Species-specific spatial and temporal variability in anuran call detection: implications for deploying autonomous recording units
Andrew Hall

A
B
C
Abstract
Ecosystem assessment using acoustic monitoring technologies can be an efficient method for determining species community composition and breeding activity, but many factors affect the quality of acoustics-data and subsequent level of confidence in derived inferences.
We aimed to assess variability in detection probabilities of five frog species using autonomous recording units (ARUs) deployed across a single 1 km2 wetland, comprising a lagoon and surrounding area, and subsequently determine the required number of ARUs with 95% confidence in derived presence–absence data.
Ten ARUs were deployed in two rings around the lagoon’s centroid close to the water’s edge. Occupancy models were used to derive detection probabilities of species calling in the lagoon from data describing the temporal pattern of calling at each site, which were derived using call recognition software.
Only two of the five target species were detected by all 10 ARUs. All target species’ non-zero ARU detection probabilities varied by a factor of 14, and the coefficients of variation in individual ARU detection probability for each species varied by a factor of seven. Simulations revealed seven or eight ARUs are required to achieve 95% confidence in confirming presence of either of the two species with the highest observed detection probabilities, given they are present and calling. Even with ten deployed ARUs, the probability of successful detection of the other three species known to be calling on any day was less than 40%.
Effective detection was not achieved for all targeted species by several ARUs during a period when hydrology and season suited recruitment activity. Despite all ARUs being deployed at locations favourable for detecting targeted species, stochastic factors drove spatial variability in detection resulting in markedly different data for each ARU and each species.
Data describing species presence derived from automated recording units may not be representative due to spatiotemporal variability in detection that varies by species. To improve ARU deployment strategies, a priori knowledge of typical detection probabilities and species spatial variability can be used to determine the required number of call recorders for a set level of confidence.
Keywords: ARU, call recorders, community composition, detection probability, frogs, occupancy, phenology, wetland, wildlife management.
Introduction
Autonomous recording units (ARUs) are widely deployed to monitor wildlife calling activity that would otherwise only be possible with skilled personnel undertaking extensive fieldwork. Semi-autonomous processing of the recorded audio provides fine scale data on species occupancy (e.g. Dorcas et al. 2009; Furnas and Callas 2015; Balantic and Donovan 2019), calling phenology (e.g. Schalk and Saenz 2016; Hoffmann and Mitchell 2022), and community composition (e.g. Ulloa et al. 2019; Melo et al. 2021). Autonomous recording units have advantages over traditional field-based surveys as they can be deployed for extended periods, capturing calling activity over an entire breeding season (Penman et al. 2005). Strategies for optimising audio data processing and improving ARU deployment efficiency are needed when seeking to maximise data quality. Improvements can include improved data collection, including combined data sources (Brinley Buckley et al. 2021) and more advanced audio data processing (Khalighifar et al. 2021). With their distinct advertisement calls, amphibians are ideal candidates for monitoring using ARUs. As the world’s most threatened group of vertebrates (Howard and Bickford 2014; Cordier et al. 2021), amphibian conservation action relies on accurate data describing spatiotemporal dynamics and breeding activity. While ARUs offer advantages over manual surveys for amphibians, particularly in terms of cost (Penman et al. 2005), each data collection method has its own biases and sensitivities. Data collection with ARUs must overcome technical challenges to ensure consistency and reliability. As is the case with ground-based surveys, quantification of detection probability is critical (Kalan et al. 2015; Clément et al. 2021). ARUs have some advantages over ground-based surveys provided that sources of error both in the field, such as declining microphone sensitivity (Turgeon et al. 2017), limited audio detection range (MacLaren et al. 2018), environmental factors on audio transmission (Muñoz and Halfwerk 2022), and identifying and accounting for software detection issues (false negatives and positives) (Swiston and Mennill 2009; Wimmer et al. 2013). The suitability of specific hardware elements, especially microphones, of ARUs is an additional consideration (Scott Brandes 2008), particularly for mobile animals, which may require multiple recorders to achieve required levels of detection.
For pond breeding amphibians, ARUs are typically placed at the edge of, or wholly within, the water body (e.g. Schalk and Saenz 2016; Ulloa et al. 2019; Melo et al. 2021). Large floodplain wetlands, typical of many inland waterways across the world, present additional challenges to the deployment of ARUs and analysis and interpretation of subsequent datasets. Floodplain wetlands are dynamic in space and time, often larger than the detection range of single ARUs, with spatially heterogeneous and variable water levels changing the shape and extent of habitat suitability for amphibians. Wetland vegetation in response to spatial variability in bathymetry and inundation frequencies, and soil subsequent characteristics, is similarly heterogeneous (Raulings et al. 2010; Szantoi et al. 2015), with different vegetation types and densities affecting call attenuation rates and detection ranges (Haupert et al. 2023), further complicating detection. How well calls carry over distance is a key consideration for ARU placement. For those calls that carry well, ARUs will have greater success at detecting species over wider areas (Leseberg et al. 2022). Furthermore, species mobility (Holloway and Miller 2017) adds further temporal and spatial variability in species presence within detection range of deployed ARUs.
Cues can be taken from species calls’ detection ranges and environmental factors, such as vegetation density and structure, topographic features, and knowledge of species’ preferences to place ARUs with a degree of improved performance. However, with many factors affecting call detection, some of which are stochastic, attempting to account for their combined effect on ARU deployment design to be confident in minimising false negative detection outcomes is generally unrealistic. Afterall, the set of detection probabilities for a set of target species will be unique at each ARU. In cases of monitoring a patch size that exceeds ARU detection radius, sufficient spatial coverage using multiple ARUs will likely be required to capture species diversity (e.g. Kalan et al. 2015). With knowledge of calling detection probabilities, i.e. the chance of detecting a species given that it is present and calling, design of ARU deployment to monitoring sites can be optimised, and a level of confidence in results quantified.
Creating an ARU array with reliable species detection for larger areas requires an estimate of the number of ARUs to reach a specified level of confidence in detection. The objective of this study was, therefore, to compare detection probabilities for a group of floodplain amphibian species, with a specific focus on testing the role of fine scale spatial and temporal variability in detection probability, and the subsequent number of recorders required to achieve a desired overall level of detection or else determine the percentage confidence in a result produced with fewer than optimal ARUs.
