The persistence of a SIR disease in a metapopulation: Hendra virus epidemics in Australian black flying foxes (Pteropus alecto)
Jaewoon Jeong
A Environmental Futures Research Institute, Griffith University, Brisbane, Qld 4111, Australia.
B Present address: Centre for Veterinary Epidemiological Research, Department of Health Management, Atlantic Veterinary College, University of Prince Edward Island, Charlottetown, Prince Edward Island, C1A 4P3, Canada.
C Environmental Futures Research Institute and School of Environment and Science, Griffith University, Southport, Qld 4222, Australia.
D Corresponding author. Email: jjeong@upei.ca
Australian Journal of Zoology 69(1) 1-11 https://doi.org/10.1071/ZO20094
Submitted: 19 November 2020 Accepted: 14 April 2021 Published: 24 May 2021
Journal Compilation © CSIRO 2021 Open Access CC BY-NC-ND
Abstract
Understanding how emerging viruses persist in bat populations is a fundamental step to understand the processes by which viruses are transmitted from reservoir hosts to spillover hosts. Hendra virus, which has caused fatal infections in horses and humans in eastern Australia since 1994, spills over from its natural reservoir hosts, Pteropus bats (colloquially known as flying foxes). It has been suggested that the Hendra virus maintenance mechanism in the bat populations might be implicated with their metapopulation structure. Here, we examine whether a metapopulation consisting of black flying fox (P. alecto) colonies that are smaller than the critical community size can maintain the Hendra virus. By using the Gillespie algorithm, stochastic mathematical models were used to simulate a cycle, in which viral extinction and recolonisation were repeated in a single colony within a metapopulation. Given estimated flying fox immigration rates, the simulation results showed that recolonisation occurred more frequently than extinction, which indicated that infection would not go extinct in the metapopulation. Consequently, this study suggests that a collection of transient epidemics of Hendra virus in numerous colonies of flying foxes in Australia can support the long-term persistence of the virus at the metapopulation level.
Keywords: black flying fox, Hendra virus, infection dynamics, metapopulation, reservoir hosts, stochastic model, viral invasion, viral persistence.
Introduction
Bats are a common source of emerging zoonotic diseases that can become serious threats. Among bat-borne viruses, Hendra virus (genus Henipavirus) has raised serious public health concerns in eastern Australia. Spillover of Hendra virus from bats of the genus Pteropus (colloquially known as flying foxes or fruit bats) has led to high mortality rates in horses, with onward infection to humans by horses, also resulting in high mortality (Halpin et al. 2000). These spillovers of Hendra virus have stimulated research effort to elucidate how the virus persists in its natural reservoir hosts, flying foxes (Breed et al. 2011; Plowright et al. 2011; Wang et al. 2013). Because long-term maintenance of a virus is a fundamental difference between reservoir hosts and spillover hosts, the understanding of the maintenance mechanism is a fundamental step to understand the whole system of the reservoir-spillover system of emerging infectious diseases (Haydon et al. 2002).
The dynamics of Hendra virus in flying foxes are not fully understood, and researchers have proposed different mechanisms for virus maintenance in flying fox populations. Breed et al. (2011) suggested, from serosurveillance evidence, that a spectacled flying fox (P. conspicillatus) population was endemically infected with Hendra virus. Wang et al. (2013) proposed that recrudescent infection contributes to the maintenance of Hendra virus in flying fox populations. Although these arguments are based on reasonable data and inferences, they cannot be confirmed because current empirical data are inadequate to provide conclusive evidence (Plowright et al. 2016). Plowright et al. (2011) stressed the importance of metapopulation structure for Hendra virus maintenance in flying fox populations. Hendra virus spillover or maintenance is deeply implicated with the metapopulation structure of flying foxes. Thus, depending on the metapopulation dynamics, the duration and the magnitude of epidemics can vary. Out of these suggestions, this study concentrates on the impact of metapopulation (Plowright et al. 2011) and explores the effects of population size, herd immunity, and transmission mode of the virus on the viral epidemics in the metapopulation structure.
Bats often form spatially discrete but frequently interacting colonies, establishing a metapopulation structure (Calisher et al. 2006). The role of metapopulation dynamics in viral maintenance is closely related to the rescue effect, which is defined as transmission between colonies that acts to reinfect colonies where the infection has gone extinct (Keeling 2000). The effect contributes to preventing a local extinction from extending into a global one (Hagenaars et al. 2004). If Hendra virus causes an acute immunising infection, Hendra virus in a single population would be maintained less likely due to a short infectious period and high herd immunity. In that case, virus maintenance could become more dependent on the rescue effect provided by the metapopulation structure (Metcalf et al. 2013). Therefore, to estimate role of the rescue effect, an investigation of metapopulation on virus maintenance is undertaken by evaluating the relative movement frequency of flying foxes compared with the infectious period, to understand the viral persistence mechanism on flying fox populations (Cross et al. 2005).
