Updating the Australian digital soil texture mapping (Part 1*): re-calibration of field soil texture class centroids and description of a field soil texture conversion algorithm
Brendan Malone
A CSIRO Agriculture and Food, Clunies Ross Street, Black Mountain, ACT 2601, Australia.
B CSIRO Agriculture and Food, 306 Carmody Road, St Lucia, Qld 4067, Australia.
C Corresponding author. Email: brendan.malone@csiro.au
Soil Research 59(5) 419-434 https://doi.org/10.1071/SR20283
Submitted: 30 September 2020 Accepted: 11 February 2021 Published: 27 April 2021
Journal Compilation © CSIRO 2021 Open Access CC BY
Abstract
Soil texture (% sand, silt and clay sized particles) is one of the most important of soil characteristics affecting the function of soils. To better understand the behaviour of soils, reliable spatial estimates of soil texture need to be available. Digital soil mapping has been an enabler in delivering this sort of information. Delivered as two connected pieces, we present new efforts to update the soil texture maps for Australia (Version 1 was delivered in 2015). The main distinguishing enhancement is the merging of field descriptions of soil texture with the traditional laboratory analysed data. This greatly increases the number of available data, yet also calls for an elaboration of methods of how to convert texture class data into continuous variables, how to deal with the associated uncertainties of these conversions, and how these can be propagated in any sort of spatial modelling. Here we report on research to re-calibrate the soil texture centroids that were first determined by Minasny et al. (2007). Then we describe our approach on how the centroids and their uncertainty can be used to generate acceptable soil texture fractions for all qualitive soil profile texture descriptions in the Australian soil database.
Keywords: soil texture, digital soil mapping, spatial resolution, compositional data analysis, isometric log-ratio, soil function, soil variability, centroids
Introduction
Evaluating the functional characteristics of soils inevitably requires a study of its soil texture characteristics. Soil texture here is the proportion of sand, silt and clay sized particles that make up the mineral fraction of the soil. The relative proportions of these particle fractions largely determine how a soil behaves physically (Dexter 2004), chemically (Plante et al. 2006) and biogeochemically (Grandy et al. 2009; Haddix et al. 2020). Generally, understanding the storage, transport and cycling of water, gas phases and nutrients within soil at a given site requires a good understanding of soil texture.
It is not surprising therefore, that there has been a significant amount of research put into understanding what the spatial patterns of soil texture fractions are across spatial extents from field plots right up to the entire globe. Testament to this are organised and well-resourced national, continental and global programs to digitally map soil resources at these extents; e.g. Grundy et al. (2015), Ballabio et al. (2016) and Hengl et al. (2017), in which soil texture is possibly one of the most thoroughly explored.
The relative abundance of soil texture mapping as indicated above, together with other digital soil mapping activities around the world is indicative of the amount of soil texture information held within legacy soil databases (compared to other soil characteristics), despite it being a soil attribute that requires quite a lot of time consuming and diligent laboratory work. In this regard, here we make mention of only the laboratory analysed soil texture fractions, which are disproportionately less than the number of field texture observations that soil surveyors have made and exist in soil databases.
In the case of Australia, as of 2020 and largely thanks to ongoing coordination of harmonising soil databases from the different government jurisdictions, agencies and some private and public organisations, there are over 17 000 sites that have laboratory analysed soil texture information (Searle 2015). However, there are more than 150 000 sites that have soil texture class (STC) descriptors. These data are invaluable because they can potentially be used to infill spatial gaps and significantly enhance the fitting of spatial models. Moreover, these data could potentially be exploited for other more general use-cases such as mechanistic modelling and soil hydrological studies where some quantitative information regarding soil texture will be invaluable.
This last point is contingent upon proper handling of such data and to have methods that can deal with their imprecision and uncertainty relative to that of using only laboratory analysed data (which is not always error free either). Our efforts, specifically described in Malone and Searle (2021) (companion paper to the present study), are not the first nor will be the last to utilise field measured data in a digital soil mapping exercise. Efforts by Carlile et al. (2001) and Taylor and Minasny (2006) are demonstrative of this.
Common to those research contributions mentioned above is the use of soil texture centroids that summarise the basic statistical moments of STCs; namely the mean and median of the empirical distribution of the clay, silt and sand fractions of a given STC. Often the standard deviation and other statistical moments are also provided. Other approaches have also considered geometric methods where polygon shape analysis within a texture triangle was used to identify texture class centroids (Levi 2017).
Carlile et al. (2001) derived soil texture fraction summary statistics of six STCs using data stored in the Australian Soil Resource Information System (ASRIS; https://www.asris.csiro.au/index.html) at the time. These centroids were then used to estimate via weighted averaging (guided by information recorded in soil mapping unit metadata) the spatial patterns of the soil texture fractions across Australia. Taylor and Minasny (2006) using STC centroids derived by Minasny and McBratney (2001) attributed their STC allocations of numerous soil profile descriptions in order to apply ordinary kriging across two different vineyards at two depths (0–30 cm and 30–90 cm).
Further discussion about approaches to consider for digital soil mapping of soil texture fractions with respect to merging both laboratory and field measurements is provided in Malone and Searle (2021) (companion paper to this one). Moreover, that discussion considers an approach for the inclusion of uncertainty that comes with using field-based measurements, which the studies summarised above did not explicitly address.
