Estimating feral cat (Felis catus) population density in eastern Australia’s subtropical rainforest using spatial capture-recapture incorporating unidentified individuals
Darren McHugh


A
B
C
D
E
F
Abstract
Management of broadly distributed invasive species requires knowledge of population densities across multiple ecosystems. The feral cat (Felis catus) has a continental distribution in Australia and has caused many declines and extinctions; however, density estimates from several ecosystems are few or lacking. Camera trapping data coupled with spatially explicit capture–recapture analysis is a suitable approach for estimating cat densities. However, if a large portion of individuals cannot be identified, density estimation may be difficult and estimates may be too low.
We aimed to estimate the feral cat density and population size within the subtropical Gondwanan Rainforest of eastern Australia, an area with world heritage status and high biodiversity values, and in which cat density was not known.
We used feral cat data from a grid of 60 camera traps deployed over 305 days in Border Ranges National Park, totalling 18,300 camera trapping nights. We employed a ‘random thinning’ spatially explicit capture–recapture model that considered detections of both known and unknown feral cat identities. Our modelling included a primary detection history of identified individuals and a secondary detection history that included pelage type, which allowed us to account for individuals with homogeneous pelage types.
Feral cat density was estimated at 0.858 cats km−2 (95% HPDI 0.432, 1.385), which is much higher than the average feral cat density across the Australian continent of 0.27 cats km−2 (95% CI: 0.18–0.45). The probability of identifying unique individuals was low for black and tabby cats compared with other pelage types. Population sizes during the four survey periods were estimated to be similar, with posterior medians ranging from 197 to 202 individuals and 95% highest posterior density intervals ranging from 95 to 329 individuals within a ~234 km2 area.
Our study provides the first robust feral cat density estimate from a subtropical rainforest ecosystem.
Our results add to a growing body of literature that suggests feral cat densities are high in productive mesic reserves of eastern Australia. Management of feral cats in Gondwanan World Heritage Areas should be prioritised to limit impacts on narrow-range endemic species that are likely prey to feral cats.
Keywords: eastern Australia, Felis catus, feral cat, invasive predator, population density, rainforest, spatially explicit capture–recapture.
Introduction
Feral cats (Felis catus) have invaded ecosystems across the world (Long 2003), contributing to the extinction of many vertebrate species (Doherty et al. 2016). Many extant taxa continue to be threatened by feral cat predation (Medina et al. 2011; Hardman et al. 2016) with these impacts being greatest in Australia, New Zealand and many other smaller islands (Campbell et al. 2011; Nogales et al. 2013). In Australia, cats have a continental-scale distribution and are also present on many of the larger islands (Legge et al. 2017). Their diet is broad, with cats being known to consume >790 native vertebrate species (Woinarski et al. 2019). Cats have been the primary driver for the extinctions of 22 Australian endemic mammals, and they threaten over 230 conservation listed species with extinction under Australian environmental law (Woinarski et al. 2019).
Given the major impacts feral cats have had on Australian biodiversity, predation by feral cats is listed as a key threatening process under Australian federal legislation, with policy and management strategies in place to reduce feral cat impacts (Commonwealth of Australia 2015; Legge et al. 2020a, 2020b). For example, the Australian Government’s 2022–2032 Threatened Species Action Plan highlights targets and actions to manage feral cats to protect priority species and in priority locations (Department of Climate Change, Energy, the Environment and Water 2022). The Australian federal, state and territory governments, environmental NGOs, Indigenous land managers, and private landholders all invest substantial effort into cat control (Garrard et al. 2020).
Understanding the variation of feral cat densities across different ecosystems, and the distributions of cat-susceptible native species, is fundamental to management strategy (Doherty et al. 2014). Most feral cat density studies conducted in Australia have occurred in arid and semi-arid regions, with fewer studies having been conducted in mesic ecosystems with more complex vegetation structure, such as rainforests (Legge et al. 2017). The geographic bias in survey effort for feral cats may be because cats are easier to study in open ecosystems using longstanding density estimation methods such as cage trapping and spotlighting, coupled with mark–recapture statistical approaches. Indeed, the lack of feral cat density estimates from productive mesic ecosystems may have contributed to the assumption that feral cats exist at lower densities in these ecosystems, and that their impacts there may also be lower (Chisholm and Taylor 2007; Legge et al. 2017). Owing to recent advances in technology, camera traps now provide an improved method for surveying feral cats in mesic ecosystems (McHugh et al. 2019, 2022; Rees et al. 2019; Bruce et al. 2022).
The average cat density across Australia has been estimated at 0.27 cats km−2 (95% confidence interval [CI]: 0.18–0.45), based on modelling the spatial variation in cat density from over 90 site-based studies (Legge et al. 2017). However, when these spatial models were developed, feral cat density estimates were not available for rainforest, wetland, mangrove or dense heath ecosystems. A later study by Rees et al. (2019) within mesic tall wet eucalypt forests and cool temperate rainforest in south-eastern Australia found the feral cat density to be 1.14 cats km−2 (95% CI: 0.89–1.47), which was five times higher than the national average from the continental-scale analysis (Legge et al. 2017). This highlights the need for additional feral cat density estimates from under-represented ecosystems to inform large-scale meta-analyses.