Virus maintenance in a metapopulation structure could be easily achieved if some populations were larger than critical community size (CCS), which is the threshold population size above which pathogen persistence is more likely (Bartlett 1957). This situation was described as the ‘mainland–island’ model, in which mainland and island represent populations larger than CCS and populations smaller than CCS, respectively (Grenfell and Harwood 1997; Hanski and Gilpin 1997). Even in exceptionally large population sizes of grey-headed flying foxes (P. poliocephalus), Hendra virus could be expected to become extinct within 10 years with the assumption of a seven-day infectious period and seasonal breeding (Peel et al. 2014). Grey-headed flying foxes may not be as biologically competent hosts as black flying foxes (P. alecto), which are more likely to be involved with Hendra virus spillover. Thus, we need to investigate whether a metapopulation consisting of only colonies smaller than CCS can maintain Hendra virus persistence. This situation can be described as ‘island–island’ model. In an ‘island–island’ model, global persistence would be substantially assisted by the rescue effect via an ensemble of transient epidemics (Plowright et al. 2011).
Three hypotheses for Hendra virus dynamics have been suggested: susceptible–infectious–immune (SIR), susceptible– infectious–immune–susceptible (SIRS), and susceptible– infectious–latent–infectious (SILI) dynamics (Plowright et al. 2016). SIR dynamics are not consistent with Hendra virus persistence in a single colony of flying foxes, so long-term persistence requires metapopulation dynamics. For this reason, this study is based on SIR dynamics, not on SIRS or SILI dynamics that provide more favourable conditions for Hendra virus to be maintained than SIR dynamics. The lack of understanding of the transmission mode of Hendra virus should facilitate the investigation of Hendra virus transmission dynamics in both density-dependent and frequency-dependent transmission modes. Although it has been suggested that Hendra virus might be transmitted via a combination of the two transmission modes in flying foxes (Plowright et al. 2015), the details of the effects of each mode in transmission dynamics have remained obscure.
Plowright et al. (2011) generated important findings on the effects of metapopulation structures of flying foxes on Hendra virus dynamics. The authors showed how extrinsic factors such as anthropogenic land-use changes might affect the metapopulation dynamics, modifying the virus dynamics to facilitate Hendra virus spillover. They emphasised the importance of an ensemble of epidemic types for virus maintenance in metapopulation. Nevertheless, numerical evidence is needed to show the suitability of flying fox metapopulations for Hendra virus maintenance. For this purpose, we model a single population of black flying foxes in the context of a metapopulation structure to closely observe the virus extinction and recolonisation in the colony. The model is used to estimate the periods of an infected state and the periods of an infection-free state of the colony, and the model is based on the black flying foxes, because this bat species is the most involved with Hendra virus spillover. The two kinds of periods are utilised in the strict-sense Levins metapopulation model (Levins 1969) to determine whether long-term virus maintenance is plausible in the black flying fox metapopulation.
Materials and methods
Model development
The circular structure of Hendra virus extinction and recolonisation consisted of three stages (Fig. 1). In Stage 1, an infectious bat was introduced into an infection-free colony, which had been previously exposed to the virus and thus was partially immune. The introduction of an infectious bat simulated a rescue effect, possibly triggering an epidemic. Following this virus introduction, the virus could disappear from the population (i.e. viral fadeout). The herd immunity at the point of viral fadeout was recorded. In Stage 2, the herd immunity of the population decreased during the infection-free state, as immune bats died and were replaced by susceptible young. In Stage 3, we estimated the duration of the infection-free state as the time elapsed between infection extinction and introduction of the first infectious individual. Our model was specifically designed to explore the following three questions in each stage, given a certain set of parameter values: (1) How long are the periods from recolonisation to extinction? (2) How fast does herd immunity decline in an infection-free population? (3) How long are the periods between viral extinction and recolonisation?
In our model, the cycle was simulated in population sizes of 1000 to 150 000 to simulate the broad range of colony sizes of flying fox colonies. We iterated this cycle 1000 times and discarded the first 200 iterations to remove the effect of the arbitrary choice of the initial herd immunity (HI = 0.5). From those remaining 800 iterations, we obtained the distribution of the periods of the infected state. The stochastic simulations were implemented using GillespieSSA (Gillespie stochastic simulation algorithm) (Gillespie 2007; Pineda-Krch 2008). The time of events and which event would occur were stochastically determined by the previous events with their transition rates (Keeling and Rohani 2008; Lu et al. 2013). We used R package ‘GillespieSSA’ to run the stochastic models (Pineda-Krch 2010).
We applied both density- and frequency-dependent transmissions in the models. However, previous mathematical modelling studies of Hendra virus in flying foxes have been based on density-dependent transmission (Plowright et al. 2011; Wang et al. 2013), and the frequency-dependent transmission rate was not explored. Thus, we estimated the frequency-dependent transmission rate (β′) through density-dependent transmission rate (β) and the population size of our models (Table 1). Among total population (N), susceptible (S) individuals become infectious (I) at the rate βSI in a density-dependent transmission model or β′SI/N in a frequency-dependent transmission model (Table 2). The frequency-dependent transmission rate was set to be equivalent to the density-dependent transmission rate in a population size of 10 000. Individuals were assumed to be infectious until recovery at rate γ, 1/(infectious period) and survived as immune (R) for life. The transmission rates and recovery rate were referred from the estimated values in Plowright et al. (2011).