In this study, we want to build on the STC centroid idea with the view that a method is developed whereby one can simulate plausible realisations of soil texture fractions down a given soil profile where STCs are recorded. The STC centroids and associated summary statistics are particularly important in this regard because they define the realm of possibilities of what the clay, sand and silt proportions might be at a given site and depth.
There is also much site contextual and field soil science knowledge to consider and embedded in the realm of possibilities too. For example, in the case of a synthetic, three horizon soil with sand, light clay and heavy clay texture classes assigned to each of the horizons respectively, the possibilities if we consider just the statistical properties of the centroids (means) and standard deviation, it is possible (though not very probable) to estimate texture fractions that make it less easy to distinguish the soil layers than what was probably observed in the field. Therefore, some sort of contextual information about ensuring the clay content of a light clay must be lower than that of a heavy clay in this synthetic soil example when plausible realisations are to be generated.
Similarly, soil texture qualifiers, which soil scientists use as an instrument to refine their classifications, represent further contextual information and not to be considered independently of a full soil profile characterisation either. Here, soil texture qualifiers refer to the practice of appending a ‘+’ or ‘–’ to a STC to specify either an increase or decrease respectively in clay content from the expected mean for that STC.
Another consideration is sub-plasticity, which Butler (1955) used to describe the consistence of certain soil materials appearing to have less clay than they actually contain. The contextual information required to evaluate whether one is dealing with a sub-plastic soil in this case will largely rely on drawing upon other pertinent data such as soil type classification, if it is available in the database.
There are potentially many other contextual data to draw upon like lithology and spatial position that will deliver some refinement in the simulation of plausible estimates, and these need to be specified where possible for the sake of repeatability and method improvement.
So the question is, why the need to generate plausible realisations? The answer is that this is a means to an end. It is our proposition that this approach will enable method development in merging laboratory and field measured data (with the intention of spatial modelling these data) that avoids just attributing centroid values only to texture classes. An approach that is cognisant of the within class variation is desired. The caveat is that this sort of analysis could potentially shine a light on work that scientists are probably not too comfortable about sharing. For example, errors from sources such as classification blunders, measurement errors, method differences between laboratories, method differences in general, and data input errors are just a few that exist and are managed in soil databases all round the world.
Even with the most stringent and careful methods of data filtering, imperfections and variability will always persist. Regardless of potential errors, the inherent variability within STC can be striking, and we would advocate for approaches that are responsive rather than be blind to this variability.
This method development of generating plausible realisations is a secondary aim of this research piece as it proceeds work of re-defining or updating STC centroids for Australia. This is needed as much more new data is now available to augment the work done by Minasny et al. (2007) whose work to date represents the most indicative summary of soil texture analysis for Australian soils. That work drew upon 19 500 soil samples from both ASRIS and Queensland Government. Each of these data had field texture observations and corresponding laboratory data of the clay, sand and silt fractions. Given the creation of the National Soil Site Collation (NSSC; Searle 2015), a much larger database is available and presents an opportunity to revisit Minasny et al. (2007) in order to augment and update work specifically about estimation of STC centroids and their associated statistical moments. Our querying of the NSSC resulted in finding over 50 000 observations with both field and laboratory data, which is inclusive of the data used in 2007.
Our overall aim is to consolidate and update the national mapping of soil texture for Australia. There is a layered approach to achieving this due to the need to incorporate soil texture class descriptions with laboratory analysed data into a spatial and quantitative modelling framework. In this paper, the first aim is to update the estimates of Australian STC centroids. The texture class centroids are of critical importance to next aim, which is to generate plausible clay, sand and silt fractions for whole soil profiles. This second aim is concerned with development of an algorithm that contextualises (rooted in field soil science principles) the simulation of soil texture fractions down a whole soil profile, yet also embodies all the variability and uncertainty that exists in the available data.
Materials and methods
The dataset
Data for Australian particle size fractions and field texture estimates were compiled from the National Soil Site Collation (NSSC). A total of 56 143 mineral soil samples had corresponding laboratory analysed soil particle-size data and field texture descriptions. Where available we also retrieved other data related to soil texture qualifiers (+ and –), which are an instrument soil surveyors’ have used to refine soil texture classification. Where recorded (52% of cases), particle size analyses have primarily been done by either the hydrometer method (29% of cases; Gee and Bauder 1986) or either pipette methods (16% of cases) from Coventry and Fett (1979) and Bowman and Hutka (2002). Relatively fewer samples were measured with the Plummet Balance method (7% of cases; Marshall 1956).
In the soil data compilation, soil texture fractions are represented as percentage mass of coarse sand (200–2000 µm), fine sand (20–200 µm), silt (2–20 µm) and clay (<2 µm) particles. Both coarse sand and fine sand were not recorded for every sample; instead, a composite sand fraction (20–2000 µm) was recorded. For this study, where applicable, we summed the coarse sand and fine sand fractions to generate a complete dataset of samples with clay, silt, and sand fractions. Some screening of the data entailed removing samples where the sum of the texture fractions was not greater than 90%. For samples where the sum of fractions was between 90% and 100% (non-inclusive), each fraction was normalised to sum to 100%. Further screening involved removing data where the occupancy to a texture class was low (<50 observations). This resulted in having a compiled dataset of soil texture fractions for 46 STCs, which covers only 54% of all observed STCs in Australia. Despite this seemingly low coverage, the data in these 46 STCs represent 99% of the cases in the compiled data. The texture classes are described in Table 1.
