Using expert elicitation to identify effective combinations of management actions for koala conservation in different regional landscapes
Emma B. Camus A B , Jonathan R. Rhodes A B , Clive A. McAlpine A B , Daniel Lunney C D E , John Callaghan F , Ross Goldingay F , Angie Brace G , Murray Hall A B , Scott Benitez Hetherington H , Marama Hopkins B , Marek J. Druzdzel I and Helen J. Mayfield
A Centre for Biodiversity and Conservation Science, University of Queensland, Brisbane, Qld 4072, Australia.
B School of Earth and Environmental Sciences, University of Queensland, Brisbane, Qld 4072, Australia.
C Department of Planning and Environment, Locked Bag 5022, Parramatta, NSW 2124, Australia.
D Faculty of Science, School of Life and Environmental Sciences, University of Sydney, Sydney, NSW 2006, Australia.
E Australian Museum, 1 William Street, Sydney, NSW 2010, Australia.
F Faculty of Science and Engineering, Southern Cross University, Lismore, NSW 2480, Australia.
G Lismore City Council, 43 Oliver Avenue, Goonellabah, NSW 2480, Australia.
H Tweed Shire Council, PO Box 816, Murwillumbah, NSW 2484, Australia.
I Faculty of Computer Science, Bialystok University of Technology, Bialystok, Poland.
Wildlife Research 50(7) 537-551 https://doi.org/10.1071/WR22038
Submitted: 26 February 2022 Accepted: 3 July 2022 Published: 21 July 2022
© 2023 The Author(s) (or their employer(s)). Published by CSIRO Publishing. This is an open access article distributed under the Creative Commons Attribution-NonCommercial-NoDerivatives 4.0 International License (CC BY-NC-ND)
Abstract
Context: Choosing the most effective combinations of actions to manage threatened species is difficult.
Aims: This study aimed to identify the most effective combinations of six management actions for reversing population declines of koala populations in three regional landscapes (Coastal, Riverine and Hinterland) in north-east New South Wales, Australia, based on expert-elicited knowledge.
Methods: A Bayesian network was used to combine continuous probability distributions representing the impact of specific management actions and combinations of actions. Data were derived from expert elicitation. Variation within and among expert judgements was captured by incorporating submodels of individual responses. We evaluated alternative management solutions to address four mortality factors (disease and injury, vehicle strikes, domestic dogs and wild dogs/dingoes (Canis familiaris), and two habitat-related management actions (restoration and protection).
Key results: We show that there are marked differences in the expected response of the koala populations to the various management options in the three regional landscapes over a 20-year period (2019–2039), and that multiple management actions are required to arrest and reverse the decline in koala populations of north-east NSW.
Conclusions: Management actions for koala conservation should be based on regional context. Our model, in conjunction with robust expert-elicitation procedures, allows decision makers to distinguish effective from ineffective combinations of management actions for threatened species management, and has been structured so that new data can be incorporated into the model.
Implications: Model design could be easily adapted to different species or conservation contexts, and updated as new evidence becomes available, making it valuable in adaptive management for local to regional-scale conservation problems.
Keywords: adaptive management, Bayesian network, expert elicitation, habitat restoration, koala conservation, roadkill, targeted management, threatened species recovery, wild dogs.
Introduction
Biodiversity is in crisis, with current rates of extinction exceeding background rates (Johnson et al. 2017). These extinctions are driven by different combinations of threatening processes (Brook et al. 2008; Mantyka-pringle et al. 2012), with interactions among the threats influencing populations in different ways (Bal et al. 2018). Identifying priorities for conservation actions is essential to effectively manage this complex system (Santika et al. 2015; Bal et al. 2018). Doing so requires identifying the highest impact threats, and then the most effective combination of management actions. However, such decisions are often limited by the availability of data and imperfect knowledge of specific threats (Scheele et al. 2018).
The koala (Phascolarctos cinereus) is impacted by numerous interacting threats across its distribution, which covers four states of eastern Australia, including inland and coastal areas (McAlpine et al. 2015; Tisdell et al. 2017). The major threats are habitat loss (clearing for urban and rural development, and resource extraction such as mining and logging), fire, disease, vehicle strikes, dog attacks, and impacts of climate change, especially increased drought and heatwaves (Melzer et al. 2000; Rhodes et al. 2011; McAlpine et al. 2015; DAWE 2022). The impact of each threat varies from population to population, even within regions (Adams-Hosking et al. 2015; McAlpine et al. 2015), which makes priority setting for regional management actions difficult. Setting management action priorities, therefore, needs to consider populations separately, and for interventions to be designed based on the particular characteristics of the local threats (Melzer et al. 2000).
Knowing which management actions, or combination of actions, are likely to prove effective for different koala populations or regional landscapes is crucial for deciding where to allocate management resources. Local knowledge is invaluable when considering the full range of local or regional-scale threats and solutions, with a view to an efficient prioritisation of resource allocation to koala conservation and management. An approach that fails to consider the range of local management issues risks placing too much emphasis on the more prominent or visible issues, such as roadkill, while potentially failing to address higher impact threats.
Although critical management actions, such as preventing habitat loss through improved planning to avoid development impacts, are essential, they are unlikely to be sufficient to achieve koala population recovery and sustainability unless other impacts are also managed. In the absence of concrete monitoring data or studies, expert knowledge can be used to estimate a species response to management (Burgman 2016; Mayfield et al. 2020a). In this context, it provides an ideal platform for articulating the local-scale differences in expected benefits to koala populations from different management scenarios. However, the challenge is how to combine this expert knowledge into a suitable framework for making predictions on management effectiveness and capture the uncertainty inherent in such an estimate, and to provide flexibility within the framework to incorporate new information. We addressed this challenge by combining a data modelling framework with a robust expert elicitation process to predict the best combinations of actions based on local knowledge and an ecological outlook.
The study aimed to identify the most effective management actions for reversing koala population declines in four Local Government Areas (LGAs) on the far north coast of New South Wales (NSW). We used a Bayesian network model (Pearl 1988) to integrate local expert knowledge on the threats and perceived effectiveness of management actions, and to capture the uncertainty around the impact of alternative actions. The outcome not only provides a necessary tool for koala management within the study region, but is also relevant to decision-making processes for other locations, state governments, and nationally, particularly in light of the national recovery plan for koalas (DAWE 2022), and the formal uplisting of the koala’s status in February 2022 for NSW, Queensland and the ACT from vulnerable to endangered under the Commonwealth legislation, following a discussion paper in 2021 (DAWE 2021).
Methods
The study encompassed three regional landscapes in north-east New South Wales. For each of the regional landscapes, a Bayesian network was developed to capture the change in koala population size over a 20-year period. Data to parameterise the Bayesian networks were collected, using expert elicitation, from a diverse group of experts. Experts were consulted in preliminary stages of the project – defining each management action and developing a conceptual model – and then participated in the four-stage elicitation process by completing structured questionnaires. The answers to the questionnaires were used as the data for the Bayesian networks.
