The Sentinel Bait Station: an automated, intelligent design pest animal baiting system
G. Charlton




A School of Science and Technology, University of New England, Armidale, NSW 2351, Australia.
B College of Science and Engineering, Flinders University, Adelaide, SA 5001, Australia.
C Vertebrate Pest Research Unit, NSW Department of Primary Industries, Orange Agricultural Institute, 1447 Forest Road, Orange, NSW 2800, Australia.
D School of Environmental and Rural Science, University of New England, Armidale, NSW 2351, Australia.
E Vertebrate Pest Research Unit, NSW Department of Primary Industries, Building C02, University of New England, Armidale, NSW 2351, Australia.
F Vertebrate Pest Research Unit, NSW Department of Primary Industries, Coffs Harbour, NSW 2450, Australia.
Abstract
Ground baiting is a strategic method for reducing vertebrate pest populations. Best practice involves maximising bait availability to the target species, although sustaining this availability is resource intensive because baits need to be replaced each time they are taken. This study focused on improving pest population management through the novel baiting technique outlined in this manuscript, although there is potential use across other species and applications (e.g. disease management).
To develop and test an automated, intelligent, and semi-permanent, multi-bait dispenser that detects target species before distributing baits and provides another bait when a target species revisits the site.
We designed and field tested the Sentinel Bait Station, which comprises a camera trap with in-built species-recognition capacity, wireless communication and a dispenser with the capacity for five baits. A proof-of-concept prototype was developed and validated via laboratory simulation with images collected by the camera. The prototype was then evaluated in the field under real-world conditions with wild-living canids, using non-toxic baits.
Field testing achieved 19 automatically offered baits with seven bait removals by canids. The underlying image recognition algorithm yielded an accuracy of 90%, precision of 83%, sensitivity of 68% and a specificity of 96% throughout field testing. The response time of the system, from the point of motion detection (within 6–10 m and the field-of-view of the camera) to a bait being offered to a target species, was 9.81 ± 2.63 s.
The Sentinel Bait Station was able to distinguish target species from non-target species. Consequently, baits were successfully deployed to target species and withheld from non-target species. Therefore, this proof-of-concept device is able to successfully provide baits to successive targets from secure on-board storage, thereby overcoming the need for daily bait replacement.
The proof-of-concept Sentinel Bait Station design, together with the findings and observations from field trials, confirmed the system can deliver multiple baits and increase the specificity in which baits are presented to the target species using artificial intelligence. With further refinement and operational field trials, this device will provide another tool for practitioners to utilise in pest management programs.
Keywords: artificial intelligence, baiting, invasive species, machine vision, pest control, pest management, recognition, 1080.
Introduction
Vertebrate pests such as feral cats (Felis catus), European red foxes (Vulpes vulpes), feral pigs (Sus scrofa) and wild dogs (Canis familiaris) cause major negative impacts to agriculture and the environment (Braysher 1993; Fleming et al. 2017). Controlling introduced pests requires the integration of methods such as aerial and ground baiting to distribute toxic baits (Newsome 1990; Fleming et al. 2014; Ballard et al. 2020), trapping (Meek et al. 1995; Fleming et al. 1998; Meek et al. 2022) and the use of other devices (e.g. Canid Pest Ejectors (CPE’s); Busana et al. 1998; Marks et al. 1999; Marks and Wilson 2005) to achieve reductions in abundance that exceed the pest population’s ability to increase (Hone 1994). Ground poison-baiting can mitigate negative impacts but can be costly, and sustaining the level of control necessary to overcome population increase can be operationally demanding. Consequently, ongoing improvement of existing methods via technological innovation (Meek et al. 2020) is a useful objective.
A study on the effectiveness of controlling the reinvasion of a peninsula by foxes (Vulpes vulpes) highlighted the challenges associated with maintaining predators at low levels (Dexter et al. 2007). Invasion by foxes across a narrow isthmus provided an opportunity to increase the encounter rate with toxic baits. However, traditional bait stations can hold only single baits, so maximising availability to targets required daily replacement of baits (Fleming 1996, 1997). Time spent replacing baits in situations such as this comes at the cost of other operational imperatives, and demonstrates the need for a baiting system that can deliver toxin to successive targets with a reduced reliance on people to frequently replenish baits.
Non-target species add to the demand on bait replenishment by consuming, moving and/or degrading baits. Behavioural means of discriminating between targets and non-targets is already utilised in some control technologies (e.g. CPEs), but arguably reduces the likelihood of targets consuming toxin. Advances in image recognition, especially by our research group (Falzon et al. 2012; Falzon et al. 2020; Shepley et al. 2021a, 2021b), on incorporating such technology into a baiting device, show potential for creating target specific baiting that both protects vulnerable non-target species and reduces the removal of baits intended for pests by unaffected non-target vertebrates and invertebrates.
With the technological advances in computer science and engineering, designing automated toxin delivery devices specific to target species is now achievable (Meek et al. 2020; Moseby et al. 2020; Ross et al. 2020; Corva et al. 2022). We aimed to provide a reliable means of automatically replenishing baits while minimising undesirable bait loss (e.g. removal by non-targets) to increase the number of a target species encountering baits per unit of human effort. We designed, built and tested a proof-of-concept prototype to evaluate the potential to automatically and repeatedly deliver a bait to target pest species (such as wild dogs and foxes) using image recognition technology.
Methods
Overview
A prototype Sentinel Bait Station (SBS) was developed by integrating camera trap technology with a machine-vision-equipped single-board computer to provide the capacity to run species recognition algorithms on board a relatively small device. Low-power wireless connections between the camera and a bait-dispensing carousel (able to hold up to five baits) enabled a bait to be deployed only when the camera trap detected a species of interest. Both the camera and ground unit were able to stay active in the field for more than 7 days when using a custom-designed 120 Wh lithium–polymer battery pack. Initial target species for the SBS were wild dogs and foxes (hereafter referred to as ‘targets’). The study described below comprised four phases: (1) development; (2) collection of simulation images; (3) simulation testing; and (4) field testing.