![]() |
![]() |
Stage 1 – infected state
Stage 1 estimated the duration of the infected state of a population following viral introduction into a population. The duration of the infected state was defined as the time in days from the viral introduction until the number of infectious bats became zero. This stage was simulated for up to two years, or as long as the infectious hosts existed. If the infection persisted for two years, it was defined as ‘viral persistence’. If the maximum number of infectious hosts did not exceed 50 and the infection disappeared in 50 days, it was defined as initial fadeout. Epidemic fadeout indicates viral fadeout due to a deep trough in several infected individuals right after a high peak of epidemics, while endemic fadeout can occur due to a stochastic decrease in the number of infected individuals. However, we did not differentiate between epidemic fadeout and endemic fadeout, because of the very low number of infectious hosts in an endemic equilibrium state, given plausible parameter values. Both fadeouts were defined to be viral extinction within two years after an epidemic.
Stage 2 – infection-free state
Stage 2 modelled the declining herd immunity during infection-free state after virus extinction (end of Stage 1) until the next introduction of an infectious individual (Stage 3). Stage 2 was devised to estimate herd immunity to be used in Stage 3. To calculate decreasing herd immunity, we used an equation

where HI′, HI, μ, and d, respectively, denote decreasing herd immunity over time, herd immunity at the moment of virus extinction, daily mortality rate, and days. This equation was solved deterministically. Assuming lifelong immunity based on SIR dynamics, a reduction in the number of immune bats was caused only by replacement following death of susceptible or immune adults with birth of susceptible newborns. Immigrating and emigrating bats were assumed not to affect the herd immunity. We kept the colony size constant by assuming the same number of births and deaths, and the same number of immigrants and emigrants.
Stage 3 – immigration of an infectious bat into an infection-free population
In Stage 3, the duration between viral extinction until viral reintroduction was estimated with the parameter values that could generate the longest possible duration, under the least favourable conditions for the virus to be maintained. Two factors were required to estimate the duration. First, it was necessary to assess the movement rate (m) of black flying foxes among colonies. We analysed the movement data of the bats in a metapopulation in the region of the Sunshine Coast, Queensland, Australia (Towsey 2017). The movement rate was assessed from the mean movement rate of black flying foxes among colonies between the mean rate (m = 1/13 days) and the median rate (1/5 days). Second, we considered the prevalence (Prev) in colonies from which bats migrated into the infection-free colony. This source prevalence (Prev = 0.0017) was obtained from the endemic equilibrium of the SIR dynamics in Stage 1, in which a colony size of 10 000 was assumed. This estimated prevalence is much lower than the prevalence (0.03) observed by Edson et al. (2015), which found an overall mean prevalence across more than 1400 individual P. alecto samples. The prevalence might fluctuate seasonally as the virus’s environmental load follows seasonal patterns. Thus, we used the estimated prevalence of 0.0017 to represent the low prevalence between the peaks of prevalence, which is a difficult scenario for Hendra virus persistence. We assumed that immigration and emigration occurred at the same rates, keeping the colony size constant.
To calculate the period until at least one infectious bat migrated into an infection-free colony from other infected colonies, we first calculated the probability (R) that no infectious bats migrated into an infection-free colony per day. For this purpose, we used the equation

Then, we calculated the number of days until the probability of migration of at least one bat exceeded 0.95. For this purpose, we used the equation
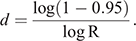
This equation was obtained from R′ ≥ 0.95 = 1–Rd, where R′ represented the probability that at least one infectious bat migrated from other infected colonies to an infection-free colony during a period of d. Details of the equations are shown in Appendix 1.
Levins metapopulation model
The strict-sense Levins metapopulation model (Levins 1969) was used to represent the metapopulation structure required to support the long-term maintenance of Hendra virus. In the Levins metapopulation model, a colony was simply defined as being either infection-free or infected (Keeling and Rohani 2008). The fraction of occupied patches, denoted by P, is the probability that a particular host patch is infected by the disease (Foley et al. 1999), and the dynamics are given by
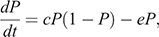
where c and e are the colonisation rate and the per-colony viral extinction rate, respectively. We estimated c and e as a reciprocal of the mean periods of infected state from the repetition of the cycle and as a reciprocal of the mean periods of infection-free state from Stage 1, respectively. The Levins metapopulation equilibrium value of P is given by

(Foley et al. 1999). If > 0, then the strict-sense Levins metapopulation would not go extinct across all patches (Foley et al. 1999), which indicates that Hendra virus would persist within the metapopulation of black flying foxes in the long term.
