.
Although species coverage of the joint dataset increased by up to 100%, spatial completeness among the five platforms was low. The network of individual platforms had low values of clustering, and the network of the joint dataset had low connectance and high nestedness.
Each platform had a somewhat unique contribution. Pooling these datasets and integrating them with standardised data can inform our knowledge on bird distributions and trends in this fragile biome. Nevertheless, we encourage observers to provide precise coordinates, dates and other data (and platforms to accommodate such data) and recommend submitting data from all platforms into the Global Biodiversity Information Facility to support wildlife research and conservation.
If new platforms engage more and different people, new initiatives can cover poorly represented areas and successfully expand monitoring effort for Atlantic Forest endemic bird species.
Keywords: biodiversity, Brazil, citizen science, conservation, mobile nature apps, network analysis, participatory monitoring, public engagement.
Introduction
Community (citizen) science, i.e. involving the public in scientific research (Bonney 2021; Vohland et al. 2021), has profoundly changed the way biodiversity is studied, especially by facilitating large-scale and long-term monitoring of wildlife (Ellwood et al. 2020). With the exponential increase of digital data and the routine use of smartphones worldwide, community science has gained a primary role (Callaghan et al. 2021). There are now 1000s of mobile apps available for biodiversity-data collection, many based on the gamification of nature (Fraisl et al. 2022). For certain taxonomic groups, particularly birds, butterflies, and frogs, large datasets have been accumulated (Troudet et al. 2017; Rowley et al. 2019; Sanderson et al. 2021; Forti and Szabo 2023). The resulting databases often supply important data for biodiversity repositories, such as the Global Biodiversity Information Facility (GBIF) (Callaghan et al. 2020; Jarić et al. 2020). Analysing these curated large-scale and long-term datasets have shed light on various aspects of ecology and natural history (Howard and Davis 2009; Szabo et al. 2010; Hurlbert and Liang 2012; Forti et al. 2022a, 2022b). These studies also provide evidence for conservation decision-making (Dennis et al. 2017; Newman et al. 2017).
Community-collected data are of utmost importance for wildlife research, predominantly for taxa that are attractive to a human observer and are easy to identify, such as some chordates, vascular plants and arthropods (particularly Lepidoptera) (Troudet et al. 2017; Mesaglio and Callaghan 2021). Nevertheless, the quality and therefore the effective contribution of particular initiatives focused on mapping species occurrence is often unknown. Although traditional research to answer objectives at larger scales and longer time frames is often prohibitively costly, community-science projects are well suited for these kind of problems (Tulloch et al. 2013a). Nevertheless, community science projects still need time, expertise and financial investment, including resources to design, create and maintain a platform and human resources to facilitate effective communication between professional scientists and volunteers to sustain engagement (Aceves-Bueno et al. 2015). Therefore, before investing in a community-science initiative, it should be evaluated whether the extra investment would improve the quality of already existing data by minimising redundancy with already existing projects and filling data gaps.
Herein, we compare datasets from five large community-science platforms of species-occurrence (presence-only) data. We focus on endemic birds of the Atlantic Forest (218 species), given the importance of this taxon in South America and the provenance of birds in community-collected data compared with other groups (Alexandrino et al. 2022; Forti and Szabo 2023). Although these five platforms somewhat differ in target species, geographic coverage, geographic accuracy and type of data collected (Table 1), they all supply information collected by volunteer observers on avian species presence in the Atlantic Forest in Brazil in a somewhat overlapping time period. We compared the number of species recorded, the number of observers and observations, and the number of duplicate observations among the five platforms. To identify spatial gaps, we plotted the number of observations per municipality and the exact location of the observations for the four platforms that record accurate geographic coordinates. Data in the fifth platform (WikiAves) are areal, i.e. defined only at a fixed number of spatial regions (in this case, municipalities). We applied network analysis to visualise and describe the ways observers interact with species overall and in each platform. This method has been used to understand complex systems in sociology, epidemiology and other fields (Posfai and Barabasi 2016). In ecology, network analysis has been used to describe and understand species interactions (Delmas et al. 2019), and to guide environmental management and conservation of ecosystem functions (Tylianakis et al. 2010; Allen et al. 2022). We also used this approach to evaluate the observers’ contribution. We predicted that network patterns would differ between specialised and generalist platforms. In particular, we expected the networks of specialised platforms to be more connected and generalist platforms to have higher nestedness. We also expected observers who contributed to specialist platforms to submit a more balanced ratio of rare and common species, and presumed that in non-specialist platforms, contribution would be dependent more on species detectability. Finally, we illustrate the value of this approach to evaluate the relative contribution of different platforms and community scientists.
Platform | Species coverage | Geographic coverage | Active since | Location accuracy | Types of observations | Link | |
---|---|---|---|---|---|---|---|
eBird | Birds | Global | 2002 | Geographic coordinates | Species lists, photos, videos, and audio recordings | https://ebird.org/ | |
WikiAves | Birds | Brazil | 2008 | Municipality | Photos | https://www.wikiaves.com.br/ | |
iNaturalist | General | Global | 2008 | Geographic coordinates | Photos and audio | https://www.inaturalist.org/ | |
Biofaces | General | Brazil | 2012 | Geographic coordinates | Photos, audio and videos | https://www.biofaces.com/ | |
TáxeusA | General | Brazil | 2011–2021 | Geographic coordinates | Species lists | https://www.Táxeus.com.br/ |
Methods
We used the list of Atlantic Forest endemic birds by Vale et al. (2018) to select the target species, and used taxonomy from Data Zone on BirdLife International (at http://datazone.birdlife.org/) to compare platforms. We compiled data from five community-science platforms, including all data from the first observation of each platform (note that some platforms accept historical data) until November 2021 in the case of Táxeus, March 2023 for eBird, iNaturalist and WikiAves, and April 2023 for Biofaces.
eBird is a global platform specialised in bird observations. Managed by the Cornell Laboratory of Ornithology, eBird has been active since 2002 and is currently considered the largest biodiversity-related community-science project in the world. Its observer community of hundreds of thousands of users has been contributing over 100 million bird observations (species lists, photos, videos and audio recordings) annually, making it the main contributor to GBIF (see https://ebird.org/home). Cumulatively, volunteers in this platform have contributed more than 1.3 billion bird observations. eBird employs automated quality-control algorithms to flag potential errors or outliers in submitted checklists, which are reviewed by experts.
