Applying two remotely-sensed methods for monitoring grazing impacts in the Australian arid zone
Gary Bastin A * , Robyn Cowley B , Margaret Friedel
A
B
C
D
Abstract
Objective monitoring methods that reliably identify grazing impact are required for long-term sustainable management in the arid rangelands. In Australia such methods must contend with highly unpredictable rainfall and large paddocks incorporating spatially complex land types with differing grazing preferences. Retrospective analysis of data collected following very dry and very wet periods removes vegetation dynamics generated by lesser rainfall events and should increase our ability to separate grazing effects from seasonal variability. Two remote-sensing methods were tested for their capacity to quantify trends over 30 years in vegetation-cover dynamics on a pastoral lease in central Australia with a history of heavy grazing. Following destocking by 2002, one section became a conservation reserve and another transitioned to a research station. During drought, the Dynamic Reference Cover Method (DRCM) showed that ground-cover deficit was less negative on both areas towards the end of the study. This improvement was attributable to increased ground cover after removal of grazing, followed by a reduced, but variable, grazing intensity on the research station and the spread of an introduced palatable perennial grass. Ground-cover response following rainfall was highest in 2011. Likewise, increased ground cover meant that the percentage Cover Production Loss (%CPL) index, calculated using grazing gradient methods (GGMs), was considerably less than a decade earlier following similar rainfall. Results from an associated recovery index (R) were inconclusive. Landscape heterogeneity potentially affected calculation of cover deficit using DRCM but, because heterogeneity was stable over time, reported change between dry years reliably indicated a trend owing to grazing. Interpreting trend from successive %CPL values in wet periods was complicated on the research station by altered waterpoint locations being superimposed on pre-existing degradation; however, the method should be effective in large paddocks with stable waterpoint locations. Despite their limitations, both methods can assist in objectively judging the long-term sustainability of grazing practices in contrasting seasonal conditions.
Keywords: cover production loss, ground-cover deficit, ground‐cover response, grazing gradients, landscape heterogeneity, rainfall variability, remote sensing, stocking rate, trend monitoring.
Introduction
Remote-sensing technologies have been widely researched and adopted for monitoring arid and semi-arid landscapes in many parts of the world. In particular, long-term studies have commonly sought to separate the effects of climate variability from management impacts, and to detect or quantify degradation, using multi-temporal imagery, such as, for example, studies of Washington-Allen et al. (1998) (Bolivia), Washington-Allen et al. (2004, 2006) (Utah, USA), Wessels et al. (2007) (South Africa), Paudel and Andersen (2010) (Nepal), and Wylie et al. (2012) (Great Basin, USA). A substantial proportion of these studies depend on indicators of peak plant productivity during regular growing seasons, such as Normalised Difference Vegetation Index (NDVI) (e.g. Wessels et al. 2007; Paudel and Andersen 2010; Wylie et al. 2012). Some specifically address the influence of spatial variability on change detection (e.g. Washington-Allen et al. 2004, 2006).
Over a similar period, Australian scientists have also investigated the use of multi-temporal imagery for separating the effects of climate variability from management impacts in arid and semi-arid landscapes, but the context has been different. Unpredictable occurrence of growing seasons and ephemeral vegetation mitigate against the use of NDVI or other greenness indices. Additionally grazing infrastructure can be minimal, given large paddocks and distant watering points, and spatial and temporal complexity can be high. Early approaches sought robust vegetation indices that were minimally affected by transient greenness and background soil colour (Pickup et al. 1993; Graetz et al. 1988) and variously examined temporal and spatial trends to identify grazing impact. For example, land cover-change analysis (Karfs 2002) examined temporal patterns of change, and used inferences drawn from ancillary data to separate grazing effects from natural variation. Paddocks or properties with differing time traces might then indicate varied grazing histories, provided the region had experienced the same general rainfall and wildfire had not affected cover dynamics. VegMachine.net is an online implementation of land cover-change analysis; users can graph historical (1990–) cover on one or more user-defined areas of interest, produce comprehensive monitoring reports by paddock or property, and view a range of land-cover raster images through the website map pane (Beutel et al. 2019). Grazing gradient methods (GGMs) (Pickup and Chewings 1994; Pickup et al. 1994) search for temporal change in systematic spatial patterns of vegetation cover that are explicitly related to grazing.