Results
Repetition of the cycle
In the process of repeated viral recolonisation and extinction, extinction (marking the end of Stage 1) occurred in one of three forms – initial, epidemic, or endemic fadeout – unless viral persistence occurred without extinction for two years. A considerable portion of viral introductions did not bring about a substantial increase in the number of infected hosts and failed to trigger an epidemic, resulting in initial fadeout (Fig. 2). In both density- and frequency-dependent models, a relatively small portion of viral introductions succeeded in triggering epidemics, leading to epidemic fadeout, endemic fadeout, or viral persistence. The low number of infectious individuals in the endemic equilibrium state made epidemic fadeout and endemic fadeout difficult to distinguish in these simulations. Large colony sizes that resulted in a higher proportion of viral persistence generated a high ratio of infected periods to infection-free periods with density-dependent transmission. In contrast, that result was not observed in the simulations with frequency-dependent transmission (Fig. 3).
![]() |
![]() |
Fig. 4 shows an example of simulation results, in which the period of infected state and herd immunity at the timing of viral fadeout that each viral introduction resulted in were presented. Red lines in Fig. 4 (i.e. herd immunity at the timing of viral fadeout) show very abrupt increases and relatively gradual decreases. More frequently occurring initial fadeouts contributed little to the decrease in herd immunity, while less frequently occurring long-term infections increased herd immunity massively. A single long-term infection and a series of short-term infections occurred alternatingly to maintain the herd immunity within a certain range. Long-term infection leading to epidemic or endemic fadeout produced a large increase in herd immunity, while short-term infection causing initial fadeout resulted in a slight decrease in herd immunity. As a result, periods of the infected state consisted of a low frequency of long-term infections and high frequency of short-term infections, and thereby the variance in the periods of the infected state was wide.
![]() |
The mean length of the periods in the infected state increased as colony size increased, but the length was more substantially affected by colony size in density-dependent transmission than frequency-dependent transmission (Fig. 5). The mean length of the periods in the infection-free state decreased regardless of the transmission mode. Except for the markedly small-sized colonies, the mean length of the periods of the infected state was longer than the mean periods of infection-free state for both density-dependent and frequency-dependent transmission.
![]() |
Levins metapopulation model
The colonisation rate (c) was overall higher than the extinction rate (e). Consequently, the Levins metapopulation equilibrium value, , was higher than zero and overall close to 1, except for the small-sized colonies with density-dependent transmission (Fig. 6). The modelling result predicted that extinction of Hendra virus in the metapopulation was highly unlikely. Therefore, given relatively regular immigration of infectious bats from outside a colony, it was predicted that, at the chosen parameters, Hendra virus could be indefinitely maintained in a metapopulation of black flying foxes that did not include colonies sufficiently large to be able to maintain persistent endemic infection.
In addition to the Gillespie algorithm, we simulated a deterministic model with Ordinary Differential Equations (ODE) to perform sensitivity analysis (Appendix 2). This investigation was conducted to see how robust the results of the Gillespie method were. The variations in key parameter values did not affect the equilibrium values substantially in density-dependent transmission, whereas the variations resulted in considerable differences in the equilibrium values in frequency-dependent transmission.
Discussion
Our results show that it is possible for Hendra virus to persist in metapopulation dynamics of flying foxes. The modelling results showed that a colony of flying foxes was estimated to be in an infected state longer than in an infection-free state, given that infectious flying foxes reasonably regularly immigrated into the colony from other infected colonies. The results also reveal that a collection of transient epidemics can ensure global persistence, even without colonies larger than CCS.
Plowright (2007) suggested that the balance between extinction and recolonisation is critical for the maintenance of the Hendra virus in flying foxes, based on the Levins effect (Hanski 1999). By employing the Levins metapopulation model (Levins 1969), this study showed that the recolonisation rate was higher than the extinction rate, which meant that the periods of the infected state were longer than the periods of infection-free state in colonies in accordance with Plowright et al. (2011). The study provides further modelling evidence that if the Hendra virus is a SIR disease, metapopulation dynamics may be significant in maintaining the Hendra virus.
This study improved the understanding of the maintenance mechanism of Hendra virus-based metapopulation dynamics as explored in Plowright et al. (2011). While these authors simulated many colonies in metapopulations to show comprehensively the role of metapopulation structure on virus dynamics, we modelled a single colony in the context of a metapopulation to examine specifically the events occurring in a colony within a metapopulation. Our modelling results showed how the virus fadeout or persistence is determined by the basic epidemiological factors such as population size or transmission modes and emphasise the importance of accurate identification of transmission mode.
Whether Hendra virus transmission strictly follows SIR dynamics or a combination of SIR, SIRS and SILI remain unclear (Plowright et al. 2016). Nevertheless, if we suppose SIR dynamics and exclude reactivation of the virus, metapopulation dynamics are essential to understand Hendra virus persistence because individual colonies of flying foxes do not appear to be larger than CCS (Peel et al. 2014). Appendix 3 shows that a single colony comprising more than 100 000 flying foxes is necessary to support endemic persistence of the virus for longer than two years. Flying fox colonies of such size exist only for short periods. Colony sizes change seasonally in response to feeding availability (Giles et al. 2018) and the population size fluctuates due to seasonal breeding (Peel et al. 2014). The large CCS for Hendra virus infection in flying foxes is mainly attributable to the short infectious period of Hendra virus (Plowright et al. 2011) (estimated mean period of seven days, although some bats may stay infectious longer: see Plowright et al. (2016)). The short infectious period leaves a small number of infected individuals in endemic equilibrium so that the infection becomes vulnerable to stochastic extinction (that is, endemic fadeout). It should be referable that an infectious period of ~36.5 days would be required to have a 50% probability of maintaining Hendra virus infection in a single population of 10 000 seasonally breeding grey-headed flying foxes, given HI = 0.5 at the moment of viral introduction (Peel et al. 2014).