Launched in 2008, WikiAves is a Brazilian platform specialised in birds, with content being moderated by volunteers who approve observations before publications (see https://www.wikiaves.com.br/index.php). At the time of the analysis, WikiAves had a community of 45,441 observers who have submitted over 4.3 million photos and 262,729 sound recordings of 1960 species. WikiAves observations lack geographical coordinates and are reported at the scale of municipality. The data are not entered into GBIF.
iNaturalist is a generalist platform, accepting photos and audio records of all kind of organisms. The platform was initiated in 2008 and until 2023 was managed by the California Academy of Sciences and National Geographic, when it became an independent non-profit organisation with a global network of over 2.5 million observers. Observations are entered into GBIF after validation to ‘research grade’ level, i.e. when they reach a 2/3 consensus among the identifiers on the suggested taxon. At the time of writing this article, iNaturalist contained 275,325 research-grade observations of 1685 bird species in Brazil (see https://www.inaturalist.org/). For our study, we considered only research-grade observations. This distinction was available only for this particular platform.
Biofaces is a national-scale platform to share photos, sound recordings and videos of animals. The initiative has been active since 2012 with 6716 users and data on 19,006 species (4420 birds). Observers share occurrence lists from particular sites on wild species (see https://www.biofaces.com/). This platform is linked to SiBBr (see https://sibbr.gov.br/), a GBIF node in Brazil. We did not find any information about the data-curation processes of this platform.
Táxeus is a Brazilian community-science platform used to share biodiversity data (species lists) since 2011. When the platform started, it accepted only bird observations, but in the following year, it was extended to mammals, and in 2013 to amphibians. Similar to Biofaces, Táxeus is registered with SiBBr. The platform reached 3542 observers with 13,427 species lists including birds, mammals and amphibians; however, it was recently (November 2021) discontinued (see https://www.Táxeus.com.br/). Similar to WikiAves, data in this platform are areal, with no exact coordinates available for particular observations, and data are aggregated spatially as well as by observer. We did not find any information about the data-curation processes of this platform.
We formally requested raw data from eBird and iNaturalist through their webpage API. As this option was not available for the other three platforms, we used Instant Data Scraping (see https://webrobots.io/instantdata/). The full dataset (raw data, including duplicate observations) contained 1,263,330 observations, with 57.1% of the observations from eBird, 39.6% from WikiAves, 1.4% from iNaturalist, 1.1% from Táxeus and 0.9% from Biofaces.
For each platform and for the full dataset, we calculated the proportion of duplicate observations considering the species, the date and the location of the observation, by using the distinct function in the dplyr package (ver. 1.0.9, H. Wickham, R. François, L. Henry, K. Müller and D. Vaughan, see https://CRAN.R-project.org/package=dplyr) in R (ver. 4.3.1, see http://www.R-project.org/). We listed user names for each platform to calculate the overlap in the use of platforms among volunteers and a researcher not involved with the analyses matched user names with proper names where publicly available. To analyse observer–bird species networks, we constructed incidence matrices on the basis of the community of observers and bird species for four community-science platforms, because Táxeus did not include enough information to perform this analysis. We calculated and visualised the metrics for weighted and non-directed networks by using the R packages bipartite (ver. 2.20, see https://cran.r-project.org/package=bipartite; Dormann et al. 2008) and igraph (ver. 1.5.0, see https://CRAN.R-project.org/package=igraph; Csárdi and Nepusz 2006). We calculated four indices to describe different structural patterns in the networks. (1) Connectance, which is a complexity value that describes how connected the network nodes are in general. Connectance is based on the proportion of realised-to-possible interactions (Lau et al. 2017). In our case, higher connectance values indicate that observers are more or less equally connected to bird species (i.e. people report similar sets of species), and low values mean that most species are connected to a small number of different observers. (2) Nestedness based on overlap (NODF) describes the degree to which nodes interact with particular subsets of the interaction partners of better-connected nodes (Mariani et al. 2019). In our case, NODF reflects the tendency of species with fewer connections to be reported by a subset of observers with more species recorded. Networks with high nestedness are formed by a heterogeneous pattern of interactions, with some observers and species having few interactions with more connected species or observers in the system. This means that in a nested network ‘super-observers’ record species that are in general more rarely observed. (3) The clustering coefficient is a measure of the degree to which nodes tend to cluster together (Zhang et al. 2008), quantifying the abundance of connected subgroups (triangles) of nodes (in our case, species and observers) in a network. We interpret this metric as a measure of local cohesiveness among species and observers. Finally, (4) the mean degree is the mean of all the node degrees in a network (Barrat et al. 2004), in our case the average number of interactions among species and observers, i.e. the number of individual birds (regardless of species) seen by an observer. The mean degree can be sensitive to the number of observers, once the probability of multiple interactions with a species is higher. Therefore, this number can be considered a measure of complexity.
We obtained a GIS layer for the extent of the Atlantic Forest from Brazilian Institute of Geography and Statistics (Instituto Brasileiro de Geografia e Estatística 2008), with limits defined by the Atlantic Forest Law (Brazilian Federal Constitution, Law 11.428/2006), and using the cleaned dataset for the four datasets with spatial information (Biofaces, eBird, iNaturalist, and WikiAves) in QGIS (ver. 3.30.2, see http://qgis.osgeo.org), we created maps displaying the locations, where available (Biofaces, eBird, and iNaturalist) and density of the observations per municipality (for the four datasets).
Results
Each platform had more than 80% species coverage for endemic Atlantic Forest bird species, with the joint dataset reaching 100%. Even though Biofaces had the fewest observers and a bit over of a decade of activity, this platform had the higher species coverage (96%), surpassing even platforms specialised in birds. iNaturalist had the lowest proportion (3.8%) of within-platform duplicates, followed by Biofaces (8.4%). About a third (29.7%) of the observations were duplicated within datasets, particularly in WikiAves (142,266) and eBird (227,463) (Table 2). The number of duplicates among datasets was only 14 (less than 0.002%). eBird represented 55.6% of the joint dataset, submitted by a relatively low number of observers, particularly compared with WikiAves.
Characteristic | Platform | ||||||
---|---|---|---|---|---|---|---|
Biofaces | eBird | iNaturalist | Táxeus | Wikiaves | All | ||
Year of first observation | 2000 | 1885 | 1979 | 1995 | 1900 | 1885 | |
Year of last observation | 2023 | 2023 | 2023 | 2023 | 2023 | 2023 | |
Total number of observations | 10,808 | 721,452 | 17,091 | 13,651 | 500,328 | 1,263,330 | |
Number (%) of within-platform duplicates | 910 (8.4) | 227,463 (31.5) | 652 (3.8) | 4013 (29.4) | 142,266 (28.4) | 375,318 (29.7) | |
Number of observers | 524 | 4872 | 1860 | NA | 10,143 | 17,202 | |
Species richness | 210 | 194 | 191 | 184 | 207 | 218 | |
%Species coverage | 96 | 89 | 88 | 84 | 95 | 100 |
The contribution of observers considering all platforms together varied from 1 to 8124 observations, and 20% of the most productive observers covered 86% of the total observations in the joint dataset. Over a fifth of observers (3907 people; 23%) contributed a single observation. On the basis of Biofaces, WikiAves and iNaturalist data, at least 296 observers contributed to more than one platform, whereas 97.6% were using only one of these platforms. The largest overlap was between Biofaces and WikiAves (228 common users), followed by WikiAves and iNaturalist (64 observers), Biofaces and iNaturalist (20 observers) and 18 observers used all three platforms.