Study sites
Development of the system and all laboratory testing was conducted at the University of New England campus in Armidale, New South Wales, Australia. Simulation images were collected across two distinct environments: Tablelands – University of New England, Armidale, New South Wales, Australia, and Coastal Eucalypt forest – Redhill Flora Reserve, Coffs Harbour, New South Wales, Australia. Field testing was undertaken during 2020 at two sites in the Cooper Basin, South Australia, Australia. Field trials involving animals were conducted under animal ethics approval permit number AEC19-026.
Development
Development involved designing and integrating two main components: the smart camera trap (Fig. 1a) and the bait-dispensing unit (Fig. 1b). The fundamental concept was to transform a standard camera trap into an intelligent cyber–physical system that could reliably and automatically dispense baits to animals of target species by using recent advances in machine learning. The design specifications were to build a system that could be placed in the field unattended for extended periods of time (e.g. multiple weeks or until all baits are taken).
Sentinel Bait Station (SBS) camera trap: (a) smart camera trap (220 × 120 × 100 mm) and (b) bait-dispensing unit (180ø × 150 mm).
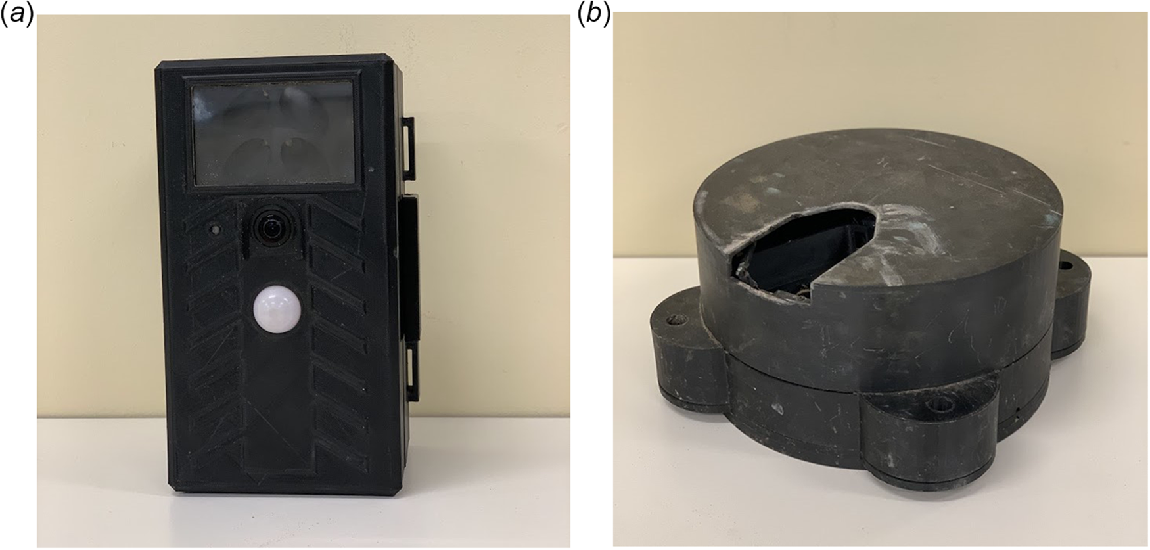
The intelligent camera consisted of a single-board computer, a combined passive infrared and microwave heat-in-motion sensing module and camera module (consisting of camera, dynamic near infrared filter and flash module outside of the visual range). This setup enabled the camera to automatically detect animal movement (day or night) at a range up to 15 m from the camera, photograph the animal and process the image to determine whether it was a target or non-target.
The SBS software system is an event-driven, finite state machine design equipped with machine vision, logic and wireless communication modules to provide advanced functionality. When the system is awaiting the presence of a target, the single board computer (Raspberry Pi 3B+, Raspberry Pi Foundation, Cambridge, UK) remains in idle mode until receiving an interrupt from the heat-in-motion sensing module. When heat-in-motion is sensed, the camera captures images that are processed by the species recognition algorithm. The species recognition algorithm was trained according to the location invariance methodology presented in Shepley et al. (2021b). During inference, the algorithm assigns a classification confidence score to detected objects (0–100%), with a higher score denoting a higher internal confidence by the algorithm that the target species is present in the image. A confidence threshold can be adjusted to the specific use case, as the main tuneable variable for determining whether the model favours false positives or false negatives. For this study, a confidence value of 5% was chosen (i.e. any image where the algorithm confidence value was more than 5% confident of a target species being present resulted in a positive detection). This value was chosen during development and early testing of the system to maintain a higher sensitivity to target species. The image detection model utilised within this design is MobileNet v1 model, which is a deep convolutional neural network (CNN) optimised for embedded system computer vision applications (Howard et al. 2017). The dataset used to train the model comprised of both publicly available images of target and non-target species sourced from FlickR and iNaturalist, along with an infusion dataset of camera trap images featuring strong similarities to those used in this study. The model was trained using transfer learning in accordance with the location invariance method to maximise robustness to unfamiliar camera trap settings. Importantly, none of these images were part of the simulation dataset mentioned below.
Once a target is detected, the computer communicates wirelessly (Fig. 2) to the bait-dispensing unit to offer a bait to the target animal. Two-way wireless communication between the single-board computer within the camera and the microprocessor (ESP32, Espressif Systems, Shanghai, China) within the bait-dispensing unit used the Message Queuing Telemetry Transport (MQTT) protocol over a wireless local area network (WLAN).
The system component diagram representing the ‘Smart Camera Trap’ equipped with a Raspberry Pi single-board computer coupled to heat-in-motion and camera units detecting the presence of a target predator and activating the bait dispenser via wireless communication to an ESP32 microprocessor.