Capture–recapture and, increasingly, spatially-explicit capture–recapture models are used to estimate populations and densities of animal populations from camera trap detections when individuals can reliably be identified from photographs. This method has been particularly useful when examining felid species that display variation in pelage patterns, including tigers (Panthera tigris) and leopards (Panthera pardus fusca) in India (Karanth et al. 2004; Singh et al. 2023), ocelots (Leopardus pardalis) and jaguars (Panthera onca) in Brazil (Trolle and Kéry 2003; Soisalo and Cavalcanti 2006) and bobcats (Lynx rurus) in North America (Thornton and Perkins 2015). However, for many species, images retrieved from camera trap studies often contain a mixture of identifiable and unidentifiable individuals, which is the case for feral cats where it is common for multiple individuals to have indistinguishable pelage patterns (Rees et al. 2019; Miritis et al. 2020). This may result in conservative cat density estimates, particularly where the majority of feral cat detections are of black cats, because unidentifiable cats have a capture probability of zero, an extreme form of individual detection heterogeneity (Cove et al. 2023).
Spatial mark-resight (SMR) models can be used to accommodate these ‘partially-marked’ populations (e.g. Rich et al. 2014) and estimate density without bias when a subset of the population is unidentifiable. Alternatively, categorical spatial capture–recapture (SCR) models (Augustine et al. 2019) allow for density estimation using only categorical individual covariates (e.g. pelage type), but estimates can be biased and imprecise when many individuals have the same combinations of individual covariates (e.g. many black cats). Recently, Cove et al. (2023) combined the SMR-like random-thinning SCR model (Jiménez et al. 2021) with the categorical SCR model to allow for the use of both known-identity detections and categorical individual covariates for unidentified detections in an SMR-like analysis to improve the accuracy and precision of density estimates over those from SMR models that ignore this partially identifying information.
In this study, we used camera trap surveys and the categorical random-thinning model (Cove et al. 2023) to generate cat density estimates from structurally complex subtropical rainforest in the Gondwana Rainforests of eastern Australia World Heritage Area. This World Heritage Area is renowned for its biodiversity values, including a high incidence of species from ancient lineages, many with small distributions (Mahony 2010; Kooyman et al. 2020; Narsey et al. 2020). Many of the cats in our study could not be individually recognised, so we applied the ‘random thinning’ spatial capture–recapture model that considered detections of both known and unknown feral cat identities (Jiménez et al. 2021; Cove et al. 2023). This model capitalises on all the cat detections, including those that could not be identified to individual, to probabilistically resolve encounter histories and improve inference on density estimates.
Materials and methods
Study area and survey design
Our study was conducted in Border Ranges National Park (NP), New South Wales, Australia (28.395°S, 153.058°E) (Fig. 1). Border Ranges NP is located within the Gondwana Rainforests of Australia World Heritage Area renowned for its high biodiversity values and high species endemicity (Mahony 2010; Narsey et al. 2020). The reserve supports significant stands of subtropical and temperate rainforest (Kooyman et al. 2020). Border Ranges NP experiences a humid subtropical climate with summer maximum and winter minimum rainfall. The average annual rainfall in the region is 1159 mm (mean annual rainfall at Kyogle, NSW Airport AWS). Temperatures are mild to warm year-round, with warm wet summers and cool dry winters.
Study area. (a) location of Border Ranges NP in Northern New South Wales, Australia, (b) location of camera trapping grid in Border Ranges NP, (c) location of camera traps within rainforest classes, Border Ranges NP (Department of Climate Change, Energy, the Environment and Water 2024).
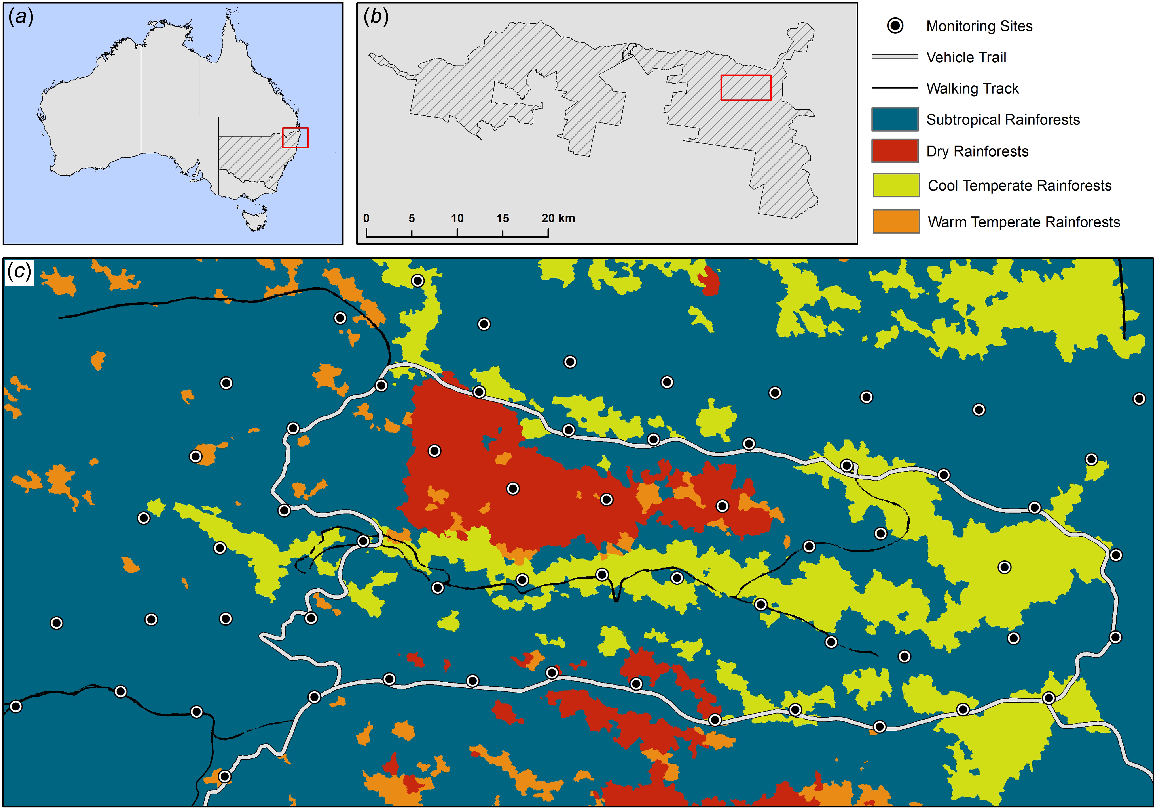
Camera traps were deployed across a grid at a minimum spacing of 500 m within the Brindle Creek area of Border Ranges NP (Fig. 1). The camera traps used were a mix of Reconyx Rapidfire™ HC 500 (infrared flash) (30 cameras) and HC 550 (white flash) (30 cameras), with their placement being randomised across the grid. Previous studies have shown that camera trap type (infrared and/or white flash) does not influence the detection of feral cats (McHugh et al. 2019; Taggart et al. 2020). Our camera traps covered a range of distances from tracks and trails (5–1305 m) and a range of elevations from 741 to 1046 m.