The central corridor of the Atlantic Forest (Espírito Santo State and southern Bahia State) and most interior areas of the biome were poorly represented in the four platforms with location data available (Fig. 1). Observations were more widely distributed in WikiAves and eBird; however, in general, the platforms had similar distribution patterns of observations, with higher concentrations in the south and on the coast.
The number of observations of endemic Atlantic Forest bird species per municipality in (a) Wikiaves (inset: the location of the study area in relation to Brazil), (b) eBird, (c) Biofaces and (d) iNaturalist, on the basis of data from 1979 to 2023. Red indicates municipalities with no observations. The actual locations of observations are shown for (e) iNaturalist, (f) eBird and (g) Biofaces, whereas (h) WikiAves records data only at municipality level.
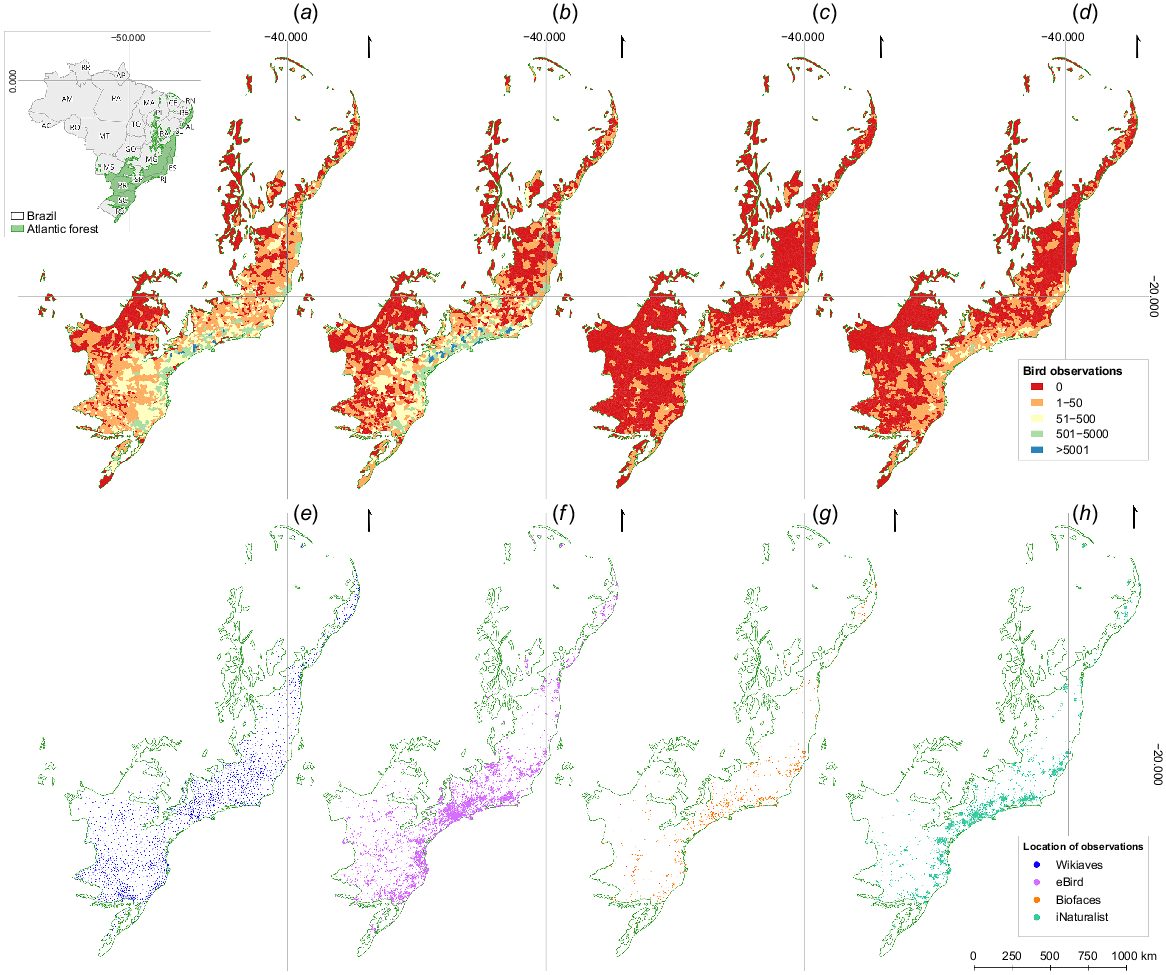
The coverage at the level of municipalities was also highly uneven (Fig. 2). In fact, of the 3416 municipalities with Atlantic Forest vegetation, 38.35% had no observations in any of the four datasets with location data, 21.40% had observations in one, 18.55% in two, 11.97% in three and 9.72% in all four. Three-quarters (75.10%) of the 731 municipalities with observations in one dataset were covered by WikiAves, followed by eBird (19.01%).
Endemic Atlantic Forest bird species observations present in each municipality in one or more of the four platforms with location data (Inset: the location of the study area in relation to Brazil): iNaturalist (I), WikiAves (W), eBird (E) and Biofaces (B) from 1979 to 2023. In red are the municipalities with no observations and in purple municipalities with data in all platforms.
