External validation of the Health Care Homes hospital admission risk stratification tool in the Aboriginal Australian population of the Northern Territory
Laura Goddard

A Northern Territory Primary Health Network, Darwin, NT, Australia.
B National Centre for Epidemiology and Population Health, Australia National University, Canberra, ACT, Australia.
C Health Statistics and Informatics, Northern Territory Department of Health, Darwin, NT, Australia.
Australian Health Review 47(5) 521-534 https://doi.org/10.1071/AH23017
Submitted: 10 April 2022 Accepted: 7 August 2023 Published: 12 September 2023
Abstract
This study aimed to externally validate the Commonwealth’s Health Care Homes (HCH) algorithm for Aboriginal Australians living in the Northern Territory (NT).
A retrospective cohort study design using linked primary health care (PHC) and hospital data was used to analyse the performance of the HCH algorithm in predicting the risk of hospitalisation for the NT study population. The study population consisted of Aboriginal Australians residing in the NT who have visited a PHC clinic at one of the 54 NT Government clinics at least once between 1 January 2013 and 31 December 2017. Predictors of hospitalisation included demographics, patient observations, medications, diagnoses, pathology results and previous hospitalisation.
There were a total of 3256 (28.5%) emergency attendances or preventable hospitalisations during the study period. The HCH algorithm had an area under the receiver operating characteristic curve (AUC) of 0.58 for the NT remote Aboriginal population, compared with 0.66 in the Victorian cohort. A refitted model including ‘previous hospitalisation’ had an AUC of 0.72, demonstrating better discrimination than the HCH algorithm. Calibration was also improved in the refitted model, with an intercept of 0.00 and a slope of 1.00, compared with an intercept of 1.29 and a slope of 0.55 in the HCH algorithm.
The HCH algorithm performed poorly on the NT cohort compared with the Victorian cohort, due to differences in population demographics and burden of disease. A population-specific hospitalisation risk algorithm is required for the NT.
Keywords: chronic disease management, external validation, health policy, indigenous health, performance and evaluation, predictive risk model, primary health care, sensitivity analysis.
Background
Chronic conditions are now the predominant cause of illness, premature mortality and health system utilisation in Australia.1 Compared with the national average, the Northern Territory (NT) population has a higher burden of chronic disease. Of the NT population, 30% (74 546) are Aboriginal Australians, and about 75% of the 98 341 people living in remote or very remote areas of the NT are Aboriginal Australians.2 There is a particularly high burden of disease in remote and Aboriginal communities, much (59%) of which is avoidable, as well as high rates of hospitalisation.3,4 There is a correlation between remoteness, socioeconomic status, and health inequality,5 but ultimately health inequality is associated with the ongoing process of colonisation and systemic racism, which benefits non-Aboriginal people and results in poor health outcomes for Aboriginal Australians.6
Primary health care (PHC) has an essential role in the prevention, early detection and management of chronic disease and in reducing potentially preventable hospitalisations (PPH) and associated morbidity and mortality.7–10 Furthermore, there is a strong association between PHC and better health outcomes, lower costs and greater equity in health across population subgroups, including in remote communities in Australia.7,10–12
Between 2017 and 2021, the Australian Federal Government trialled an initiative, Health Care Homes (HCH). The aims were to improve health outcomes for patients with chronic diseases through enhanced access to holistic, coordinated PHC and to reduce healthcare costs through strengthening PHC and reducing PPH.13 Participating PHC practices identified patients with chronic health conditions using an algorithm developed by the Commonwealth Scientific and Industrial Research Organisation (CSIRO) and Precedence Health Care.14,15 The HCH model was developed using a primary care cohort linked to hospitalisation records in Victoria, Australia. The model excludes ‘previous hospitalisation’ (a strong predictor of the risk of hospitalisation).15
Differences in the Victorian and remote NT population demographics, burden of disease, and rates of hospitalisation suggested that the HCH algorithm required external validation prior to use in the NT. Moreover, the nationally mandated use of an algorithm developed in Victoria has the potential to introduce an algorithm bias that is not useful in addressing inequality or reducing hospitalisations for Aboriginal Australians.16
Using a retrospective cohort design, our primary objective was to externally validate the HCH algorithm by assessing whether the HCH algorithm’s performance was generalisable (whether the model performs satisfactorily in a separate population with different patient characteristics) to the remote NT Aboriginal population (external validation cohort). Our secondary objective was to demonstrate the value of including the predictor ‘previous hospitalisation’ in the model. For both objectives, predictive performance is assessed using measures of discrimination and calibration.
Methods
Study design and data sources
We used a retrospective cohort design to investigate the ability of the HCH algorithm to correctly and accurately classify individuals at risk of hospitalisation. Three datasets were used. Data were extracted from the primary care information system (54 remote PHC clinics) for the financial years 2011–2012 to 2017–2018 and from the inpatient activity and emergency attendance datasets (all six public hospitals in the NT) for the financial years 2012–2013 to 2019–2020 and 2010–2011 to 2019–2020, respectively. Data were linked using hospital registration number.
Outcome variable
The outcome of interest was whether there was a PPH or emergency attendance, whichever came first, within 1 year of the prediction date. PPH were defined according to the National Health Agreement indicator PI 18 – Selected PPH,17 using principal and secondary diagnoses and procedure codes of the International Classification of Diseases and Related Health Problems, 10th Revision, Australian Modification (ICD-10 AM).18
Predictors
Predictors were grouped into the categories of demographics, patient observations, medications, pathology results, and diagnoses (Supplementary Tables S1–S5) to replicate the CSIRO methods.15 In addition to CSIRO predictors, we included the number of previous hospitalisations (0–7), which was defined as an inpatient or emergency hospitalisation in the past year, calculated as within 365 days prior to the clinic visit date. Individuals who had missing data for the predictors ‘diagnosis families’ or ‘prescribed medication groups’ were excluded (n = 165 or ~1%), given that a risk probability would not be able to be calculated for these individuals. When data were missing for the predictors ‘body mass index’, ‘smoking status’ and ‘alcohol consumption’, these individuals were retained in the analysis but included missing values coded as ‘unknown’. Methods such as complete case analysis and multiple imputation that assume that data are missing at random were avoided because this may be incorrect (e.g. the missing value may be connected to the reason for the GP visit), and excluding individuals who are not representative of the population could introduce bias.