In a metapopulation, a relatively high movement rate compared with a recovery rate is required for infectious individuals to be able to have a high probability of spreading the infection to other populations while they are infectious (Cross et al. 2005). Consequently, a low movement rate inhibits the spread of infection. On the other hand, a movement rate that is too high reduces spatial heterogeneity and may result in synchronised epidemics in each population (Jesse et al. 2008). Synchronised epidemics reduce the rescue effect because when a colony loses infection, it would be more likely to be surrounded by infection-free colonies. As a result, it has been found that persistence is maximised at an intermediate level of movement rate in a metapopulation structure (Grenfell and Harwood 1997). Also, in the case of Hendra virus maintenance, a combination of various duration and magnitude of epidemics is important to maintain asynchronous epidemics (Plowright et al. 2011). The availability of more realistic data on flying fox distributions could allow a quantitative analysis of the effect of synchrony on the persistence of the Hendra virus in a metapopulation (Tran-Thi et al. 2016).
The metapopulation structure of black flying foxes is related not only to viral persistence but also to epidemic magnitude. Increasing numbers of flying foxes in urban areas (Tait et al. 2014) may have two opposing implications for the viral epidemic magnitude. On the one hand, overall flying foxes move more frequently between nearby colonies than between distant colonies (Roberts et al. 2012). Colonies in urban areas tend to be closer to each other than are colonies in rural areas, which would likely result in higher intercolony movement rates for urban bats than for rural bats. Consequently, the frequent introduction of infectious bats into infection-free colonies in urban areas appears to reduce the impact of epidemics, because the short period in the infection-free state would result in only a slight diminishment of herd immunity (i.e. still high herd immunity). On the other hand, urban bats may move less frequently than rural bats, because closer locations between roosting sites and feeding sites and stable sources of food in urban areas decrease the necessity for movement (Plowright et al. 2011). Such decreased movement of urban bats might facilitate high-impact epidemics by allowing more time for herd immunity to decline in infection-free colonies. It is not certain how increasing bats in urban areas affect epidemic magnitude with the two contradicting hypotheses. More detailed observations of flying fox movements in urban areas, as compared with rural areas, are required to better understand the effects of increasing urban colonies on Hendra virus epidemic magnitude and further spillover risk.
In conclusion, the metapopulation structure of black flying foxes appeared to support the maintenance of Hendra virus in the bat populations. The maintenance was possible because of the frequent movement of individual bats among nearby colonies, which supports frequent epidemics in each colony. Hendra virus maintenance in its reservoir populations may rely on a collection of epidemics in numerous colonies that are smaller than CCS. This study found that the recolonisation rate was higher than the extinction rate, indicating that the metapopulation structure of flying foxes contributed to the ability of bats to function as reservoir hosts for the Hendra virus. The long-term persistence could be possible mainly because the high movement rate of flying foxes between colonies introduces infectious bats into infection-free colonies soon after the infection becomes extinct in the colonies. Increasing data of flying fox distributions are expected to facilitate more detailed modelling studies dealing with metapopulation structures (Meade et al. 2019).
Conflicts of interest
The authors declare no conflicts of interest.
Declaration of funding
This work was supported by the Commonwealth of Australia, the states of New South Wales and Queensland under the National Hendra Virus Research Program, awarded through the Rural Industries Research and Development Corporation (RIRDC). JJ is supported by a Griffith University Postgraduate Research Scholarship and Griffith University International Postgraduate Research Scholarship and was supported by Ocean Frontier Institute. HM was supported by DARPA BAAHR001118S0017 D18AC00031 and the National Science Foundation DEB-1716698.
Acknowledgements
The authors appreciate the valuable support from Raina K. Plowright and Alison J. Peel.