Study area
State-wide studies of koala distribution have identified the far north coast of NSW as being the major stronghold since the first major state-wide survey in 1986–87 (Reed et al. 1990; Adams-Hosking et al. 2016). Our study spans four local government areas: Lismore (1290 km2); Ballina (485 km2); Byron (567 km2); and Tweed (1321 km2) (Fig. 1), and supports significant koala populations (Harris and Goldingay 2003; Brown et al. 2018). The coastal fringe mainly comprises urbanised environment, whereas inland areas are predominantly rural with National Parks and State Forests in sections of rugged terrain, but also include the regional centre of Lismore City with a residential population 29 000 (Australian Bureau of Statistics 2018). While the north coast has seen localised extinction of koala populations (such as in Iluka in the 1990s; Lunney et al. 2019b) from a combination of threats, interventions in other parts, for example Coffs Harbour (Lunney et al. 2019a), have allowed populations to persist.
![]() |
All four local governments have adopted a range of measures for protecting and managing koalas, and skilled staff have been appointed to design and implement koala conservation planning programs (Lismore City Council 2013; Byron Shire Council 2015; Ballina Shire Council 2017; Tweed Shire Council 2020). The strong community support for koala conservation is evidenced by the sustained efforts of local koala conservation and koala rehabilitation groups, such as the well-established Friends of the Koala (Lunney et al. 2022a). Koala habitat within the broader region typically varies, containing a range of eucalypt forest communities that support differing compositions and densities of preferred koala food and shelter tree species (Callaghan et al. 2011). Based on discussions with local experts, we captured variations in koala numbers and threats across the study area by dividing it into three regional landscapes – Coastal, Hinterland, and Riverine (Fig. 1). These regional landscapes were based on topography, vegetation cover, land use, and local knowledge of koala distribution and threats to koalas.
Defining management actions
We identified six management actions (Table 1, Supplementary material Appendix S1) grouped into two habitat-related management actions (restoration and protection) and four mortality-related management actions (vehicle strike hotspot management, wild dog management, disease and injury management, and domestic dog management). The actions address each threat and involve a suite of potential activities that may or may not be feasible or appropriate for specific locations. The actions were identified based on a review of existing literature and an initial consultation with local koala rehabilitation group Friends of the Koala, together with the four local councils involved in koala conservation.
![]() |
Conceptual model
A conceptual model was developed based on the literature and consultation with local experts to represent the interactions among threats, management actions, and population trends in the study area (Fig. 2). Populations were defined as the number of adult koalas in each regional landscape. The conceptual model illustrates the main direct influences of four management actions aimed to address the impact on koala population size of disease and injury, vehicle strikes, domestic dogs, and wild dogs/dingoes. Both domestic and wild dogs/dingoes (Canis familiaris) are present in north-east NSW (Lunney et al. 2021). Two management actions (habitat restoration and habitat protection) restrict the impact of these direct actions via carrying capacity, which ultimately limits population size. In this conceptualisation, changes to the upper components influence the overall change in population through effects on koala mortality and habitat.
Bayesian network design
Bayesian networks are probabilistic models based on directed acyclic graphs, in which nodes represent system variables and directed edges define influences among them (Pearl 1988). Variables in a Bayesian network can be both discrete and continuous, and interactions among them can be modelled by using both equations and conditional probability distributions. A Bayesian network model represents the joint probability distribution over its variables, reducing it into a product of conditional probability distributions expressing local interactions among directly related subsets of these variables (Marcot et al. 2006; Fenton and Neil 2013). Having a representation of the joint probability distribution allows for Bayesian inference, which amounts to calculating the posterior conditional probability distribution over variables of interest given observations of other variables. In those domains, for which the exact functional form of interactions among variables is not known, Bayesian networks offer a flexible representation of relationships among key components of the modelled system. Because the directed graph of a Bayesian network can represent the causal relations within a system, they are useful tools for evaluating potential impacts of management decisions, i.e. external manipulations of the modelled system (Marcot et al. 2001; McCann et al. 2006; Mata et al. 2017). Importantly, Bayesian networks can easily be parameterised using empirical data (Mayfield et al. 2020b), or by using expert elicitation (Smith et al. 2007; Richards et al. 2013), or a combination of the two, making them suitable for use in both data-rich and data-poor situations.
Based on the conceptual model (Fig. 2), we developed a Bayesian network structure (common for each of the regional landscapes; Fig. 3) and parameterised each network separately, using expert judgments for each landscape (Coastal, Riverine and Hinterland). This resulted in three separate models with identical network structures, but different parameters. Experts provided values for their highest, lowest, and best guess estimates for population response to management, as well as their certainty in their estimates (see following section for description of the elicitation process). For each landscape, we used the values provided by each expert (see following section for description of the elicitation process) to develop individual-level submodels (Appendix S2). The target node (response variable) of the Bayesian network was ‘Change in population size’ and it represented the predicted proportionate change in koala population from 2019 to 2039 (i.e. a span of time equal to approximately three generations of koalas).
Within each submodel, change in population size was calculated as ‘Population size 2039’ divided by ‘Population size 2019’ (Eqn 1). To account for uncertainty in the estimates, the range of values for the population size in 2019 derived during elicitation (Appendix S3, Question 1) is represented by a triangular distribution of the lower bound (L), best guess (B), and upper bound (U) values (Eqn 2), each value standardised to 80% based on the experts’ confidence levels using linear extrapolation prior to input (Bedford and Cooke 2001; Adams-Hosking et al. 2016). ‘Population size 2039’ reflects the lower of the two values of ‘Population response’ and ‘Carrying capacity 2039’ (Eqn 3), effectively capping the population size by the estimated carrying capacity of the habitat.



Each individual level submodel was split into two components based on the conceptual model (Fig. 2). The first calculated ‘Carrying capacity 2039’ by using the elicited carrying capacity for 2019 and multiplying this figure by the predicted change in habitat area. It was assumed that change in habitat area directly resulted in a proportionate change in the carrying capacity of the individual landscape. The formula for ‘Change in habitat area’ combined three nodes (Eqn 4).

The first node, ‘Habitat area 2019’ was an elicited reference point (Appendix S3, Question 3), to which ‘Area of habitat restored’ (Eqn 5) and ‘Area of habitat lost’ (Eqn 6) were combined to give a change in habitat area.