![]() |
To aid in achieving the second aim of this study, which is concerned with development of an algorithm that contextualises the simulation of soil texture fractions from STCs within a whole soil profile, we also matched each sample to its corresponding soil class where it was available. In the NSSC, the soil class information could be defined either in terms of the Great Soil Groups scheme (Stephens 1953), the Factual Key (Northcote 1979) or the Australian Soil Classification system (Isbell 2002) depending on when the soil sampling occurred (though many sites have been classified using more than one classification system).
Data analysis of compositional data
As soil texture is expressed in terms of relative abundances of different particle size fractions of the whole mineral soil mass, it is a compositional variable. Compositional data have properties that preclude the application of standard statistical techniques on such data in raw form. These include compositional data that are vectors of non-negative components showing the relative weight or importance of a set of parts in a total, meaning that the total sum of a compositional vector is considered irrelevant. Another property is that when analysing compositional data, no individual component can be interpreted in isolation from the other. For compositional data, the sample space (or set of possible values) is called the simplex, which is the set of vectors of positive (or zero) components that could be a described as a proportion, percentage or any other closed form expression such as parts per million (ppm). Because of these specific properties, compositional data are not amenable to analysis by common statistical methods designed for use with unconstrained data (Chayes 1960; Aitchison 1986). For example, standard techniques are designed to be used with data that are free to range from –∞ to +∞.
Aitchison (1986) introduced tools for appropriate treatment of compositional variables namely in the form of additive-log (alr) and centred-log-ratio (clr) transformations. As was demonstrated by Aitchison (1986), these transformations from the simplex to an n-dimensional Euclidean vector exhibit important properties that enables the data to be analysed in the same way as standard data. A soil texture composition of clay, sand and silt fractions would be considered as a three-part Aitchison-simplex and the alr-transform would map the composition non-isometrically to a 2-dimensional Euclidean vector, treating the last part as common denominator of the others. The isometry (or lack thereof with respect to alr-transformation) is a geometric concept about the association of angles and distances in the simplex (following the Aitchison geometry) to angles and distances in the Euclidean space. Because the mapping is done non-isometrically, this precludes using alr-transforms in distance-based data analytics, which a commonly used in pedometric applications such as soil entity allocations (Odgers et al. 2011). The other drawback of using alr-transforms is that by changing the part in the denominator, we obtain different alr transformations, which is likely to result in different analysis outcomes. The clr-transform maps a composition in the D-part Aitchison-simplex isometrically to a D-dimensional Euclidean vector subspace. Obviously, this is an advantage over alr-transformation; however, the transformation is not injective, resulting in the covariance matrices of the Euclidean variables to be always singular. Egozcue et al. (2003) identified the few shortcomings of both alr and clr transformations and proposed the isometric log-ratio transformation (ilr) to address these. The data in the D-1-dimensional Euclidean vector generated by ilr-transformation can be analysed in this space by classical multivariate analysis tools. However, the interpretation of the results may be difficult since there is no one-to-one relation between the original parts and the transformed variables.
All the data analysis performed in this research is done upon data subjected to ilr-transformation as this appears to most suitable in the context of work to be described about generating plausible soil profile texture fraction data from STCs. We note the caveats of using ilr-transformation and use this instrument merely for data analysis, and all data interpretations and summaries are informed by back-transformed data; i.e. data the original D-part simplex. Ultimately, doing data analysis in log ratio transforms of compositional data solves issues of data closure in that once the variables are back transformed, you are guaranteed of exact closure of the simplex; i.e. no leakage or shortfalls, which is a common phenomenon if the data were treated in a standard way.
For convenience, we step through the calculations required to convert a soil texture simplex to its ilr-transformed variables. Note that these calculations can be done using existing functions in our case from the R statistical software (R Core Team 2018) and the ‘compositions’ R package (van den Boogaart et al. 2018). Here, we will use the centroid for light clay that was defined in Minasny et al. (2007), which has the following components: clay, 40%; sand, 44%; silt, 16%.

V is a matrix of D rows and D-1 columns such that (identity matrix of D-1 elements and
, where a may be any value, and 1 is a matrix full of ones. From Egozcue et al. (2003), the matrix elements of V are the basis elements for the canonical basis of the clr-plane needed for the ilr transform. The default basis for a three-part simplex is:

The transpose of the basis is then multiplied by the clr-transform of the data. The clr-transform of the data is equated as:

From this, Eqn 1 is implemented as below

The inverse of ilr(x) is performed by converting ilr to clr then performing the inverse as for clr.

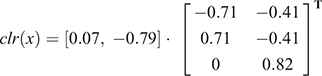

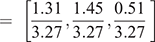

Derivation of soil texture class centroids
For each of the 46 STC groups, the mean, median, standard deviation and 10th and 90th percentiles were derived. The 20 STCs, studied in Minasny et al. (2007), were comparatively assessed to the same corresponding classes in this study.
Simulation of soil texture fractions
The first step to generate plausible soil texture characterisations for whole soil profiles is to simulate from the empirical distributions of the STCs. Ideally the generated simulations should collectively match the empirical distribution, which can be assessed by such metrics as the Kullback–Leibler (KL) divergence (Kullback and Leibler 1951). Also called relative entropy, which is related to Shannon’s information criterion (Shannon and Weaver 1949), KL divergence provides a measure of coherence between empirical distributions. The KL divergence can be computed as:

where Xi and Yi are the two distributions to be compared. In our case, Xi could be the empirical distribution of either clay, silt or sand, or their respective ilr-transforms for a specified texture class, meaning that Yi is the corresponding distribution that is simulated from some specified distribution. The KL divergence decreases towards zero as the empirical and sample distributions converge.