Study population and sample
NT residents aged 18 years or over who had attended an NT Government PHC clinic at least once during the study period (1 January 2013–31 December 2017) were included. As per the CSIRO methods, this cohort was filtered to include those who had at least 1 year of ‘look back’ and 1 year of ‘look forward’, so that every patient visited the clinic within a year of the prediction period (and was alive for the entire prediction period).15 To do this, two dates were calculated for each patient. The first date was 1 year ahead from the date of the patient’s first visit (minimum value 1 January 2014) and the second date was 1 year back from the date of the patient’s last visit (minimum value 31 December 2016). The patient cohort was then filtered to include only patients for whom the 1 year back date occurred on or after the 1 year ahead date. To avoid bias arising from consistently choosing the earliest or latest date as a patient’s prediction date, a random prediction date was chosen between the 1 year ahead and the 1 year back dates (Fig. 1).
We excluded patients whose sex was other than male or female and physiological observations and pathology records with inconsistent or incorrect units and values (Fig. 2).
Statistical analysis
Logistic regression using the CSIRO coefficients15 was performed to obtain probabilities of the HCH model on the NT cohort. Another regression was performed to refit the HCH model to the NT cohort, with and without the addition of the predictor ‘previous hospitalisation’. Coefficients, standard error, odds ratios (OR) and 95% confidence intervals (95% CI) for the refitted HCH model were obtained. For categorical predictors the reference category is the lowest level.
The models’ performance was assessed using measures of discrimination (area under the receiver operating characteristic (ROC) curve; AUC) and calibration (calibration in the large; calibration plot; and Hosmer–Lemeshow goodness of fit). An AUC of 0.5 indicates that the model performs no better than chance, a score of 0.7–0.8 indicates acceptable discrimination, and a score of >0.8 indicates good discrimination.19 Good calibration was defined as where the average predicted risk approximates the overall event rate (calibration in the large), where observed and predicted risks are equal (as represented by a diagonal line in calibration plots20), or where the P-value is >0.05 (Hosmer–Lemeshow goodness of fit).
Other performance measures included the sensitivity, specificity, positive predictive value and negative predicted value for defined risk group cut-offs (deciles of predicted risk of hospitalisation, with the highest group being most at risk and the lowest group being least at risk).
Data were analysed using Stata version 16 (Stata Corp, College Station, TX)21 and the study followed the Transparent Reporting of a multivariable prediction model for Individual Prognosis Or Diagnosis (TRIPOD) Statement (Supplementary material).22
Results
Of the eligible cohort (n = 17 790), a total of 6367 (35.7%) were excluded. Most (n = 6201; 97.4%) were excluded due to age being less than 18 years at cohort entry, one due to sex being recorded as neither male nor female, and a small number due to missing data (diagnosis families, n = 158; or prescribed medication group data, n = 7). The characteristics of the final cohort (n = 11 423) are listed in Table 1.
Characteristic | Not hospitalised (n = 8167) | Hospitalised (n = 3256) | All patients (n = 11 423) | |
---|---|---|---|---|
Demographics | ||||
Age (years), median (range) | 38 (18–96) | 38 (18–88) | 38 (18–96) | |
Sex, n (%) | ||||
Male | 3499 (42.8) | 1322 (40.6) | 4821 (42.2) | |
Female | 4668 (57.2) | 1934 (59.4) | 6602 (57.8) | |
Ethnicity, n (%) | ||||
Aboriginal | 7521 (92.1) | 3110 (95.5) | 10 631 (93.1) | |
Non-Aboriginal | 646 (7.9) | 146 (4.5) | 792 (6.9) | |
SEIFA IRSAD, n (%) | ||||
1 | 4247 (52.0) | 1954 (60.0) | 6201 (54.3) | |
2 | 2401 (29.4) | 731 (22.5) | 3132 (27.4) | |
3 | 1519 (18.6) | 571 (17.5) | 2090 (18.3) | |
Patient observations | ||||
Body mass index (kg/m2), n (%) | ||||
7–25 | 3482 (42.6) | 1226 (37.7) | 4708 (41.2) | |
25–30 | 2018 (24.7) | 884 (27.2) | 2902 (25.4) | |
30–35 | 1196 (14.6) | 533 (16.4) | 1729 (15.1) | |