The triangular distributions used in the latter two nodes are defined by the set of elicited values (lower bound (L), best guess (B) and upper bound (U)) relevant to each numbered question. The ‘choose function’ was used to select a formula based on a ‘Yes’ or ‘No’ response for each management action. The management actions – in this case, habitat protection and habitat restoration – became the decision nodes for this component of the network. Decision nodes in this design acted as the interactive components of the model – the user would set each decision node to either ‘Yes’ or ‘No’, activate the model (update beliefs) and then interpret the results of the output at the target node.
In the second component, the four mortality-related management actions were incorporated as decision nodes: ‘disease and injury management’, ‘domestic dog management’, ‘vehicle strike hotspot management’, and ‘wild dog management’ (actions outlined in Table 1, Appendix S1). Each had an option of a ‘Yes’ or ‘No’ choice. Combinations of management actions were incorporated into a conditional probability table (chance node), in which ‘Yes’ (Y) and ‘No’ (N) responses for each action were each assigned either 100% or 0% chance of occurring. Thus, there were 16 possible combinations of these four management actions. Values for each combination of management actions were elicited individually.
Each combination of actions activated unique values in the child node, ‘Population response’ (Eqn 7), which would sample 50 000 possible values from the activated triangular distribution. As with habitat-related management actions, the triangular distributions are defined by the set of elicited values [lower bound (L), best guess (B) and upper bound (U)] relevant to each numbered question (Appendix S3, Questions 8–23), standardised to 80% based on the expert’s confidence using linear extrapolation.
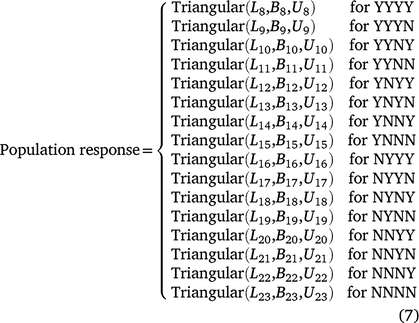
Once both population response and carrying capacity were defined, the network estimated the population size for 2039 by taking the lowest of these two components (Eqn 3), effectively capping the population size at the predicted carrying capacity of the habitat.
The seven, individual-level Bayesian networks (one per expert) for each regional landscape were designed as submodels within the final model (Fig. 3). Decision nodes representing the management actions were programmed to activate management action combinations for all submodels simultaneously, generating seven individual-level estimates for change in population size as a result of a management action combination, each with triangular distributions representing uncertainty. The distribution for the overall change in population was the mean distribution of the seven individual distributions (Fig. 3). The Bayesian networks were designed to incorporate continuous data to represent the triangular distributions elicited through the questionnaires. Thus, each node contained an equation that included, at a minimum, lower bound (L), upper bound (U) and best guess (B) estimates for that value (), standardised to 80% based on their confidence levels using linear extrapolation (Bedford and Cooke 2001; Adams-Hosking et al. 2016). The submodels (including each node, equation and state) are described in Appendix S2.
Expert elicitation
The data collection phase of this study was conducted in 2019, before the bushfires in 2019–2020. These bushfires covered only a small area of our north-east NSW study area, so the issues we identified were not overwhelmed by the fires. To estimate the conditional probabilities in the Bayesian networks, the expert elicitation process used here followed the IDEA protocol – ‘Investigate’, ‘Discuss’, ‘Estimate’, ‘Aggregate’ (Hemming et al. 2018), and used a four-point elicitation question style (Burgman 2016). Experts were defined by their qualifications, track record, professional standing, and experience (Burgman et al. 2011; Burgman 2016). A diverse selection of experts participated, including those with direct, local experience in koala management, those with specialisations in some aspect of koala conservation, and those with broader koala conservation experience across Australia. The elements of the IDEA protocol were adapted according to the timeframe and resources available. The number of experts completing each step of the process ranged from 7 to 16 (Table 2).
![]() |
A preliminary consultation was held, involving 16 experts from a range of backgrounds and professions comprising representatives from local government (3), state government (1), NGOs (2), environmental consultants (2), university-based koala ecologists (6), and modellers (2). Experts defined the three regional landscapes of the study area and began to consider management actions in these landscapes. All experts from the preliminary consultation were then contacted via email, presented with introductory material, and asked to complete the questionnaire individually (Appendix S3) over 2 weeks (‘Investigate’ phase). Each question, following the four-point format outlined by Burgman (2016), asked experts to provide estimates for the highest, lowest, and most likely values for conditional probabilities. Experts were also asked to rate their confidence that the true value would be between their lowest and highest estimates. Responses were compiled (n = 8), aggregated, and visualised using Microsoft Excel for use as a visual guide in a facilitated workshop (‘Discuss’ phase).
The ‘Discuss’ phase was conducted in the expert elicitation workshop, held in August 2019. The workshop involved nine experts – local and state government conservation officers (3), university-based koala ecologists (3), and volunteers from koala-focused, non-government organisations (3). Experts (n = 9) were provided with tailored questionnaires, each containing a record of their initial estimate (for those experts who took part in the Investigate phase), and a space to record new estimates if necessary. Discussion was facilitated by EBC and HJM and focused on clarification of terms, reasoning, and evidence, rather than aiming towards reaching a consensus, and this goal was made clear to the experts. The structure of the discussion mirrored the structure of the questionnaire in order to give experts the opportunity to evaluate each question. For questions 8–23 (Appendix S3), there was a strong focus on defining each mortality-related management action, as well as considering the impacts of each action.
After the group discussion, each expert was given the opportunity to amend their estimates (the ‘Estimate’ phase). Each questionnaire was divided into two sections. The first section contained seven questions about baseline population sizes, the impact of research on specific management actions, the coverage and carrying capacity of koala habitat in each regional landscape, and potential for change in habitat coverage over the next 20 years (2019–2039). The second section contained 16 questions about four conservation actions to reduce mortality (actions defined in Table 1, Appendix S1). It is acknowledged that gauging the success of any single management action, or combinations of actions, can be difficult because drought or good rains will have strong effects on population sizes. Questions were therefore structured such that estimates were provided assuming non-drought conditions.
Only the data collected from experts who participated in all stages of the elicitation process were included in the final output (n = 7). However, input from all experts was welcomed during the ‘Discuss’ phase, including data from experts who had filled out the questionnaire but were not present at the workshop. The finalised conditional probabilities from each expert were standardised to 80% based on their confidence levels using linear extrapolation (Bedford and Cooke 2001; Adams-Hosking et al. 2016). These data were then used to parameterise the Bayesian networks for subsequent analysis.