Materials and methods
Ten audio recording units (Song Meter SM4, Wildlife Acoustics Inc., Maynard Massachusetts), which are commonly used for monitoring and research (MacLaren et al. 2018), were deployed in approximately two concentric circles close to the edge of a 1 km2 wetland waterbody (Duck Lagoon in Murray Valley National Park, New South Wales, Fig. 1). Manufacturer’s default audio settings were applied to the ten SM4 Song Meters, except channel was set to zero to specify single channel (mono, rather than stereo) recording and preamplifier gain was set to 26 dB on ARUs 1–5 for a higher sensitivity to low-noise. Half the deployed ARUs (those with preamplifier gain enabled) were therefore set to more likely detect relatively quiet calls when there is little background noise, and the other half were set to detect louder calls during periods with more background noise. Table 1 summarises the deployed ARU settings. All call recorders were set to record simultaneously – every hour (a temporal resolution previously demonstrated in similar work by Sugai et al. (2021) to overcome diel variability in anuran calling) for a duration of five minutes following similar protocols for anuran acoustic monitoring (Walls et al. 2014; Anunciação et al. 2022) from 29 November 2016 until 1 May 2017 (153 Days), giving a total of 306 recording hours per unit.
Study location map, representative satellite imagery and digital terrain/bathymetric map (m AHD) of Duck Lagoon with call recorder locations overlaid (yellow dots). The boundary is defined as areas regularly inundated to be generally devoid of terrestrial vegetation.
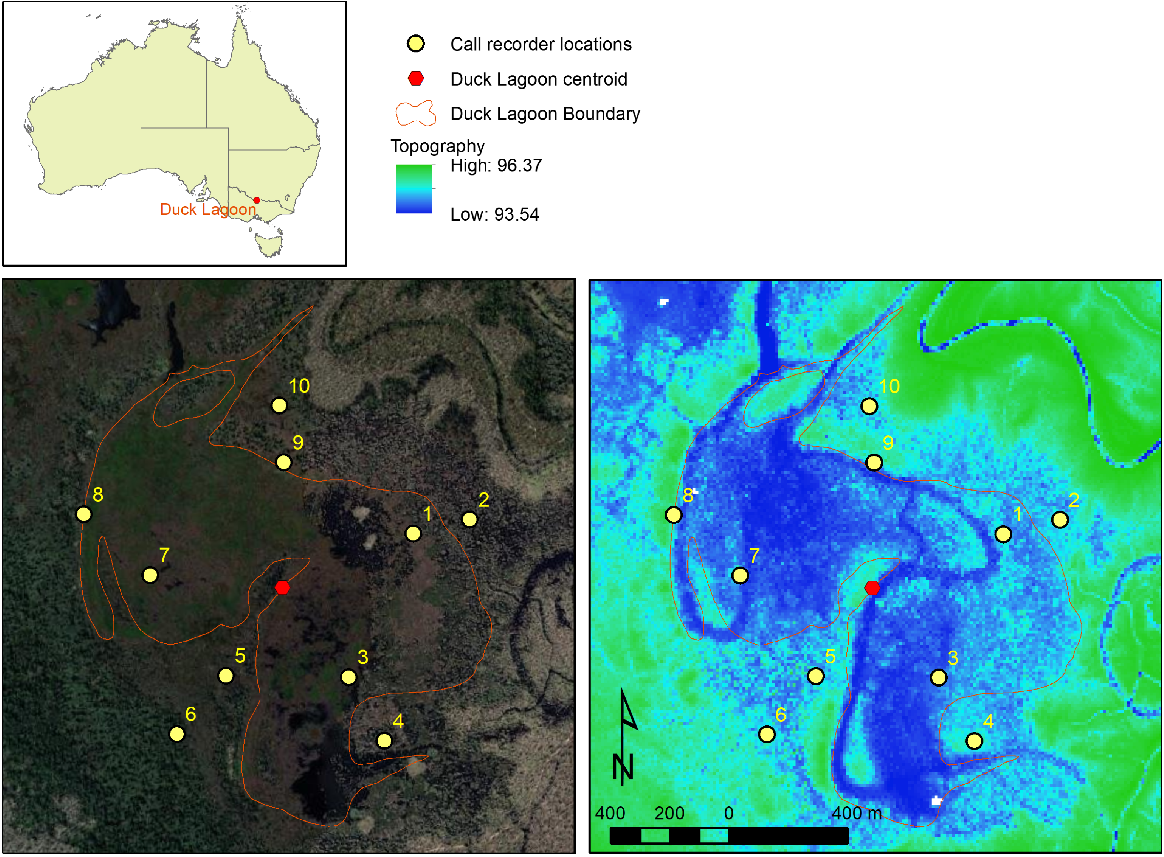
Parameter | ARUs 1–5 | ARUs 6–10 | |
---|---|---|---|
Channel | 0 (mono) | 0 (mono) | |
Gain | 16 dB | 16 dB | |
Filter | Off | Off | |
Sample rate | 24,000 Hz | 24,000 Hz | |
Preamplifier gain | 26 dB | Off |
Duck Lagoon was selected as it provided a suitable habitat for the target species and had a size and shape appropriate to the scale of the study. A stratified ARU deployment pattern was designed to maximise coverage of the patch with due consideration to optimising placement at the edge of the waterbody. Stratified deployment enables greater coverage of the patch by reducing areas with overlapping call radiuses, with ARUs at a distance of greater than 100 m considered independent in similar anuran communities (Mathwin 2024). Deployment at the edge of waterbodies has advantages in terms of detection for the five species considered here, due to their preference for shallower, well vegetated water which is more common at wetland edges. The centroid of Duck Lagoon was located, which served as the centre location of the ARU layout design. Using the zonal geometry tool of ArcGIS, the ‘thickness’ shape parameter (the radius of the largest circle that can be drawn within a shape, a useful objective base unit of distance from the centroid to the edge for any irregular 2-dimensional shape) of Duck Lagoon was calculated as 311 m. Starting at a random angle, five survey lines were drawn that radiated out from Duck Lake’s centroid at equidistant angles. Due to its irregular shape and wide transition zone between open water and terrestrial vegetation, 311 m from the centroid along each survey line tended more towards locations with open water. Therefore, initial sample locations were planned at both 311 m and 622 m from the centroid along each radiating survey line. Coordinates for the ten sample locations were prepared and identified in the field using handheld GPS units. The nearest suitable location for each call recorder was then identified, i.e. a location that enabled the call recorder to be set up that minimised the chances of being subject to damage or inundation. The actual location of the ARUs was recorded using the GPS. The shortest distance between any two deployed ARUs was 195 m, so we assumed there were no overlapping call radii. As the study was not concerned with accurate identification of abundance, but rather detection of calling in any part of the patch, there was no requirement to identify coincident recordings of more than one ARU of a single call. Nevertheless, the ARU deployment design allowed for a comparison of detection probabilities at outer ring locations to inner ring locations.