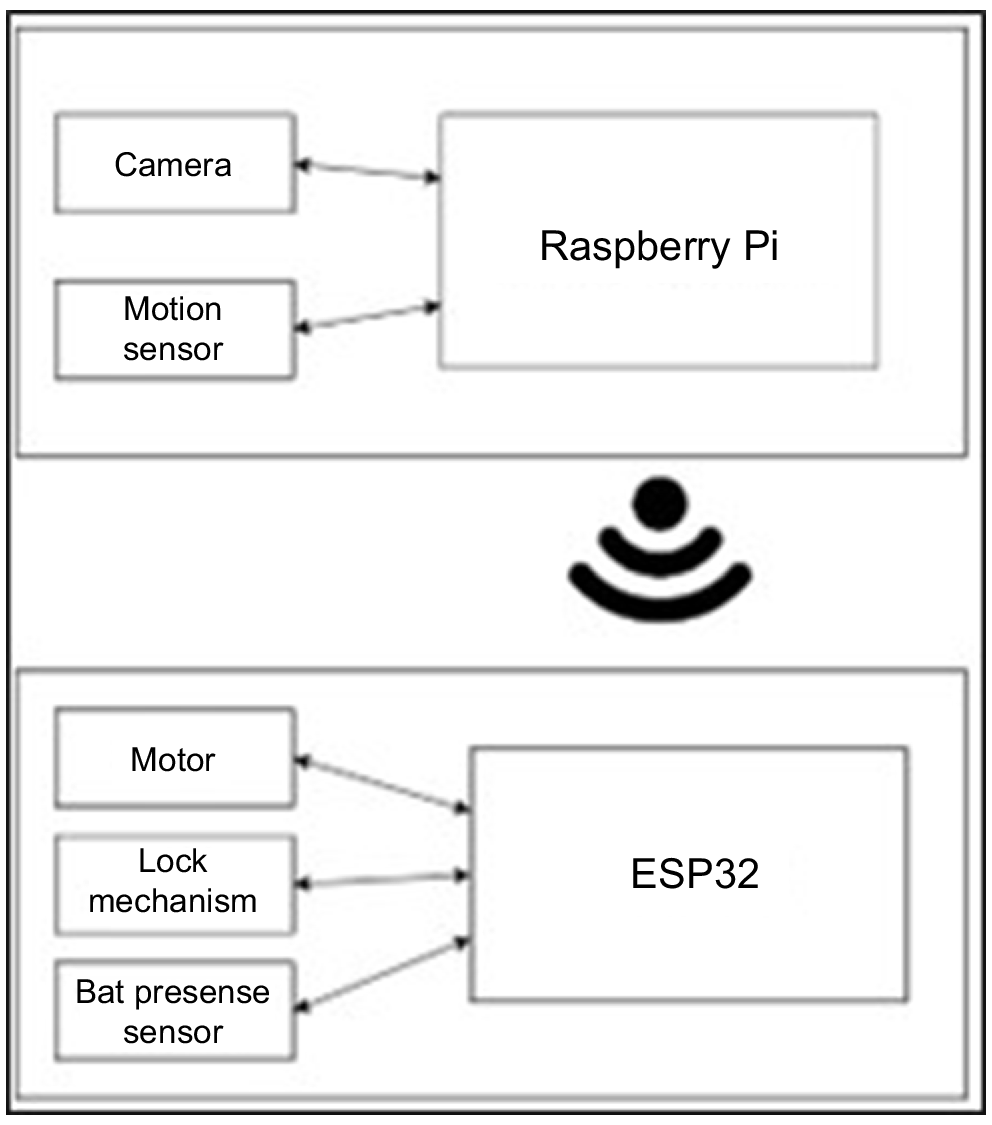
The rotating carousel within the bait-dispensing unit presents either an empty or baited compartment (Fig. 3) as required. During development, the carousel was designed to house five standard-sized manufactured dog baits (60 g each), but capacity could be increased. A stepper motor operates the carousel and a locking pin is used to maintain the desired position when the motor is inactive. An infrared light beam sensor (outside the visible range for canids) is used to determine if a bait is present or absent from the currently available compartment; this allows the SBS to ensure baits are presented to target animals, and if not consumed, enclosed for safe-keeping until the next detection occurs.
Computer-aided design (CAD) image of the bait dispenser showing the internal carousal design (the square structures within each triangle segment are the bait chambers and the empty segment is the starting point).
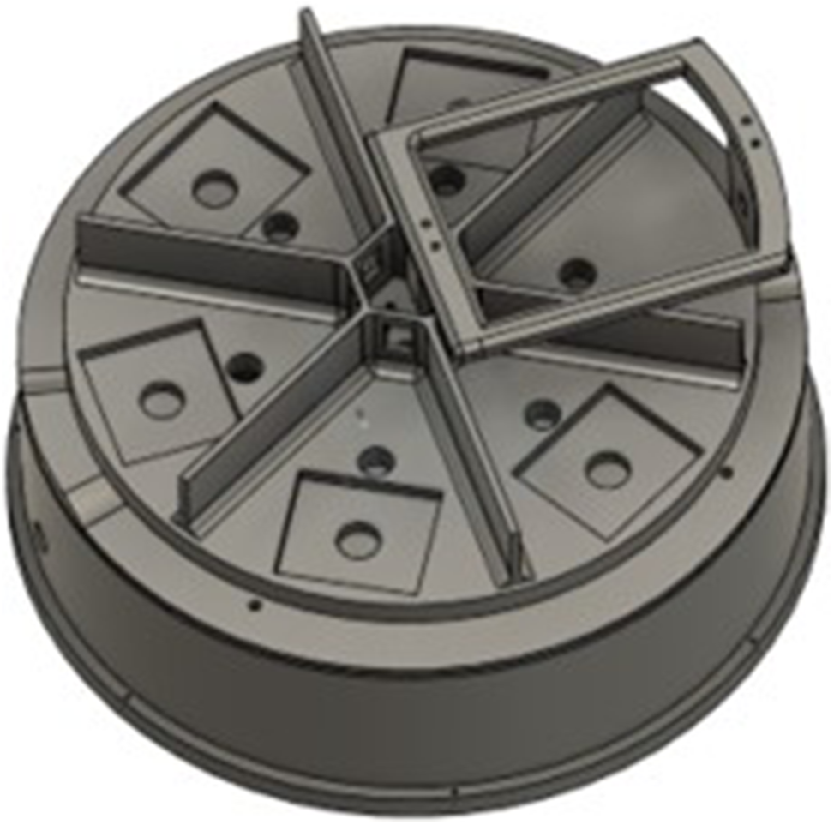
The field-tested design enabled the following operating scenario: the SBS camera is deployed with the bait dispenser approximately 5 m away, within the field of detection (Fig. 4). When the camera detects heat-in-motion it takes a pre-programmed number of photographs (e.g. for this study, 10 images at 1-s intervals). The on-board computer then rapidly performs image recognition to identify whether the animal is a target species or not. When a target is recognised, the computer instructs the bait-dispensing unit to offer a bait by turning the carousel to an open position. If the bait is not removed after 120 s, the carousel closes and awaits a new target detection. Alternatively, if the bait is removed, the device recognises this and will not expose another bait until 120 s has passed and another target is detected.