Analysis
To quantify the consequences of different choices of management actions, we defined a range of management scenarios and used the model to assess the implications for koala population trends under each scenario. These scenarios consisted of combinations of all, one, or no management actions for each regional landscape, combinations of all but one management action, and paired management actions. To derive estimates from the model, clustered sampling algorithms were applied using 50 000 samples generated by the GeNIe modeller software (https://www.bayesfusion.com/genie). That is, at each node, 50 000 possible values were sampled from each triangular distribution and used in the calculations for the subsequent child nodes. High upper estimates, combined with low confidence declared by one or more experts, sometimes led to the possibility of very extreme estimates being sampled in very low frequencies by chance. Therefore, the upper bound of the domain for ‘change in population size’ was set at 6 (i.e. an increase in population size of 600%).
We also included scenarios that combined habitat protection plus one other management action (i.e. disease and injury management, domestic dog management, wild dog management, or management of vehicle strike hotspots); then habitat restoration with one other action; domestic and wild dog management plus one other action; disease and injury, and vehicle strike hotspot plus one other action; and then finally combinations of all actions with one action missing. The results are presented separately for each landscape and expressed as the change in population size by 2039 (i.e. a 20-year horizon).
Results
Under the ‘no change to management’ scenario, i.e. the status quo, populations were predicted to decline in all three regional landscapes (Table 3, s1; Appendix S4). Estimated decline was greatest in the Riverine landscape (0.69), where a value below 1.0 identifies a population decline. The Hinterland landscape showed the least-estimated decline, with the proportion surviving the 20-year period being 0.87 (Table 3, s1). In contrast, if all management actions were undertaken, the populations in each regional landscape were predicted to increase over the 20-year time frame, with the Riverine showing the greatest increase of 1.40. When mortality-related actions were implemented as sole actions in the Coastal and Riverine landscapes, the most effective was disease and injury management, whereas wild dog management was the least effective (Table 3, s4, s7). By contrast, vehicle strike hotspot management was the most effective mortality related management action in the Hinterland landscape, and the least effective was disease and injury management (Table 3, s6, s4).
In the multi-action scenarios, where all but one actions were implemented, all regional landscapes were estimated to have a positive response, with the Riverine landscape showing the best response overall (Table 4). The Riverine landscape showed the best two responses of the entire suite of all regional landscapes, with scenarios implementing all actions, except either domestic dog management or habitat protection, showing the highest koala population growth rate over the 20 years (2019–2039). Although there is little discernible difference in the growth response when only one management action is missing (i.e. no one action tips the balance in favour of growth), it was noticed that removing disease and injury management from the combination resulted in the lowest predicted increase in population for both Coastal and Hinterland regional landscapes (Table 4, s27–s32; Appendix S5).
In scenarios with two to three actions implemented (Table 4, s8–s26), the six strongest management responses are in the Hinterland and the Riverine landscapes. Habitat restoration, vehicle strike hotspot, and disease and injury management for the Riverine landscape had the strongest response, with a 21% increase in population in the next 20 years. In the Hinterland landscape, a combination of habitat protection, vehicle strike hotspot, and disease and injury management had a strong estimated response, with a 17% increase in population. The same combination also showed an increase in Coastal and Riverine landscapes. The third highest score was scenario 26, in the Hinterland landscape – a combination of wild dog management, vehicle strike hotspot management, and disease and injury management. When disease and injury management and hotspot management are considered with one other management action, there are positive responses across the board (Table 4, s22–s26). Overall, the best set of responses are in the Hinterland landscape, with the clearest responses coming in conjunction with either habitat protected in the Hinterland landscape or habitat restoration in the Riverine landscape (Table 4, s23–s24).
Neither habitat protection nor habitat restoration alone were sufficient to reverse the decline of koala populations in any landscape (Table 3, s2, s3, Table 4, s8). For the Hinterland landscape, every combination of either habitat restoration or habitat protection with one other management action produced an increase in population size, except for the combination of these two alone (i.e. habitat restoration and habitat protection). In the Coastal landscape, neither of the habitat measures was sufficient to stem the population decline.
When domestic and wild dog management were considered together, with one other management action, most results showed weak increases or negative growth responses for koala populations in the Coastal landscape, but not for Riverine or Hinterland landscapes, where there were small gains. This is especially true for Riverine landscape, with the addition of habitat restoration, and in the Hinterland landscape with disease and injury management (Table 4, s17–s21). The Hinterland landscape showed the strongest response, with the best combination being wild and domestic dog management combined with disease and injury management (Table 4, s20). When habitat protection or restoration were added to the management of both wild and domestic dogs, there was positive population growth, although not strong (Table 4, s18–s19).
Regional landscapes differed in their potential to respond to management actions (Table 4). The Coastal landscape was estimated to have the weakest overall response, whereas the Hinterland landscape showed the strongest positive response in terms of koala population growth. The Riverine landscape showed a stronger positive response than the Hinterland landscape when only one management action was omitted. The response in growth rate across all actions ranged from 0.73 to 1.40 (Tables 3, 4). However, most responses were clustered nearer to 1.0.
In general, there was little variation in trends of the experts’ best guess estimates, indicating a consensus on the relative effectiveness on the management actions in the regions (Appendix S6). Generally, the strongest consensus was in the Riverine region, and the lowest was in the Hinterland region, where most actions were positively skewed, suggesting that more experts estimated values below the mean and fewer experts estimated values above the mean for each of the scenarios. Scenario 4 (disease and injury management as a standalone action) was an exception to this rule, with the strongest consensus for this action in the Hinterland region, and the weakest consensus in the Coastal region. In the Riverine region, although most estimates for Scenario 4 were below 1 (i.e. a decrease in population size), the maximum best guess for this region was over 1 (i.e. increase in population size). The single action with the strongest consensus was wild dog management in the Coastal region, and the weakest consensus was wild dog management in the Hinterland region.
Discussion
The koala is impacted by interacting threats, so it is crucial for managers to know which actions, or combination of actions, are likely to prove effective for the specific populations. This paper addressed the challenge of combining expert knowledge with a framework for making predictions on management effectiveness to capture the uncertainty and to provide flexibility to incorporate new information. A major finding was that, if no additional management is carried out over the 2019 baseline, the koala populations in the far north coast region of NSW were predicted to continue declining over the 20-year period of 2019–2039. Further, the nature of the decline was found to differ across three regional landscapes in the study area of north-east NSW. If single management actions are taken, there will be, by and large, little gain, with one of the regional landscapes – the Hinterland – gaining marginally more than either of the other two – the Riverine and Coastal landscapes.
Koala populations in all three regional landscapes were predicted to differ in their response to management actions. In the Hinterland, most mortality-related actions were predicted to support the recovery of the koala populations, subject to habitat protection and restoration, with the most successful estimated to be wild dog/dingo management. The Hinterland is the largest of the three regional landscapes, and its size, and extent of remaining forest, in rugged terrain possibly provides a more secure foundation for koala populations. In contrast, in the Riverine landscape, disease and injury management was the only mortality-related action predicted to lead to an increase of the koala population when implemented as a sole action, and only by a small margin.