Two candidate sampling approaches were trialled to ensure the compositional properties of the data. The first was via Dirichlet random value simulation. This is a relatively simple algorithm where for each untransformed vector, independent gamma values are generated and divided by their sum. The inputs required for the Dirichlet simulation are the means the texture fractions of the specified STC. The results of this simulation (n, 1000) as illustrated for light clay soils in Fig. 1, which show a clear centering of the data about the mean but minimal correspondence to the original data as shown on the top left panel of Fig. 1. We will report on the KL-divergence values shortly after we introduce the second candidate sampling approach. This (the second approach) was performed using ilr-transformed data and the multivariate random sampling of specified normal distributions (Ripley 1987). In our case, this sampling is parameterised by the means of the D-1 ilr-transformed data (for the specified STC) and a positive definite symmetric matrix specifying the covariance matrix of these two variables. For the same light clay data, the multi-variate sampling (n, 1000) resulted in the data that is in Fig. 1, albeit after back-transformation to the simplex.
![]() |
Visually the multivariate sampling generates values that correspond to the actual data much better than using the Dirichlet simulation. This qualitive observation is confirmed by much smaller KL-divergence values for multivariate sampling compared to Dirichlet simulation. The data on the KL-divergence comparisons is not shown here specifically but available as online Supplementary material (see Table S1) along with the derived estimates for each of the other STCs. In all cases, multivariate sampling was a more superior simulation approach. While there was some variability in the relative differences in the KL-divergence values, this was put down to the small data size of some STCs. Similar plots to that shown in Fig. 1 are also available (see figures in Supplementary material 2) for each of the investigated STCs. Furthermore, the covariance matrices derived for each of the 46 STCs are shown in Supplementary Material 3 (.rds file).
Plausible soil texture characterisations for whole soil profiles
Simulation from the empirical distributions of the STCs is the fundamental mechanism needed to underpin plausible soil texture characterisations down a soil profile. A purely agnostic approach, i.e. one that is not underpinned by soil science concepts in the simulations, is to treat each soil layer in the profile independently and then just generate outcomes accordingly. Generating enough simulations and aggregating will result in the convergence towards means of the respective STCs in the profile, but a single outcome may not appear to be based on reality. This is due to the inherent variability of soil texture in each class. Therefore, a bespoke algorithm was developed to incorporate some basic concepts so that a single simulation for a whole layered soil profile would be first and foremost guided by the underlying variability of the empirical distributions but also have some basis. Naturally, any number of concepts could be considered, but we think the main principles are:
-
Classification into texture classes is underpinned by some field assessment of clay content of the soil. In the case of clays, one would expect, taking an example of three layers of a soil profile assigned light clay, medium clay and heavy clay respectively, to also have a measurable increase in clay content (an outcome of simulations) that respects this field observation. In general, when ordered correctly, there is linear increase in clay content with STC as shown visually in Minasny et al. (2007). Programmatically, this concept is handled by ordering the STCs in a profile from lightest to heaviest in terms of clay content. This was guided by the class centroids, and the sampling from one texture class to the next ensures that the clay content of the latter is measurably higher than the former. This is imposed simply by constraining the available sample space, to ensure that the clay content of the latter class does not get sampled from below the estimated clay content of the former texture class.
-
More than one or multiple observations of the same STC should have relatively similar soil texture values. Obviously if one considered the whole sample space of a STC, two separate samplings could result in wildly different values that does not fit well with what was probably observed in the field. Exploiting the Euclidean geometry that is given when working with ilr-transformed data, it is relatively straightforward to select from x-number of nearest neighbours to give relatively uniform soil texture fractions for each instance of a texture class in a profile. It is noted that there is an arbitrary decision to be made about how many nearest neighbours to choose from, or what proportion of the total sample can be made available to select from that could be considered near neighbours. In our case, the decision made was a conservative one in that of the 10 000 samples drawn from a given distribution, we would select a companion composition from 100 of the nearest neighbours to the originally selected sample.
-
Soil scientists can be very creative and many are seemingly abled to prescribe exacting soil texture fractions from a field observation. The instruments available to do this are clearly evident by the number of available STCs that can potentially be selected from, and further refined with the use of ‘+’ and ‘–’ texture qualifiers to marginally increase or decrease the texture content away from the considered mean. Rather than overlooking these refinements, they are harnessed in the algorithm as a logical decision whereby if there is an instance of a ‘+’ qualifier, the available sample space to choose from gets limited to the upper 60th percentile of the distribution. And the a ‘–’ qualifier the sample space gets reduced to the lower 40th percentile of the distribution. Qualifiers will override the considerations made in the previous point about relative uniformity of texture grades for multiple instances of a STC within a profile. Multiple instances of the same qualifier and texture class, however, retain those stipulations. The selection of a given percentile to limit the available sample space is again an arbitrary decision yet guided by underlying principles to ensure that the information observed in the field is in some way approximated through a plausible outcome.