35–40 | 548 (6.7) | 235 (7.2) | 783 (6.9) | |
40+ | 322 (4.0) | 134 (4.0) | 456 (4.0) | |
Unknown | 601 (7.4) | 244 (7.5) | 845 (7.4) | |
Smoking status, n (%) | ||||
Non-smoker | 1224 (15.0) | 475 (14.6) | 1699 (14.9) | |
Ex-smoker | 113 (1.3) | 51 (1.5) | 164 (1.4) | |
Smoker | 3197 (39.2) | 1291 (39.7) | 4488 (39.3) | |
Unknown | 3633 (44.5) | 1439 (44.2) | 5072 (44.4) | |
Alcohol consumption, n (%) | ||||
Non-drinker | 1319 (16.2) | 428 (13.1) | 1747 (15.3) | |
Drinker | 1938 (23.7) | 1058 (32.5) | 2996 (26.2) | |
Unknown | 4910 (60.1) | 1770 (54.4) | 6680 (58.5) | |
Medication, n (%) | ||||
Statins | 3105 (38.0) | 1510 (46.4) | 4615 (40.4) | |
Anticoagulants | 443 (5.4) | 370 (11.4) | 813 (7.1) | |
Antidepressants | 680 (8.3) | 406 (12.5) | 1086 (9.5) | |
Antipsychotics | 557 (6.8) | 321 (9.9) | 878 (7.7) | |
Anti-inflammatories | 5805 (71.1) | 2605 (80.0) | 8410 (73.6) | |
Steroids | 1862 (22.8) | 1026 (31.5) | 2888 (25.3) | |
Prescribed medication groups, n (%) | ||||
0 | 1207 (14.8) | 269 (8.3) | 1476 (12.9) | |
1 | 3307 (40.5) | 1067 (32.8) | 4374 (38.3) | |
2 | 2244 (27.5) | 952 (29.2) | 3196 (28.0) | |
3 | 1035 (12.7) | 666 (20.5) | 1701 (14.9) | |
4 | 320 (3.9) | 245 (7.5) | 565 (5.0) | |
5 | 52 (0.6) | 53 (1.6) | 105 (0.9) | |
6 | 2 (0.0) | 4 (0.1) | 6 (0.1) | |
Disease group, n (%) | ||||
Respiratory | 1058 (13.0) | 603 (18.5) | 1661 (14.5) | |
Atrial fibrillation | 132 (1.6) | 103 (3.2) | 235 (2.1) | |
Cardiovascular | 1059 (13.0) | 716 (22.0) | 1775 (15.5) | |
Osteoarthritis | 70 (0.9) | 29 (0.9) | 99 (0.9) | |
Osteoporosis | 51 (0.6) | 34 (1.0) | 85 (0.7) | |
Rheumatoid arthritis | 41 (0.5) | 34 (1.0) | 75 (0.7) | |
Mental health | 457 (5.6) | 221 (6.8) | 678 (5.9) | |
Cancer | 216 (2.6) | 111 (3.4) | 327 (2.9) | |
Digestive | 153 (1.9) | 165 (5.1) | 318 (2.8) | |
Hypertension | 1903 (23.3) | 891 (27.4) | 2794 (24.5) | |
Bloodfats | 1046 (12.8) | 548 (16.8) | 1594 (14.0) | |
Chronic kidney disease | 1139 (14.0) | 589 (18.1) | 1728 (15.1) | |
Diabetes (type 1) | 21 (0.3) | 16 (0.5) | 37 (0.3) | |
Diabetes (type 2) | 2070 (25.4) | 1127 (34.6) | 3197 (28.0) | |
Venous thromboembolism | 56 (0.7) | 35 (1.1) | 91 (0.8) | |
Other conditions | 98 (1.2) | 65 (2.0) | 163 (1.4) | |
Number of diagnosis families, n (%) | ||||
0 | 3539 (43.3) | 1061 (32.6) | 4600 (40.3) | |
1 | 1998 (24.5) | 754 (23.2) | 2752 (24.1) | |
2 | 1225 (15.0) | 553 (17.0) | 1778 (15.6) | |
3 | 720 (8.8) | 364 (11.2) | 1084 (9.5) | |
4 | 414 (5.1) | 280 (8.6) | 694 (6.1) | |
5 | 170 (2.1) | 130 (4.0) | 300 (2.6) | |
6+ | 101 (1.2) | 114 (3.4) | 215 (1.8) |
IRSAD, Index of Relative Socioeconomic Advantage and Disadvantage; SEIFA, Socioeconomic Indexes for Areas.
The proportion of the cohort who had either a PPH or emergency hospitalisation within 1 year of PHC clinic attendance (event rate) is 28.5% (Table 2).
Type of hospitalisation | Count | Percentage of total cohort | |
---|---|---|---|
Total hospitalisationsA (any duration) | 10 233 | 89.6 | |
Emergency attendance within 1 year of PHC visit | 2812 | 24.6 | |
PPH within 1 year of PHC visit | 444 | 3.9 | |
Total patients | 11 423 | 100.0 |
PHC, primary health care; PPH, potentially preventable hospitalisation.
AIncludes non-PPH hospitalisations, PPH hospitalisations and emergency attendances.
Results from the logistic regression using the HCH coefficients and the refitted model are listed in Table 3. In contrast to the HCH model, in the refitted model being female (OR 0.92, 95% CI: 0.81–1.05) and non-Aboriginal were protective (OR 0.61, 95% CI: 0.49–0.77), although the association with sex was not significant. Lower body mass index was associated with increased hospitalisation risk, smoking was not a significant risk for hospitalisation (OR 1.04, 95% CI: 0.90–1.21) and alcohol was significant (OR 1.63, 95% CI: 1.39–1.90). Similar associations in both models were observed for medications and pathology biomarkers. Hospitalisation risk associated with specific diseases varied between the two populations, with increasing comorbidity a consistent risk in the two models. Previous hospitalisation was a significant predictor of future hospitalisation in the NT model, with a higher number of previous hospitalisations associated with an increased risk of hospitalisation.