Koalas in both urbanised and rural environments are at risk from mortality related to vehicular collisions (Dique et al. 2003; Rhodes et al. 2014; Gonzalez-Astudillo et al. 2017; Lunney et al. 2022a, 2022b), but our results predict that the management of vehicle strike hotspots is likely to be more effective in the Hinterland and Riverine landscapes than in the Coastal landscape. Although it was not possible to validate model predictions against empirical data, the results accurately reflect the best guess of experts in the area. These findings highlight the need for local planning, and coordination across each of the councils to find regionally specific solutions. The three regional landscapes we identified therefore offer management advantages by focusing strategic planning and conservation actions to address needs based on geographical similarities, rather than local government boundaries.
The study outcomes confirm that the best way forward is to implement combinations of actions, but with careful emphasis on the specific suite of measures most likely to produce the most positive response within each of the regional landscapes. Notably, solely protecting and/or restoring habitat will not be sufficient to arrest the population decline. If all six management actions are implemented, then koala populations are predicted to increase. However, if any one of the mortality management actions is dropped, the population’s recovery will be similar to what would have occurred had all six actions been taken.
When each management action was combined with other actions, the recovery, or failure to recover, was telling. It was the various combinations of actions that either failed to prevent the decline in koala populations or provided the potential for recovery over the 20-year period of 2019–2039. From a manager’s perspective, it is valuable to consider each local landscape as a separate unit for strategic planning and management purposes. Some combinations of management actions that are suited to the needs of one landscape are not predicted to be optimal in another. The lesson becomes clear: one action alone is not enough to prevent extinction, and although some combinations slow but do not stop the decline, some combinations offer the most efficient and effective approach for koala conservation in the far north coast of NSW to reverse the decline to extinction.
The six management actions fall into two categories, namely reducing mortality factors, e.g. death rates, through wild dog/dingo management, domestic dog management, vehicle strikes, i.e. roadkill, injury and disease management; and increasing population growth potential through protecting and restoring habitat. Although habitat protection and/or habitat restoration alone were not predicted to be sufficient to reverse the decline of koala populations in any landscape, any changes in habitat area will result in changes in the potential stability, viability, and maximum size of the koala population. Our model predicts that a combination of habitat management and any other individual action would likely reverse the decline of the koala populations, with the exception of one combination in the Coastal landscape (habitat restoration, habitat protection, and either wild or domestic dog management). In addition, the potential to stabilise or increase the koala populations by 2039 using a single action to reduce mortality in any landscape was increased by protecting and restoring habitat. This makes logical sense because populations would be expected to decline if habitat is decreased, but have potential to expand where habitat is protected and ultimately increased or enhanced through restoration programs. If managers seek to reverse the decline in koala numbers through the improvement of a single, mortality-related management action in the Coastal landscape, they will therefore also need to protect and restore habitat.
The need for combinations of actions is consistent with other work recognising that multiple management actions are required to ensure the success of a recovery strategy (Brook et al. 2008; Mantyka-pringle et al. 2012; Beyer et al. 2018). Our study takes the next step by establishing a potential mechanism to systematically compare and contrast the likely outcomes from specific combinations of conservation actions.
Given our findings that multiple actions are required to improve the prognosis for koala populations, consideration is needed on the feasibility of this occurring, given the range of government jurisdictions responsible for implementation. For example, the National Wild Dog Action Plan 2020–2030 (https://wilddogplan.org.au/wp-content/themes/nwdap/docs/NWDAP2020–2030.pdf, accessed 16 April 2021) is a responsibility of the NSW State government. However, the licensing and management of domestic dogs is the responsibility of local councils, and a separate state government department collates the figures.
For habitat protection, the selection and dedication of National Parks and Nature Reserves is a state government matter (Lunney et al. 2018); however, local councils also operate as the consent authority for legislation established by the state government and have a key role to play in land-use decisions and the overall shire-wide plans for development or habitat retention. Habitat restoration programs can be conducted at different scales, individually or collectively, and can be supported through local, state and commonwealth government initiatives or grants or philanthropic organisations. Disease and injury management has been largely taken up by local volunteer groups operating under licence from the NSW State government (Lunney et al. 2022a). Vehicle strike hotspot management is the responsibility of the State government for highways, but the management of local and arterial roads falls to local councils. Thus, it becomes apparent that any management decisions aimed at recovering koala populations will have responsibilities and costs that fall to different people at different levels of government as well as individuals, and to koala conservation and rehabilitation groups. This highlights the importance of collaboration across different administration levels in achieving conservation outcomes.
The decision-making approach we developed to assess the use of conservation resources for koala management is both timely and beneficial for local conservation planning and action. Central to the success of this project was the approach to the modelling and data acquisition. The data used were based on judgements from a range of experts, and aggregating those responses is a common challenge in expert elicitation programs, particularly where expert estimates vary considerably (Kuhnert et al. 2010). Commonly, these results are simply averaged (Hemming et al. 2018); however, doing so may not adequately represent the breadth of opinions (Adams-Hosking et al. 2016; Burgman 2016). We applied an innovative modelling approach by integrating each expert into the main design as a separate submodel, with associated levels of confidence incorporated into each node.
A key advantage of the model design over more traditional, categorised Bayesian networks is that uncertainty from the elicitation process has been preserved by using continuous distributions. Doing so allowed the model outputs to be presented as minimum, maximum and average values for each scenario. This extra information becomes meaningful in the context of prioritisation, where decision makers may opt for more certain outcomes. Another advantage of this approach over previous designs is that it is a more formal integration of individual expert data. By constructing detailed submodels for each set of expert data and embedding triangular distributions into all stages of the model, our study was better able to capture uncertainty than if answers had been aggregated prior to input. This uncertainty is apparent in the full range of values (Appendix S7). Although the bulk of data is centred on the mean, the wide range and long tails attached to each value show much uncertainty that could be reduced by further research, or by incorporating empirical data into the model.
Another advantage of this model is the flexibility of the design, which allows for the inclusion of new data and is such that the model can be scaled to a variety of contexts. New expertise, data or evidence can quickly be integrated into the model as a submodel. This may include population studies that prompt a revision of current population estimates, studies regarding one or more threatening processes, or outcomes from an implemented management action. Further, additional threatening processes, such as climate change or adverse fire regimes, could be added to the model if new data (elicited or otherwise) become available. In addition, the style and structure of this model could be adapted to different threatened species and other conservation problems. Individual components of the model may be refined through further elicitation. For simplicity, the carrying capacity of the habitat was assumed to be linearly related to an increase in habitat area. In addition, it was assumed that carrying capacity would not be exceeded, even when pressures from other threats were reduced. This assumption may not hold in all situations; koalas can become overpopulated in the absence of regulating impacts (Masters et al. 2004; Whisson et al. 2016). However, to date this has not been an issue in NSW.