-
Some preliminary data analysis of the 56 143 samples in correspondence with available soil classification information found there to be distinct differences in the overall centroids of a STC and those of soils that are known to exhibit sub-plastic properties. These soils include Ferrosols from Australian Soil Classification; Kraznozems, Euchrozems from the Great Soil Groups scheme and GN3.11, GN3.12, GN3.14, GN3.10 and GN3.17 from the Principal Profile Forms. There were 2284 samples that belonged to either of these classes, and as shown in Fig. 2, there is for some STCs, about a 10% increase in clay content of soils that are likely to display sub-plastic behaviour. This was found for the Clay Loams (CL) and clay soils. This relationship was indistinguishable or uninterpretable for the other STCs, which is why these results are not displayed here. Accounting for sub-plastic soils programmatically within the algorithm required drawing upon the available soil classification data together with the soil texture information, then adjusting the clay content of the sample after all the other prior processes have been implemented. This correction is essentially a last step of the algorithm where the adjustment (increase) made to the clay content (based on the corresponding correction for a given STC), is counterbalanced by subtracting from the sand and silt fractions in equal proportions.
The bespoke algorithm is designed to process through collections of soil profile data that are contained within databases. Notably this algorithm has been customised to work solely on Australian soils, but the general architecture would be extensible to other databases albeit after defining centroids for given STCs, and where applicable, incorporating soil classification information other than the various Australian systems. Currently, the algorithm used in this study is coded in the R programming language and is available from Github (https://github.com/AusSoilsDSM/SLGA/tree/master/SLGA/Development/soiltexture/morphological_conversion).
Plausible soil texture characterisations for whole soil profiles
In order to illustrate the efficacy of the whole soil texture profile algorithm, this was tested with four separate profiles that were generated synthetically. The first three profiles are possibly implausible but demonstrate the key functionalities of the algorithm in terms of considering the relative differences between STCs within a profile, the recognition of soil texture qualifiers and sub-plastic properties. The fourth profile is a plausible one, which is a texture contrast soil of a sandy topsoil over a clay subsoil. Each of the profiles is 1.6 m thick and has eight layers of 20 cm thickness. The specifics of each profile are summarised in Table 1.
Results and discussion
Derivation of soil texture class centroids
Table 2 contains the summary statistics for each of the 46 studied STCs in terms of the clay, silt and sand fractions. The data have been ordered according to the mean clay content. Figure 3 shows a plot of the centroid (median) clay % for each class. Comparing the soil texture fractions reported here with those previously done by Minasny et al. (2007), this newer work would be best described as a consolidation of that done previously. Table 3 provides side-by-side comparison of the STC centroids from each study where no striking differences are seen. This can also be observed in Fig. 3 with regard to clay content only. In most instances, there is a 1–2% difference in the texture fractions between both studies. The greatest differences were found for the medium heavy clay (MHC) and heavy clay (HC) STCs where a 3–4% difference in the clay % mean was observed. In terms of the new data studied here compared with Minasny et al.’s (2007), the number of soil samples for each STC increased, but the relative increase was much greater for the clayey soils. Therefore, the revised centroids for MHC and HC may be considered as being more reliable estimates.
![]() |
![]() |
Figure 4 shows a soil texture triangle with the centroids of each of the 46 soil classes plotted on it. This helps provide an overview of the relative differences and similarities between STCs. The markings are coloured up differently to highlight the 20 classes studied in both this research and Minasny et al. (2007) (red square markers) and just this research alone (blue round markers), which represent the other 26 classes. Except for the MHC class, 20 classes are those based on Northcote’s STCs (Northcote 1979) and appear to be the most used throughout Australia based on number of observations within the NSSC. The blue round markings are the other studied 26 STCs, which are predominantly from the classification by McDonald et al. (1990); this is in addition to some other ones of unknown origins. Clearly, these other texture classes provide coverage in the gaps brought about by using just the Northcote system. For example, where the clay content is 10–20% and where clay is > 40%, the additional classes provide some coverage in the blank spaces of the texture triangle. Figure 3 also illustrates this too, where gaps along the clay content gradient can be easily observed. In general, there are a high concentration of texture classes with relatively high sand contents, relatively few in the sandy loam area of 10–25% clay, before quite a dense number of classes in the clay loam region. This last observation is probably to be expected given that clay content may be relatively similar in STCs in this region of the texture triangle, but it is the relative abundances of silt and sand that better distinguish one class from another. For the STCs where clay content is >40%, there is a more open spread of the classes relative to other parts on the texture triangle, and the classes that are not of the Northcote System usefully provide an ability to differentiate soil texture classification with better precision if one is able to detect relative differences in sand and silt contents.
![]() |
A notable issue though is regarding the MHC and HC texture classes and the ability to differentiate between them in the field. Each class had more than 4000 observations attributed to each and there does not appear to be any evidence to suggest there is a difference between them based on the mean centroid value. Note from Table 1, there is a 1% difference between these classes when assessed based on the medians. As depicted in the data summaries in Table 2 and visually in the figures provided in Supplementary material 2, there is a lot of variability in each of these classes, which masks out any ability of differentiate them and poses the question of whether the MHC texture class is perhaps redundant.
As previously mentioned about the areas on the texture triangle where there is a high concentration of texture classes, there is other similar redundancy. A task for the future could be to integrate both soil texture systems to consolidate any redundancy and then perhaps assign new texture classes where clear gaps in coverage persist.