Variable | Refitted HCH model | Khanna et al. (2019)15 | |||||||
---|---|---|---|---|---|---|---|---|---|
Coefficient | Std error | OR | 95% CI | Coefficient | Std error | OR | 95% CI | ||
Intercept | −2.6172 | 0.437769 | 0.073 | (0.031, 0.172) | −2.7551480 | ||||
Age | 0.039483 | 0.030471 | 1.040 | (0.980, 1.104) | −0.0379528 | 0.00663 | |||
Age2 | −0.00103 | 0.000666 | 0.999 | (0.998, 1.000) | 0.000615945 | 0.00014 | |||
Age3 | 0.000000783 | 0.000000451 | 1.000 | (1.000, 1.000) | −0.00000104739 | 0.0000000878 | |||
Sex | |||||||||
Female | −0.08334 | 0.067767 | 0.920 | (0.806, 1.051) | 0.2216384 | 0.01588 | 1.248 | (1.210, 1.288) | |
Ethnicity | |||||||||
Non-indigenous | −0.48726 | 0.118218 | 0.614 | (0.487, 0.774) | 0.4033629 | 0.07675 | 1.497 | (1.288, 1.740) | |
Unknown | −0.0662497 | 0.0166 | 0.936 | (0.906, 0.967) | |||||
SEIFA IRSAD | |||||||||
2 | −0.41181 | 0.056604 | 0.662 | (0.593, 0.740) | −0.0123849 | 0.02505 | 0.988 | (0.940, 1.037) | |
3 | −0.16168 | 0.064661 | 0.851 | (0.749, 0.966) | −0.0742180 | 0.0593 | 0.928 | (0.827, 1.043) | |
Body mass index (kg/m2) | |||||||||
25–30 | 0.155751 | 0.058651 | 1.169 | (1.042, 1.311) | 0.032942 | 0.02884 | 1.033 | (0.977, 1.094) | |
30–35 | 0.110089 | 0.070923 | 1.116 | (0.971, 1.283) | 0.193983 | 0.03225 | 1.214 | (1.140, 1.293) | |
35–40 | 0.030564 | 0.095866 | 1.031 | (0.854, 1.244) | 0.271227 | 0.042 | 1.312 | (1.208, 1.424) | |
40+ | −0.07546 | 0.122108 | 0.927 | (0.730, 1.1780) | 0.464362 | 0.04627 | 1.591 | (1.453, 1.742) | |
Unknown | −0.00029 | 0.094019 | 1.000 | (0.831, 1.202) | 0.139507 | 0.02198 | 1.15 | (1.101, 1.200) | |
Smoking status | |||||||||
Ex-smoker | 0.178644 | 0.19641 | 1.196 | (0.814, 1.757) | 0.196932 | 0.01761 | 1.218 | (1.176, 1.260) | |
Smoker | 0.038429 | 0.075633 | 1.039 | (0.896, 1.205) | 0.405721 | 0.01636 | 1.5 | (1.453, 1.549) | |
Unknown | 0.29693 | 0.08513 | 1.346 | (1.139, 1.590) | 0.199347 | 0.02421 | 1.221 | (1.164, 1.280) | |
Alcohol consumption | |||||||||
Any alcohol | 0.4860986 | 0.0801081 | 1.626 | (1.390, 1.902) | −0.2853232 | 0.03423 | 0.752 | (0.703, 0.804) | |
Unknown | 0.0619066 | 0.0829535 | 1.064 | (0.904, 1.252) | −0.2301394 | 0.04245 | 0.794 | (0.731, 0.863) | |
Medications | |||||||||
Statins | −0.1024 | 0.069542 | 0.903 | (0.788, 1.034) | −0.0152881 | 0.02606 | 0.985 | (0.936, 1.036) | |
Anticoagulants | 0.412162 | 0.094573 | 1.510 | (1.255, 1.818) | 0.2886091 | 0.04044 | 1.335 | (1.233, 1.445) | |
Antidepressants | 0.316949 | 0.082127 | 1.373 | (1.169, 1.613) | 0.2025163 | 0.0302 | 1.224 | (1.154, 1.299) | |
Antipsychotics | 0.206396 | 0.087647 | 1.229 | (1.035, 1.460) | 0.3923084 | 0.03527 | 1.48 | (1.382, 1.586) | |
Anti-inflammatories | 0.3866 | 0.055756 | 1.472 | (1.32, 1.642) | 0.1301034 | 0.01442 | 1.139 | (1.107, 1.172) | |
Steroids | 0.235552 | 0.059202 | 1.266 | (1.127, 1.421) | 0.148835 | 0.018 | 1.16 | (1.120, 1.202) | |
Number of diagnosis families | |||||||||
No. of diagnosis families | 0.289299 | 0.116737 | 1.335 | (1.062, 1.679) | 0.3369661 | 0.03298 | |||
No. of diagnosis families2 | −0.01688 | 0.019081 | 0.983 | (0.947, 1.021) | −0.0397663 | 0.00653 | |||
No. of diagnosis families3 | 0.00026 | 0.001629 | 1.000 | (0.997, 1.003) | 0.0019304 | 0.00057 | |||
Disease groups | |||||||||
Respiratory | 0.045888 | 0.1462 | 1.047 | (0.786, 1.394) | −0.0715037 | 0.04264 | 0.931 | (0.856, 1.012) | |
Atrial fibrillation | −0.13518 | 0.178059 | 0.874 | (0.616, 1.238) | 0.2234789 | 0.05779 | 1.25 | (1.117, 1.400) | |
Cardiovascular | 0.162469 | 0.138994 | 1.176 | (0.896, 1.545) | 0.4764327 | 0.04877 | 1.61 | (1.464, 1.772) | |
Osteoarthritis | −0.4313 | 0.268242 | 0.650 | (0.384, 1.099) | −0.2060183 | 0.03764 | 0.814 | (0.756, 0.876) | |
Osteoporosis | 0.652895 | 0.498697 | 1.921 | (0.723, 5.106) | 0.0595034 | 0.08957 | 1.061 | (0.890, 1.265) | |
Rheumatoid arthritis | 0.175776 | 0.274371 | 1.192 | (0.696, 2.041) | 0.1149149 | 0.07502 | 1.122 | (0.968, 1.299) | |
Mental health | −0.32126 | 0.179014 | 0.725 | (0.511, 1.030) | 0.0686955 | 0.04483 | 1.071 | (0.981, 1.169) | |
Cancer | −0.1187 | 0.160949 | 0.888 | (0.648, 1.217) | 0.0600825 | 0.04073 | 1.062 | (0.980, 1.150) | |
Digestive | 0.310781 | 0.160978 | 1.364 | (0.995, 1.871) | 0.1796635 | 0.03992 | 1.197 | (1.107, 1.294) | |
Hypertension | −0.39754 | 0.12948 | 0.672 | (0.521, 0.866) | −0.1591489 | 0.041 | 0.853 | (0.787, 0.924) | |
Bloodfats | −0.07763 | 0.122827 | 0.925 | (0.727, 1.177) | −0.3726723 | 0.03771 | 0.689 | (0.640, 0.742) | |