We fixed camera traps to trees at a height of 40 cm, directed towards a wooden stake (40 cm) that was positioned 2.5 m from the camera trap. A 5 × 15 cm PVC canister was fixed to each stake, housing a standard bait of peanut butter and oats (Stobo-Wilson et al. 2020). McHugh et al. (2019) showed that bait type (peanut butter and oats vs chicken neck and tuna oil) did not influence the detection of feral cats in the study area. In McHugh et al. (2019), feral cats were attracted to both lure types; however, many detections were made of feral cats where they were simply moving past the field of view of the camera without giving attention to the lure. Cameras were set to medium/high sensitivity and to take five images per trigger (one image per second) with a quiet period of 1 min between triggers. Cameras were set to operate for 24 h per day for a duration of 35 days in period one (7 October 2017–11 November 2017), 90 days in period two (20 July−18 October 2018), 90 days in period three (19 October 2018–17 January 2019) and 90 days in period four (18 January 2019–18 April 2019).
Individual feral cat identification
Image tagging software Exifpro version 2.1 (Exiv2 Software, France) was used to tag camera trap images in which feral cats were detected. Images were first tagged into groups on the basis of similar pelage characteristics, namely, black, black–ginger, black–white, tabby, tabby–white, ginger, and calico (Fig. 2). Individual cats were then identified and tagged with a unique identifying number on the basis of pelage colours and unique distinctive features, including stripes, spots and patterns on various parts of cat face, legs, feet, tail, flanks and dorsum. Two independent observers identified individual cats and deliberated over these identifications until a consensus was reached. Where consensus was not reached on individual identification, images were tagged with pelage type only.
Feral cat density estimation
To use both the individually identified detections and the observed pelage types for detections that were not individually identified to estimate density, we applied the categorical random-thinning model (Cove et al. 2023). In this model, the observed data are (1) a typical detection history where 1 indicates presence and 0 indicates absence over each day for individuals identified in photographs over the four survey periods, (2) the pelage types for these identified individuals, (3) the trap and occasion of detection for unidentified detections, and (4) the pelage type of unidentified detections. This information is then used to jointly estimate the unobserved individual identities (equivalent to the true capture history) and the regular SCR parameters in the hierarchal model presented below.
Because our data span multiple camera deployments over a duration during which population closure is likely to be violated, we structured our model into multiple sessions (multisession SCR; Sutherland et al. 2019), regarding individuals as independent between sessions. We divided our dataset into four sessions, with km being the number of trap occasions (days) in Session m. We assigned the k1 = 35 daily occasions from 2017 to Session 1 and divided the daily occasions from 2018 and 2019 to Sessions 2–4, with 90 daily occasions within each to balance the number of detections per session and meet the assumption of population closure within each session. The trapping array consisted of j = 1,…,60 camera traps located at xj = (xj,1, xj,2), which did not vary across sessions. Coordinates from camera trap locations were represented in kilometres for SCR modelling.
We define D to be the expected density shared across sessions and to be the expected abundance in Session m, where Am is the area of the state space (study area) of Session m. Because our sessions are temporal replicates of the same study area, we replaced session-specific study areas with a single value, A, i.e. λN = DA. To define the state space, we buffered the x and y extent of the trapping array with a rectangular buffer of 5 km, yielding a state space area of A = 233.6 km2. Although there are no feral cat home-range studies from subtropical rainforests, we suspect our 5 km buffer to be adequate, given the home range of feral cats on other tall wet forests where prey is abundant (Buckmaster and Dickman 2012). In addition, our estimated sigma was 0.68 km. A three times sigma buffer as recommended by Royle et al. (2013) is 2.04 km, therefore our buffer of 5 km is more than sufficient.
We then allow the realised abundance in Session m, Nm, to vary as follows:
Within each session, individuals have both an activity centre and a coat type. The individual activity centre of Cat i = 1,…,Nm during Session m (si,m,1, si,m,2) was modelled as a homogeneous point process such that si,m ~ Uniform (S), where S is the rectangular state space. Then, we designated seven coat types (black, black–ginger, black–white, tabby, tabby–white, ginger, and calico), and modelled the coat type of Individual i in Session m as
where γ is a vector of length seven, with γl corresponding to the probability that an individual will have Coat pattern l, which we assume does not vary across sessions. Elements of can only be observed directly for individuals whose identity can be observed.