Collection and testing of simulation images
The images (n = 250) used for simulation testing were extracted from images collected by the camera during the development of the system. Images of ‘targets’ were only dogs or foxes (day: n = 100 images; night: n = 25 images), and ‘non-target’ images included people, cars, buildings and livestock (day: n = 100 images; night: n = 25 images). These images were not used in the training of the recognition algorithm to ensure a true ‘out of sample’ test. The data collection sites provided two habitat classes of high relevance for predator control: (1) Tablelands, which contained the presence of people, cars and livestock; and (2) Coastal Eucalypt forest, which contained a high biodiversity of mammals and birds. All sites included the target species (i.e. dogs and foxes) as well as non-target species and objects (i.e. birds, people, cars, building and other mammals). The prototype camera was deployed for periods of up to 14 days to collect images used in the simulation testing.
Field testing
The Cooper Basin desert dune system was used for field trials because wild dogs are locally abundant (Meek and Brown 2017). Birds such as the Australian raven (Corvus coronoides), Torresian crow (Corvus orru), wedge-tailed eagle (Aquila audax) and brown falcon (Falco berigora) are also locally common, regularly detected on camera traps and renowned for interfering with baits intended for mammalian pests (Allen et al. 1989). Field testing was undertaken to assess the performance of the end-to-end system based on encounters with real animals. Importantly, this was designed to assess whether the end-to-end system would offer bait to targets and withhold bait from non-targets, and whether animals offered baits would remove a bait made available to them. Two non-toxic bait types (approximately 50 × 50 × 40 mm) were used in the trial; manufactured 60 g Doggone® (Animal Control Technologies (Australia) Pty Ltd. Somerton, Vic., Australia) and fresh beef pieces (~60 gm) were used throughout the field trial. It was observed by one of the authors (PM) that there was little interest by the targets in consuming Doggone®. To gain a sense of attractiveness of Doggone® to the target species at this site, non-toxic baits were thrown to targets to observe their interest in consuming the bait. Because of the lack of interest in the Doggone®, the bait was changed over to beef pieces during the trial to increase the likelihood that baits would be removed/taken by the target species and confirm that the bait chamber design was big enough for a target species muzzle to access the bait.
The prototype housings for both modules were three-dimensionally (3D) printed using acrylonitrile butadiene styrene (ABS) to ensure a sufficiently robust system for proof-of-concept testing. The SBS camera was positioned approximately 1 m above ground on a post beside a well-used animal track. The bait-dispensing unit was partially buried and secured using tent pegs. The bait-dispensing unit was deployed approximately 4–5 m away from and in centre view of the camera. A battery power-pack was connected to the bait dispenser by a cable and buried so that animals could not interfere with it.
The system described above was left to operate in situ for 8 days at two sites in the Cooper Basin. Two commercial camera traps (Reconyx Ultrafire Reconyx, Inc. Holmen, Wisconsin, USA) were used to monitor the field-testing area (one positioned immediately below the SBS camera and a second facing along the animal track to detect fauna).
Statistical analysis
System performance was evaluated under daylight (natural illumination), night (infrared flash illumination) and for overall performance irrespective of illumination. Within the laboratory-based testing, each image was visually distinct and therefore evaluated as independent events, providing an understanding of model performance on a per image basis across environments. In field testing, an animal could enter the SBS camera detection zone, trigger the heat-in-motion sensor, and generate sets of multiple images (10 images at 1-s intervals). Combined, this full set of images was the detection event, because the first metric of interest was whether or not the SBS dispensed a bait when encountered by the target animal.
To quantify image data, each event was manually reviewed and then categorised as one of the following: (1) a true positive (TP), where a target was present and the system offered a bait; (2) a true negative (TN), where a target was not present and the system did not offer a bait; (3) a false positive (FP), where a target was not present and the system offered a bait; or (4) a false negative (FN), where a target was present and the system did not offer a bait.
Based on these categorisations, the system’s performance was determined by calculating values for accuracy, sensitivity, precision and specificity-balanced accuracy. Accuracy was calculated as the ratio of correctly predicted events (TP + TN) to the total number of events. Sensitivity was calculated as the ratio of true positives to the number of events with a target present (TP/(TP + FN)). Precision was calculated as the ratio of true positives to the number of events where the system predicted a target was present (TP/(TP + FP)). Specificity was calculated as the ratio of true negatives to events without targets present (TN/(TN + FP)). Balanced accuracy was calculated as the arithmetic mean of the sensitivity and specificity. The use of balanced accuracy addresses bias (inflated performance estimates) resulting from the imbalanced data classes encountered in field conditions (e.g. an unequal number of images with a target species present compared to the number of images with a target species not being present). Each of these values are presented as a ratio between 0 and 1, where a higher value represents higher performance.
Lastly, the time taken from a trigger to the single board computer analysing the picture and the bait being offered was also analysed using the system log files, which had millisecond precision. These values are presented as mean time period ± standard deviation.