Some redefinitions of STCs has been proposed in Minasny and McBratney (2001) where it was proposed that Australia should adopt the USDA/FAO definition of silt size particles as 2–50 µm rather than the currently used system that defines silt as ranging 2–20 µm. This would give a more widely and evenly distribution of STCs, which is notable as one compares the USDA/FAO soil texture system with the International system that Australia uses for soil texture definitions. There have been some objections to this, largely based on field perceptions of texture (Marshall 2003), which has perhaps stalled any sort of re-consideration. Obviously, there is an opportunity to re-visit this discussion again into the future, yet this is beyond the scope of the present research and will not be considered any further.
Plausible soil texture characterisations for whole soil profiles
For each of the profiles, one simulation was done without using any contextual information, but rather just the multivariate random sampling. In Figs 5, 6, 7 and 8, this simulation is the topmost plot and will be described as the zero simulation henceforth. This provides a counterpoint to using the contextual information where three independent simulations using the soil profile algorithm are presented for each profile on the other three plots of Figs 5, 6, 7 and 8. The clay, sand and silt fractions are presented for each profile at each depth. Note that in a simulation exercise where one would envisage using converted field texture data, the number of simulations considered would be drastically increased. The selection of just three simulations per synthetic soil profile in these examples is to just give the reader a general understanding of the soil texture algorithm and the sorts of outputs it generates given the data that is entered into it, and an indication of the potential variability in the simulations from one realisation to the next.
![]() |
![]() |
![]() |
![]() |
Profile 1
This profile illustrates the realisations that can be attained where there is a gradational change in STCs from light to heavy. The zero simulation does a reasonable job on representing this change for this particular randomisation, but there is clearly an issue with how the relationship between the Clay Loam and Light Clay is presented, and from the field soil science perspective, would appear implausible. Simulations 1–3 appear as slight variants of each other, with each displaying and expected gradational profile in terms of clay and sand fractions.
Profile 2
This profile is probably the least interesting because there is no change in soil textures for the whole profile. Treating each layer independently, which is what is happening for the zero simulation, does generate a plausible result except perhaps for the two layers at 120–160 cm. Obviously, this is not an issue for the profiles generated by the algorithm. It could be argued though that the profile looks too uniform, but this can easily be addressed inside the algorithm by adjusting the number of nearest neighbours to sample from in terms of the multivariate distributions. Ultimately this is an arbitrary decision that expert oversight could address given the context and application for generating plausible soil texture profiles.
Profile 3
This profile embodies all the contextual information needed to generate plausible soil texture profiles. Clearly this contextual information; i.e. the soil texture qualifiers and modifications for sub-plastic properties is not captured in the zero simulation. The other simulations display soil profile characteristics that would be expected given the contextual information. That is, ‘–’ qualifiers having a texture lighter in clay content than the expected mean for that class (40–80 cm), and vice versa for the ‘+’ qualifier (80–120 cm). The soil layers with the sub-plastic properties (120–160 cm) have an incremental (~10% in fact) increase in clay content relative to the 0–40 cm layers. These features are by design, but as discussed about Profile 2, there are arbitrary decisions about sample location of the multivariate distribution to accommodate the texture qualifiers. Currently, this is set to the lower and upper 40th and 60th percentiles of the distributions, respectively. In the case of the sub-plastic properties, this is determined by the empirical finding of our analysis form the NSSC and the common soil types that are likely to have this property.
Profile 4
The texture contrast soil seems to be adequately represented with the zero simulation but may not be ideal in that there is a lot of variability in the layers with the same texture class. The soil profile algorithm corrects this issue where all three simulations are plausible outcomes. Our treatment of soil texture contrast soils in the soil profile algorithm is purely done based on the STCs. This is an adequate solution, particularly where there is a large increase in clay content between texture classes. But where the differences are not as substantial, it may become difficult to accommodate implied information when soil type is considered. The Australian Soil Classification (Isbell 2002) has three soil orders for soils that have soil texture contrasts between top and subsoil: Chromosols, Kurosols and Sodosols. These can be distinguished by differences in the subsoil pH and base cation status to be placed in either of these classes. But in order to be classified into either one of these classes criteria, around ‘Clear or abrupt textural B horizon’ need to be met. There are two stipulations:
-
If the clay content of the material above the clear, abrupt or sharp boundary is less than 20%, (and/or has a field texture of sandy loam or less) then the clay content immediately below must be at least twice as high. However, there must be a minimum of 20% clay (and/or a minimum field texture of sandy clay loam) at the top of the B horizon.
-
If the material above the transition has 20% clay or more but less than 35% clay (and/or has a field texture of sandy clay loam or greater but less than light clay), then the material below must show an absolute increase of at least 20% clay; e.g. 25% increasing clearly, sharply or abruptly to at least 45%, (and/or a field texture of light medium clay or greater). Note that a clear or abrupt textural change is not allowed within the clay range.
It is possible to accommodate for this contextual information by considering the soil classification for a site, and then guiding the sampling from the multivariate distributions that would ensure meeting the requirements of the texture contrast stipulations. Obviously, these concessions are purely focused on Australian soils, but highlight further modifications that could be made to the algorithm to accommodate any contextual information that is thought to be relevant. In the present research, we have tried to design the algorithm to make it as general as possible and to suit varied applications, although we acknowledge that the basic analysis structure is configured to process data from the NSSC efficiently and would need to be modified for another soil database.