We used a standard Poisson half-normal observation model (e.g. Chandler and Royle 2013; Sollmann et al. 2013) where the expected detection rate is modelled as a decreasing function of the distance between the latent activity centres and the respective camera traps. We modelled the individual by trap by session encounter rate as follows:
where λ0 is the expected number of detections per occasion when the activity centre is located at the camera trap (si,m = xj) and σ is the scale parameter of the half-normal detection function, which determines how fast expected detections decline with an increasing distance from the activity centre.
Next, we define as the latent capture history of Individual i, at Trap j, during Sssion m, consisting of the number of detections summed over occasions. We model these capture histories as Poisson random variables where
Conditional on the true capture histories is a model to determine which samples provide an individual identity. We assumed that individuals with the same coat type have the same probability that their samples can be assigned an individual identity. We defined θl to be the probability that a sample from an individual with Coat type l will be assigned an individual identity (described as the ‘thinning rate’ in Jiménez et al. (2021)). Then, we defined as the capture history of cats that were able to be individually identified, and model it as a realisation of a binomial thinning process conditional on the true capture histories, , where
and is indexing the coat pattern of Individual i in Session m, .
We fit the model via Markov-chain Monte Carlo (MCMC) using NIMBLE ver. 0.12.2 (de Valpine et al. 2017) through R ver. 4.2.0 (R Core Team 2022) to sample from the posterior distributions of model parameters. We specified vague priors for all parameters as follows: D ~ Uniform (0,15), λ0 ~ Uniform (0,15), σ ~ Uniform (0,10), γ ~ Dirichlet (1,1,1,1,1,1,1), and θl ~ Uniform (0,1) for each of the coat types. We summarised parameters with medians and 95% highest posterior density intervals (HPDI). We ran five chains for 150,000 iterations, saving every 10th sample for analysis, and ensured the Gelman–Rubin convergence diagnostic (Gelman and Rubin 1992) 95% upper confidence limit was <1.1 for all parameters. We required two custom MCMC updates to deal with (1) the latent individual identities and (2) the unknown abundances.
Results
In total, 18,300 camera trap nights were completed over the course of the study, during which 211 feral cat detections were made. Feral cats were detected at 68% of camera trap sites. Of all detections, 18.9% (40) came from 18 cats that could be identified to the individual. The number of individuals detected across each survey session was consistent over the course of the study (Fig. 3). We also recorded 100 detections of black cats, 60 detections of tabby cats, 26 detections of black–white cats, four of ginger cats, five of black–ginger cats, eight detections of tabby–white cats and calico cats. The identification probabilities (thinning rates) of black (0.026 [95% HPDI 0.003, 0.061]) and tabby cats (0.214 [95% HPDI 0.125, 0.316]) were lowest, with the highest probabilities for black–ginger and one detection of a calico cat (Fig. 4, Table 1). Feral cat density was estimated to be 0.858 cats km−2 (95% HPDI 0.432, 1.385). Population sizes during the four periods were estimated to be similar, with posterior medians ranging from 197 to 202 individuals and 95% HPDIs ranging from 95 to 329 individuals (Table 1, Fig. 5).
Posterior distributions of number of individuals detected across the four survey periods. Black triangles = number of individuals identified. Black point intervals = posterior distributions with 95% HPDI’s.
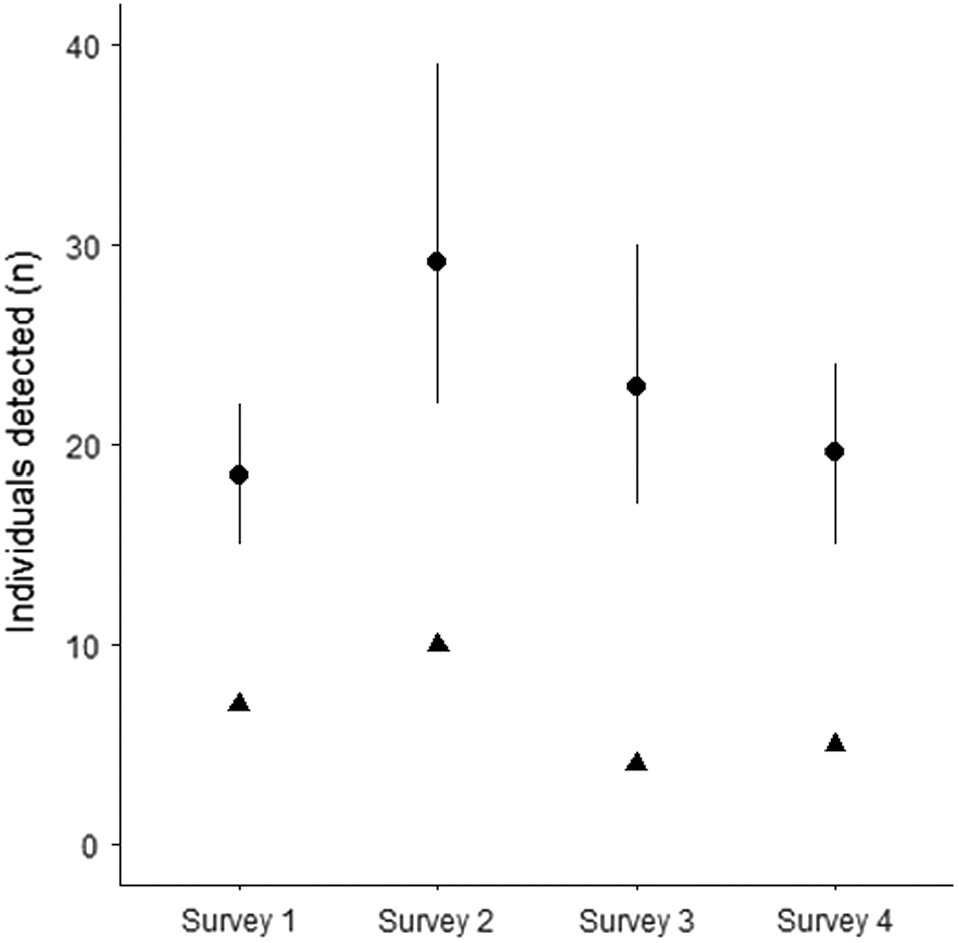
Parameter | Median | s.d. | 95% HPDI | Prior | |
---|---|---|---|---|---|
D | 0.858 | 0.251 | 0.432, 1.385 | U(0, 10) | |
N1 | 202 | 59.78 | 102, 329 | ||
N2 | 197 | 58.76 | 95, 318 | ||
N3 | 201 | 59.93 | 98, 325 | ||
N4 | 201 | 59.86 | 95, 322 | ||
λ0[1] | 0.0047 | 0.0016 | 0.0021, 0.0082 | U(0, 15) | |
λ0[2] | 0.0089 | 0.0023 | 0.0047, 0.0136 | U(0, 15) | |
λ0[3] | 0.0031 | 0.001 | 0.0014, 0.0053 | U(0, 15) | |
λ0[4] | 0.0024 | 0.0008 | 0.0011, 0.0042 | U(0, 15) | |
σ | 0.68 | 0.13 | 0.49, 0.973 | U(0, 10) | |
θblack–ginger | 0.841 | 0.163 | 0.474, 0.999 | U(0, 1) | |
θcalico | 0.839 | 0.164 | 0.472, 0.999 | U(0, 1) | |
θblack–white | 0.499 | 0.096 | 0.313, 0.688 | U(0, 1) | |