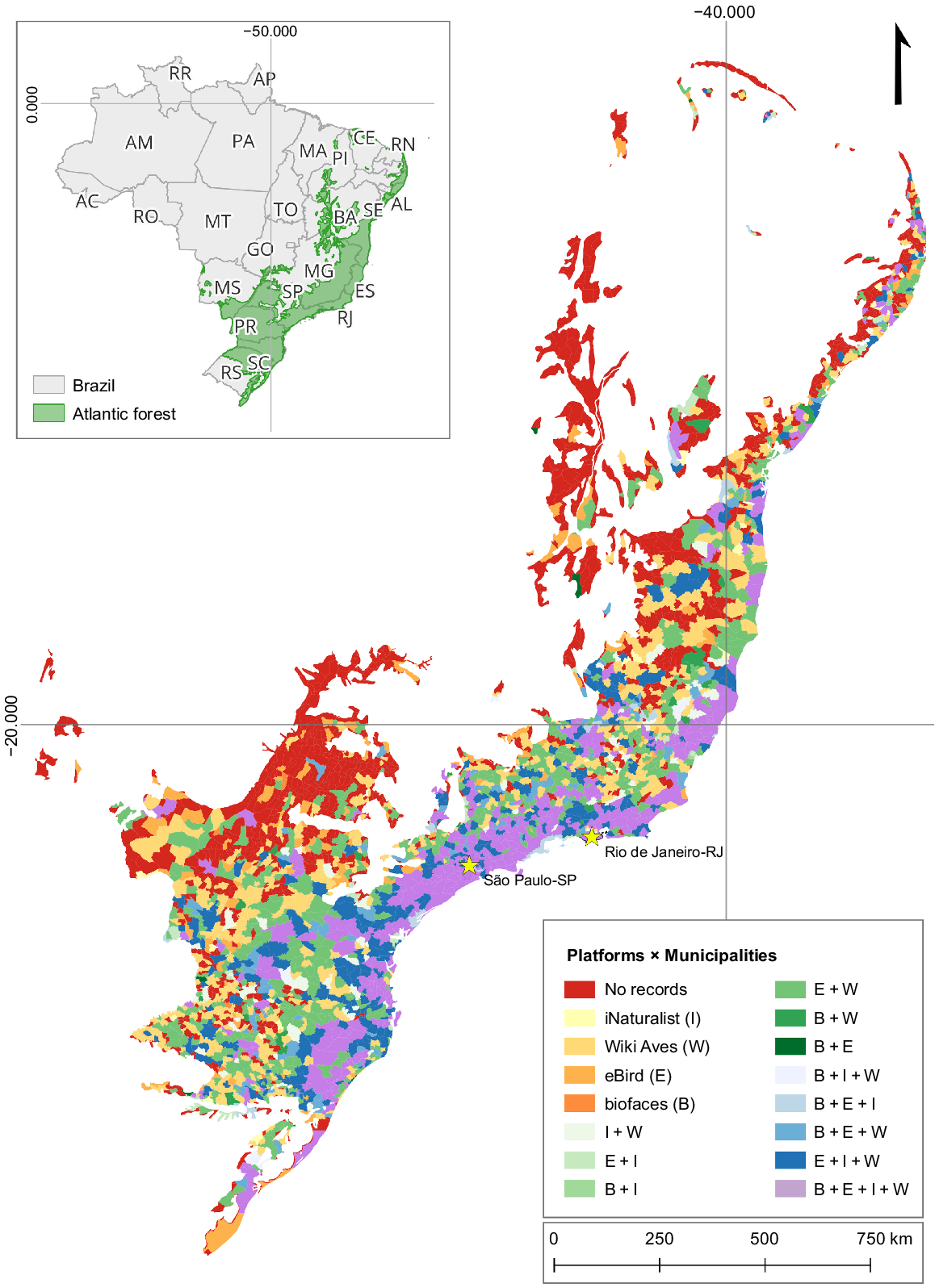
In the observer–species network of the platforms, most bird species occupied central positions, surrounded by observers in the periphery. Most species were observed by many observers, and the mean (degree) number of interactions between species and observers varied between 9.5 and 45.8 for these networks, meaning that people saw, on average, this number of individuals of different bird species. Biofaces had the most unique network, with many non-centralised species linked to fewer observers. This platform had three disconnected interactions, and the fewest (524) observers among the four (Fig. 3).
Observer–bird species interaction networks based on communities of four large community-science platforms. Blue dots are observers and yellow dots are endemic Atlantic Forest bird species.
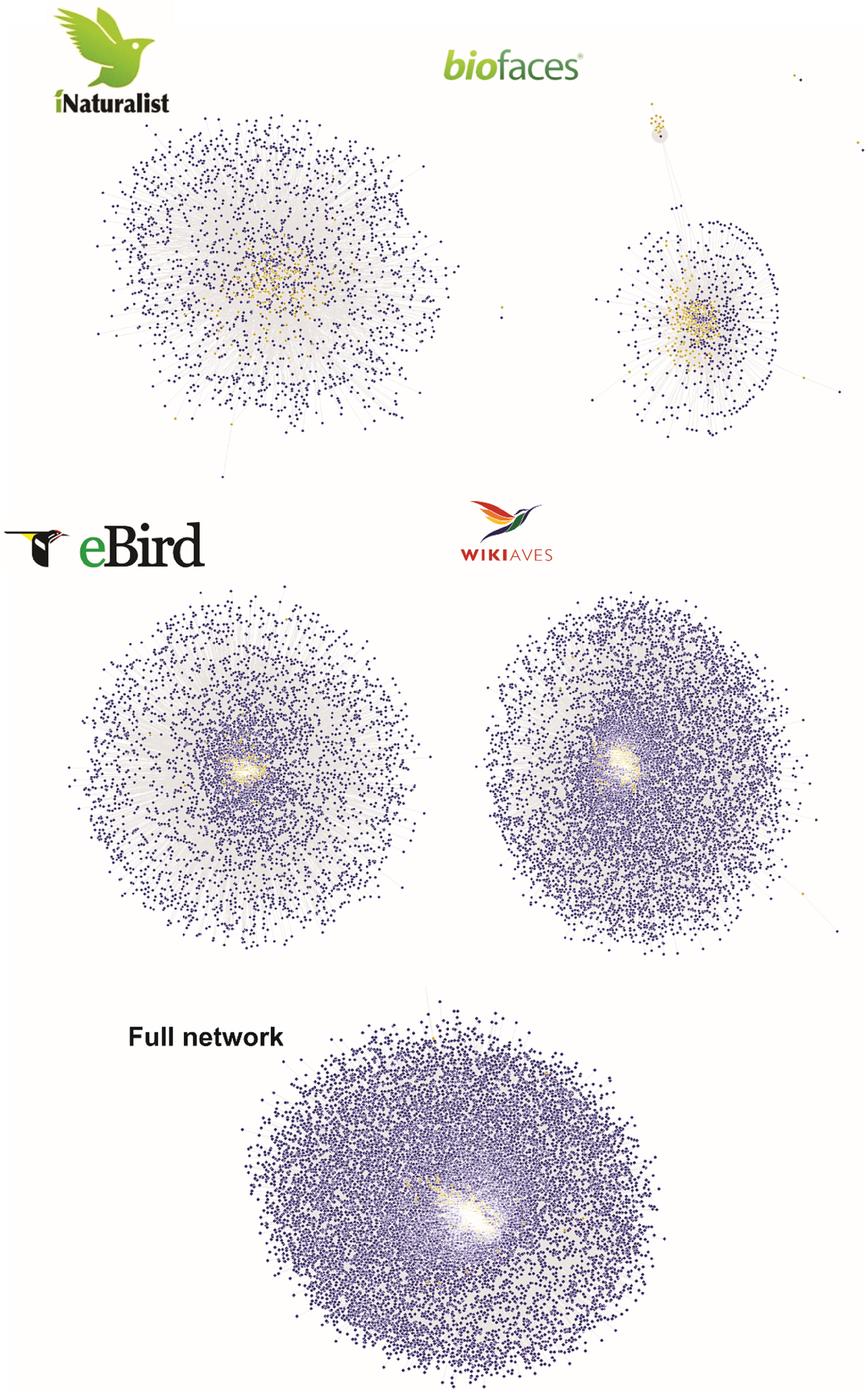
eBird had the most connected network with the highest nestedness, whereas iNaturalist had the lowest values for both connectance and nestedness (Table 3). Cluster coefficients were low for all platforms. The joint dataset network had low connectance and a high nestedness.