Results
Simulation testing
Overall, 55% of the image recognitions were accurately identified, due mainly to the high proportion of false negative events (sensitivity: 0.11). However, the precision of the algorithm was 0.93 (day: 0.90; night; 1.00), with just one false positive event occurring within the day dataset. The sensitivity of 0.11 indicates that the system offered a bait in 11% of events where a target was present (day: 0.09; night: 0.20). Finally, the specificity was 0.99, meaning that the bait was withheld when a target was not present, but an image was captured on 99% of occasions (day: 0.99; night: 1.00).
The response from the bait-dispensing unit aligned 100% with the algorithm. No miscommunication was observed throughout testing. The response time of the system was 9.81 ± 2.63 s to dispense a bait (day: 9.53 ± 2.50 s; night: 10.35 ± 3.11 s; Table 1).
Time of day | Total events (count) | True positive (count) | True negative (count) | False positive (count) | False negative (count) | Accuracy (ratio) | Balanced accuracy (ratio) | Precision (ratio) | Sensitivity (ratio) | Specificity (ratio) | |
---|---|---|---|---|---|---|---|---|---|---|---|
Day | 200 | 9 | 99 | 1 | 91 | 0.54 | 0.54 | 0.90 | 0.09 | 0.99 | |
Night | 50 | 5 | 25 | 0 | 20 | 0.60 | 0.60 | 1.00 | 0.20 | 1.00 | |
Overall | 250 | 14 | 124 | 1 | 111 | 0.55 | 0.55 | 0.93 | 0.11 | 0.99 |
Field testing
Although both wild dogs and foxes were potential targets, no foxes were detected during field testing. Camera traps used to monitor the SBS system and immediate area recorded target and non-target species displaying interest in the SBS bait dispenser (Fig. 5). The SBS machine vision recognition module was able to detect the target species under a wide range of conditions; however, some false detections still occurred (Fig. 6d, f). During field testing, the accuracy of the system was 0.90 (day: 0.96; night: 0.69; Table 2). The balanced accuracy was 0.82 (day: 0.85; night: 0.73; Table 2), indicating a drop in performance due to class imbalance but the classifier still maintained average–good per event classification performance. The precision value of 0.83 indicates that the system offered a bait when there was a target present in 83% of events (day: 0.71; night; 0.88), with four false positive events occurring throughout the trial. The sensitivity value of 0.68 indicates that a bait was offered in 68% of events where a target was present (day: 0.71; night: 0.61), and the specificity value of 0.96 indicates that bait was withheld in 96% of events when a target was not present (day: 0.98; night: 0.85).
Detection events as recorded by the SBS camera module. Successful detections occurred under a range of challenging conditions including: (a) multiple target species present; (b) motion blur due to fast moving target species; (c) both target species and non-target (human) within the same image; and (d) camera trap illumination obscuring target species (note shadow of target species within image). In some circumstances, such as (e), both a true positive and false positive detection occurred simultaneously, and in other situations, such as (f), a false positive detection (the bait dispenser was incorrectly detected as the target species) occurred.
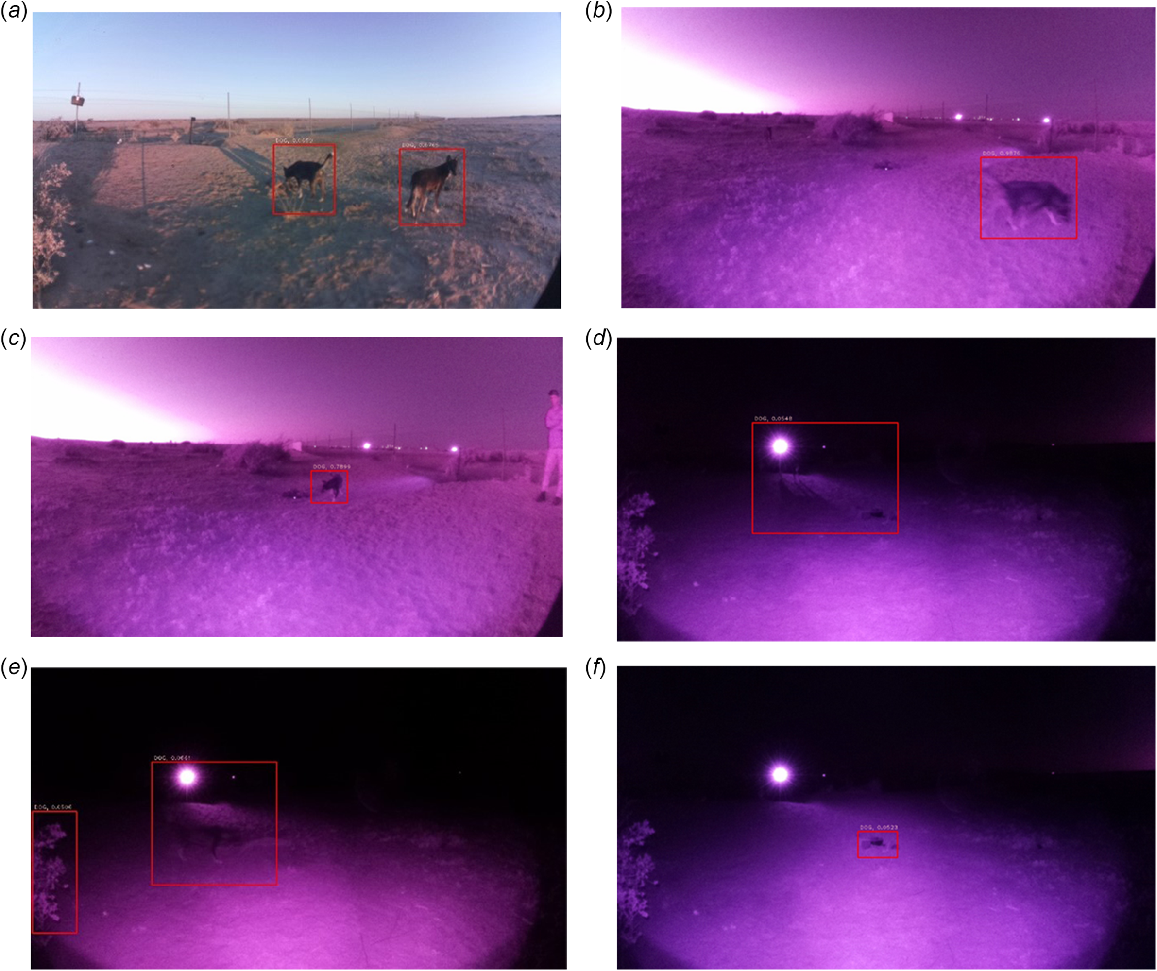
Time of day | True positive (count) | Baits taken (count) | True negative (count) | False positive (count) | False negative (count) | Accuracy (ratio) | Balanced accuracy (ratio) | Precision (ratio) | Sensitivity (ratio) | Specificity (ratio) | |
---|---|---|---|---|---|---|---|---|---|---|---|
Day | 5 | 3 | 90 | 2 | 2 | 0.96 | 0.85 | 0.71 | 0.71 | 0.98 | |
Night | 14 | 4 | 11 | 2 | 9 | 0.69 | 0.73 | 0.88 | 0.61 | 0.85 | |
Overall | 19 | 7 | 101 | 4 | 11 | 0.90 | 0.82 | 0.83 | 0.68 | 0.96 |
From 19 bait offerings made to targets, seven were removed from the device by a target species. When using the Doggone® baits, 2 of 8 baits that were offered were taken, but after switching to fresh beef pieces, 5 of 13 baits that were offered were taken. There were four false positive events, where the image recognition falsely detected non-targets as a target. The misclassified objects were the bait-dispensing unit (n = 2), vegetation (n = 1) and a person (n = 1). There were 11 false negative events (day: n = 2; night: n = 9) where a target was present, but a bait was not offered due to misclassification.
Discussion
To the authors’ knowledge, the SBS is a world-first solution comprising a camera trap with in-built species recognition capacity and wireless communication to a multiple bait delivery unit. Laboratory and field testing of this prototype device has yielded proof-of-concept evidence that the SBS can detect a target and present a bait (i.e. the system can detect heat-in-motion, rapidly capture and process a series of images to determine the presence of the target species and transmit a message to the carousel to offer a bait).