References
Bartlett, M. S. (1957). Measles periodicity and community size. Journal of the Royal Statistical Society. Series A (General) 120, 48–70.| Measles periodicity and community size.Crossref | GoogleScholarGoogle Scholar |
Breed, A. C., Breed, M. F., Meers, J., and Field, H. E. (2011). Evidence of endemic Hendra virus infection in flying-foxes (Pteropus conspicillatus) – implications for disease risk management. PLoS One 6, e28816.
| Evidence of endemic Hendra virus infection in flying-foxes (Pteropus conspicillatus) – implications for disease risk management.Crossref | GoogleScholarGoogle Scholar | 22194920PubMed |
Calisher, C. H., Childs, J. E., Field, H. E., Holmes, K. V., and Schountz, T. (2006). Bats: important reservoir hosts of emerging viruses. Clinical Microbiology Reviews 19, 531–545.
| Bats: important reservoir hosts of emerging viruses.Crossref | GoogleScholarGoogle Scholar | 16847084PubMed |
Cross, P. C., Lloyd-Smith, J. O., Johnson, P. L. F., and Getz, W. M. (2005). Duelling timescales of host movement and disease recovery determine invasion of disease in structured populations. Ecology Letters 8, 587–595.
| Duelling timescales of host movement and disease recovery determine invasion of disease in structured populations.Crossref | GoogleScholarGoogle Scholar |
Edson, D., Field, H., McMichael, L., Jordan, D., Kung, N., Mayer, D., and Smith, C. (2015). Flying-fox roost disturbance and Hendra virus spillover risk. PLoS One 10, e0125881.
| Flying-fox roost disturbance and Hendra virus spillover risk.Crossref | GoogleScholarGoogle Scholar | 26625128PubMed |
Foley, J. E., Foley, P., and Pedersen, N. C. (1999). The persistence of a SIS disease in a metapopulation. Journal of Applied Ecology 36, 555–563.
| The persistence of a SIS disease in a metapopulation.Crossref | GoogleScholarGoogle Scholar |
Giles, J. R., Eby, P., Parry, H., Peel, A. J., Plowright, R. K., Westcott, D. A., and McCallum, H. (2018). Environmental drivers of spatiotemporal foraging intensity in fruit bats and implications for Hendra virus ecology. Scientific Reports 8, 9555.
| Environmental drivers of spatiotemporal foraging intensity in fruit bats and implications for Hendra virus ecology.Crossref | GoogleScholarGoogle Scholar | 29934514PubMed |
Gillespie, D. T. (2007). Stochastic simulation of chemical kinetics. Annual Review of Physical Chemistry 58, 35–55.
| Stochastic simulation of chemical kinetics.Crossref | GoogleScholarGoogle Scholar | 17037977PubMed |
Grenfell, B., and Harwood, J. (1997). (Meta)population dynamics of infectious diseases. Trends in Ecology & Evolution 12, 395–399.
| (Meta)population dynamics of infectious diseases.Crossref | GoogleScholarGoogle Scholar |
Hagenaars, T., Donnelly, C., and Ferguson, N. (2004). Spatial heterogeneity and the persistence of infectious diseases. Journal of Theoretical Biology 229, 349–359.
| Spatial heterogeneity and the persistence of infectious diseases.Crossref | GoogleScholarGoogle Scholar | 15234202PubMed |
Halpin, K., Young, P., Field, H., and Mackenzie, J. (2000). Isolation of Hendra virus from pteropid bats: a natural reservoir of Hendra virus. The Journal of General Virology 81, 1927–1932.
| Isolation of Hendra virus from pteropid bats: a natural reservoir of Hendra virus.Crossref | GoogleScholarGoogle Scholar | 10900029PubMed |
Hanski, I. (1999). ‘Metapopulation Ecology.’ (Oxford University Press: Oxford.)
Hanski, I., and Gilpin, M. E. (1997). ‘Metapopulation Biology.’ (Academic Press: Cambridge, MA.)
Haydon, D. T., Cleaveland, S., Taylor, L. H., and Laurenson, M. K. (2002). Identifying reservoirs of infection: a conceptual and practical challenge. Emerging Infectious Diseases 8, 1468–1473.
| Identifying reservoirs of infection: a conceptual and practical challenge.Crossref | GoogleScholarGoogle Scholar | 12498665PubMed |
Jesse, M., Ezanno, P., Davis, S., and Heesterbeek, J. (2008). A fully coupled, mechanistic model for infectious disease dynamics in a metapopulation: movement and epidemic duration. Journal of Theoretical Biology 254, 331–338.
| A fully coupled, mechanistic model for infectious disease dynamics in a metapopulation: movement and epidemic duration.Crossref | GoogleScholarGoogle Scholar | 18577388PubMed |
Keeling, M. J. (2000). Metapopulation moments: coupling, stochasticity and persistence. Journal of Animal Ecology 69, 725–736.
| Metapopulation moments: coupling, stochasticity and persistence.Crossref | GoogleScholarGoogle Scholar |
Keeling, M. J., and Rohani, P. (2008). ‘Modeling Infectious Diseases in Humans and Animals.’ (Princeton University Press: Princeton.)