For five ARUs, microphone preamplifier gain was enabled. Pre-amplification enhances the volume and clarity of weak calls/sounds, but the recorders’ ability to accurately detect calls, with or without this setting, is unknown. At quiet volumes, recorded audio may exclude distant, or faint calls, as indicated by a lower total number of call detections; in these cases, pre-amplification would likely result in greater detection success. Calling at loud volumes, on the other hand, might saturate the recording, resulting in distortion known as digital clipping, potentially leading to inaccurate audio, which would be exacerbated by pre-amplification. Turning off pre-amplification may enhance automated recognition when exposed to comparatively louder environments.
Recognition models were built for each of the five frog species using exemplary calls derived from this dataset. All call detections were verified, and all inaccurate call detections were removed so that the dataset contained only true positive (accurate) call detections. The proportion of accurate species call detections is presented in Supplementary Table S1. Model sensitivities were tested and adjusted to improve the accuracy of the call detections and minimise false negative error (i.e. failure of the recognition model to detect a species call). To estimate the likelihood that the recognition model failed to detect a species call, call records were selected at random and checked for the target species call until a threshold margin of error of 0.08 was reached. Given that all call detections were checked (and therefore correct) and false negative error was consistently low across all sites and species, we have a high confidence in the dataset and the subsequent insight it provides.
We used occupancy models (MacKenzie et al. 2002) as implemented by the R-package unmarked ver. 1.2.5 (Fiske and Chandler 2011) in R ver. 4.1.3 (R Core Team 2022) to determine detection probabilities for each of the species at each site. The presence data for each species was organised in time series at a temporal resolution of 1 day, with the species recorded as present for at least one recognised call in any of the 24 h records for each day. Two covariates tested whether detection was influenced by being closer to the middle of the lagoon compared to closer to the edge or by the settings of the call recorder being set to high or low sensitivity (i.e. with or without pre-amplifier gain). Both covariates were described categorically in terms of each of the two possible cases: inner or outer for location, and low or high for sensitivity. For each species, four occupancy models were, therefore, produced for each combination of covariates, i.e. no covariates, sensitivity, location, and sensitivity + location. Other than the effect of the location and sensitivity covariates on detection, the call recorders were assumed identical in probability of detection. The Akaike Information Criterion (AIC) was used to select the most parsimonious model from the four for each species. Models were considered to have substantial support, given the data, where the difference in AIC between compared models was < 2 (Burnham and Anderson 2002).
A final model treated each call recorder as having its own individual detection probability, effectively taking into consideration all combined unique environmental factors that may influence frog calling activity and any physical characteristics of the devices themselves that may additionally influence detection. These individual detection probabilities and their confidence intervals (CI) were then used to simulate the probability of detection of each species on a single day, assuming the species was present and calling, using 1 to 10 (N) call recorders. With a small sample size of 10 or fewer, the confidence interval was expected to be wide; a 90% CI was selected over a 95% CI to provide narrower intervals and a more informative comparison. A Monte Carlo method was employed, whereby 10,000 sets of N call recorders were randomly selected (without replacement) from the pool of 10. For each selected call recorder, the logit probability and its corresponding standard deviation were used to produce a normal distribution from which a probability was randomly selected. For each of the 10,000 permutations, the overall probability of detection (PN) by at least one of the N selected devices was calculated as follows.
where pn is the randomly selected value from the normal distribution of the detection probability call recorder n from the set of N randomly selected devices.
Results
Across the study period there was recognisable partitioning in species detection (Fig. 2). Crinia parinsignifera was active in the first part of the study period (in late spring/early summer, from 11 November to December 25), reaching up to 20 identified calls per day. Also from the beginning of the study period (till 10 January), Litoria peronii was dominant with up to 100 calls identified across all 10 recorders per day. Limnodynastes fletcheri was the third species calling from the start of the study period, with up to 20 calls a day extending out to 6 March (late summer). Limnodynastes tasmaniensis was detected on 4 days with about 10 calls per day in mid-January and for a longer period from 2 February (mid-summer) with 1 to 4 calls per day detected to the end of the study period on 1 May (mid-autumn). Crinia signifera was the last species to start calling in late March (early autumn), with more calls per day detected from mid-April (mid-autumn) until the end of the study period. Two species known to occur in the region, Limnodynastes dumerilii and Neobatrachus sudellae (Howard et al. 2023), were not detected. We assumed that these species were not present at the survey site during the period of monitoring and that the anuran community for the purpose of this study comprised the five detected species.
Temporal pattern of calling intensity by each species over the duration of the study period. Calling intensity was calculated as identified calls per day summed for all 10 call recorders.
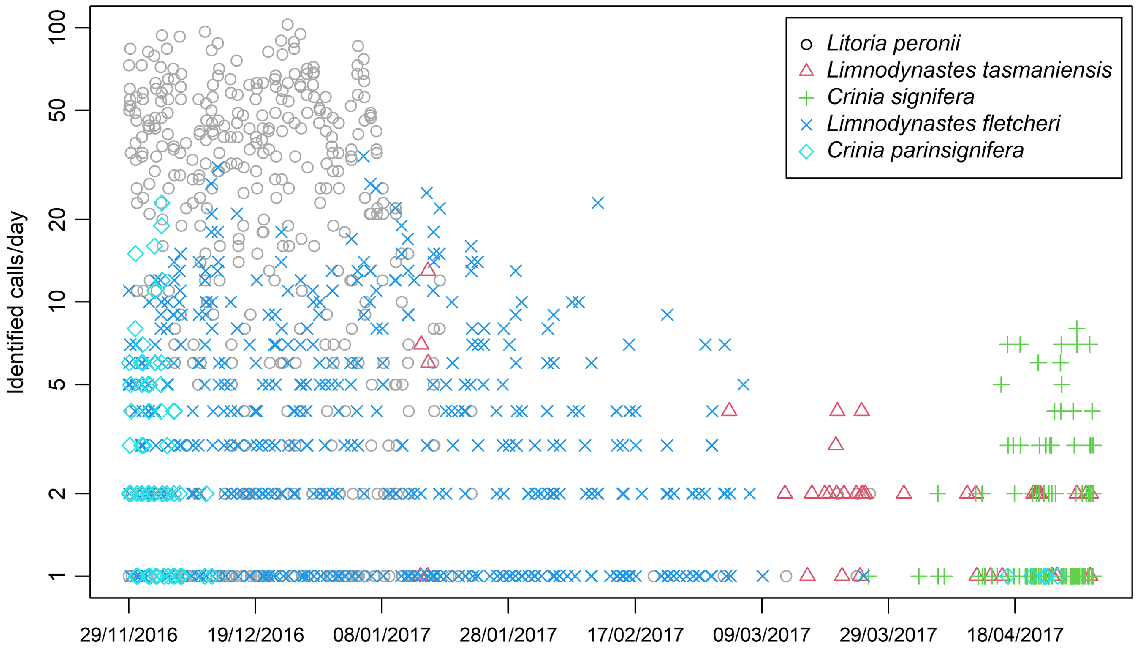
The number of calls detected varied considerably between recorder units and species. Litoria peronii and L. fletcheri, were detected by all 10 ARUs and had the highest number of detection days per ARU, 43.1 and 44.5, respectively. The number of ARUs detecting calls was lower for C. signifera which was detected on eight ARUs, but had a few (7.4 per ARU) detection days, while L. tasmaniensis was recorded on six of the ARUs (average of 8 detection days), and C. parinsignifera was recorded on just five of the ARUs, averaging 5.8 detection days. There was also variability in the community identified by each ARU, three of the 10 units detected all five species, three detected four species and remaining units detected three or fewer species.