θtabby | 0.214 | 0.049 | 0.125, 0.316 | U(0, 1) | |
θtabby–white | 0.842 | 0.163 | 0.474, 0.999 | U(0, 1) | |
θginger | 0.685 | 0.178 | 0.328, 0.969 | U(0, 1) | |
θblack | 0.026 | 0.016 | 0.003, 0.061 | U(0, 1) |
D, density; N, abundance; λ, expected number of detections. Please refer to methods for detailed description of parameters.
Discussion
Our study applied a recently developed statistical approach to provide the first robust feral cat density estimate from a subtropical rainforest ecosystem in Australia. A large proportion (~80%) of detected cats in our study could not be identified to individual level, which precludes using typical SECR approaches to estimating density from all available detections. Here, we applied an approach to account for unknown individuals by using cat pelage type and detection histories to estimate individual identities for use in the model (Cove et al. 2023). This means that we were able to account for cats with homogeneous pelage types, for example, black cats and also cats that were not able to be identified because of posturing in the camera field of view, for example, some tabby cats, and others that did not display unique markings. Our results showed that the probability of identifying black and tabby cats is low when compared with that for other pelage types, which is not surprising because cats with more colour differentiation and unique features allow for easier identification of individuals. However, this was an important step to refine our feral cat density estimate.
The feral cat density in our study site was estimated to be 0.858 cats km−2 (95% HPDI: 0.432, 1.385), which is higher than the estimated continental average of 0.27 cats km−2 (95% CI 0.18, 0.45) (Legge et al. 2017). Cat density estimates from dense vegetation including rainforest were lacking when the continental cat density model was developed, but cats were considered rarer in these vegetation types (Legge et al. 2017). However, recent work has reported high cat occupancy from tropical rainforest ecosystems in northern Queensland (Bruce et al. 2022) and high feral cat densities in mesic eucalypt forest and cool temperate rainforests in south-eastern Australia (Rees et al. 2019). Taken together, these recent works and our study suggest that feral cats can have high densities within mesic ecosystems in eastern Australia.
The driver behind such a high density of feral cats is unclear. It may be driven by high densities of prey species and limited top-down pressure on the cat population from predators. The dingo (Canis familaris) was present in our study area (McHugh et al. 2019) and may influence feral cat dynamics and activity in some ecosystems throughout its broad distribution (Brook et al. 2012; Kennedy et al. 2012; Feit et al. 2019). However, a recent study that examined the dietary preference of the dingo in the Border Ranges NP, across our study area, suggested dingo has little influence on the cat population (McLean et al. 2023), which is consistent with other studies e.g. Fancourt et al. (2019).
Our study site within the Border Ranges has a high elevation (741–1046 m), receives a mean annual rainfall of ~1200 mm, and has fertile volcanic soils, causing high ecosystem productivity that supports an abundance and diversity of suitable feral cat prey species such as small mammals, birds and reptiles (Stobo-Wilson et al. 2022). In an observational note, McHugh and Robyns (2019) dissected a feral cat stomach from our study area and found five reptile species, including the threatened three-toed snake-tooth skink (Coeranoscincus reticulatus), golden-crowned snake (Cacophis squamulosus), small-eyed snake (Cryptophis nigrescens), marsh snake (Hemiaspis signata) and pink-tongued skink (Cyclodomorphus gerrardii), and one small mammal (Antechinus sp.). This demonstrates what a single cat can consume over a single day in our study area. A comprehensive meta-analysis of cat diet studies from across Australia (summarised in Stobo-Wilson et al. 2022) showed that a feral cat consumes an average of 2.2 mammals, birds and reptiles each day, or 791 animals per year. Given the density of cats in our study, this may translate to an overall annual cat predation toll of 641 animals per km2, or 2.35 million native animals killed by cats each year over the World Heritage Area (3665 km2). However, further empirical studies are required to accurately quantify the impact of feral cat predation and dietary preferences in our study area.