Chronic kidney disease | −0.3289 | 0.13826 | 0.720 | (0.549, 0.944) | 0.0268266 | 0.085 | 1.027 | (0.870, 1.213) | |
Diabetes (type 1) | 0.341516 | 0.528982 | 1.407 | (0.499, 3.968) | 0.5844975 | 0.1063 | 1.794 | (1.457, 2.210) | |
Diabetes (type 2) | −0.1227 | 0.133226 | 0.885 | (0.681, 1.148) | 0.1332004 | 0.04722 | 1.142 | (1.041, 1.253) | |
Venous thromboembolism | −0.37544 | 0.272125 | 0.687 | (0.403, 1.171) | 0.3623621 | 0.06477 | 1.437 | (1.265, 1.631) | |
Other conditions | 0.725017 | 0.24856 | 2.065 | (1.269, 3.361) | 0.5157983 | 0.08211 | 1.675 | (1.426, 1.967) | |
Haemoglobin (g/L) | |||||||||
Medium | 0.157894 | 0.064586 | 1.171 | (1.032, 1.329) | 0.4416708 | 0.06741 | 1.555 | (1.363, 1.775) | |
High | 0.161708 | 0.134634 | 1.176 | (0.903, 1.530) | 0.1546069 | 0.02639 | 1.167 | (1.108, 1.229) | |
No test history | 0.288812 | 0.20288 | 1.335 | (0.897, 1.987) | 0.0450222 | 0.17061 | 1.046 | (0.749, 1.461) | |
Platelets (per L) | |||||||||
High | 0.28582 | 0.175048 | 1.331 | (0.944, 1.876) | 0.1398411 | 0.0895 | 1.15 | (0.965, 1.371) | |
No test history | −0.69748 | 0.178542 | 0.498 | (0.351, 0.706) | −0.0147097 | 0.17048 | 0.985 | (0.706, 1.376) | |
Alanine aminotransferase (u/L) | |||||||||
Medium | −0.06838 | 0.140923 | 0.934 | (0.709, 1.231) | −0.0619518 | 0.09056 | 0.94 | (0.787, 1.122) | |
High | −0.21628 | 0.212515 | 0.806 | (0.531, 1.222) | 0.0550267 | 0.06484 | 1.057 | (0.930, 1.200) | |
No test history | −0.44963 | 1.049026 | 0.638 | (0.082, 4.985) | −0.2940664 | 0.24315 | 0.745 | (0.463, 1.200) | |
γ-Glutamyl transferase (u/L) | |||||||||
Medium | 0.091626 | 0.090158 | 1.096 | (0.918, 1.308) | 0.2209109 | 0.04884 | 1.247 | (1.133, 1.372) | |
High | 0.227167 | 0.101757 | 1.255 | (1.028, 1.532) | 0.1261477 | 0.04895 | 1.134 | (1.031, 1.249) | |
No test history | 0.028084 | 0.79902 | 1.028 | (0.215, 4.924) | 0.1979201 | 0.21908 | 1.219 | (0.793, 1.873) | |
Haemoglobin A1C (mmol/mol) | |||||||||
Medium | 0.00571 | 0.118297 | 1.006 | (0.798, 1.268) | 0.1746667 | 0.04153 | 1.191 | (1.098, 1.292) | |
High | −0.02717 | 0.087021 | 0.973 | (0.821, 1.154) | 0.1720256 | 0.05061 | 1.188 | (1.076, 1.312) | |
No test history | −0.13412 | 0.091843 | 0.874 | (0.730, 1.047) | −0.0461721 | 0.01882 | 0.955 | (0.920, 0.991) | |
Total cholesterol (mmol/L) | |||||||||
Medium | 0.11175 | 0.157397 | 1.118 | (0.821, 1.522) | 0.0453054 | 0.07726 | 1.046 | (0.899, 1.217) | |
High | −0.18146 | 0.284733 | 0.834 | (0.477, 1.457) | −0.0363534 | 0.0464 | 0.964 | (0.880, 1.056) | |
No test history | 11.57308 | 451.64 | 106200.1 | (0) | 0.1749709 | 0.153 | 1.191 | (0.883, 1.608) | |
Albumin creatinine ratio (mg/mmol) | |||||||||
Medium | 0.088848 | 0.058852 | 1.093 | (0.974, 1.227) | 0.304275 | 0.08592 | 1.356 | (1.146, 1.604) | |
High | 0.165975 | 0.080711 | 1.181 | (1.008, 1.383) | 0.1151562 | 0.05232 | 1.122 | (1.013, 1.243) | |
No test history | −0.16111 | 0.166119 | 0.851 | (0.615, 1.179) | 0.1059512 | 0.02951 | 1.112 | (1.049, 1.178) | |
Low-density lipoprotein (mmol/L) | |||||||||
Medium | −0.10093 | 0.055513 | 0.904 | (0.811, 1.008) | −0.0585925 | 0.03821 | 0.943 | (0.875, 1.016) | |
High | −0.30308 | 0.138265 | 0.739 | (0.563, 0.968) | −0.0539600 | 0.02062 | 0.947 | (0.910, 0.987) | |
No test history | −0.29366 | 0.411453 | 0.746 | (0.333, 1.670) | 0.1556545 | 0.05693 | 1.168 | (1.045, 1.306) | |
Estimated glomerular filtration rate (mL/min) | |||||||||
Medium | 0.555204 | 0.160764 | 1.742 | (1.271, 2.388) | 0.0891486 | 0.07968 | 1.093 | (0.935, 1.278) | |
High | 0.67618 | 0.192986 | 1.966 | (1.347, 2.870) | 0.0950474 | 0.05205 | 1.1 | (0.993, 1.218) | |
No test history | 0.141722 | 0.056636 | 1.152 | (1.031, 1.288) | 0.045979 | 0.02027 | 1.047 | (1.006, 1.089) | |
Blood pressure (mmHg) | |||||||||
Medium | −0.15697 | 0.106768 | 0.855 | (0.693, 1.054) | 0.2946162 | 0.10197 | 1.343 | (1.099, 1.640) | |
High | 0.415422 | 0.22942 | 1.515 | (0.966, 2.375) | 0.1839175 | 0.03706 | 1.202 | (1.118, 1.292) | |
No test history | 0.034025 | 0.059946 | 1.035 | (0.920, 1.164) | 0.0112105 | 0.01543 | 1.011 | (0.981, 1.042) | |
Bilirubin (μmol/L) | |||||||||
Medium or high | 0.406445 | 0.603774 | 1.501 | (0.460, 4.903) | 0.1803894 | 0.15242 | 1.198 | (0.888, 1.615) | |
No test history | 0.317444 | 0.825361 | 1.374 | (0.272, 6.925) | 0.0784321 | 0.21276 | 1.082 | (0.713, 1.641) | |
Creatinine (μmol/L) | |||||||||