Network metrics | Platform | |||||
---|---|---|---|---|---|---|
Biofaces | eBird | iNaturalist | Wikiaves | All | ||
Mean (±s.d.) degree | 19 (±29.2) | 45.8 (±153.5) | 9.5 (±28.9) | 35.7 (±181.9) | 36.2 (±227.2) | |
Network connectance | 0.063522 | 0.122775 | 0.027448 | 0.088162 | 0.079388 | |
NODF | 2.929.705 | 4.338.556 | 1.808.536 | 4.066.559 | 3.631.572 | |
Cluster coefficient | 0.045802 | 0.09493 | 0.013441 | 0.057182 | 0.054645 |
Please note that these calculations were not possible for Táxeus, because this platform does not include information about the observer. NODF, nestedness based on overlap.
Discussion
In spite of the large variability in the number of observations and observers among the platforms, Atlantic Forest bird species were well represented in all five. Nevertheless, full species coverage was achieved only after merging all datasets, meaning that each platform was important not only by increasing the number of observations of already present species, but also added unique species. Observers in all platforms contributed with rarely observed species, evidenced by the high mean degree of the networks.
The low spatial completeness among the different datasets is concerning. However, if the community of observers continues to submit observations from new locations, pooling these datasets can help support bird-species monitoring in the Atlantic Forest. A variety of platforms (Schaaf et al. 2024) and a variety of observers (Tulloch and Szabo 2012) are known to improve data quality. The low overlap between the two bird-specific platforms, eBird and WikiAves suggest that the two initiatives cater for a different audience. WikiAves users are traditionally Brazilian and the interface (including species information and problem-solving) is in Portuguese, even though, recently, eBird interface on the mobile app became available in Portuguese and Brazilian Portuguese names are displayed for South American species that have it, eBird is traditionally used by visitors to the country (J. K. Szabo, unpubl. data). Possible explanations could be that WikiAves has been active in Brazil for a longer period and has built a strong and engaged community among birdwatchers and bird guides, it has established partnerships with local organisations, universities and conservation groups in Brazil, which help promote the platform and encourage participation among bird enthusiasts across the country and uses a taxonomy Brazilians are presumably more familiar with (see https://www.wikiaves.com.br/wiki/aves), as opposed to the Clements Checklist used by eBird (see https://science.ebird.org/en/use-ebird-data/the-ebird-taxonomy).
A detailed and focused study on the demographics of the five platforms collecting data on endemic Atlantic Forest birds could confirm whether any potential collaborators are missed by these platforms and to identify why apps attract different users. Nevertheless, to model species distribution, data integration is preferable to improve the precision of the estimated environmental relationships as well as the predictive performance of models (Fletcher et al. 2019). Integrated distribution models (IDMs) were developed to include multiple datasets in a single model, while accounting for different data collection protocols (Miller et al. 2019; Isaac et al. 2020). Increasing the sample size of presence–absence data and having no correlated covariates (to explain bias) can also improve estimation. Nevertheless, in the case of presence-only data, integration alone cannot correct for spatial bias (Simmonds et al. 2020). When modelling species distributions using combined data sources that have vastly different sampling methodologies and amounts of data, weighted joint likelihoods can offer a solution (Fletcher et al. 2019). Unfortunately, original-source metadata are not routinely considered when processing GBIF data and modelling based on GBIF data does not explicitly acknowledge data sources in the modelling process.
Spatially biased citizen-science data can be used to estimate species occupancy in regions with stationary environmental relationships and good sampling across environmental space (Johnston et al. 2020). Unfortunately, in our datasets, spatial sampling was inadequate in inland municipalities of the Atlantic Forest biome, with many having no records in any of the datasets with spatial information. As some of these regions (southern Piauí, south-western Paraná, south-eastern Minas Gerais and central Rio Grande do Sul states) also contain larger fragments of primary forest (Vancine et al. 2024), they should be prioritised for standardised (paid) surveys (Tulloch et al. 2013a). Bayesian priors obtained from a robust (but often smaller) dataset can then be included as an offset in log-linear species distribution models (Miller et al. 2019). Thereby linking standardised observations to a presence-only dataset through point processes makes it possible to estimate local density or occurrence probability directly rather than just obtaining an estimate of relative occurrence probability (Miller et al. 2019; Isaac et al. 2020). In the case of areal data (e.g. WikiAves), conditionally autoregressive models (CAR) are applied that use the adjacency matrix of the regions (Miller et al. 2019). Although geostatistical models are arguably more flexible and realistic, in CAR, dependence is defined locally, which can be exploited using sparse matrix operations, resulting in computational simplicity (Miller et al. 2019).