The relationship between high feral cat densities and the potential impact on at-risk native animal populations in our study area is unclear. In Australia cat-caused extinctions have generally clustered in more open, semi-arid and arid ecosystems rather than productive mesic ecosystems (Woinarski et al. 2019) and rainforest species are considered to be among those least affected (Hohnen et al. 2016; Woinarski et al. 2017). However, there are several reasons to be concerned about the potential effects of high feral cat densities in the Gondwanan Rainforests which we outline below.
First, many species of the Gondwanan Rainforests have restricted geographic distributions, and their populations could be quickly reduced by increases in cat predation (e.g. the nationally Endangered black-tailed antechinus (Antechinus arktos) and Tryon’s skink (Karma tryoni) (Riordan et al. 2020). Small mammals comprise ~70% of feral cat diet in tropical rainforest ecosystems (Rowland et al. 2020) that have similar ecological characteristics to those of our study site, and this coupled with the findings of Doherty et al. (2015) suggests that feral cats pose a great threat to narrow-range small mammals and reptiles within the rainforests. Second, high feral cat densities have been implicated in local extinctions of small mammals and ground-dwelling birds in productive tropical rainforest ecosystems that share similar characteristics with our study area (Lavery et al. 2020). Third, some at-risk species that occur in the Gondwanan Rainforests are susceptible to cat predation in other ecosystems, including threatened ground dwelling/nesting birds such as the eastern bristlebird (Dasyornis brachypterus) and the rufous scrub-bird (Atrichornis rufescens).
Feral cats co-exist with threatened medium-sized mammals, for example, long-nosed potoroo (Potorous tridactylus) and red-legged pademelon (Thylogale stigmatica) (McHugh et al. 2019; Miritis et al. 2020), suggesting that cat impacts on these threatened medium-sized mammals are of less concern. However, there are precedents for prolonged periods of co-existence of feral cats with native species that are abruptly ended when other factors (such as changes in rainfall or temperatures) contribute to, or interact with, cat predation, leading to population declines (Fancourt 2016). We recommend ongoing monitoring for populations of at-risk species where feral cat densities are known to be high.
Our study is the first to estimate feral cat density in a mesic subtropical rainforest ecosystem, showing a density being much higher than are average cat densities from other Australian ecosystems. Given the profound impacts of cats on Australian fauna from other ecosystems, monitoring the density of cats, other potential interacting threats, and trends in native animal populations, is critical for detecting signals of decline as early as possible. This would allow prompt intervention (e.g. with cat control programs) before local population extinctions occur in the Gondwana World Heritage Area. Cat density estimation has been challenging in areas with high proportions of unidentifiable cats, but camera trapping data coupled with the statistical approach employed here can be applied to such problematic feral cat data sets to refine density estimates.
Data availability
The data that support this study will be shared upon reasonable request to the corresponding author.
Conflicts of interest
Sarah Legge is an editor of Wildlife Research. To mitigate this potential conflict of interest they had no editor-level access to this manuscript during peer review. The author(s) have no further conflicts of interest to declare.
Declaration of funding
Data for this study were generated through the Burning–Hotspots–Gondwana threatened species and fire SOS partnership project that was supported by the New South Wales Environmental Trust. The authors did not receive any specific funding for the preparation of this paper.
Acknowledgements
We thank Kersten Tuckey at NSW Department of Climate Change, Energy, the Environment and Water for supporting our research. We thank Matthew Wiseman and Allan Goodwin from the New South Wales National Parks and Wildlife Service for providing logistical input to the project. We thank Ross Goldingay, Liam Bolitho, Sergio Jacomy, Jonathan Parkyn and Kersten Tuckey who helped with camera deployments. We thank Danielle Stokeld for her comments on an earlier version of the paper. We also appreciate the comments of two anonymous reviewers whose feedback helped improve this paper.
References
Augustine BC, Royle JA, Murphy SM, Chandler RB, Cox JJ, Kelly MJ (2019) Spatial capture–recapture for categorically marked populations with an application to genetic capture–recapture. Ecosphere 10, e02627.
| Crossref | Google Scholar |
Brook LA, Johnson CN, Ritchie EG (2012) Effects of predator control on behaviour of an apex predator and indirect consequences for mesopredator suppression. Journal of Applied Ecology 49, 1278-1286.
| Crossref | Google Scholar |
Bruce T, Williams SE, Amin R, L’Hotellier F, Hirsch BT (2022) Laying low: rugged lowland rainforest preferred by feral cats in the Australian Wet Tropics. Ecology and Evolution 12, e9105.
| Crossref | Google Scholar |
Buckmaster T, Dickman CR (2012) Feral cats in the tall forests of Far East Gippsland, Australia. In ‘Proceedings of the vertebrate pest conference’. (University of California) 10.5070/V425110488
Chandler RB, Royle JA (2013) Spatially explicit models for inference about density in unmarked or partially marked populations. The Annals of Applied Statistics 7, 936-954.
| Crossref | Google Scholar |
Chisholm R, Taylor R (2007) Null-hypothesis significance testing and the critical weight range for Australian mammals. Conservation Biology 21, 1641-1645.