The response time of the system was similar for images taken during the day or night. The images were able to be processed in less than 2 s (i.e. the time taken from a motion trigger to the single-board computer analysing the picture) with the system completing the bait dispensing in approximately 10 s (i.e. the time taken from a motion trigger to the bait being offered). Therefore, on average, an 8-s delay was observed in offering the bait due to both the time taken for the actuators to move the bait into the open position and power saving methods with the wireless interface required to reduce the power consumption of the ground unit. This is likely adequate time for bait presentation prior to a target leaving the immediate area; additional testing could assess this.
It is highly likely the software can be optimised to further improve system performance. During the field testing, just over a third of bait offerings resulted in a bait being taken by the target. This was despite targets at the site having a ready supply of fresh food, possibly reducing their interested in baits when compared with targets under greater food stress or elsewhere. It was also possible that the manufactured baits were not attractive to dogs at the study site. As outlined in the methods, during the field trial the bait was changed over to beef pieces to increase the palatability and attractiveness of the bait to the target species. Although the change in bait increased the proportion of baits being taken when offered, the purpose of this study was to review the ability of the target species (in this example, wild dogs) to take baits from the bait-dispensing device rather than the baits’ palatability. Therefore, the authors assert that the change in bait type has little bearing on the results or findings.
Based on the simulation data, the sensitivity of the system was slightly better at night when using the infrared illumination to take photos in low-light scenarios. Specifically, the sensitivity (0.20) for night-time images indicates that for every five images of a target species taken at night-time, one of these images will result in a true positive event and is therefore a bait being offered to the target species. Conversely, the sensitivity for images taken during the day (0.09) suggests that 10 images would be required for one true positive event to occur and a bait to be offered to the target species. During field testing, the difference between day and night events was not evident, and this is largely attributed to how an event was considered. Specifically, within the field testing, 10 images were taken in succession after motion was detected, which increased the likelihood of a correct image recognition during each event and reduced the limitation of low sensitivity shown in the simulation. This system design decision was based on practical observations, where the target species investigated the bait dispenser, permitting multiple images to be collected in succession. This set of images can be rapidly processed by the machine vision module, with a high likelihood that at least some of the images within the batch will register detection of the target species. By utilising this algorithm design, it was feasible to incorporate a detection algorithm of lower computational complexity and hence more rapid processing times and lower power usage (at a cost of slightly reduced detection accuracy per image). The overall sensitivity during field testing (0.68) would indicate that approximately four out of five times (that a target entered the field of view of the camera) a bait was offered.
The low sensitivity observed in the simulations, which resulted in a low accuracy value, was due to the high number of false negative events. Such low sensitivity, and therefore accuracy, was not as prevalent during the field testing due to taking multiple images in succession when heat-in-motion was detected. The field testing showed that when a target was present near the system, a bait was offered in 68% of circumstances. This was slightly higher during the day (71%) than during the night (61%). Laboratory testing also involved a wider range of environments than field testing, which provides a challenging scenario for machine vision systems (Shepley et al. 2021b). Evaluations on a per image basis across a broad range of environments could have contributed substantially to the number of false negatives. Optimising model performance across diverse environments needs further investigation. Finally, due to the low number of false positive events compared with true negative events, the system demonstrated a very high specificity value for both the laboratory simulations (0.99) and field testing (0.96).