Similarly, simple methods provide biased trend estimates from noisy citizen-science datasets (Isaac et al. 2014). Uneven sampling effort (both spatially and temporally), as well as detectability, lead to low power or failure of statistical models. In our case, the datasets available for Atlantic Forest birds are not only biased, but their sample size is too low for temporal analyses in most municipalities. Therefore, increasing observer engagement is crucial, because a high number of people submitted only a single observation. Our datasets confirmed to the Pareto rule, i.e. 20% of the most active observers contributed 80% of the observations, as also reported from other community-science projects (Wood et al. 2011; Cunha et al. 2017; Parrish et al. 2019; Allf et al. 2022). The number of observations submitted to multiple platforms was low, possibly resulting from different user base for each platform, as over 97% used only one platform and few (0.14%) observers participated in multiple initiatives. Nevertheless, some observers use different platforms for different reasons (e.g. submitting bird observations to a bird-specific platform and other organisms to a more generalist platform). On the basis of network analysis, observers use these platforms in different ways. The iNaturalist observer community, for example, shows low connectance and low nestedness compared with other platforms, and most observers seem to be somewhat specialised in the bird species they observe, similar to the results found in Western Australia for Atlas observers (Tulloch and Szabo 2012). In contrast, eBird and WikiAves, both of which specialise in birds, had highly nested interactions, with many highly generalist observers. Biofaces seems to have a variety of observers with different profiles, with many observers recording common species, and others also recording rare species. This difference can result from the habitats the observers visit, their preference for rare or threatened species or their identification skills (Szabo et al. 2012). By identifying patterns in observer behaviour, the network approach can inform us about the ways observers interact with biodiversity at different scales, and in different geographical regions.
Integrating data from different sources, such as academic research and participatory monitoring, is an effective way to monitor biodiversity, describe ecological patterns and guide conservation decisions (Tulloch et al. 2013b; Binley and Bennett 2023). Recently, the contribution of community-science data has been increasing, and currently most data on wildlife occurrence in global protected areas come from the citizen scientists (Mandeville et al. 2023). If different platforms engage more people that have different surveying skills and preferences, new initiatives can focus on poorly represented areas or observational details missing from other initiatives or atlases (Schaaf et al. 2024). Community science can further enhance bird monitoring in the Atlantic Forest (Forti et al. 2024) and its contribution could be elevated by aggregating different datasets (Hertzog et al. 2021), including combining expert- and volunteer-collected data (Robinson et al. 2020; Farias et al. 2022). For instance, WikiAves, one of the major platforms to produce data on birds in Brazil, is not linked to GBIF, because observations are not georeferenced at a fine resolution. However, this platform had the widest distribution of observations collected by a large community of users, and, although spatially imprecise, the dataset by this platform has an inestimable value for studying bird species behaviour (Tubelis and Sazima 2020, 2021; Alexandrino et al. 2022; Schneider et al. 2023), migration (Schubert et al. 2019; Degroote et al. 2021) and tracking spatio-temporal patterns of occurrence (Zulian et al. 2021; Cunha et al. 2022; Atwood 2023).
In summary, although regional and national-scale projects, especially Biofaces and WikiAves, cannot yet offer a large-enough or long-enough dataset to support wildlife monitoring by themselves, they still play a vital role aggregated to other (global) initiatives. Combining data can paint a better picture on species occurrence in threatened biomes, such as the Atlantic Forest.
Declaration of funding
This research received no specific grant from any funding agency, commercial or not-for-profit sectors. Kauane Freitas (number 151994/2021-8), Arthur Queiros (number 127626/2022-0) and Talita Oliveira (number 135165/2022-9) received funding from Programa Institucional de Bolsas de Iniciação Científica (PIBIC), fornecida pelo Conselho Nacional de Desenvolvimento Científico e Tecnológico (CNPq) and Juan Victor de Lima Maia (number 88887.687023/2022-00) from Coordenação de Aperfeiçoamento de Pessoal de Nível Superior (CAPES).
Acknowledgements
We thank all volunteers who contributed observations of birds in community-science platforms that help wildlife research of the Atlantic Forest. We also thank three anonymous reviewers and the handling editor for reviewing and improving our work.
References
Aceves-Bueno E, Adeleye AS, Bradley D, Tyler Brandt W, Callery P, Feraud M, Garner KL, Gentry R, Huang Y, McCullough I, Pearlman I, Sutherland SA, Wilkinson W, Yang Y, Zink T, Anderson SE, Tague C (2015) Citizen science as an approach for overcoming insufficient monitoring and inadequate stakeholder buy-in in adaptive management: criteria and evidence. Ecosystems 18, 493-506.
| Crossref | Google Scholar |
Alexandrino ER, da Silva GA, Corbo MC, Demuner BA, Szabo JK (2022) Urban southern house wren (Troglodytes aedon musculus) nesting in apparently unsuitable human-made structures: is it worth it? Ornitología Neotropical 33(1), 44-52.
| Crossref | Google Scholar |
Allen WJ, Bufford JL, Barnes AD, Barratt BIP, Deslippe JR, Dickie IA, Goldson SL, Howlett BG, Hulme PE, Lavorel S, O’Brien SA, Waller LP, Tylianakis JM (2022) A network perspective for sustainable agroecosystems. Trends in Plant Science 27(8), 769-780.
| Crossref | Google Scholar | PubMed |
Allf BC, Cooper CB, Larson LR, Dunn RR, Futch SE, Sharova M, Cavalier D (2022) Citizen science as an ecosystem of engagement: implications for learning and broadening participation. BioScience 72(7), 651-663.
| Crossref | Google Scholar | PubMed |
Atwood JL (2023) Seasonal patterns of least tern distribution along the Atlantic Coasts of North, Central, and South America. Waterbirds 46(1), 85-90.
| Crossref | Google Scholar |
Barrat A, Barthélemy M, Pastor-Satorras R, Vespignani A (2004) The architecture of complex weighted networks. Proceedings of the National Academy of Sciences 101(11), 3747-3752.
| Crossref | Google Scholar | PubMed |
Binley AD, Bennett JR (2023) The data double standard. Methods in Ecology and Evolution 14, 1389-1397.
| Crossref | Google Scholar |
Bonney R (2021) Expanding the impact of citizen science. BioScience 71(5), 448-451.
| Crossref | Google Scholar |
Callaghan CT, Ozeroff I, Hitchcock C, Chandler M (2020) Capitalizing on opportunistic citizen science data to monitor urban biodiversity: a multi-taxa framework. Biological Conservation 251, 108753.
| Crossref | Google Scholar |
Callaghan CT, Poore AGB, Mesaglio T, Moles AT, Nakagawa S, Roberts C, Rowley JJL, Vergés A, Wilshire JH, Cornwell WK (2021) Three frontiers for the future of biodiversity research using citizen science data. BioScience 71(1), 55-63.
| Crossref | Google Scholar |
Csárdi G, Nepusz T (2006) The igraph software package for complex network research. InterJournal, Complex Systems 1695(5), 1-9.
| Google Scholar |
Cunha DGF, Marques JF, Resende JCD, Falco PBD, Souza CMD, Loiselle SA (2017) Citizen science participation in research in the environmental sciences: key factors related to projects’ success and longevity. Anais da Academia Brasileira de Ciências 89, 2229-2245.