| Crossref | Google Scholar | PubMed |
Cove MV, Herrmann V, Herrera DJ, Augustine BC, Flockhart DTT, McShea WJ (2023) Counting the capital’s cats: estimating drivers of abundance of free-roaming cats with a novel hierarchical model. Ecological Applications 33, e2790.
| Crossref | Google Scholar |
de Valpine P, Turek D, Paciorek CJ, Anderson-Bergman C, Lang DT, Bodik R (2017) Programming with models: writing statistical algorithms for general model structures with NIMBLE. Journal of Computational and Graphical Statistics 26, 403-413.
| Crossref | Google Scholar |
Department of Climate Change, Energy, the Environment and Water (2024) Available at https://datasets.seed.nsw.gov.au/dataset/nsw-state-vegetation-type-map
Doherty TS, Bengsen AJ, Davis RA (2014) A critical review of habitat use by feral cats and key directions for future research and management. Wildlife Research 41, 435-446.
| Crossref | Google Scholar |
Doherty TS, Davis RA, van Etten EJB, Algar D, Collier N, Dickman CR, Edwards G, Masters P, Palmer R, Robinson S (2015) A continental-scale analysis of feral cat diet in Australia. Journal of Biogeography 42, 964-975.
| Crossref | Google Scholar |
Doherty TS, Davis RA, van Etten EJB, Goldizen AW (2016) Impacts of the introduced predator, the feral cat, on Australian vertebrate wildlife. Mammal Review 46, 81-101.
| Google Scholar |
Fancourt BA (2016) Diagnosing species decline: a contextual review of threats, causes and future directions for management and conservation of the eastern quoll. Wildlife Research 43, 197-211.
| Crossref | Google Scholar |
Fancourt BA, Cremasco P, Wilson C, Gentle MN (2019) Do introduced apex predators suppress introduced mesopredators? A multiscale spatiotemporal study of dingoes and feral cats in Australia suggests not. Journal of Applied Ecology 56, 2584-2595.
| Crossref | Google Scholar |
Feit B, Feit A, Letnic M (2019) Apex predators decouple population dynamics between mesopredators and their prey. Ecosystems 22, 1606-1617.
| Crossref | Google Scholar |
Garrard GE, Kusmanoff AM, Faulkner R, Samarasekara CL, Gordon A, Johnstone A, Peterson IR, Torabi N, Wang Y, Bekessy SA (2020) Understanding Australia’s national feral cat control effort. Wildlife Research 47, 698-708.
| Crossref | Google Scholar |
Gelman A, Rubin DB (1992) Inference from iterative simulation using multiple sequences. Statistical Science 7(4), 457-472.
| Crossref | Google Scholar |
Hardman B, Moro D, Calver M (2016) Direct evidence implicates feral cat predation as the primary cause of failure of a mammal reintroduction programme. Ecological Management & Restoration 17, 152-158.
| Crossref | Google Scholar |
Hohnen R, Tuft K, McGregor HW, Legge S, Radford IJ, Johnson CN (2016) Occupancy of the invasive feral cat varies with habitat complexity. PLoS ONE 11, e0152520.
| Crossref | Google Scholar |
Jiménez J, Augustine BC, Linden DW, Chandler RB, Royle JA (2021) Spatial capture–recapture with random thinning for unidentified encounters. Ecology and Evolution 11, 1187-1198.
| Crossref | Google Scholar | PubMed |
Karanth KU, Chundawat RS, Nichols JD, Kumar NS (2004) Estimation of tiger densities in the tropical dry forests of Panna, central India, using photographic capture–recapture sampling. Animal Conservation 7, 285-290.
| Crossref | Google Scholar |
Kennedy M, Phillips BL, Legge S, Murphy SA, Faulkner RA (2012) Do dingoes suppress the activity of feral cats in northern Australia? Austral Ecology 37, 134-139.
| Crossref | Google Scholar |
Kooyman RM, Watson J, Wilf P (2020) Protect Australia’s Gondwana rainforests. Science 367, 1083.
| Crossref | Google Scholar |
Lavery TH, Alabai M, Holland P, Qaqara C, Vatohi N (2020) Feral cat abundance, density and activity in tropical island rainforests. Wildlife Research 47, 660-668.
| Crossref | Google Scholar |
Legge S, Murphy BP, McGregor H, Woinarski JCZ, Augusteyn J, Ballard G, et al. (2017) Enumerating a continental-scale threat: how many feral cats are in Australia? Biological Conservation 206, 293-303.
| Crossref | Google Scholar |
Legge S, Woinarski JCZ, Dickman CR, Doherty TS, McGregor H, Murphy BP (2020a) Cat ecology, impacts and management in Australia. Wildlife Research 47, i-vi.
| Crossref | Google Scholar |
Legge S, Murphy BP, McGregor HW, van Etten EJB, Berman D, Dickman CR (2020b) The impacts of feral cats on Australian wildlife and the implications for conservation management. Biological Conservation 244, 108476.
| Google Scholar |
McHugh D, Robyns L (2019) Coeranoscincus reticulatus (three-toed snake-tooth skink): predation. Herpetological Review 51, 329-330.
| Google Scholar |
McHugh D, Goldingay RL, Link J, Letnic M (2019) Habitat and introduced predators influence the occupancy of small threatened macropods in subtropical Australia. Ecology and Evolution 9, 6300-6317.
| Crossref | Google Scholar | PubMed |
McHugh D, Goldingay RL, Letnic M (2022) Occupancy and co-occurrence patterns of endemic mammals and introduced predators across a broad geographical gradient in eastern Australia. Biodiversity and Conservation 31, 989-1021.