Spatially averaged, annual ground cover on grazed Australian arid-zone landscapes is typically dynamic due to high interannual variation in rainfall (e.g. Fig. 1). Embedded within this natural variation are the effects of any recent pasture utilisation and varying degrees of land degradation that may either retard or enhance ground cover (Stafford Smith and Pickup 1990; Sparrow et al. 2003; Tongway et al. 2003). By focusing on change among the wettest years or the driest years, much of the rainfall-induced variation in ground cover is bypassed, potentially improving the ability to detect grazing-induced trends. For the time series of available satellite imagery relevant to Fig. 1, there have been three sequences of much above-average rainfall at approximately decadal intervals and five very dry years, the most recent pair being separated by 12 years.
Bottom graph shows July–June and median annual rainfall recorded at Alice Springs Airport (80 years of rainfall data). Solid and hatched bars represent deciles 1 and 10 of rainfall respectively. Top graph shows spatially averaged ground cover of a nearby ~160 km2 area of calcareous shrubby grassland at 3-monthly intervals derived from Landsat imagery by using VegMachine.net. Filled circles indicate mean cover in years of decile 1 rainfall and solid squares match decile 10 rainfall years. Gaps in the early part of the time trace are due to missing or unsuitable imagery.
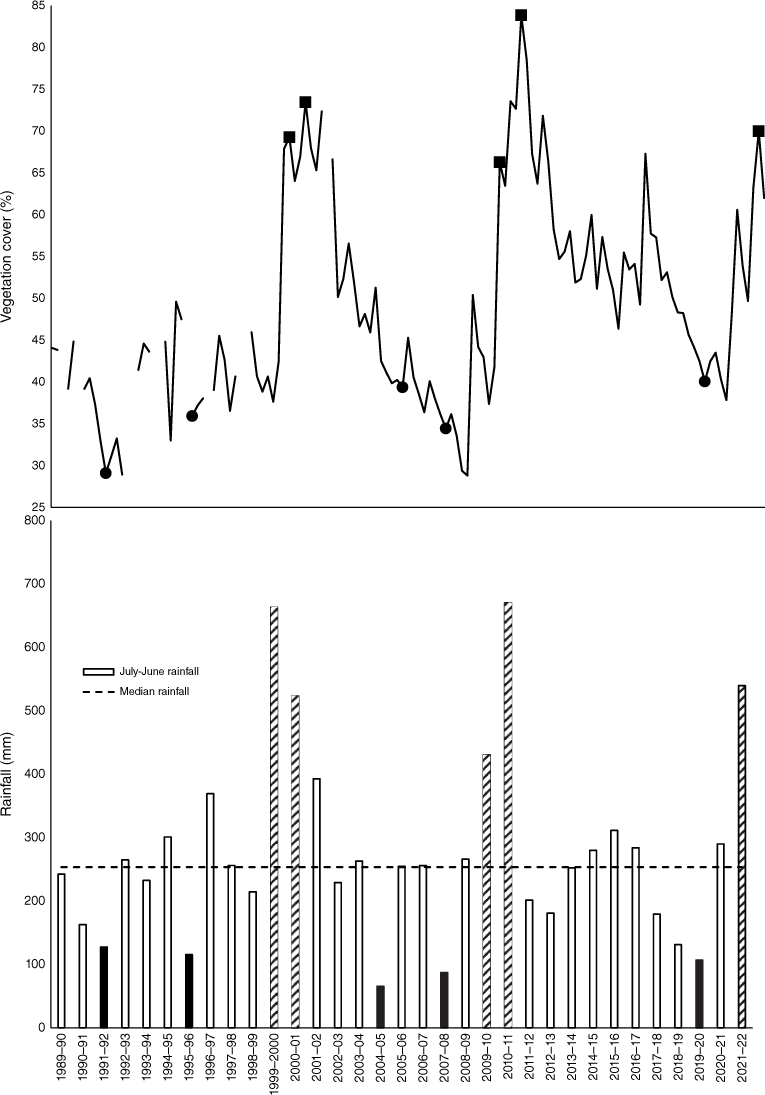
In drier years, retained ground cover reduces erosion risk and may provide insight into landscape function (Ludwig et al. 1997, 2007). Where palatable species are present, persistent ground cover provides important forage for livestock (Bastin and the ACRIS Management Committee 2008). The Dynamic Reference Cover Method (DRCM) (Bastin et al. 2012) calculates an expected (reference) level of ground cover for each pixel. The difference between the actual and reference cover (i.e. deficit) indicates the extent to which an area has been modified by past grazing, provided that recent fire has not reduced ground cover (Bastin et al. 2014).