| Crossref | Google Scholar | PubMed |
Cunha FCR, Lopes LE, Selezneva A (2022) Revealing migration schedule and potential breeding grounds of Lined Seedeaters using citizen science data. Emu – Austral Ornithology 122(3-4), 167-175.
| Crossref | Google Scholar |
Degroote LW, Hingst-Zaher E, Moreira-Lima L, Whitacre JV, Slyder JB, Wenzel JW (2021) Citizen science data reveals the cryptic migration of the Common Potoo Nyctibius griseus in Brazil. Ibis 163, 380-389.
| Crossref | Google Scholar |
Delmas E, Besson M, Brice M-H, Burkle LA, Dalla Riva GV, Fortin M-J, Gravel D, Guimarães PR, Jr, Hembry DH, Newman EA, Olesen JM, Pires MM, Yeakel JD, Poisot T (2019) Analysing ecological networks of species interactions. Biological Reviews 94(1), 16-36.
| Crossref | Google Scholar | PubMed |
Dennis EB, Morgan BJT, Brereton TM, Roy DB, Fox R (2017) Using citizen science butterfly counts to predict species population trends. Conservation Biology 31(6), 1350-1361.
| Crossref | Google Scholar | PubMed |
Dormann CF, Gruber B, Fründ J (2008) Introducing the bipartite package: analysing ecological networks. R News 8(2), 8-11.
| Google Scholar |
Ellwood ER, Sessa JA, Abraham JK, Budden AE, Douglas N, Guralnick R, Krimmel E, Langen T, Linton D, Phillips M, Soltis PS, Studer M, White LD, Williams J, Monfils AK (2020) Biodiversity science and the twenty-first century workforce. BioScience 70(2), 119-121.
| Crossref | Google Scholar | PubMed |
Farias M, Roper J, Cavarzere V (2022) Bird communities and their conservation priorities are better understood through the integration of traditional and citizen science data: an example from Brazilian Atlantic Forest. Citizen Science: Theory and Practice 7(1), 9.
| Crossref | Google Scholar |
Fletcher RJ, Jr, Hefley TJ, Robertson EP, Zuckerberg B, McCleery RA, Dorazio RM (2019) A practical guide for combining data to model species distributions. Ecology 100(6), e02710.
| Crossref | Google Scholar | PubMed |
Forti L (2023) Dataset of five citizen science platforms with reagards endemic bird species of the Atlantic Forest. Zenodo 2023. [Dataset, posted 20 November 2023] doi:10.5281/zenodo.10177895
Forti LR, Szabo JK (2023) The iNaturalist platform as a source of data to study amphibians in Brazil. Anais da Academia Brasileira de Ciências 95(1), e20220828.
| Crossref | Google Scholar |
Forti LR, Hepp F, de Souza JM, Protazio A, Szabo JK (2022a) Climate drives anuran breeding phenology in a continental perspective as revealed by citizen-collected data. Diversity and Distributions 28, 2094-2109.
| Crossref | Google Scholar |
Forti LR, Pontes MR, Augusto-Alves G, Martins A, Hepp F, Szabo JK (2022b) Data collected by citizen scientists reveal the role of climate and phylogeny on the frequency of shelter types used by frogs across the Americas. Zoology 155, 126052.
| Crossref | Google Scholar | PubMed |
Forti LR, Passetti A, Oliveira T, Lima J, Queiros A, Dantas Ferreira Lopes MA, Szabo JK (2024) Declining representation of imperiled Atlantic Forest birds in community-science datasets. Perspectives in Ecology and Conservation. [In press, corrected proof published 2 July 2024] doi:10.1016/j.pecon.2024.02.001.
Fraisl D, Hager G, Bedessem B, Gold M, Hsing P-Y, Danielsen F, Hitchcock CB, Hulbert JM, Piera J, Spiers H, Thiel M, Haklay M (2022) Citizen science in environmental and ecological sciences. Nature Reviews Methods Primers 2(1), 64.
| Crossref | Google Scholar |
Hertzog LR, Frank C, Klimek S, Röder N, Böhner HGS, Kamp J (2021) Model-based integration of citizen science data from disparate sources increases the precision of bird population trends. Diversity and Distributions 27, 1106-1119.
| Crossref | Google Scholar |
Howard E, Davis AK (2009) The fall migration flyways of monarch butterflies in eastern North America revealed by citizen scientists. Journal of Insect Conservation 13(3), 279-286.
| Crossref | Google Scholar |
Hurlbert AH, Liang Z (2012) Spatiotemporal variation in avian migration phenology: citizen science reveals effects of climate change. PLoS ONE 7(2), e31662.
| Crossref | Google Scholar | PubMed |
Instituto Brasileiro de Geografia e Estatística (2008) Mapa da área de aplicação da Lei nº 11.428 de 2006. (IBGE) Available at https://antigo.mma.gov.br/biomas/mata-atlântica_emdesenvolvimento/mapas-da-mata-atlântica.html [In Portuguese]
Isaac NJB, van Strien AJ, August TA, de Zeeuw MP, Roy DB (2014) Statistics for citizen science: extracting signals of change from noisy ecological data. Methods in Ecology and Evolution 5, 1052-1060.
| Crossref | Google Scholar |
Isaac NJB, Jarzyna MA, Keil P, Dambly LI, Boersch-Supan PH, Browning E, Freeman SN, Golding N, Guillera-Arroita G, Henrys PA, Jarvis S, Lahoz-Monfort J, Pagel J, Pescott OL, Schmucki R, Simmonds EG, O’Hara RB (2020) Data integration for large-scale models of species distributions. Trends in Ecology & Evolution 35, 56-67.
| Crossref | Google Scholar | PubMed |
Jarić I, Correia RA, Brook BW, Buettel JC, Courchamp F, Di Minin E, Firth JA, Gaston KJ, Jepson P, Kalinkat G, Ladle R, Soriano-Redondo A, Souza AT, Roll U (2020) iEcology: harnessing large online resources to generate ecological insights. Trends in Ecology & Evolution 35(7), 630-639.
| Crossref | Google Scholar | PubMed |
Johnston A, Moran N, Musgrove A, Fink D, Baillie SR (2020) Estimating species distributions from spatially biased citizen science data. Ecological Modelling 422, 108927.
| Crossref | Google Scholar |
Lau MK, Borrett SR, Baiser B, Gotelli NJ, Ellison AM (2017) Ecological network metrics: opportunities for synthesis. Ecosphere 8(8), e01900.
| Crossref | Google Scholar |
Mandeville CP, Nilsen EB, Herfindal I, Finstad AG (2023) Participatory monitoring drives biodiversity knowledge in global protected areas. Communications Earth & Environment 4, 240.