| Crossref | Google Scholar |
McLean D, Goldingay R, Letnic M (2023) Diet of the Dingo in subtropical Australian forests: are small, threatened macropods at risk? Animals 13(14), 2257.
| Crossref | Google Scholar |
Medina FM, Bonnaud E, Vidal E, Tershy BR, Zavaleta ES, Josh Donlan C, et al. (2011) A global review of the impacts of invasive cats on island endangered vertebrates. Global Change Biology 17, 3503-3510.
| Crossref | Google Scholar |
Miritis V, Rendall AR, Doherty TS, Coetsee AL, Ritchie EG (2020) Living with the enemy: a threatened prey species coexisting with feral cats on a fox-free island. Wildlife Research 47, 633-642.
| Crossref | Google Scholar |
Nogales M, Vidal E, Medina FM, Bonnaud E, Tershy BR, Campbell KJ, Zavaleta ES (2013) Feral cats and biodiversity conservation: the urgent prioritisation of island management. BioScience 63, 804-810.
| Crossref | Google Scholar |
Rees MW, Pascoe JH, Wintle BA, Le Pla M, Birnbaum EK, Hradsky BA (2019) Unexpectedly high densities of feral cats in a rugged temperate forest. Biological Conservation 239, 108287.
| Crossref | Google Scholar |
Rich LN, Kelly MJ, Sollmann R, Noss AJ, Maffei L, Arispe RL, Paviolo A, De Angelo CD, Di Blanco YE, Di Bitetti MS (2014) Comparing capture–recapture, mark–resight, and spatial mark–resight models for estimating puma densities via camera traps. Journal of Mammalogy 95, 382-391.
| Crossref | Google Scholar |
Riordan CE, Pearce C, McDonald BJF, Gynther I, Baker AM (2020) Vegetation structure and ground cover attributes describe the occurrence of a newly discovered carnivorous marsupial on the Tweed Shield Volcano caldera, the endangered black-tailed dusky antechinus (Antechinus arktos). Ecology and Evolution 10, 2104-2121.
| Crossref | Google Scholar | PubMed |
Rowland J, Hoskin CJ, Burnett S (2020) Distribution and diet of feral cats (Felis catus) in the Wet Tropics of north-eastern Australia, with a focus on the upland rainforest. Wildlife Research 47(8), 648-659.
| Crossref | Google Scholar |
Singh R, Pandey P, Qureshi Q, Sankar K, Krausman PR, Goyal SP (2023) Estimating population density of leopards in semi-arid habitat, Western India. Proceedings of the National Institute of Ecology of the Republic of Korea 4, 72-78.
| Crossref | Google Scholar |
Soisalo MK, Cavalcanti SMC (2006) Estimating the density of a jaguar population in the Brazilian Pantanal using camera-traps and capture–recapture sampling in combination with GPS radio-telemetry. Biological Conservation 129, 487-496.
| Crossref | Google Scholar |
Sollmann R, Gardner B, Parsons AW, Stocking JJ, McClintock BT, Simons TR, Pollock KH, O’Connell AF (2013) A spatial mark–resight model augmented with telemetry data. Ecology 94, 553-559.
| Crossref | Google Scholar | PubMed |
Stobo-Wilson AM, Stokeld D, Einoder LD, Davies HF, Fisher A, Hill BM, Mahney T, Murphy BP, Stevens A, Woinarski JCZ, Bawinanga Rangers, Warddeken Rangers, Gilespie GR (2020) Habitat structural complexity explains patterns of feral cat and dingo occurrence in monsoonal Australia. Diversity and Distributions 26, 832-842.
| Crossref | Google Scholar |
Stobo-Wilson AM, Murphy BP, Legge SM, Caceres-Escobar H, Chapple DG, Crawford HM, Dawson SJ, Dickman CR, Doherty TS, Fleming PA, Garnett ST, Gentle M, Newsome TM, Palmer R, Rees MW, Ritchie EG, Speed J, Stuart J-M, Suarez-Castro AF, Thompson E, Tulloch A, Turpin JM, Woinarski JCZ (2022) Counting the bodies: estimating the numbers and spatial variation of Australian reptiles, birds and mammals killed by two invasive mesopredators. Diversity and Distributions 28, 976-991.
| Crossref | Google Scholar |
Sutherland C, Royle JA, Linden DW (2019) oSCR: a spatial capture–recapture R package for inference about spatial ecological processes. Ecography 42, 1459-1469.
| Crossref | Google Scholar |
Taggart PL, Peacock DE, Fancourt BA (2020) Camera trap flash-type does not influence the behaviour of feral cats (Felis catus). Australian Mammalogy 42, 220-222.
| Crossref | Google Scholar |
Thornton DH, Perkins CE (2015) Spatially explicit capture–recapture analysis of bobcat (Lynx rufus) density: implications for mesocarnivore monitoring. Wildlife Research 42, 394-404.
| Crossref | Google Scholar |
Trolle M, Kéry M (2003) Estimation of ocelot density in the pantanal using capture–recapture analysis of camera trapping data. Journal of Mammalogy 84, 607-614.
| Crossref | Google Scholar |
Woinarski JCZ, Woolley LA, Garnett ST, Legge SM, Murphy BP, Lawes MJ, Comer S, Dickman CR, Doherty TS, Edwards G, Nankivill A, Palmer R, Paton D (2017) Compilation and traits of Australian bird species killed by cats. Biological Conservation 216, 1-9.
| Crossref | Google Scholar |