There are several limitations to the model designed for this study. Firstly, the koala populations in this study area face multiple threats. This model considered the recognised top six local management actions to address those threats. We have not included climate-related threats, such as drought, fire and heatwaves (Lunney et al. 2012, 2020), and although some mitigation is possible at the local level, long-term management actions to address these broad and globally important issues require input at state, national, and international levels. We have addressed those threats that have been identified as most relevant when mitigation actions are implemented at a local or regional scale. We suggest that habitat protection and restoration are among the local solutions to mitigate climate change. Last, the model does not incorporate rare events, such as the catastrophic bushfires in 2019–2020.
Conclusion
This study has confirmed that multiple actions are required to manage threats to koalas in north-east NSW, and that the contribution of each action varies from place to place. We predict ongoing losses of the koala populations if no changes are undertaken, as well as various rates of recovery when different combinations of management actions are implemented effectively. The model outcomes are important for the region because they can be used by the local governments to help direct their coordinated efforts to maximise koala conservation benefits and guide strategic planning. The value and utility of local knowledge from those involved with the ongoing survival of the local koala populations is highlighted – their expertise can be incorporated through an elicitation process to facilitate targeted conservation outcomes. Bayesian networks can be used to structure this knowledge to help decision makers decide on immediate actions for management. The design of the model presented here also captures areas of uncertainty that could benefit from further research or monitoring, as well as targeted research to help refine the models and ensure they remain relevant and tuned to address local- and regional- scale issues. The design of the model described here could be easily adapted to different species or conservation contexts. It is easy to update as new evidence become available, making it valuable in adaptive management to address local to landscape-scale conservation problems.
Supplementary material
Supplementary material is available online.
Data availability
All relevant data are contained within the paper and supplementary material.
Conflicts of interest
The authors declare that they have no conflicts of interest.
Declaration of funding
This project was supported by ARC Linkage Project (LP160100486). JRR was supported by an ARC Future Fellowship (FT 200100096).
Acknowledgements
Ethics approval was granted by the University of Queensland (approval number 201.9001648). The authors are grateful to all the experts who volunteered to take part in the elicitation process and to William Goulding for his assistance in preparing materials for the initial consultation. Our modelling effort was facilitated by GeNIe Modeler, available free of charge for academic research and teaching use at https://www.bayesfusion.com/ (BayesFusion 2019).
References
Adams-Hosking, C, McAlpine, CA, Rhodes, JR, Moss, PT, and Grantham, HS (2015). Prioritizing regions to conserve a specialist folivore: considering probability of occurrence, food resources, and climate change. Conservation Letters 8, 162–170.| Prioritizing regions to conserve a specialist folivore: considering probability of occurrence, food resources, and climate change.Crossref | GoogleScholarGoogle Scholar |
Adams-Hosking, C, McBride, MF, Baxter, G, Burgman, M, de Villiers, D, Kavanagh, R, Lawler, I, Lunney, D, Melzer, A, Menkhorst, P, Molsher, R, Moore, BD, Phalen, D, Rhodes, JR, Todd, C, Whisson, D, and McAlpine, CA (2016). Use of expert knowledge to elicit population trends for the koala (Phascolarctos cinereus). Diversity and Distributions 22, 249–262.
| Use of expert knowledge to elicit population trends for the koala (Phascolarctos cinereus).Crossref | GoogleScholarGoogle Scholar |
Australian Bureau of Statistics (2018) ‘3218.0 – Regional population growth, Australia, 2017–18: population estimates by significant urban area, 2008 to 2018.’ (ABS: Canberra, ACT, Australia)
Bal, P, Tulloch, AIT, Chadès, I, Carwardine, J, McDonald-Madden, E, and Rhodes, JR (2018). Quantifying the value of monitoring species in multi-species, multi-threat systems. Methods in Ecology and Evolution 9, 1706–1717.
| Quantifying the value of monitoring species in multi-species, multi-threat systems.Crossref | GoogleScholarGoogle Scholar |
Ballina Shire Council (2017) ‘Ballina Shire Koala Management Strategy.’ (Ballina Shire Council: Ballina, NSW, Australia)
Bedford T, Cooke RM (2001) ‘Mathematical Tools for Probabilistic Risk Analysis.’ (Cambridge University Press: Cambridge, UK)
Beyer, HL, de Villiers, D, Loader, J, Robbins, A, Stigner, M, Forbes, N, and Hanger, J (2018). Management of multiple threats achieves meaningful koala conservation outcomes. Journal of Applied Ecology 55, 1966–1975.
| Management of multiple threats achieves meaningful koala conservation outcomes.Crossref | GoogleScholarGoogle Scholar |
Brook, BW, Sodhi, NS, and Bradshaw, CJA (2008). Synergies among extinction drivers under global change. Trends in Ecology & Evolution 23, 453–460.
| Synergies among extinction drivers under global change.Crossref | GoogleScholarGoogle Scholar |
Brown, G, McAlpine, C, Rhodes, J, Lunney, D, Goldingay, R, Fielding, K, Hetherington, S, Hopkins, M, Manning, C, Wood, M, Brace, A, and Vass, L (2018). Assessing the validity of crowdsourced wildlife observations for conservation using public participatory mapping methods. Biological Conservation 227, 141–151.
| Assessing the validity of crowdsourced wildlife observations for conservation using public participatory mapping methods.Crossref | GoogleScholarGoogle Scholar |
Burgman MA (2016) ‘Trusting Judgements How to Get the Best Out of Experts.’ (Cambridge University Press: Cambridge, UK)
Burgman, MA, McBride, M, Ashton, R, Speirs-Bridge, A, Flander, L, Wintle, B, Fidler, F, Rumpff, L, and Twardy, C (2011). Expert status and performance. PLoS One 6, e22998.
| Expert status and performance.Crossref | GoogleScholarGoogle Scholar |
Byron Shire Council (2015) ‘Byron Coast Comprehensive Koala Plan of Management.’ (Byron Shire Council: Mullumbimby, NSW, Australia)
Callaghan, J, McAlpine, C, Mitchell, D, Thompson, J, Bowen, M, Rhodes, J, de Jong, C, Domalewski, R, and Scott, A (2011). Ranking and mapping koala habitat quality for conservation planning on the basis of indirect evidence of tree-species use: a case study of Noosa Shire, south-eastern Queensland. Wildlife Research 38, 89–102.
| Ranking and mapping koala habitat quality for conservation planning on the basis of indirect evidence of tree-species use: a case study of Noosa Shire, south-eastern Queensland.Crossref | GoogleScholarGoogle Scholar |
DAWE (2021) Consultation on species listing eligibility and conservation actions Phascolarctos cinereus (koala). Department of Agriculture, Water and the Environment, Canberra, ACT, Australia.