The pattern of spatial variability in detection probabilities differed by species, except for ARUs 1 and 2, which had consistently higher levels of detection probabilities for all species, and ARUs 3 and 7, which had consistently lower levels of detection for all species. Only four ARUs detected all five species at some point during the study period. The pattern of spatial variability can be partially explained using the two detection covariates. For all species, except C. parinsignifera where the null model was supported, there were consistently lower detection probabilities when the pre-amplification setting was off (Fig. 3a). For every species other than C. parinsignifera, there was also significant differences between detection probabilities for those ARUs located towards the outer locations compared to inner locations (Fig. 3b). The occupancy models with the greatest level of support (Table 2) included the pre-amplification setting as a covariate for four of the five species (all except C. parinsignifera) and location as a covariate for both C. signifera and L. fletcheri.
Boxplots showing the differences in probability of detection for each species (a) between pre-amplification being on and off, and (b) between inner and outer locations of the lagoon.
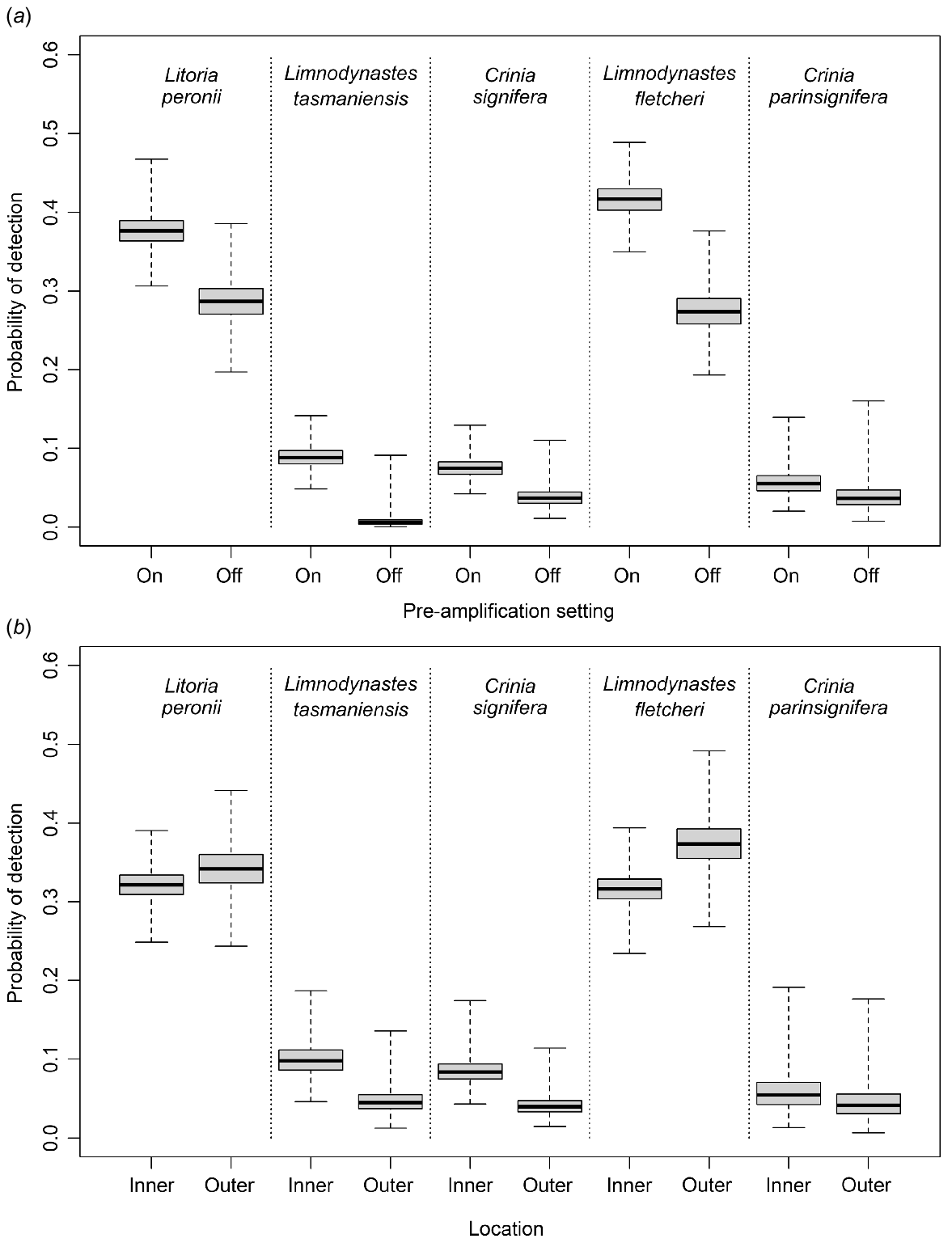
Species | Model | d.f. | logLik | AIC | ΔAIC to model with next lowest AIC | Weight | |
---|---|---|---|---|---|---|---|
Crinia parinsignifera | . | 2 | −121.69 | 249.1 | 3.08 | 0.73 | |
Crinia signifera | Location Sensitivity | 3 3 | −220.26 −221.12 | 450.5 452.2 | 1.72 2.30 | 0.54 0.23 | |
Limnodynastes fletcheri | Location + Sensitivity | 4 | −798.53 | 1613.1 | 4.88 | 0.92 | |
Limnodynastes tasmaniensis | Sensitivity | 3 | −173.50 | 357.0 | 5.78 | 0.95 | |
Litoria peronii | Sensitivity | 3 | −800.96 | 1611.9 | 3.77 | 0.85 |
ΔAIC is given for the difference in AIC between the named model and the next most supported model. For C. signifera, the top two models are given, as ΔAIC < 2. logLik is the log likelihood statistic and weight is the relative level of support compared to other models for that species (totalling 1).