Grazing gradient methods (Pickup 1989; Pickup et al. 1994) evaluate how vegetation cover at an increasing distance from waterpoints responds to large rainfall events. These methods can provide an explicit way of quantifying land degradation in large paddocks in the arid and semi-arid rangelands (Bastin et al. 1993a). There are two potentially useful metrics, namely (i) the Percentage Cover Production Loss (%CPL), which is an area-weighted index of the difference between the cover level expected if the vegetation fully recovered and that which actually occurred (see Bastin et al. 1993a for detail), and (ii) a vegetation response ratio (Pickup et al. 1998), which compares recovery on areas to a specified distance from water with reference areas at a greater distance. Systematic changes in this ratio over time can indicate a trend related to grazing management. GGMs have been used by Australian jurisdictions for monitoring the pastoral estate (Brook et al. 2001; McGregor et al. 2001), and evaluated in other arid rangelands (Dube and Pickup 2001; Kumar et al. 2015).
In this paper, we test the ability of the methods (DRCM, GGMs) to quantify the direction and magnitude of change in remotely sensed vegetation cover specifically related to grazing management over a 20–30-year interval. We do this against a background of considerable variability in interannual rainfall, likely persistent effects of degradation on vegetation dynamics and altered infrastructure influencing where cattle graze. Ground based-pasture monitoring data from the Old Man Plains Research Station (OMP) are used to contextualise remote-sensing analyses where available and suitable.
Methods
Study area: spatial extent and pastoral infrastructure
The main focus of this case study is OMP, which was formerly part of the Owen Springs pastoral lease (Fig. 2). The pastoral lease was first stocked in 1873 and was one of the earliest grazing leases in the Northern Territory (NT). The NT Government resumed the lease in 2000 to facilitate expansion of the Tjoritja/West MacDonnell National Park and to secure land closer to Alice Springs for the town’s growth.
Former Owen Springs pastoral lease showing the location of OMP and the Owen Springs Reserve. Inset (lower right): Owen Springs pastoral lease in relation to Alice Springs and the NT.
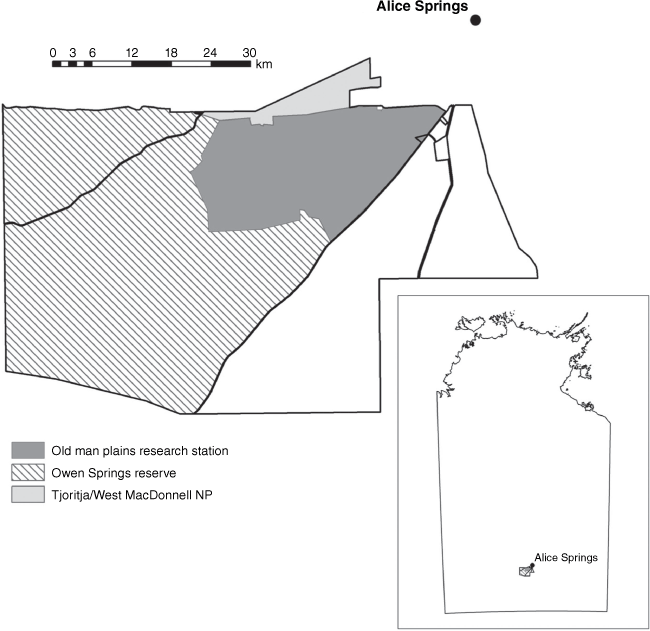
The research station was established in 2002 and is operated by the current NT Department of Industry, Tourism and Trade. Original pastoral infrastructure comprised several dams and four bores in one large paddock (~470 km2) and smaller paddocks (~30 km2) at the eastern end. Additional fencing and waterpoints were installed as budgets allowed (Fig. 3). A land-resource survey was conducted to refine existing land-system mapping (Lennartz and Whitehouse 2002).