Medium or high | 0.349408 | 0.233869 | 1.418 | (0.897, 2.243) | 1.134946 | 0.15125 | 3.111 | (2.313, 4.185) | |
No test history | −0.26019 | 0.360533 | 0.771 | (0.380, 1.563) | −0.1704331 | 0.02109 | 0.843 | (0.809, 0.879) | |
Triglycerides (mmol/L) | |||||||||
Medium or high | 0.085027 | 0.091236 | 1.089 | (0.910, 1.302) | 0.1234874 | 0.05878 | 1.131 | (1.008, 1.270) | |
No test history | −10.383 | 451.6401 | 0.000 | (0) | −0.0963018 | 0.1607 | 0.908 | (0.663, 1.244) | |
Sex–diagnosis group interactions terms | |||||||||
Sex × Respiratory | −0.14676 | 0.128032 | 0.864 | (0.672, 1.110) | 0.0570566 | 0.04067 | 1.059 | (0.978, 1.147) | |
Sex × Cardiovascular | 0.026577 | 0.122824 | 1.027 | (0.807, 1.306) | −0.2108839 | 0.05868 | 0.81 | (0.722, 0.909) | |
Sex × Osteoporosis | −0.87107 | 0.571341 | 0.419 | (0.137, 1.282) | −0.2475655 | 0.09695 | 0.781 | (0.646, 0.944) | |
Sex × Mental health | −0.07891 | 0.18962 | 0.924 | (0.637, 1.340) | −0.0643675 | 0.03514 | 0.938 | (0.875, 1.005) | |
Sex × Hypertension | 0.092476 | 0.116402 | 1.097 | (0.873, 1.378) | −0.0890935 | 0.04019 | 0.915 | (0.845, 0.990) | |
Sex × Chronic Kidney disease | 0.05746 | 0.132591 | 1.059 | (0.817, 1.373) | −0.1122160 | 0.10963 | 0.894 | (0.721, 1.108) | |
Sex × Diabetes (type 1) | −0.3869 | 0.77123 | 0.679 | (0.150, 3.079) | 0.2492323 | 0.14198 | 1.283 | (0.971, 1.695) | |
Sex × Diabetes (type 2) | 0.073414 | 0.10995 | 1.076 | (0.868, 1.335) | −0.0002784 | 0.05227 | 1 | (0.902, 1.108) | |
Sex × Other Conditions | −0.54596 | 0.360134 | 0.579 | (0.286, 1.173) | −0.1721620 | 0.10383 | 0.842 | (0.687, 1.032) | |
No. of previous hospitalisations | |||||||||
1 | 0.547107 | 0.058273 | 1.728 | (1.542, 1.937) | |||||
2 | 0.865274 | 0.078348 | 2.376 | (2.037, 2.770) | |||||
3 | 1.318836 | 0.105013 | 3.739 | (3.044, 4.594) | |||||
4 | 1.475981 | 0.13881 | 4.375 | (3.333, 5.743) | |||||
5 | 1.620475 | 0.179878 | 5.055 | (3.553, 7.192) | |||||
6 | 2.11436 | 0.246991 | 8.284 | (5.105, 13.443) | |||||
7 | 2.472888 | 0.17563 | 11.857 | (8.404, 16.729) |
HCH, Health Care Homes; IRSAD, Index of Relative Socioeconomic Advantage and Disadvantage; SEIFA, Socioeconomic Indexes for Areas.
The AUC for the HCH algorithm applied to the NT cohort was 0.58, indicating poor discrimination (Fig. 3). The AUC of the refitted HCH algorithm using coefficients from the NT cohort was 0.68 (Supplementary Fig. S1), demonstrating improved discrimination between those at risk of hospitalisation and those not at risk of hospitalisation. Including the variable for ‘previous hospitalisation’ in the modified model resulted in an AUC of 0.72, indicating that the model had acceptable discrimination (Fig. 3). Performance measures corresponding to the ROC curve for the modified model are given in Table 4. Higher sensitivity was observed with increasing decile of predicted risk of hospitalisation, while lower specificity was observed with increasing decile of predicted risk.
Receiver operating characteristic curves for the HCH model (left) and refitted model with history of previous hospitalisation (right).
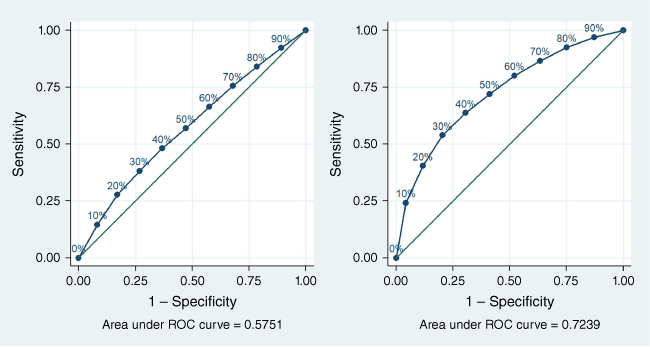
Characteristic | Decile of predicted riskA | |||||||||
---|---|---|---|---|---|---|---|---|---|---|
10% | 20% | 30% | 40% | 50% | 60% | 70% | 80% | 90% | ||
True positives | 788 | 1318 | 1753 | 2071 | 2344 | 2606 | 2819 | 3011 | 3156 | |
False positives | 356 | 967 | 1675 | 2499 | 3369 | 4249 | 5178 | 6128 | 7125 | |
True negatives | 7811 | 7200 | 6492 | 5668 | 4798 | 3918 | 2989 | 2039 | 1042 | |
False negatives | 2468 | 1938 | 1503 | 1185 | 912 | 650 | 437 | 245 | 100 | |
Sensitivity | 24.2 | 40.5 | 53.8 | 63.6 | 72.0 | 80.0 | 86.6 | 92.5 | 96.9 | |
False-positive rate | 4.3 | 11.8 | 20.5 | 30.6 | 41.3 | 52.0 | 63.4 | 75.0 | 87.2 | |
Specificity | 95.7 | 88.2 | 79.5 | 69.4 | 58.8 | 48.0 | 36.6 | 25.0 | 12.8 | |
False-negative rate | 75.8 | 59.5 | 46.2 | 36.4 | 28.0 | 20.0 | 13.4 | 7.5 | 3.1 | |
Positive predictive value | 68.9 | 57.7 | 51.1 | 45.3 | 41.0 | 38.0 | 35.3 | 33.0 | 30.7 | |
Negative predictive value | 76.0 | 78.8 | 81.2 | 82.7 | 84.0 | 85.8 | 87.2 | 89.3 | 91.2 | |
Accuracy (proportion correctly classified) | 75.3 | 74.6 | 72.2 | 67.8 | 62.5 | 57.1 | 50.8 | 44.2 | 36.8 |
The sensitivity and specificity describe the distribution of classifications given the true outcome, while positive predictive value and negative predictive value give the probabilities of the outcomes given the classification.