| Crossref | Google Scholar |
Mariani MS, Ren Z-M, Bascompte J, Tessone CJ (2019) Nestedness in complex networks: observation, emergence, and implications. Physics Reports 813, 1-90.
| Crossref | Google Scholar |
Mesaglio T, Callaghan CT (2021) An overview of the history, current contributions and future outlook of iNaturalist in Australia. Wildlife Research 48, 289-303.
| Crossref | Google Scholar |
Miller DAW, Pacifici K, Sanderlin JS, Reich BJ (2019) The recent past and promising future for data integration methods to estimate species’ distributions. Methods in Ecology and Evolution 10, 22-37.
| Crossref | Google Scholar |
Newman G, Chandler M, Clyde M, McGreavy B, Haklay M, Ballard H, Gray S, Scarpino R, Hauptfeld R, Mellor D, Gallo J (2017) Leveraging the power of place in citizen science for effective conservation decision making. Biological Conservation 208, 55-64.
| Crossref | Google Scholar |
Parrish JK, Jones T, Burgess HK, He Y, Fortson L, Cavalier D (2019) Hoping for optimality or designing for inclusion: persistence, learning, and the social network of citizen science. Proceedings of the National Academy of Sciences 116(6), 1894-1901.
| Crossref | Google Scholar | PubMed |
Robinson OJ, Ruiz-Gutierrez V, Reynolds MD, Golet GH, Strimas-Mackey M, Fink D (2020) Integrating citizen science data with expert surveys increases accuracy and spatial extent of species distribution models. Diversity and Distributions 26, 976-986.
| Crossref | Google Scholar |
Rowley JJL, Callaghan CT, Cutajar T, Portway C, Potter K, Mahony S, Trembath DF, Flemons P, Woods A (2019) FrogID: citizen scientists provide validated biodiversity data on frogs of Australia. Herpetological Conservation and Biology 14(1), 155-170.
| Google Scholar |
Sanderson C, Braby MF, Bond S (2021) Butterflies Australia: a national citizen science database for monitoring changes in the distribution and abundance of Australian butterflies. Austral Entomology 60, 111-127.
| Crossref | Google Scholar |
Schaaf AA, Haag LM, Gonzalez Baffa-Trasci NV, Yapura A, Chocobar N, Caldano SA, Ruggera RA (2024) Comparing different citizen science platforms for collecting urban ecological data from Toco toucan (Ramphastos toco) in Argentina. Austral Ecology 49(2), e13459.
| Crossref | Google Scholar |
Schneider LM, de Oliveira Santos C, Moreira-Lima L, Hingst-Zaher E (2023) Peregrine falcon Falco peregrinus in Brazil: natural history through the lens of citizen science. Ornitología Neotropical 34, 29-39.
| Crossref | Google Scholar |
Schubert SC, Manica LT, Guaraldo ADC (2019) Revealing the potential of a huge citizen-science platform to study bird migration. Emu – Austral Ornithology 119(4), 364-373.
| Crossref | Google Scholar |
Simmonds EG, Jarvis SG, Henrys PA, Isaac NJB, O’Hara RB (2020) Is more data always better? A simulation study of benefits and limitations of integrated distribution models. Ecography 43, 1413-1422.
| Crossref | Google Scholar |
Szabo JK, Vesk PA, Baxter PWJ, Possingham HP (2010) Regional avian species declines estimated from volunteer-collected long-term data using list length analysis. Ecological Applications 20(8), 2157-2169.
| Crossref | Google Scholar | PubMed |
Szabo JK, Fuller RA, Possingham HP (2012) A comparison of estimates of relative abundance from a weakly structured mass-participation bird atlas survey and a robustly designed monitoring scheme. Ibis 154, 468-479.
| Crossref | Google Scholar |
Troudet J, Grandcolas P, Blin A, Vignes-Lebbe R, Legendre F (2017) Taxonomic bias in biodiversity data and societal preferences. Scientific Reports 7, 9132.
| Crossref | Google Scholar | PubMed |
Tubelis DP, Sazima I (2020) Biologia reprodutiva do carão, Aramus guarauna (Gruiformes: Aramidae), no Pantanal brasileiro, com uso de dados da ciência-cidadã. Atualidades Ornitológicas 215, 8-11 [In Portuguese].
| Google Scholar |
Tubelis DP, Sazima I (2021) Nuptial gifts among Brazilian cuckoos: an outline based on citizen science. Ornithology Research 29, 188-192.
| Crossref | Google Scholar |
Tulloch AIT, Szabo JK (2012) A behavioural ecology approach to understand volunteer surveying for citizen science datasets. Emu – Austral Ornithology 112, 313-325.
| Crossref | Google Scholar |
Tulloch AIT, Mustin K, Possingham HP, Szabo JK, Wilson KA (2013a) To boldly go where no volunteer has gone before: predicting volunteer activity to prioritize surveys at the landscape scale. Diversity and Distributions 19, 465-480.
| Crossref | Google Scholar |
Tulloch AIT, Possingham HP, Joseph LN, Szabo J, Martin TG (2013b) Realising the full potential of citizen science monitoring programs. Biological Conservation 165, 128-138.
| Crossref | Google Scholar |
Tylianakis JM, Laliberté E, Nielsen A, Bascompte J (2010) Conservation of species interaction networks. Biological Conservation 143, 2270-2279.
| Crossref | Google Scholar |
Vale MM, Tourinho L, Lorini ML, Rajão H, Figueiredo MSL (2018) Endemic birds of the Atlantic Forest: traits, conservation status, and patterns of biodiversity. Journal of Field Ornithology 89(3), 193-206.
| Crossref | Google Scholar |
Vancine MH, Muylaert RL, Niebuhr BB, Oshima JEdF, Tonetti V, Bernardo R, De Angelo C, Rosa MR, Grohmann CH, Ribeiro MC (2024) The Atlantic Forest of South America: spatiotemporal dynamics of the vegetation and implications for conservation. Biological Conservation 291, 110499.
| Crossref | Google Scholar |
Wood C, Sullivan B, Iliff M, Fink D, Kelling S (2011) eBird: engaging birders in science and conservation. PLoS Biology 9(12), e1001220.
| Crossref | Google Scholar |
Zhang P, Wang J, Li X, Li M, Di Z, Fan Y (2008) Clustering coefficient and community structure of bipartite networks. Physica – A. Statistical Mechanics and its Applications 387, 6869-6875.
| Crossref | Google Scholar |
Zulian V, Miller DAW, Ferraz G (2021) Integrating citizen-science and planned-survey data improves species distribution estimates. Diversity and Distributions 27, 2498-2509.
| Crossref | Google Scholar |