Detection probabilities for each species were derived from respective occupancy models with greatest level of support. For any one ARU, the probability of recording at least one species’ call within a single day, given that it is calling somewhere in the study area is presented in Table 3. The modelled detection probabilities for 1 to 10 ARUs randomly deployed to each of the locations is illustrated for each species in Fig. 4. For the two species with relatively high detection probabilities, to have high confidence (>95%) in detecting at least one call across the study area in a given day, at least eight ARUs would need to be deployed for L. peronii and seven for L. fletcheri. For the three species with low detection probabilities, increasing the number of ARUs increased the overall probability of detection, but only reached 0.34 for L. tasmaniensis, 0.41 for C. signifera and 0.22 for C. parinsignifera with all ten ARUs. With all 10 ARUs deployed, following Kéry (2002, Eq. 4), the species would need to call for 8, 6 and 12 days within the study area to be 95% confident of detecting L. tasmaniensis, C. signifera and C. parinsignifera, respectively. In comparison, if only 1 ARU was deployed randomly at one of the 10 locations, the number of days of calling required for these three species to be 95% confident in detection would to 75, 58 and 119 days, respectively.
Species | Mean | s.e. | 5% confidence limit | 95% confidence limit | |
---|---|---|---|---|---|
Crinia parinsignifera | 0.0439 | 0.00820 | 0.0323 | 0.0596 | |
Crinia signifera | 0.0578 | 0.00749 | 0.0466 | 0.0714 | |
Limnodynastes fletcheri | 0.340 | 0.0135 | 0.318 | 0.3625 | |
Limnodynastes tasmaniensis | 0.0237 | 0.00788 | 0.0137 | 0.0408 | |
Litoria peronii | 0.330 | 0.0133 | 0.308 | 0.352 |
Given the species is calling somewhere within the defined study site area, the probability that a single ARU placed within the area will detect the species on a single day.
Modelled probability of detection across a 24-h period given the species is present and calling using 1–10 call recorders for (a) Litoria peronii, (b) Limnodynastes tasmaniensis, (c) Crinia signifera, (d) Limnodynastes fletcheri, and (e) Crinia parinsignifera.
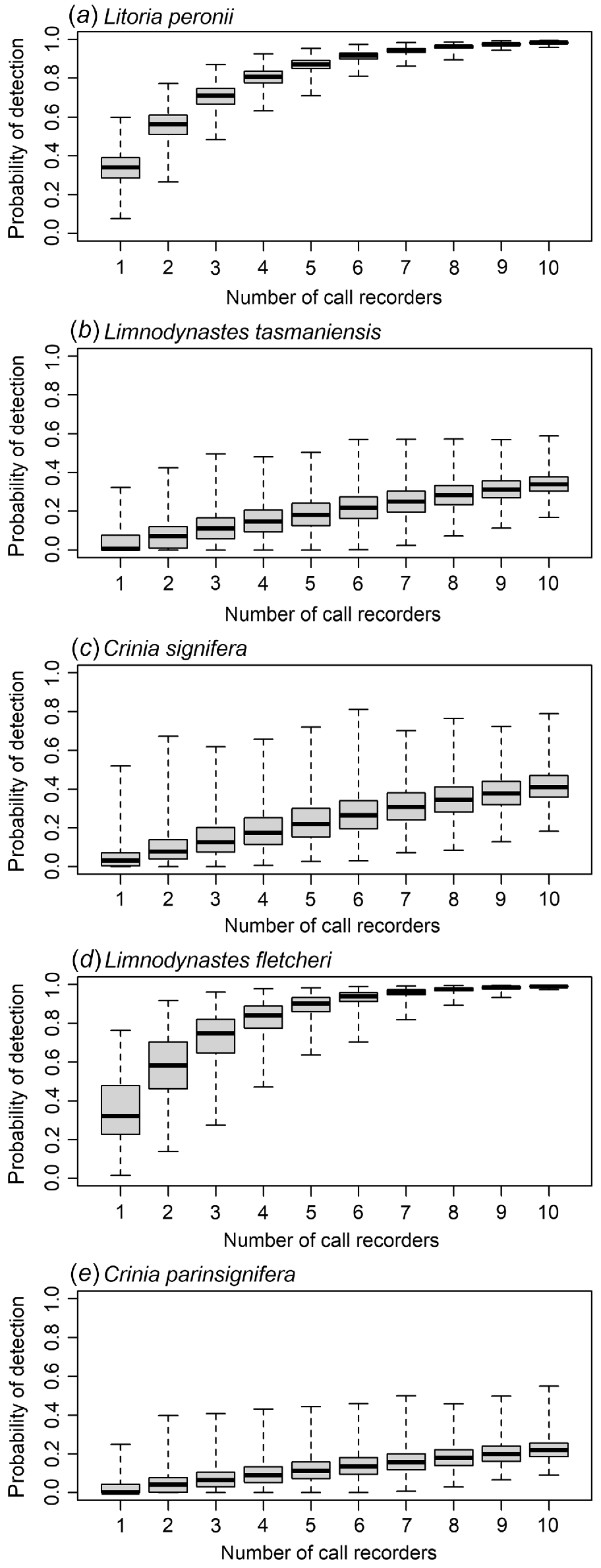
Detection probabilities (i.e. the probability of detecting a calling species within any of the 24 h 5-min recordings in a day) varied across the wetland area (Fig. 5) and by species. Litoria peronii exhibited a relatively consistent level of detectability across all ARUs, with a median estimate of detection probability ranging between 0.30 and 0.39 for nine of the ARUs and one outlier of 0.18 at ARU 7. Limnodynastes fletcheri, which had a similar level of overall detectability to L. peronii, had a more spatially variable pattern of detection with significantly greater detection probabilities at ARUs 1, 2 and 8 (0.64, 0.57 and 0.48) than ARUs 3, 6 and 7 (0.21, 0.21 and 0.06). In addition to lower levels of detection overall, the three other ground dwelling species also exhibited significant spatial variability in detection probability, including cases where detection probabilities of some species by specific ARUs were relatively high but did not detect other species at all. For example, at ARU 5, C. signifera had a detection probability of 0.34, whereas C. parinsignifera was not detected.
Mapped probability of detection at each ARU site for (a) Litoria peronii, (b) Limnodynastes tasmaniensis, (c) Crinia signifera, (d) Limnodynastes fletcheri, and (e) Crinia parinsignifera. Circle size indicates detection probability (thick line circles) and 90% confidence intervals (thin line circles).