Existing fencing and waterpoints when OMP commenced plus infrastructure added as the research station developed. More detailed analyses were conducted in the named focal paddocks.
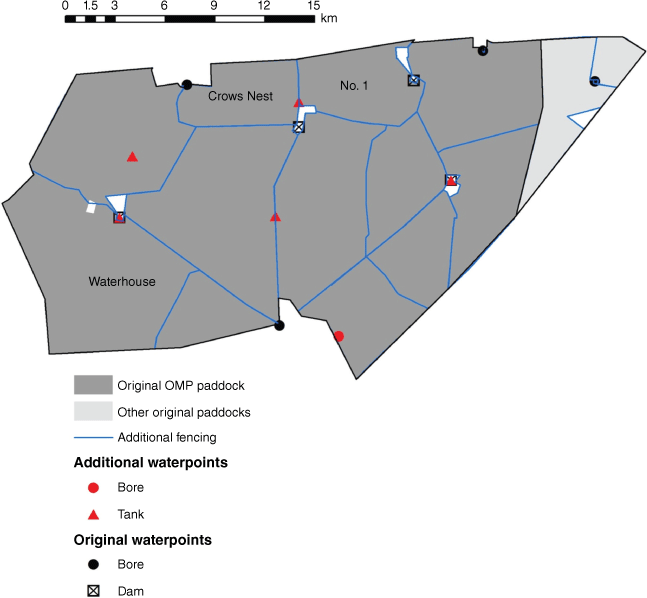
Prior to resuming the lease, a century or more of grazing by cattle, rabbits and, at times, feral horses had left the calcareous country on Owen Springs Station in a degraded state (Fig. 4). GGMs demonstrated the extent and severity of grazing impacts at landscape scale (Bastin et al. 1993a; Pickup and Chewings 1994; see their fig. 5a, Vegetation type 1). The Muller One land system, examined by Bastin et al. (1993a), is almost exclusively confined to the Owen Springs pastoral lease and broadly equates to the calcareous land units mapped by Lennartz and Whitehouse (2002). It had the second highest %CPL index value of the 29 pastorally significant land systems analysed across 38 000 km2 of grazed country in the southern NT. This was due to a much suppressed vegetation-cover response surrounding waterpoints following above-average rainfall, relative to what might be expected. Both dry- and wet-period grazing gradients extended to at least 7 km from water (fig. 6B in Bastin et al. 1993a).
Aerial photo taken in 1984, showing bare ground and erosion present on an area of calcareous country in the present Crows Nest paddock, Owen Springs pastoral lease. The small paddock in the mid-ground (right of centre) is a cattle and rabbit exclosure constructed in 1981. Photo: Geoff Pickup, CSIRO.
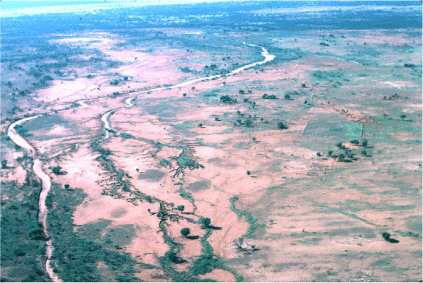
The altered distance-from-water geography in some recently established paddocks (Fig. 5) can potentially complicate contemporary grazing-gradient analyses. There are two issues, namely, the reduced maximum distance from water in most, and particularly smaller, paddocks may mean that grazing gradients are not now expressed (Pickup et al. 1994), and, where they are present, are now superimposed on the previous degradation as described above. As a comparison, the neighbouring destocked Owen Springs Reserve (Fig. 2) is included. Two distance-from-water layers were used for the GGM analyses: prior to, and following, infrastructure development on OMP (Fig. 5).