Conclusions
The overarching aim of this research is to develop an approach that permits the incorporation of field soil texture data in quantitative and statistical analyses. Our immediate use-case is to allow for the integration of field soil texture observations together with laboratory soil texture observations into a digital soil mapping framework. The general solution to realise this objective is to convert STC data into continuous qualities of clay, silt and sand fractions. Rather than approaching this by just using the centroid value of the texture class as has been done in the past, our approach generates plausible realisations given available information from a soil database where there is corresponding laboratory and field data. In our case, we were able to exploit the updated version of the Australian Soil Site Collation which contained more than 50 000 cases of STC information and associated laboratory data of the texture fractions. In doing this, we were able to consolidate existing soil texture centroids for 20 classes and generated new ones for a further 26 classes.
We then developed an algorithm that can generate plausible soil texture profiles that at its core, is informed by the STC centroids. The unique aspect of the algorithm is that simulations are made by sampling from the empirical distribution soil texture fraction data that summarises each STC. This sampling acknowledges the compositional properties of the soil texture information such that the multivariate sampling is done with data transformed via the isometric log-ratio transformation. The algorithm was further customised to accommodate soil contextual information to ensure there was some coherence between field observation and simulated data. Our results, illustrated with four synthetic soil profiles, demonstrate these accommodations with examples highlighting gradational clay content increases down a soil profile, and adjustments for soil texture qualifiers and sub-plastic properties. We also alluded to further contextual accommodations around texture contrast soils to illustrate where further developments in the algorithm could be made if needed, or to instigate discussion around other sorts of accommodations.
We see this algorithm being an important instrument for unlocking the potential of field soil survey information for better understanding of soil heterogeneity across given spatial extents. For soil texture in Australia, the differential between sites that have laboratory analysed data and only field observed STC information is upwards of 100 000. A significantly high number of field observation relative to laboratory data exist. The situation would be similar in other parts of the world too.
The algorithm that has been developed in this study should be a useful instrument to realise the potential of these under-utilised data in future digital soil mapping efforts, and other soil science applications in general where numerical representations of soil texture information is required such as pedo-transfer functions. For example, in the development of realistic inputs for calculation of plant available water content using pedo-transfer function where soil textural datasets will be an input. And realistic inputs for water balance models based on DSM gridded datasets as input datasets.
Conflict of interest
The authors declare no competing interests.
Declaration of funding
The authors acknowledge the Terrestrial Ecosystem Research Network (TERN), an Australian Government NCRIS-enabled research infrastructure project, for facilitating and supporting this research.
Acknowledgments
The authors acknowledge CSIRO colleagues Mark Thomas and Senani Karunaratne for their expert analysis and review of earlier drafts of this manuscript.
References
Aitchison J (1986) ‘The Statistical Analysis of Compositional Data.’ (Chapman & Hall: London)Ballabio C, Panagos P, Monatanarella L (2016) Mapping topsoil physical properties at European scale using the LUCAS database. Geoderma 261, 110–123.
| Mapping topsoil physical properties at European scale using the LUCAS database.Crossref | GoogleScholarGoogle Scholar |
Bowman G, Hutka J (2002) Particle size analysis. In ‘Soil physical measurement and interpretation for land evaluation’. (Eds NJ McKenzie, K Coughlan, HP Cresswell.) pp 224–239. (CSIRO Publishing: Melbourne, Vic)
Butler BE (1955) A system for the description of soil structure and consistence in the field. Journal of the Australian Institute of Agricultural Science 24, 239–249.
Carlile P, Bui E, Moran C, Minasny B, McBratney AB (2001) Estimating soil particle size distributions and percent sand, silt and clay for six texture classes using the Australian Soil Resource Information System point database. CSIRO Land and Water Technical Report 29/01: Canberra.
Chayes F (1960) On correlation between variables of constant sum. Journal of Geophysical Research (1896–1977) 65, 4185–4193.
| On correlation between variables of constant sum.Crossref | GoogleScholarGoogle Scholar |
Coventry RJ, Fett DER (1979) A pipette and sieve method of particle‐size analysis and some observations on its efficacy. CSIRO Australia, Division of Soils, Divisional Report No. 38: Australia.
Dexter AR (2004) Soil physical quality: Part I. Theory, effects of soil texture, density, and organic matter, and effects on root growth. Geoderma 120, 201–214.
| Soil physical quality: Part I. Theory, effects of soil texture, density, and organic matter, and effects on root growth.Crossref | GoogleScholarGoogle Scholar |
Egozcue JJ, Pawlowsky-Glahn V, Mateu-Figueras G, Barceló-Vidal C (2003) Isometric Logratio Transformations for Compositional Data Analysis. Mathematical Geology 35, 279–300.
| Isometric Logratio Transformations for Compositional Data Analysis.Crossref | GoogleScholarGoogle Scholar |
Gee GW, Bauder JW (1986) Particle-size analysis. In ‘Methods of soil analysis. Part 1. 2nd ed’. (Ed. A Klute.) pp. 382–411. (ASA and SSSA: Madison, WI)
Grandy AS, Strickland MS, Lauber CL, Bradford MA, Fierer N (2009) The influence of microbial communities, management, and soil texture on soil organic matter chemistry. Geoderma 150, 278–286.
| The influence of microbial communities, management, and soil texture on soil organic matter chemistry.Crossref | GoogleScholarGoogle Scholar |
Grundy MJ, Rossel RAV, Searle RD, Wilson PL, Chen C, Gregory LJ (2015) Soil and Landscape Grid of Australia. Soil Research 53, 835–844.