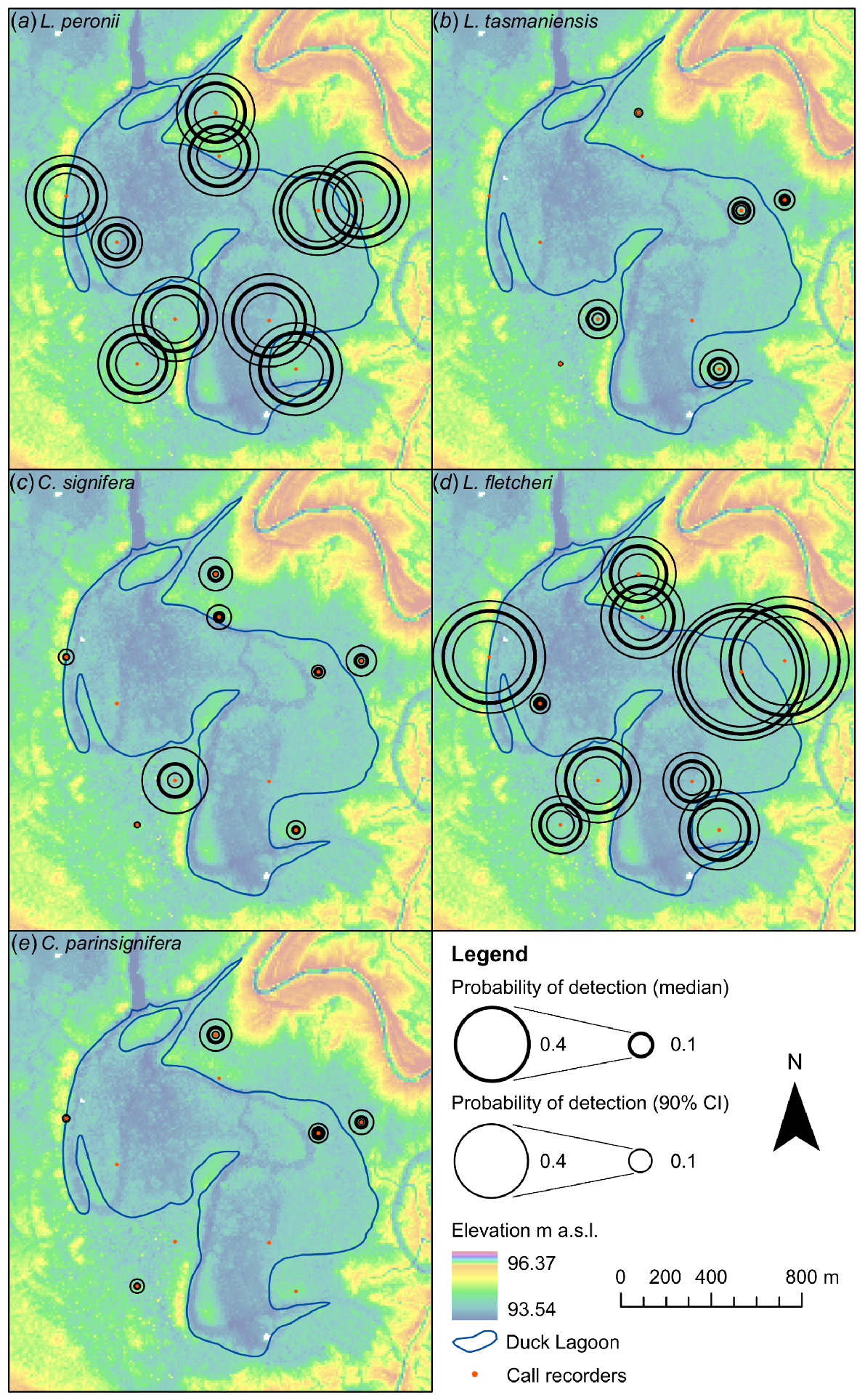
Discussion
Ecological monitoring is a crucial for effective species conservation but relies on survey methods that can accurately characterise species composition and activity across space and time. We found that detection probabilities are heterogenous across a single wetland complex, and that both the placement and settings of ARUs influence their probability of detecting amphibian species. We found that multiple ARUs are needed to accurately characterise a wetland amphibian community, and we developed a method for assessing how many ARUs are needed to confidently detect a given species.
The observed differences in detection probabilities indicated ARU placement significantly influenced detection probabilities of most of the target species across the wetland. If deploying a single ARU at the lagoon to monitor a species community, the inferred composition would have varied significantly depending upon the site selected. This outcome is consistent with previous studies that have indicated heterogeneity in species detection by ARUs (Kissling et al. 2010; Castro et al. 2019; Clément et al. 2021) but extends this knowledge by demonstrating their impact on detection data in a field setting. Many studies have previously examined the causes of detection heterogeneity. Our method uses detection probabilities to determine the number of ARUs needed to confidently assess true occupancy. This approach can be applied to similar ecological patches with species exhibiting comparable detection probabilities, providing robust data on species occupancy. By calculating the detection probabilities for each species, we were able to determine the number of ARUs needed to be 90% confident of detecting the species at the site. It should be noted that ARU placement was based on knowledge of where calling was most likely to be detected and not random. Each ARU deployed in this study was considered no more or less likely to detect the targeted species assemblage in locations chosen by the authors to have the best chance of success. Each unique monitoring mission should deploy ARUs similarly to maximise limited resources based on expert consideration of locations where target species are most likely to be detected. In cases where best monitoring locations are less well known, greater densities of ARUs will be required to compensate for lower detection probabilities at individual sites.
The extended five-month recording period used in this study was essential to detect all five target species, due to non-overlapping temporal windows of calling by some species, as previously established by Bridges and Dorcas (2000). The temporal pattern of species calling activity conformed with specific evidence of temporal partitioning for several of the target species (Heard et al. 2015). For investigations concerned with only characterising the species composition across the entire study period, given similar levels of detection observed in this study, it is unlikely a single ARU would detect all five species’ presence. A simple Monte Carlo simulation reveals that given a 30% chance of any one ARU detecting all five species, 9 ARUs would be required to reach 90% confidence that all species would be detected across the entire study period. If accurate daily data for phenological studies are required, then ARU deployment requirements must be increased by an order of magnitude. For 95% confidence in audio detection of at least one call (given the species is calling somewhere in the wetland) on each day, data would be unreliable unless eight ARUs are deployed for the two species with high detection probabilities, L. fletcheri and L. peronii. Impractically high number of ARUs would be required to detect reliable daily data for the three other species but this could be countered by decreasing the temporal resolution of the time series from daily to per 72 h or per week.
The higher sensitivity (pre-amplification) setting of the ARUs were associated with higher detection probabilities. Since records were produced every hour at each site, there would have been periods of relative lower volume during each of the day’s set of 24 5-min recordings. During periods of low calling intensity, the higher sensitivity setting could make identifying relatively quiet calls more likely. Using the lower sensitivity setting, on the other hand, may increase detection probabilities during hours of heavy calling and prevent the calls of less abundant species from being lost in the noise. Additional masking of recognisable calls during loud periods as detailed by Haupert et al. (2023) suggests that such periods may not be suited to detecting individual species calls. Fewer calls during periods of quieter ambient noise enables recording of clearer call signatures that will more likely result in a positive detection at higher levels of ARU sensitivity, which conforms with the expected general relationship between call detection and microphone sensitivity (Turgeon et al. 2017). It is reasonable to infer that for a situation in which records are collected regularly across a day, and the overall noise level varies between quiet and loud periods, the more favourable setting is to have increased sensitivity for the call recorder microphone in order to increase detection probability when concerned with simply identifying presence absence of a calling species on any single day, as was the case for the five species in this study.