DAWE (2022) National recovery plan for the koala: Phascolarctos cinereus (combined populations of Queensland, New South Wales and the Australian Capital Territory). Department of Agriculture, Water and the Environment, Canberra, ACT, Australia.
Dique, DS, Thompson, J, Preece, HJ, Penfold, GC, de Villiers, DL, and Leslie, RS (2003). Koala mortality on roads in south-east Queensland: the koala speed-zone trial. Wildlife Research 30, 419–426.
| Koala mortality on roads in south-east Queensland: the koala speed-zone trial.Crossref | GoogleScholarGoogle Scholar |
Fenton N, Neil M (2013) ‘Risk Assessment and Decision Analysis with Bayesian Networks.’ (CRC Press: New York, NY. USA)
Gonzalez-Astudillo, V, Allavena, R, McKinnon, A, Larkin, R, and Henning, J (2017). Decline causes of koalas in south east Queensland, Australia: a 17-year retrospective study of mortality and morbidity. Scientific Reports 7, 42587.
| Decline causes of koalas in south east Queensland, Australia: a 17-year retrospective study of mortality and morbidity.Crossref | GoogleScholarGoogle Scholar |
Harris, JM, and Goldingay, RL (2003). A community-based survey of the koala Phascolarctos cinereus in the Lismore region of north-eastern New South Wales. Australian Mammalogy 25, 155–167.
| A community-based survey of the koala Phascolarctos cinereus in the Lismore region of north-eastern New South Wales.Crossref | GoogleScholarGoogle Scholar |
Hemming, V, Burgman, MA, Hanea, AM, McBride, MF, and Wintle, BC (2018). A practical guide to structured expert elicitation using the IDEA protocol. Methods in Ecology and Evolution 9, 169–180.
| A practical guide to structured expert elicitation using the IDEA protocol.Crossref | GoogleScholarGoogle Scholar |
Johnson, CN, Balmford, A, Brook, BW, Buettel, JC, Galetti, M, Guangchun, L, and Wilmshurst, JM (2017). Biodiversity losses and conservation responses in the Anthropocene. Science 356, 270–275.
| Biodiversity losses and conservation responses in the Anthropocene.Crossref | GoogleScholarGoogle Scholar |
Kuhnert, PM, Martin, TG, and Griffiths, SP (2010). A guide to eliciting and using expert knowledge in Bayesian ecological models. Ecology Letters 13, 900–914.
| A guide to eliciting and using expert knowledge in Bayesian ecological models.Crossref | GoogleScholarGoogle Scholar |
Lismore City Council (2013) Comprehensive koala plan of management for south-east Lismore. Lismore City Council: Lismore, NSW, Australia.
Lunney D, Crowther MS, Wallis I, Foley WJ, Lemon J, Wheeler R, Madani G, Orscheg C, Griffith JE, Krockenberger M, Retamales M, E Stalenberg (2012) Koalas and climate change: a case study on the Liverpool Plains, north-west New South Wales. In ‘Wildlife and Climate Change: Towards Robust Conservation Strategies for Australian Fauna’. (Eds D Lunney, H Pat) (Royal Zoological Society of New South Wales: Sydney, NSW, Australia)
Lunney, D, Hope, B, and Shannon, I (2018). Protect our protected areas!: the value of protected areas for fauna research and conservation, a case study of New South Wales. Australian Zoologist 39, 296–344.
| Protect our protected areas!: the value of protected areas for fauna research and conservation, a case study of New South Wales.Crossref | GoogleScholarGoogle Scholar |
Lunney D, Matthews A, Moon C, Turbill J (2019a) Koala conservation and the role of private land – UPDATE of EMR feature. EMR Project Summaries. Available at https://site.emrprojectsummaries.org/2019/10/24/koala-conservation-and-the-role-of-private-land-update-of-emr-feature/
Lunney D, O’Neill L, Matthews A, Coburn D, Moon C (2019b) Beyond the 1990s, beyond Iluka – koalas and citizen science – UPDATE of EMR summary. EMR Project Summaries. Available at https://site.emrprojectsummaries.org/2019/10/24/beyond-the-1990s-beyond-iluka-koalas-and-citizen-science-update-of-emr-summary/
Lunney, D, Sonawane, I, Wheeler, R, Tasker, E, Ellis, M, Predavec, M, and Fleming, M (2020). An ecological reading of the history of the koala population of Warrumbungle National Park. Proceedings of the Linnean Society of New South Wales 141, S131–S154.
Lunney, D, Sonawane, I, Shannon, I, Hope, B, and Crowther, MS (2021). Combining cameras and citizen science to define the distribution and behaviour of dingoes and foxes in New South Wales. Australian Zoologist 41, 608–642.
| Combining cameras and citizen science to define the distribution and behaviour of dingoes and foxes in New South Wales.Crossref | GoogleScholarGoogle Scholar |
Lunney, D, Moon, C, Sonawane, I, Predavec, M, and Rhodes, JR (2022a). A 6-year study of mitigating koala roadkill during an upgrade of the Pacific Highway at Lindsay’s cutting, Coffs Harbour New South Wales. Australian Mammalogy , .
| A 6-year study of mitigating koala roadkill during an upgrade of the Pacific Highway at Lindsay’s cutting, Coffs Harbour New South Wales.Crossref | GoogleScholarGoogle Scholar |
Lunney, D, Predavec, M, Sonawane, I, Moon, C, and Rhodes, JR (2022b). Factors that drive koala roadkill: an analysis across multiple scales in New South Wales, Australia. Australian Mammalogy , .
| Factors that drive koala roadkill: an analysis across multiple scales in New South Wales, Australia.Crossref | GoogleScholarGoogle Scholar |
Mantyka-pringle, CS, Martin, TG, and Rhodes, JR (2012). Interactions between climate and habitat loss effects on biodiversity: a systematic review and meta-analysis. Global Change Biology 18, 1239–1252.
| Interactions between climate and habitat loss effects on biodiversity: a systematic review and meta-analysis.Crossref | GoogleScholarGoogle Scholar |
Marcot, BG, Holthausen, RS, Raphael, MG, Rowland, MM, and Wisdom, MJ (2001). Using Bayesian belief networks to evaluate fish and wildlife population viability under land management alternatives from an environmental impact statement. Forest Ecology and Management 153, 29–42.
| Using Bayesian belief networks to evaluate fish and wildlife population viability under land management alternatives from an environmental impact statement.Crossref | GoogleScholarGoogle Scholar |
Marcot, BG, Steventon, JD, Sutherland, GD, and McCann, RK (2006). Guidelines for developing and updating Bayesian belief networks applied to ecological modeling and conservation. Canadian Journal of Forest Research 36, 3063–3074.