| Soil and Landscape Grid of Australia.Crossref | GoogleScholarGoogle Scholar |
Haddix ML, Gregorich EG, Helgason BL, Janzen H, Ellert BH, Francesca Cotrufo M (2020) Climate, carbon content, and soil texture control the independent formation and persistence of particulate and mineral-associated organic matter in soil. Geoderma 363, 114160
| Climate, carbon content, and soil texture control the independent formation and persistence of particulate and mineral-associated organic matter in soil.Crossref | GoogleScholarGoogle Scholar |
Hengl T, Mendes de Jesus J, Heuvelink GBM, Ruiperez Gonzalez M, Kilibarda M, Blagotić A, Shangguan W, Wright MN, Geng X, Bauer-Marschallinger B, Guevara MA, Vargas R, MacMillan RA, Batjes NH, Leenaars JGB, Ribeiro E, Wheeler I, Mantel S, Kempen B (2017) SoilGrids250m: Global gridded soil information based on machine learning. PLoS One 12, e0169748
| SoilGrids250m: Global gridded soil information based on machine learning.Crossref | GoogleScholarGoogle Scholar | 28207752PubMed |
Isbell RF (2002) ‘The Australian Soil Classification’. (CSIRO Publishing: Melbourne.)
Kullback S, Leibler RA (1951) On information and sufficiency. The Annals of Mathematical Statistics 22, 79–86.
Levi MR (2017) Modified Centroid for Estimating Sand, Silt, and Clay from Soil Texture Class. Soil Science Society of America Journal 81, 578–588.
| Modified Centroid for Estimating Sand, Silt, and Clay from Soil Texture Class.Crossref | GoogleScholarGoogle Scholar |
Malone BP, Searle R (2021) Updating the Australian digital soil texture mapping (Part 2): spatial modelling of merged field and lab measurements. Soil Research
| Updating the Australian digital soil texture mapping (Part 2): spatial modelling of merged field and lab measurements.Crossref | GoogleScholarGoogle Scholar |
Marshall TJ (1956) A plummet balance for measuring the size distribution of soil particles. The Journal of Applied Science 7, 142–147.
Marshall TJ (2003) Particle-size distribution of soil and the perception of texture. Soil Research 41, 245–249.
| Particle-size distribution of soil and the perception of texture.Crossref | GoogleScholarGoogle Scholar |
McDonald RC, Isbell RF, Speight JG, Walker J, Hopkins MS (1990) ‘Australian soil and land survey field handbook.’ 2nd edn (Inkata Press: Melbourne)
Minasny B, McBratney AB (2001) The Australian soil texture boomerang: a comparison of the Australian and USDA/FAO soil particle-size classification systems. Soil Research 39, 1443–1451.
| The Australian soil texture boomerang: a comparison of the Australian and USDA/FAO soil particle-size classification systems.Crossref | GoogleScholarGoogle Scholar |
Minasny B, McBratney AB, Field DJ, Tranter G, McKenzie NJ, Brough DM (2007) Relationships between field texture and particle-size distribution in Australia and their implications. Soil Research 45, 428–437.
| Relationships between field texture and particle-size distribution in Australia and their implications.Crossref | GoogleScholarGoogle Scholar |
Northcote KH (1979) A factual key for the recognition of Australian soils. (Rellim Technical Publications)
Odgers NP, McBratney AB, Minasny B (2011) Bottom-up digital soil mapping. I. Soil layer classes. Geoderma 163, 38–44.
| Bottom-up digital soil mapping. I. Soil layer classes.Crossref | GoogleScholarGoogle Scholar |
Plante AF, Conant RT, Stewart CE, Paustian K, Six J (2006) Impact of Soil Texture on the Distribution of Soil Organic Matter in Physical and Chemical Fractions. Soil Science Society of America Journal 70, 287–296.
| Impact of Soil Texture on the Distribution of Soil Organic Matter in Physical and Chemical Fractions.Crossref | GoogleScholarGoogle Scholar |
R Core Team (2018) 'R: A Language and Environment for Statistical Computing.' (R Foundation for Statistical Computing: Vienna, Austria)
Ripley BD (1987) ‘Stochastic Simulation. Wiley Series in Probability and Statistics.’ (John Wiley & Sons, Inc: UK)
Searle R (2015) The Australian site data collation to support the GlobalSoilMap. In ‘GlobalSoilMap: Basis of the global spatial soil information system. CRC Press’. (Eds D Arrouays, AB McBratney, J Hempel, AC Richer-de-Forges.) pp. 127–133. (CRC Press: London, UK)
Shannon CE, Weaver W. 1949. ‘Mathematical theory of communication’. (Urbana: University of Illinois Press)
Stephens CG (1953) ‘A manual of Australian soils.’ (CSIRO: Melbourne)
Taylor J, Minasny B (2006) A protocol for converting qualitative point soil pit survey data into continuous soil property maps. Australian Journal of Soil Research 44, 543–550.
| A protocol for converting qualitative point soil pit survey data into continuous soil property maps.Crossref | GoogleScholarGoogle Scholar |
van den Boogaart KG, Tolosana-Delgado R, Bren M (2018) ‘Compositions: Compositional Data Analysis.’ (R package version 1.40–2: https://CRAN.R-project.org/package=compositions)
* Part 2, Soil Research 2021, https://doi.org/10.1071/SR20284