ADeciles of predicted risk of hospitalisation, with the highest group being most at risk and the lowest group being least at risk.
Calibration-in-the-large of the HCH model applied to the NT cohort was poor, with a mean predicted risk of 10.4% and an event rate of 28.5% (Table 2), while calibration-in-the-large of the modified model was very good, with the mean predicted risk being 28.5%, the same as the event rate. Fig. 4 shows calibration plots for risk groups defined by deciles of predicted risk, for both the HCH model and the modified model with history of previous hospitalisation. The predicted risks better corresponded to observed proportions in the modified model, for which the intercept was 0.00 and the slope was 1.00. The Hosmer–Lemeshow goodness of fit test showed poor calibration in the HCH model when applied to the NT cohort with a chi-squared (d.f. = 8) of 4746.92, much higher than the chi-squared threshold of 15.51 (P < 0.0001). This is compared with the NT model with much improved calibration (Hosmer–Lemeshow goodness of fit chi-squared of 9.78, much smaller than the threshold of 15.51, P = 0.281).
Discussion
We demonstrate that the HCH model, when applied to the remote NT Aboriginal population, has limited external validity (generalisability). The HCH algorithm was a poor predictor of hospitalisation in the remote Aboriginal population in the NT (AUC = 0.58) and was poorly calibrated (mean predicted risk of 10.4% compared with event rate of 28.5%; predicted risks did not correspond well to the observed proportions), illustrating the importance of externally validating predictive risk models in other populations prior to their use in clinical practice.
Clear differences in cohort characteristics contributed to the variation seen in the HCH algorithm’s performance. Aboriginal Australians accounted for 0.4% of the Victorian cohort, compared with 93.1% of the remote NT cohort. The Victorian cohort was much more advantaged, with only 8.8% in IRSAD (Index of Relative Socioeconomic Advantage and Disadvantage) decile 1, while the NT cohort had 54.2% in IRSAD decile 1 and the entire cohort in deciles 1–3. Smoking prevalence was twofold greater and alcohol consumption prevalence was sixfold greater in the NT cohort. Polypharmacy and comorbidity were greater in the NT cohort, with a higher prevalence of cardiovascular disease, hypertension, dyslipidaemia, chronic kidney disease and type 2 diabetes (Table 3). Although a measure of rural and remoteness was not included in the final HCH model and we were therefore unable to quantify the difference in remoteness between the NT and Victorian cohorts, the NT cohort is more remote overall.
Our results also demonstrate the value of including history of previous hospitalisation in the model, which is known to be an important predictor of future hospitalisation.14 The AUC of the modified model, inclusive of prior hospitalisation, was 0.72, demonstrating better discrimination than the HCH model applied in the NT (0.58) and Victorian cohorts (0.66). Calibration was also improved by adding history of previous hospitalisation to the model. The mean predicted risk was equivalent to the event rate of 28.5% and the predicted risks corresponded well to the observed proportions. Given these findings and that history of previous hospitalisation is not routinely available in general practice systems, an implication of our work is that the development of hospitalisation risk algorithms benefits from routine linkage of hospital and PHC data.
Limitations of this study include the differences in the study periods between the HCH model (2011–2016) and our study (2013–2017), minor differences in the definition of predictor variables, and differences in the proportion of missing data between the NT cohort and the Victorian cohort (body mass index: 7%, 69%; smoking status: 44%, 11%; alcohol consumption: 60%, 3%; ethnicity: 0%, 21%; and socioeconomic status: 0%, 0.2%, respectively).
Differences were minimised through replicating the documented CSIRO methods and by verifying our approach through direct communication with the CSIRO study authors. While we did not perform internal validation checks after adding the additional variable ‘previous hospitalisation’ to the HCH model, it was not the aim of this study to develop a completely new model, but rather to demonstrate the value of including data on previous hospitalisation. A further limitation is the historical nature of this risk algorithm research. Further research is required to utilise real-time linked data and machine learning techniques to make hospitalisation risk algorithms operationally relevant to clinicians and maximise benefits for patients. We also suggest that the inclusion of additional data (i.e. from Aboriginal Community Controlled Health Services) and predictors specific to Aboriginal PHC would further strengthen the predictive performance of the model.
Despite these limitations, the potential contribution of hospitalisation risk algorithms to the remote Aboriginal population of the NT is commensurably greater when compared with other Australian states and territories. Consistent with previous publications,4 in the NT cohort the rate of PPH or emergency hospitalisations within 1 year of PHC clinic attendance was 28.5%, fourfold higher than the rate in the Victorian cohort.15 In the NT context (high rates of chronic disease3 and PPH,23 limited number of health providers, high population enrolment) predictive risk algorithms have considerable potential to help focus preventive care efforts and allocation of scarce healthcare resources. Strengthening hospitalisation risk prediction will help target care and deliver on the objectives of the HCH initiative: improved patient and population outcomes,24 reduced PPH,25 decreased healthcare costs26 and improved experiences of care for patients and clinicians.
Conclusion
To maximise the utility and impartiality of hospitalisation risk prediction, algorithms require external validation and refinement on the populations to which they will be applied. Our work shows poor performance of the HCH model in the NT cohort and suggests that including history of previous hospitalisation through linkage of PHC and hospital data could improve model discrimination and calibration. In the high-need, resource-constrained setting of the remote NT, there is significant need and potential to improve health outcomes for the population while reducing demand for expensive evacuations and hospital care.