Fire history
Annual fire-scar maps and a ‘time-since-last burn’ image available at the North Australia & Rangelands Fire Information web site (https://firenorth.org.au/nafi3/, accessed 10 June 2023) showed that extensive areas (>100 km2) of calcareous shrubby grassland and mulga shrubland were burnt on OMP and the Owen Springs Reserve in 2002. A similar area burnt on the Reserve in 2011 with ~30 km2, mostly mulga shrubland, burning on OMP in late 2011. Most imagery used in this analysis was acquired at least 6 years after each fire season, a sufficient period for ground cover to have recovered from antecedent fire. We did not detect any fire effects in the spring 2004 DRCM image, the shortest time since wildfire, and possible confounding effects of fire are not considered further.
Stocking history
As a pastoral lease, annual branded cattle numbers on Owen Springs Station varied with rainfall, building up in good seasons and declining in drought. The station reported an average herd size of ~4350 cattle for the financial years 1953–54 to 1973–74 (range: 2264–7602) (Department of Industry, Tourism and Trade unpubl. data). On the basis of a published account of 5000 cattle in 1988 (Northern Territory Women’s Advisory Council 1991), there was a probable stocking rate of ~12 animal equivalents (AE)/km2 within the effectively watered area of Muller One land system (Appendix 1). Feral horses (unknown number) and rabbits (densities as high as 20/spotlight km, Foran et al. 1985) added to total grazing pressure. Rabbit numbers reduced with the spread of rabbit haemorrhagic disease in 1996 (Edwards et al. 2002).
Owen Springs Reserve and OMP were destocked between 2000 and 2002, the former area permanently. The three OMP paddocks forming the basis of this study, that is, the ‘focal’ paddocks, were restocked with cattle following infrastructure development. Waterhouse paddock (Fig. 5) was restocked on a continuous basis, whereas No. 1 and Crows Nest paddocks were spelled over the summer and early autumn months as part of a four-paddock rotation starting in mid-2007. Cattle AEs were calculated with the AE calculator (see Blakeley and McLean v14.63, McLean and Blakeley 2014; McLennan et al. 2020) for each class of animals using their actual measured performance for each quarter. The AE by class by quarter for each paddock was multiplied by the number of animals in each class during that time period to get total paddock AEs.
Stocking rate was calculated within the watered area, defined as all the area within 3 km and half the area between 3 and 5 km from water, which is close to the area within 4 km from water, the distance within which cattle mostly graze except in very dry seasons (Hodder and Low 1976).
Landscape stratification
Land-unit mapping (1:50 000 scale, Lennartz and Whitehouse 2002) was confined to the eastern half of Owen Springs Station. The coarser-scale (1:250 000) land-system mapping of Shaw and Bastin (1988), which covered the entire station, was used to compare ground-cover dynamics on the Owen Springs Reserve with that of the OMP area (Fig. 6, top map). This analysis was restricted to Muller One land system (gently undulating plains with witchetty bush (Acacia kempeana)). Land units were combined to form two land types within OMP (Fig. 6, bottom map). Calcareous shrubby grasslands are a preferred area for grazing. They were delineated by combining land units 4.01 (sandy plains over calcrete, sparse acacia shrubland over an Enneapogon tussock grassland) and 4.02 (pebbly calcrete plains, scattered A. kempeana and Enneapogon species) (Lennartz and Whitehouse 2002). The exotic, although naturalised, buffel grass (Cenchrus ciliaris) has progressively established across this landscape. Mulga shrublands are less preferred by cattle. Units 2.01 (gently undulating sandy rises of mainly mulga (A. aneura) above Aristida holathera, other marginally palatable grasses and herbage) and 2.08 (sandy rises with mulga over mainly spinifex (Triodia spp.)) were combined to map this land type. Land system/type areas for tenures and paddocks are summarised in Table 1.
The extent of Muller One land system on the former Owen Springs pastoral lease (top) and the distribution of calcareous shrubby grasslands and mulga shrublands on OMP (bottom).