Overall, the system was better at detecting when a target species was not present within an image as opposed to identifying that there was a target species within the image. This aspect was a component of the machine vision algorithm design: detection thresholds were set to be conservative to minimise the number of bait offerings to non-target species. From a practical perspective for the specific use case field tested, a low false positive rate is more favourable because it is preferable to withhold a bait from a target species than to offer a bait to a non-target species, as currently occurs in most baiting situations. Additional development of the identification algorithms could be undertaken to further improve the accurate identification of target and non-target species (e.g. more images from the camera and environments used for deployment within the training dataset would likely improve the overall specifies recognition performance). To incorporate the SBS in other applications, it could be more effective to tune the algorithm to improve the false negative rate at the expense of the false positive rate, depending on the risk to other species if they were to access a bait.
There were four images where a non-target was incorrectly recognised as a target. The bait-dispensing unit was not highly represented in the training data and this may have contributed to the bait-dispensing unit being incorrectly recognised as a target species on two occasions. To avoid such false positives in the future, it would be important to include the bait-dispensing unit within further training images. Interestingly, in the other two instances a bush and a person at the outer edge of the field of view were misidentified. All objects detected as false positives had a low confidence value compared with that of most true positive events. This supports further adjustments to the algorithm detection threshold, requiring multiple target detections per SBS unit trigger, iterative training revisions and improvements to the algorithm (e.g. the inclusion of more negative samples such as images with people close to the border would make the algorithm more robust to those variations, thus reducing false positives).
Overall, we sought to offer baits to targets, rather than non-targets, and automatically replace these baits, so that more targets could be baited per unit of human effort. With this considered, the most important metric for the performance of the system is the precision value (i.e. the number of baits offered to a target in comparison with all baiting events). During field testing, precision was relatively high (0.83) due to the low false positive event count. Compared with current practice, this would significantly reduce the number of baits available to non-targets. Further model improvements could enable the precision of the system to be improved through the modification of the confidence value (5% used in this trial). The precision could be increased by increasing the target confidence threshold (e.g. only dispense baits where the algorithm is 50% certain that a target is present). However, there is a trade-off between avoiding dispensing a bait to non-targets and dispensing a sufficiently high number of baits to targets. Further algorithm training would allow for a higher confidence value to be used within the model and therefore reduce the number of false positive events without significantly reducing the sensitivity of the system. In contrast to unconcealed baits (the current practice), the SBS only permitted bait availability for 2 min. Critically, the SBS permitted access to baits in circumstances where there was a high likelihood that the target species was present.
Future development of the SBS could be centred upon improving algorithm performance to detect target species across environmental conditions, which would translate to higher precision and sensitivity. Also, broader field tests are required to understand SBS performance under different contact rates with target and non-target species. Fortunately, the modular design of the machine vision component of the software permits model substitution, so the SBS could be trained to offer baits to a broader range of target species, such as rodents, feral pigs or feral cats.
The MobileNet v1 Convolutional Neural Network was state of the art at the time of development, integration and testing of the SBS. The fast pace of machine vision research has offered additional innovations and increase in performance that could be included in future algorithms. Options could include: extreme learning machine architectures that are optimised for drones and field robotics (Sadgrove et al. 2018); modifications and improvements to the MobileNet architecture (Ayi and El-Sharkawy 2020; Kavyashree and El-Sharkawy 2021); or modifications to improve the robustness of object detection algorithms (Shepley et al. 2020). The development of a more specific and extensive dataset for model training would also be very useful. Due to the prototype nature of the SBS, very limited image data were available to develop the machine vision model. Empirical evaluations of camera trap-specific CNN algorithm performance indicate that substantially more data could be required per class across environments to optimise performance (Shahinfar et al. 2020). Inclusion of negative classes that are known (from field trials) to trigger false positive detections (such as the bait dispenser) in further model training will permit further model refinement.
The engineering design of the bait-dispensing system could also be modified to increase its robustness and ability to securely store more baits. Further, to be able to use toxic baits during field trials and ultimately in practice, the system would be required to address legislative requirements for storing and distributing multiple baits in a single location. Although not observed within the field trials, it is the opinion of the authors that refinements to the mechanical design of the system to reduce noise associated with the bait-dispensing unit when opening and closing may help to limit the associated startle behaviours by the target species in response to unnatural noises (Meek et al. 2016).
Conclusion
Laboratory testing found the SBS was able to recognise target species (i.e. dogs and foxes) and dispense baits to them. Field testing was highly target specific, and target species were willing to take baits from the bait-dispensing unit when offered. The performance indicates that the SBS system could be developed for use in real-world control programs to reduce both non-target uptake and human effort required to provide baits to multiple target species.
Data availability
This proof-of-concept device is still the subject of further research applications, and is a potential commercial product for the Centre of Invasive Species and University of New England. As such, data will remain confidential until this process is complete, and will remain the subject of commercial confidentiality requirements.
Declaration of funding
Funding for this research was provided by the Department of Agriculture, Water and the Environment through the Centre for Invasive Species Solutions. Dr Ballard, Dr Fleming and Dr Meek were funded by the NSW Department of Primary Industries, and Dr Falzon and Mr Charlton were funded by the University of New England.
Acknowledgements
The authors acknowledge the University of New England and NSW Department of Primary Industries staff who made a significant contribution to this and predecessor projects, including Derek Schneider, Jaimen Williamson, Joshua Stover, Ehsan Oshtorjani, Robert Farrell, Christopher Lawson, David Luckey, Phil Mendelson, Heath Milne, Elrond Chong and James Bishop, along with UNE Smart Farms Staff. Thank you to staff of the Cooper Basin for their support during these trials. Funding was provided by the Department of Agriculture, Fisheries and Forestry through the Centre for Invasive Species Solutions, and in-kind funding was provided by NSW Department of Primary Industries.