Acknowledgements
This project was undertaken as part of the Master of Philosophy in Applied Epidemiology (MAE) at Australian National University and forms part of the MAE thesis completed in 2021.
References
2 Australian Bureau of Statistics. Estimated resident Aboriginal and Torres Strait Islander and Non-Indigenous population, States and Territories, Remoteness Areas - 30 June 2016. Canberra: Australian Bureau of Statistics; 2016. Available at https://www.abs.gov.au/AUSSTATS/abs@.nsf/DetailsPage/3238.0.55.001June%202016?OpenDocument
3 Vos T, Barker B, Begg S, et al. Burden of disease and injury in Aboriginal and Torres Strait Islander Peoples: the Indigenous health gap. Int J Epidemiol 2009; 38(2): 470-477.
| Crossref | Google Scholar |
5 Zhao Y, You J, Wright J, et al. Health inequity in the Northern Territory, Australia. Int J Equity Health 2013; 12(1): 79-87.
| Crossref | Google Scholar |
6 Griffiths K, Coleman C, Lee V, et al. How colonisation determines social justice and Indigenous health: a review of the literature. J Popul Res 2016; 33(1): 9-30.
| Crossref | Google Scholar |
7 Zhao Y, Thomas SL, Guthridge SL, et al. Better health outcomes at lower costs: the benefits of primary care utilisation for chronic disease management in remote Indigenous communities in Australia’s Northern Territory. BMC Health Serv Res 2014; 14(1): 463-472.
| Crossref | Google Scholar |
8 Gador-Whyte AP, Wakerman J, Campbell D, et al. Cost of best-practice primary care management of chronic disease in a remote Aboriginal community. Med J Aust 2014; 200(11): 663-666.
| Crossref | Google Scholar |
9 Wakerman J, Sparrow L, Thomas SL, et al. Equitable resourcing of primary health care in remote communities in Australia’s Northern Territory: a pilot study. BMC Fam Pract 2017; 18: 75.
| Crossref | Google Scholar |
10 Zhao Y, Wright J, Guthridge S, et al. The relationship between number of primary health care visits and hospitalisations: evidence from linked clinic and hospital data for remote Indigenous Australians. BMC Health Serv Res 2013; 13: 466.
| Crossref | Google Scholar |
11 Thomas SL, Zhao Y, Guthridge SL, et al. The cost-effectiveness of primary care for Indigenous Australians with diabetes living in remote Northern Territory communities. Med J Aust 2014; 200(11): 658-662.
| Crossref | Google Scholar |
12 Thomas SL, Wakerman J, Humphreys JS. Ensuring equity of access to primary health care in rural and remote Australia – what core services should be locally available? Int J Equity Health 2015; 14(1): 111-119.
| Crossref | Google Scholar |
13 Ahn E, Kim J, Rahman K, et al. Development of a risk predictive scoring system to identify patients at risk of representation to emergency department: a retrospective population-based analysis in Australia. BMJ Open 2018; 8(9): e021323.
| Crossref | Google Scholar |
14 Hippisley-Cox J, Coupland C. Predicting risk of emergency admission to hospital using primary care data: derivation and validation of QAdmissions score. BMJ Open 2013; 3: e003482.
| Crossref | Google Scholar |
15 Khanna S, Rolls DA, Boyle J, et al. A risk stratification tool for hospitalisation in Australia using primary care data. Sci Rep 2019; 9(1): 5011.
| Crossref | Google Scholar |
16 Walter M, Kukutai T. Artificial Intelligence and Indigenous Data Sovereignty. Input paper for the Horizon Scanning Project “The Effective and Ethical Development of Artificial Intelligence: An Opportunity to Improve Our Wellbeing” on behalf of the Australian Council of Learned Academies. 2018. Available at https://acola.org/wp-content/uploads/2019/07/acola-ai-input-paper_indigenous-data-sovereignty_walter-kukutai.pdf
17 Australian Institute of Health and Welfare (AIHW). National Healthcare Agreement: PI 18–Selected potentially preventable hospitalisations, 2019. Canberra: Australian Institute of Health and Welfare; 2019. Available at https://meteor.aihw.gov.au/content/index.phtml/itemId/698904 [accessed 9 March 2020].
18 National Centre for Classification in Health. International statistical classification of diseases and related health problems, 10th revision, Australian modification (ICD-10-AM). Sydney: National Centre for Classification in Health, University of Sydney; 2019. Available at https://icd.who.int/browse10/2019/en [accessed 15 June 2020].
19 Wallace E, Stuart E, Vaughan N, et al. Risk Prediction models to predict emergency hospital admission in community-dwelling adults: A systematic review. Med Care 2014; 52(8): 751-765.
| Crossref | Google Scholar |
20 Van Calster B, McLernon DJ, van Smeden M, et al. Calibration: the Achilles heel of predictive analytics. BMC Med 2019; 17(1): 230.
| Crossref | Google Scholar |
22 Collins GS, Reitsma JB, Altman DG, Moons KGM. Transparent Reporting of a multivariable prediction model for Individual Prognosis or Diagnosis (TRIPOD): the TRIPOD statement. Ann Intern Med 2015; 162(1): 55-63.
| Crossref | Google Scholar |
24 Davy C, Bleasel J, Liu H, et al. Effectiveness of chronic care models: opportunities for improving healthcare practice and health outcomes: a systematic review. BMC Health Serv Res 2015; 15(1): 194.
| Crossref | Google Scholar |
25 Howard R, Sanders R, Lydall-Smith SM. The implementation of Restoring Health: a chronic disease model of care to decrease acute health care utilization. Chron Respir Dis 2008; 5(3): 133-141.
| Crossref | Google Scholar |
26 Sack C, Phan VA, Grafton R, et al. A chronic care model significantly decreases costs and healthcare utilisation in patients with inflammatory bowel disease. J Crohns Colitis 2012; 6(3): 302-310.
| Crossref | Google Scholar |