This experiment was not designed to specifically explain the effect of environmental covariates on detection, such as nearby areas of open water or number of trees within a radius (which would have required a randomised design). ARUs were instead placed at relatively consistent locations close to the lagoon’s edge using the research team’s knowledge of where detection is more likely. Nevertheless, detection probability was higher in locations closer to the outer edge of the lagoon for two species and at locations closer to the centre of the lagoon for the three other species. The tree-dwelling species, L. peronii, had a higher detection probability near the outer edge, which could be explained by closer proximity to woodlands. Calls from higher elevations experience lower levels of sound attenuation due to less dense vegetation compared to areas closer to the ground, as demonstrated for bird calls (Thomas et al. 2020). Less sound attenuation for calls of tree-dwelling species is a possible explanatory factor for higher detection probabilities for L. peronii in this study. Based on these observations, if repeating a similar survey, further refinements to the deployment strategy would be made.
This study has shown that full coverage of a survey area to ensure detection based on objective consideration of sound attenuation, using stratified array such as that used by Kalan et al. (2015), was not necessary to determine community composition in this case. Six strategically placed ARUs in the 1 km2 area would have reliably detected the five target species, which had calling detection probabilities of 0.332, 0.048, 0.057, 0.345 and 0.048, with 95% confidence, given the same duration of sampling (153 days, December to April inclusive). This technique could be applied in similar studies monitoring community composition where there is a stochastic element to where species can be detected within large study site areas that exceed the detection range of the calling species. High spatial variability in ARU detection probabilities for some species highlights the need to qualify inferences made from ARU based surveys, particularly when inferring species absence. Determining and reporting detection probabilities derived from data collected by multiple ARUs within a habitat patch may improve the representativeness of community composition datasets.
Conclusions
Call recorders are a powerful tool capable of providing call activity data at fine temporal scales and over long periods. However, our data demonstrates that detectability can vary considerably even for relatively common and widespread species, and that care needs to be taken when interpreting data particularly when evaluating species occupancy and community composition derived from the deployment of single ARUs. Some of the spatial variability could be explained by local environmental factors: better detection for tree-dwelling species in the outer ring of ARUs closer to trees, better detection for ground-dwelling species in the ring of ARUs closer to the centre of the wetland, and poor detection at the ARU in the most homogeneous open-water location. We also found that a higher sensitivity setting produced higher detection probabilities for calling in any one day for all five study species. Since recordings were collected every hour, there were opportunities for detection of less common calls during relatively quiet periods that suited the higher sensitivity setting (although not statistically significantly higher for C. parinsignifera). Extending sampling periods beyond 5 min would likely improve detection probabilities during quieter periods. Longer sampling periods are becoming increasingly feasible with ongoing improvements in capacity for data storage and computer processing.
This study clearly indicated that a priori estimates of detection probabilities of species along with knowledge of calling phenology can greatly aid study design and ARU deployment. Similar to power analyses that determine required sampling effort to produce statistically significant outcomes, ARU deployment requirements can be similarly ascertained with the aid of Eqn 1. With a reasonable estimate of the expected detection probability, the number of ARUs required for a desired level of confidence in producing a true outcome in a particular location of interest can be estimated. The knowledge gained from this study on detection probabilities can guide refinements in ARU placement strategies. It also highlighted the importance of considering temporal variability in species’ calling periods and diel variability to guide ARU sensitivity settings to make future monitoring of anuran community more efficient.
Data availability
The data used to generate the results of the paper are available by emailing the corresponding author.
Declaration of funding
This project was funded through a grant from the Office of Environment and Heritage (NSW) Project Code 101928.
References
Anunciação PR, Sugai LSM, Martello F, de Carvalho LMT, Ribeiro MC (2022) Estimating the diversity of tropical anurans in fragmented landscapes with acoustic monitoring: lessons from a sampling sufficiency perspective. Biodiversity and Conservation 31(12), 3055-3074.
| Crossref | Google Scholar |
Balantic C, Donovan T (2019) Dynamic wildlife occupancy models using automated acoustic monitoring data. Ecological Applications 29(3), e01854.
| Crossref | Google Scholar | PubMed |
Bridges AS, Dorcas ME (2000) Temporal variation in anuran calling behavior: implications for surveys and monitoring programs. Copeia 2000(2), 587-592.
| Crossref | Google Scholar |
Brinley Buckley EM, Gottesman BL, Caven AJ, Harner MJ, Pijanowski BC (2021) Assessing ecological and environmental influences on boreal chorus frog (Pseudacris maculata) spring calling phenology using multimodal passive monitoring technologies. Ecological Indicators 121, 107171.
| Crossref | Google Scholar |
Castro I, De Rosa A, Priyadarshani N, Bradbury L, Marsland S (2019) Experimental test of birdcall detection by autonomous recorder units and by human observers using broadcast. Ecology and Evolution 9(5), 2376-2397.
| Crossref | Google Scholar | PubMed |
Clément M, Shonfield J, Bayne EM, Baldwin R, Barrett K (2021) Quantifying vocal activity and detection probability to inform survey methods for barred owls (Strix varia). Journal of Raptor Research 55(1), 45-55.
| Crossref | Google Scholar |
Cordier JM, Aguilar R, Lescano JN, Leynaud GC, Bonino A, Miloch D, Loyola R, Nori J (2021) A global assessment of amphibian and reptile responses to land-use changes. Biological Conservation 253, 108863.
| Crossref | Google Scholar |
Fiske I, Chandler R (2011) Unmarked: an R package for fitting hierarchical models of wildlife occurrence and abundance. Journal of Statistical Software 43(10), 1-23.
| Crossref | Google Scholar |
Furnas BJ, Callas RL (2015) Using automated recorders and occupancy models to monitor common forest birds across a large geographic region. The Journal of Wildlife Management 79(2), 325-337.
| Crossref | Google Scholar |
Haupert S, Sèbe F, Sueur J (2023) Physics-based model to predict the acoustic detection distance of terrestrial autonomous recording units over the diel cycle and across seasons: insights from an Alpine and a Neotropical forest. Methods in Ecology and Evolution 14(2), 614-630.
| Crossref | Google Scholar |
Heard GW, Canessa S, Parris KM (2015) Interspecific variation in the phenology of advertisement calling in a temperate Australian frog community. Ecology and Evolution 5(18), 3927-3938.