Levins, R. (1969). Some demographic and genetic consequences of environmental heterogeneity for biological control. Bulletin of the Entomological Society of America 15, 237–240.
| Some demographic and genetic consequences of environmental heterogeneity for biological control.Crossref | GoogleScholarGoogle Scholar |
Lu, Z., Schukken, Y. H., Smith, R. L., and Grohn, Y. T. (2013). Using vaccination to prevent the invasion of Mycobacterium avium subsp paratuberculosis in dairy herds: a stochastic simulation study. Preventive Veterinary Medicine 110, 335–345.
| Using vaccination to prevent the invasion of Mycobacterium avium subsp paratuberculosis in dairy herds: a stochastic simulation study.Crossref | GoogleScholarGoogle Scholar | 23419983PubMed |
Meade, J., Van der Ree, R., Stepanian, P. M., Westcott, D. A., and Welbergen, J. A. (2019). Using weather radar to monitor the number, timing and directions of flying-foxes emerging from their roosts. Scientific Reports 9, 10222.
| Using weather radar to monitor the number, timing and directions of flying-foxes emerging from their roosts.Crossref | GoogleScholarGoogle Scholar | 31308411PubMed |
Metcalf, C. J. E., Hampson, K., Tatem, A. J., Grenfell, B. T., and Bjornstad, O. N. (2013). Persistence in epidemic metapopulations: quantifying the rescue effects for measles, mumps, rubella and whooping cough. PLoS One 8, e74696.
| Persistence in epidemic metapopulations: quantifying the rescue effects for measles, mumps, rubella and whooping cough.Crossref | GoogleScholarGoogle Scholar |
Peel, A. J., Pulliam, J. R. C., Luis, A. D., Plowright, R. K., O’Shea, T. J., Hayman, D. T. S., Wood, J. L. N., Webb, C. T., and Restif, O. (2014). The effect of seasonal birth pulses on pathogen persistence in wild mammal populations. Proceedings of the Royal Society B: Biological Sciences 281, 20132962.
| The effect of seasonal birth pulses on pathogen persistence in wild mammal populations.Crossref | GoogleScholarGoogle Scholar | 24827436PubMed |
Pineda-Krch, M. (2008). GillespieSSA: implementing the Gillespie stochastic simulation algorithm in R. Journal of Statistical Software 25, 1–18.
| GillespieSSA: implementing the Gillespie stochastic simulation algorithm in R.Crossref | GoogleScholarGoogle Scholar |
Pineda-Krch, M. (2010). GillespieSSA: Gillespie’s stochastic simulation algorithm (SSA). R package version 0.5-4. Available at https://CRAN.R-project.org/package=GillespieSSA
Plowright, R. K. (2007). The ecology and epidemiology of Hendra virus in flying foxes. Ph.D. Thesis, University of California, Davis, USA.
Plowright, R., Foley, P., Field, H., Dobson, A., Foley, J., Eby, P., and Daszak, P. (2011). Urban habituation, connectivity, and stress synchrony: Hendra virus emergence from flying foxes (Pteropus spp.). EcoHealth 7, S36–S37.
Plowright, R. K., Eby, P., Hudson, P. J., Smith, I. L., Westcott, D., Bryden, W. L., Middleton, D., Reid, P. A., McFarlane, R. A., Martin, G., Tabor, G. M., Skerratt, L. F., Anderson, D. L., Crameri, G., Quammen, D., Jordan, D., Freeman, P., Wang, L. F., Epstein, J. H., Marsh, G. A., Kung, N. Y., and McCallum, H. (2015). Ecological dynamics of emerging bat virus spillover. Proceedings of the Royal Society B: Biological Sciences 282, 20142124.
| Ecological dynamics of emerging bat virus spillover.Crossref | GoogleScholarGoogle Scholar | 25392474PubMed |
Plowright, R. K., Peel, A. J., Streicker, D. G., Gilbert, A. T., McCallum, H., Wood, J., Baker, M. L., and Restif, O. (2016). Transmission or within-host dynamics driving pulses of zoonotic viruses in reservoir–host populations. PLoS Neglected Tropical Diseases 10, e0004796.
| Transmission or within-host dynamics driving pulses of zoonotic viruses in reservoir–host populations.Crossref | GoogleScholarGoogle Scholar | 27489944PubMed |
Roberts, B. J., Catterall, C. P., Eby, P., and Kanowski, J. (2012). Long-distance and frequent movements of the flying-fox Pteropus poliocephalus: implications for management. PLoS One 7, e42532.
| Long-distance and frequent movements of the flying-fox Pteropus poliocephalus: implications for management.Crossref | GoogleScholarGoogle Scholar | 22880021PubMed |
Tait, J., Perotto-Baldivieso, H. L., McKeown, A., and Westcott, D. A. (2014). Are flying-foxes coming to town? Urbanisation of the spectacled flying-fox (Pteropus conspicillatus) in Australia. PLoS One 9, e109810.
| Are flying-foxes coming to town? Urbanisation of the spectacled flying-fox (Pteropus conspicillatus) in Australia.Crossref | GoogleScholarGoogle Scholar | 25295724PubMed |
Towsey, J. (2017). Pteropus alecto Sunshine Coast. Available at https://www.movebank.org/panel_embedded_movebank_webapp
Tran-Thi, C.-G., Choisy, M., and Zucker, J. D. (2016). Quantifying the effect of synchrony on the persistence of infectious diseases in a metapopulation. In ‘Computing, and Communication Technologies, Research, Innovation, and Vision for the Future (RIVF), 2016 IEEE RIVF International Conference’. pp. 229–234. (IEEE.)