| Guidelines for developing and updating Bayesian belief networks applied to ecological modeling and conservation.Crossref | GoogleScholarGoogle Scholar |
Masters, P, Duka, T, Berris, S, and Moss, G (2004). Koalas on Kangaroo Island: from introduction to pest status in less than a century. Wildlife Research 31, 267–272.
| Koalas on Kangaroo Island: from introduction to pest status in less than a century.Crossref | GoogleScholarGoogle Scholar |
Mata, L, Garrard, GE, Kutt, AS, Wintle, BC, Chee, YE, Backstrom, A, Bainbridge, B, Urlus, J, Brown, GW, Tolsma, AD, Yen, AL, New, TR, and Bekessy, SA (2017). Eliciting and integrating expert knowledge to assess the viability of the critically endangered golden sun-moth Synemon plana. Austral Ecology 42, 297–308.
| Eliciting and integrating expert knowledge to assess the viability of the critically endangered golden sun-moth Synemon plana.Crossref | GoogleScholarGoogle Scholar |
Mayfield, HJ, Brazill-Boast, J, Gorrod, E, Evans, MC, Auld, T, Rhodes, JR, and Maron, M (2020a). Estimating species response to management using an integrated process: a case study from New South Wales, Australia. Conservation Science and Practice 2, e269.
| Estimating species response to management using an integrated process: a case study from New South Wales, Australia.Crossref | GoogleScholarGoogle Scholar |
Mayfield, HJ, Smith, C, Gallagher, M, and Hockings, M (2020b). Considerations for selecting a machine learning technique for predicting deforestation. Environmental Modelling & Software 131, 104741.
| Considerations for selecting a machine learning technique for predicting deforestation.Crossref | GoogleScholarGoogle Scholar |
McAlpine, C, Lunney, D, Melzer, A, Menkhorst, P, Phillips, S, Phalen, D, Ellis, W, Foley, W, Baxter, G, de Villiers, D, Kavanagh, R, Adams-Hosking, C, Todd, C, Whisson, D, Molsher, R, Walter, M, Lawler, I, and Close, R (2015). Conserving koalas: a review of the contrasting regional trends, outlooks and policy challenges. Biological Conservation 192, 226–236.
| Conserving koalas: a review of the contrasting regional trends, outlooks and policy challenges.Crossref | GoogleScholarGoogle Scholar |
McCann, RK, Marcot, BG, and Ellis, R (2006). Bayesian belief networks: applications in ecology and natural resource management. Canadian Journal of Forest Research 36, 3053–3062.
| Bayesian belief networks: applications in ecology and natural resource management.Crossref | GoogleScholarGoogle Scholar |
Melzer, A, Carrick, F, Menkhorst, P, Lunney, D, and St. John, B (2000). Overview, critical assessment, and conservation implications of koala distribution and abundance. Conservation Biology 14, 619–628.
| Overview, critical assessment, and conservation implications of koala distribution and abundance.Crossref | GoogleScholarGoogle Scholar |
Pearl J (1988) ‘Probabilistic Reasoning in Intelligent Systems: Networks of Plausible Inference.’ (Morgan Kaufmann Publishers Inc.: Burlington, MA, USA)
Reed P, Lunney D, Walker P (1990) Survey of the koala Phascolarctos cinereus (Goldfuss) in New South Wales (1986-87), with an ecological interpretation of its distribution. In ‘Biology of the Koala’. (Eds AK Lee, KA Handasyde, GD Sanson) pp. 55–74. (Surrey Beatty and Sons: Chipping Norton, NSW, Australia)
Rhodes, JR, Ng, CF, de Villiers, DL, Preece, HJ, McAlpine, CA, and Possingham, HP (2011). Using integrated population modelling to quantify the implications of multiple threatening processes for a rapidly declining population. Biological Conservation 144, 1081–1088.
| Using integrated population modelling to quantify the implications of multiple threatening processes for a rapidly declining population.Crossref | GoogleScholarGoogle Scholar |
Rhodes, JR, Lunney, D, Callaghan, J, and McAlpine, CA (2014). A few large roads or many small ones? How to accommodate growth in vehicle numbers to minimise impacts on wildlife. PLoS One 9, e91093.
| A few large roads or many small ones? How to accommodate growth in vehicle numbers to minimise impacts on wildlife.Crossref | GoogleScholarGoogle Scholar |
Richards, R, Sanó, M, Roiko, A, Carter, RW, Bussey, M, Matthews, J, and Smith, TF (2013). Bayesian belief modeling of climate change impacts for informing regional adaptation options. Environmental Modelling & Software 44, 113–121.
| Bayesian belief modeling of climate change impacts for informing regional adaptation options.Crossref | GoogleScholarGoogle Scholar |
Santika, T, McAlpine, CA, Lunney, D, Wilson, KA, and Rhodes, JR (2015). Assessing spatio-temporal priorities for species’ recovery in broad-scale dynamic landscapes. Journal of Applied Ecology 52, 832–840.
| Assessing spatio-temporal priorities for species’ recovery in broad-scale dynamic landscapes.Crossref | GoogleScholarGoogle Scholar |
Scheele, BC, Legge, S, Armstrong, DP, Copley, P, Robinson, N, Southwell, D, Westgate, MJ, and Lindenmayer, DB (2018). How to improve threatened species management: an Australian perspective. Journal of Environmental Management 223, 668–675.
| How to improve threatened species management: an Australian perspective.Crossref | GoogleScholarGoogle Scholar |
Smith, CS, Howes, AL, Price, B, and McAlpine, CA (2007). Using a Bayesian belief network to predict suitable habitat of an endangered mammal – the Julia Creek dunnart (Sminthopsis douglasi). Biological Conservation 139, 333–347.
| Using a Bayesian belief network to predict suitable habitat of an endangered mammal – the Julia Creek dunnart (Sminthopsis douglasi).Crossref | GoogleScholarGoogle Scholar |
Tisdell, CA, Preece, HJ, Abdullah, S, and Beyer, HL (2017). Strategies to conserve the koala: cost-effectiveness considerations. Australasian Journal of Environmental Management 24, 302–318.
| Strategies to conserve the koala: cost-effectiveness considerations.Crossref | GoogleScholarGoogle Scholar |
Tweed Shire Council (2020) ‘Tweed Coast Comprehensive Koala Plan of Management.’ (Tweed Shire Council: Murwillumbah, NSW, Australia)
Whisson, DA, Dixon, V, Taylor, ML, and Melzer, A (2016). Failure to respond to food resource decline has catastrophic consequences for koalas in a high-density population in Southern Australia. PLoS One 11, e0144348.
| Failure to respond to food resource decline has catastrophic consequences for koalas in a high-density population in Southern Australia.Crossref | GoogleScholarGoogle Scholar |