| Crossref | Google Scholar | PubMed |
Hoffmann EP, Mitchell NJ (2022) Breeding phenology of a terrestrial-breeding frog is associated with soil water potential: implications for conservation in a changing climate. Austral Ecology 47(2), 353-364.
| Crossref | Google Scholar |
Holloway P, Miller JA (2017) A quantitative synthesis of the movement concepts used within species distribution modelling. Ecological Modelling 356, 91-103.
| Crossref | Google Scholar |
Howard SD, Bickford DP (2014) Amphibians over the edge: silent extinction risk of data deficient species. Diversity and Distributions 20(7), 837-846.
| Crossref | Google Scholar |
Howard K, Durkin L, Scroggie M, Ward K (2023) The Living Murray–turtle and frog condition monitoring in Barmah–Millewa forest. Report for the 2022–2023 survey season. Arthur Rylah Institute for Environmental Research Technical Report Series No. 367, Heidelberg, Victoria. Available at https://www.mdba.gov.au/sites/default/files/publications/barmah-millewa-turtle-and-frog-condition-monitoring-report-2022-2023.pdf
Kalan AK, Mundry R, Wagner OJJ, Heinicke S, Boesch C, Kühl HS (2015) Towards the automated detection and occupancy estimation of primates using passive acoustic monitoring. Ecological Indicators 54, 217-226.
| Crossref | Google Scholar |
Kéry M (2002) Inferring the absence of a species – a case study of snakes. The Journal of Wildlife Management 66(2), 330-338.
| Crossref | Google Scholar |
Khalighifar A, Brown RM, Goyes Vallejos J, Peterson AT (2021) Deep learning improves acoustic biodiversity monitoring and new candidate forest frog species identification (genus Platymantis) in the Philippines. Biodiversity and Conservation 30(3), 643-657.
| Crossref | Google Scholar |
Kissling ML, Lewis SB, Pendleton G (2010) Factors influencing the detectability of forest owls in southeastern Alaska. The Condor 112(3), 539-548.
| Crossref | Google Scholar |
Leseberg NP, Venables WN, Murphy SA, Jackett NA, Watson JEM (2022) Accounting for both automated recording unit detection space and signal recognition performance in acoustic surveys: a protocol applied to the cryptic and critically endangered Night Parrot (Pezoporus occidentalis). Austral Ecology 47(2), 440-455.
| Crossref | Google Scholar |
MacKenzie DI, Nichols JD, Lachman GB, Droege S, Royle JA, Langtimm CA (2002) Estimating site occupancy rates when detection probabilities are less than one. Ecology 83(8), 2248-2255.
| Crossref | Google Scholar |
MacLaren AR, Crump PS, Royle JA, Forstner MRJ (2018) Observer-free experimental evaluation of habitat and distance effects on the detection of anuran and bird vocalizations. Ecology and Evolution 8(24), 12991-13003.
| Crossref | Google Scholar | PubMed |
Melo I, Llusia D, Bastos RP, Signorelli L (2021) Active or passive acoustic monitoring? Assessing methods to track anuran communities in tropical savanna wetlands. Ecological Indicators 132, 108305.
| Crossref | Google Scholar |
Muñoz MI, Halfwerk W (2022) Amplification of frog calls by reflective leaf substrates: implications for terrestrial and arboreal species. Bioacoustics 31(4), 490-503.
| Crossref | Google Scholar |
Penman TD, Lemckert FL, Mahony MJ (2005) A cost-benefit analysis of automated call recorders. Applied Herpetology 2(4), 389-400.
| Crossref | Google Scholar |
R Core Team (2022) ‘R: A language and environment for statistical computing.’ (R Foundation for Statistical Computing: Vienna, Austria) Available at https://www.R-project.org/
Raulings EJ, Morris K, Roache MC, Boon PI (2010) The importance of water regimes operating at small spatial scales for the diversity and structure of wetland vegetation. Freshwater Biology 55(3), 701-715.
| Crossref | Google Scholar |
Schalk CM, Saenz D (2016) Environmental drivers of anuran calling phenology in a seasonal neotropical ecosystem. Austral Ecology 41(1), 16-27.
| Crossref | Google Scholar |
Scott Brandes T (2008) Automated sound recording and analysis techniques for bird surveys and conservation. Bird Conservation International 18(S1), S163-S173.
| Crossref | Google Scholar |
Sugai LSM, Silva TSF, Llusia D, Siqueira T (2021) Drivers of assemblage-wide calling activity in tropical anurans and the role of temporal resolution. Journal of Animal Ecology 90(3), 673-684.
| Crossref | Google Scholar | PubMed |
Swiston KA, Mennill DJ (2009) Comparison of manual and automated methods for identifying target sounds in audio recordings of Pileated, Pale-billed, and putative Ivory-billed woodpeckers. Journal of Field Ornithology 80(1), 42-50.
| Crossref | Google Scholar |
Szantoi Z, Escobedo FJ, Abd-Elrahman A, Pearlstine L, Dewitt B, Smith S (2015) Classifying spatially heterogeneous wetland communities using machine learning algorithms and spectral and textural features. Environmental Monitoring and Assessment 187(5), 262.
| Crossref | Google Scholar | PubMed |
Thomas A, Speldewinde P, Roberts JD, Burbidge AH, Comer S (2020) If a bird calls, will we detect it? Factors that can influence the detectability of calls on automated recording units in field conditions. Emu - Austral Ornithology 120(3), 239-248.
| Crossref | Google Scholar |
Turgeon PJ, Van Wilgenburg SL, Drake KL (2017) Microphone variability and degradation: implications for monitoring programs employing autonomous recording units. [Variabilité et dégradation des microphones: implications pour les programmes de surveillance utilisant des unités d’enregistrement autonomes]. Avian Conservation and Ecology 12(1), 9.
| Crossref | Google Scholar |
Ulloa JS, Aubin T, Llusia D, Courtois ÉA, Fouquet A, Gaucher P, Pavoine S, Sueur J (2019) Explosive breeding in tropical anurans: environmental triggers, community composition and acoustic structure. BMC Ecology 19(1), 28.
| Crossref | Google Scholar | PubMed |
Walls SC, Hardin Waddle J, Barichivich WJ, Bartoszek IA, Brown ME, Hefner JM, Schuman MJ (2014) Anuran site occupancy and species richness as tools for evaluating restoration of a hydrologically-modified landscape. Wetlands Ecology and Management 22(6), 625-639.
| Crossref | Google Scholar |
Wimmer J, Towsey M, Roe P, Williamson I (2013) Sampling environmental acoustic recordings to determine bird species richness. Ecological Applications 23(6), 1419-1428.
| Crossref | Google Scholar | PubMed |