References
Allen LR, Fleming PJS, Thompson JA, Strong K (1989) Effect of presentation on the attractiveness and Palatability to wild dogs and other wildlife of 2 unpoisoned wild-dog bait types. Wildlife Research 16, 593-598.
| Crossref | Google Scholar |
Ballard G, Fleming PJS, Meek PD, Doak S (2020) Aerial baiting and wild dog mortality in south-eastern Australia. Wildlife Research 47, 99-105.
| Crossref | Google Scholar |
Busana F, Gigliotti F, Marks CA (1998) Modified M-44 cyanide ejector for the baiting of red foxes (Vulpes vulpes). Wildlife Research 25, 209-215.
| Crossref | Google Scholar |
Corva DM, Semianiw NI, Eichholtzer AC, Adams SD, Mahmud MAP, Gaur K, Pestell AJL, Driscoll DA, Kouzani AZ (2022) A smart camera trap for detection of endotherms and ectotherms. Sensors 22, 4094.
| Crossref | Google Scholar |
Dexter N, Meek P, Moore S, Hudson M, Richardson H (2007) Population responses of small and medium sized mammals to fox control at Jervis Bay, Southeastern Australia. Pacific Conservation Biology 13, 283-292.
| Crossref | Google Scholar |
Falzon G, Lawson C, Cheung K-W, Vernes K, Ballard GA, Fleming PJS, Glen AS, Milne H, Mather-Zardain A, Meek PD (2020) ClassifyMe: a field-scouting software for the identification of wildlife in camera trap images. Animals 10, 58.
| Crossref | Google Scholar |
Fleming PJS (1996) Ground-placed baits for the control of wild dogs: evaluation of a replacement-baiting strategy in north-eastern New South Wales. Wildlife Research 23, 729-740.
| Crossref | Google Scholar |
Fleming PJS (1997) Uptake of baits by red foxes (Vulpes vulpes): implications for rabies contingency planning in Australia. Wildlife Research 24, 335-346.
| Crossref | Google Scholar |
Fleming PJS, Allen LR, Berghout MJ, Meek PD, Pavlov PM, Stevens P, Strong K, Thompson JA, Thomson PC (1998) The performance of wild-canid traps in Australia: efficiency, selectivity and trap-related injuries. Wildlife Research 25, 327-338.
| Crossref | Google Scholar |
Fleming PJS, Ballard G, Reid NCH, Tracey JP (2017) Invasive species and their impacts on agri-ecosystems: issues and solutions for restoring ecosystem processes. The Rangeland Journal 39, 523-535.
| Crossref | Google Scholar |
Howard AG, Zhu M, Chen B, Kalenichenko D, Wang W, Weyand T, Andreetto M, Adam H (2017) Mobilenets: efficient convolutional neural networks for mobile vision applications.
| Crossref | Google Scholar |
Marks CA, Wilson R (2005) Predicting mammalian target-specificity of the M-44 ejector in south-eastern Australia. Wildlife Research 32, 151-156.
| Crossref | Google Scholar |
Marks CA, Busana F, Gigliotti F (1999) Assessment of the M-44 ejector for the delivery of 1080 for red fox (Vulpes vulpes) control. Wildlife Research 26, 101-109.
| Crossref | Google Scholar |
Meek PD, Brown SC (2017) It’s a dog eat dog world: observations of dingo (Canis familiaris) cannibalism. Australian Mammalogy 39, 92-94.
| Crossref | Google Scholar |
Meek PD, Jenkins DJ, Morris B, Ardler AJ, Hawksby RJ (1995) Use of two humane leg-hold traps for catching pest species. Wildlife Research 22, 733-739.
| Crossref | Google Scholar |
Meek P, Ballard G, Fleming P, Falzon G (2016) Are we getting the full picture? Animal responses to camera traps and implications for predator studies. Ecology and Evolution 6, 3216-3225.
| Crossref | Google Scholar |
Meek PD, Ballard G, Falzon G, Williamson J, Milne H, Farrell R, Stover J, Mather-Zardain AT, Bishop JC, Cheung EK-W, Lawson CK, Munezero AM, Schneider D, Johnston BE, Kiani E, Shahinfar S, Sadgrove EJ, Fleming PJS (2020) Camera trapping technology and related advances: Into the new millennium. Australian Zoologist 40, 392-403.
| Crossref | Google Scholar |
Meek PD, Ballard GA, Mifsud G, Fleming PJS (2022) Foothold trapping: Australia’s history, present and a future pathway for humane use. In ‘Mammal trapping: wildlife management, animal welfare & international standards. Vol. 5’. (Ed. G Proulx) pp. 81–95. (Alpha Wildlife Publications: Alberta, Canada)
Moseby KE, McGregor H, Read JL (2020) Effectiveness of the Felixer grooming trap for the control of feral cats: a field trial in arid South Australia. Wildlife Research 47, 599-609.
| Crossref | Google Scholar |
Newsome A (1990) The control of vertebrate pests by vertebrate predators. Trends in Ecology & Evolution 5, 187-191.
| Crossref | Google Scholar |
Ross R, Parsons L, Thai BS, Hall R, Kaushik M (2020) An IoT smart rodent bait station system utilizing computer vision. Sensors 20, 4670.
| Crossref | Google Scholar |
Sadgrove EJ, Falzon G, Miron D, Lamb DW (2018) Real-time object detection in agricultural/remote environments using the multiple-expert colour feature extreme learning machine (MEC-ELM). Computers in Industry 98, 183-191.
| Crossref | Google Scholar |
Shahinfar S, Meek P, Falzon G (2020) “How many images do I need?” Understanding how sample size per class affects deep learning model performance metrics for balanced designs in autonomous wildlife monitoring. Ecological Informatics 57, 101085.
| Crossref | Google Scholar |
Shepley A, Falzon G, Kwan P (2020) Confluence: a robust non-IoU alternative to non-maxima suppression in object detection.
| Crossref | Google Scholar |
Shepley A, Falzon G, Lawson C, Meek P, Kwan P (2021a) U-infuse: democratization of customizable deep learning for object detection. Sensors 21, 2611.
| Crossref | Google Scholar |
Shepley A, Falzon G, Meek P, Kwan P (2021b) Automated location invariant animal detection in camera trap images using publicly available data sources. Ecology and Evolution 11, 4494-4506.
| Crossref | Google Scholar |