Vardon, M. J., and Tidemann, C. R. (2000). The black flying-fox (Pteropus alecto) in north Australia: juvenile mortality and longevity. Australian Journal of Zoology 48, 91–97.
| The black flying-fox (Pteropus alecto) in north Australia: juvenile mortality and longevity.Crossref | GoogleScholarGoogle Scholar |
Vynnycky, E., and White, R. (2010). ‘An Introduction to Infectious Disease Modelling.’ (Oxford University Press: Oxford.)
Wang, H. H., Kung, N. Y., Grant, W. E., Scanlan, J. C., and Field, H. E. (2013). Recrudescent infection supports Hendra virus persistence in Australian flying-fox populations. PLoS One 8, e80430.
| Recrudescent infection supports Hendra virus persistence in Australian flying-fox populations.Crossref | GoogleScholarGoogle Scholar | 24312221PubMed |
Appendix 1. Equations calculating the period of migration of an infectious bat in Stage 3
We devised two equations to calculate the period until an infectious bat migrates into an infection-free colony from other infected colonies. The two are:

in which the result of the first equation was used to calculate the second equation.
The first equation calculates the probability (R) that no infectious bats migrate into an infection-free colony per day. (1 – P(prevalence)) is the probability that a bat migrating from other infected colonies to an infection-free colony is not an infectious bat. To apply this probability for all immigrating bats into the infection-free colony per day, (1 – P) was raised to the power of the daily number of the immigrating bats, m*N, where m and N denote the daily rate of migration (movement rate) and colony size, respectively.
The second equation was devised to calculate the period of days (d) for at least one infectious bat to migrate into an infection-free colony from other infected colonies. For this purpose, we should estimate the probability (R′) that at least one infectious bat migrates from other infected colonies to an infection-free colony during a period of time, based on R calculated from the first equation. R′ exponentially decreases over time, and R′ ≥ 0.95 was assumed to assure the migration of at least one infectious bat. The processes to obtain the second equation is shown below:



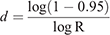
Appendix 2. Sensitivity analyses
The modelling results for Fig. 6 were reproduced by using ordinary differential equations with deterministic simulations. The deterministic simulations tested how the equilibrium value () was determined with five different values of three parameters of transmission rate, recovery rate, and herd immunity. In the simulations, one out of three parameter values varied, while keeping other two values constant, which was the value used in the Gillespie method.
In density-dependent transmission, the effect of various parameter values on the equilibrium value was generally subdued by colony size, except when the colony size was medium. In frequency-dependent transmission, the effect of various parameter values on the equilibrium value was two distinctive groups of high values and low values of the equilibrium value. This result indicates that there is a critical point in the range of parameter values that can determine the outcome of the equilibrium value. This result shows that frequency-dependent transmission is more sensitively reacting to the changes in parameter values than density-dependent transmission. As a result, the outcome in the main document is more robust with density-dependent transmission than with frequency-dependent transmission (Fig. A1).
Appendix 3. Magnitude and period of epidemics in the density-dependent transmission model and the frequency-dependent transmission model
In addition to modelling the cycle consisting of three stages, we modelled epidemics following viral introduction into infection-free populations. This modelling was used to explore the effects of colony size (N) and herd immunity at viral introduction (HI) on the magnitude and duration of epidemics. The epidemic magnitude was defined as the proportion of the maximum number of infected bats to the colony size (N), and epidemic duration was defined as the time in days from the viral introduction until the number of infectious bats became zero. Each combination of a range of colony sizes and herd immunities at viral introduction was simulated once for density-dependent transmission and once for frequency-dependent transmission over a two-year timeframe. In the model, two years were sufficient to encompass a complete epidemic peak and a subsequent trough. Fig. A2 shows the magnitude and duration of epidemics as a function of transmission modes, colony size (N), and herd immunity at viral introduction (HI). The magnitude of epidemics (as a proportion of the total colony size) increased with increasing colony size (N) in the density-dependent transmission model, whereas epidemic magnitude was constant regardless of colony size (N) in the frequency-dependent transmission model. This is in accord with an epidemiological principle that force of infection increases with increasing population size in density dependence, while the force of infection does not change with population size in frequency dependence (Vynnycky and White 2010).
Viral fadeout can be classified into three forms by the concept of net reproduction number (Rn), which means an average number of secondary infectious individuals resulting from one infectious individual in a partially susceptible population (Vynnycky and White 2010). When Rn < 1, epidemic did not occur (initial fadeout). When Rn > 1, epidemics could occur, and the epidemic patterns were different between when Rn was considerably higher than 1 and when Rn was slightly higher than 1. In the former, viral fadeout occurred at the deep trough following the high-impact epidemic (epidemic fadeout), whereas in the latter the infection survived the trough and lasted until the infection became extinct due to random fluctuations in the number of infected individuals (endemic fadeout). Additionally, the continuously high number of infected individuals in large-sized colonies excluded the possibility of endemic fadeout in density-dependent transmission mode.