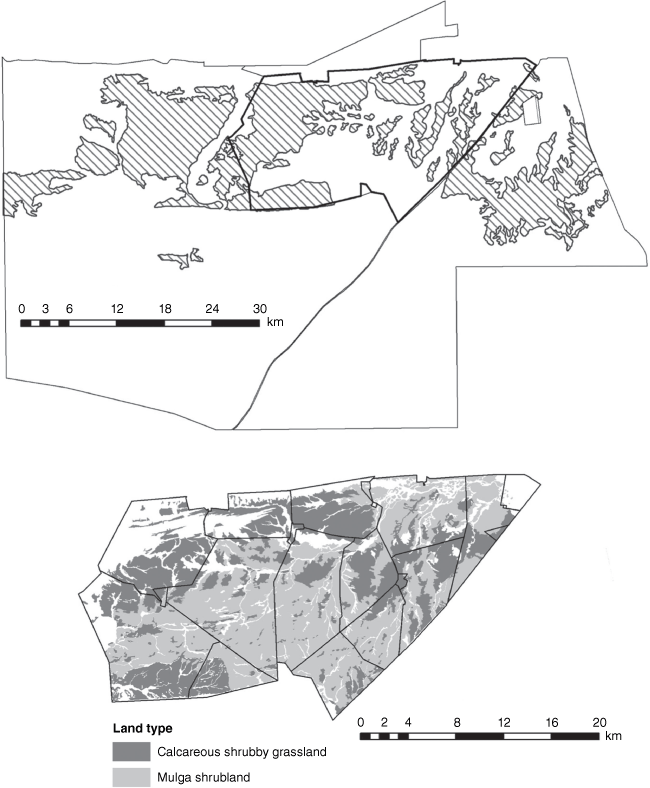
Tenure | Land system/type | Area (km2) in paddock | Area (km2) to 4 km from water | |
---|---|---|---|---|
Owen Springs Reserve | Muller One land system | 202 (13) | 116 (7) | |
Original OMP paddock (prior to establishing Old Man Plains Research Station) | 182 (33) | 99 (18) | ||
Original OMP paddock | Calcareous shrubby grassland land type | 150 (32) | 93 (20) | |
Mulga shrubland land type | 212 (45) | 135 (29) | ||
Focal paddocks | ||||
Crows Nest | Calcareous shrubby grassland | 10.6 (43) | 8.3 (33) | |
Mulga shrubland | 0.2 (1) | 0.1 (0) | ||
No. 1 | Calcareous shrubby grassland | 18.6 (66) | 11.6 (41) | |
Mulga shrubland | 3.3 (12) | 0 (0) | ||
Waterhouse | Calcareous shrubby grassland | 27.9 (37) | 9.6 (13) | |
Mulga shrubland | 30.3 (40) | 18.8 (25) |
Numbers in parentheses are the percentage area of each land system/type.
Remotely sensed data
DRCM images are derived from Landsat-based seasonally composited fractional cover (Scarth et al. 2010). Images covering dry periods were accessed 14 February 2021 at http://qld.auscover.org.au/public/data/landsat/seasonal_fractional_cover/groundcover_drcm/nt/ (see Bastin et al. 2012 for calculation of pixel-level cover deficit values, ΔGC). Fractional cover images were downloaded from http://qld.auscover.org.au/public/data/landsat/seasonal_fractional_cover/fractional_cover/nt/ (accessed 24 July 2022). Ground-cover images were also available but have been less rigorously validated in the Northern Territory than is fractional cover. The photosynthetic (PV) and non-photosynthetic (NPV) components were summed to provide percentage vegetation cover. This was treated as ground cover for the calcareous shrubby grassland land type because of generally sparse woody cover. Vegetation cover in the mulga shrublands includes woody cover and the portion of ground cover amongst trees and shrubs detected from space. Here, ΔGC effectively represents persistent vegetation cover in dry years rather than ground cover.
Both image types were subset to the spatial extent of the former Owen Springs lease and reprojected to match other spatial data (GDA94, MGA Zone 53).
DRCM and fractional-cover images were selected according to percentile-ranked rainfall (Table 2).
Image type | Seasonal composite | Percentile rainfall | Purpose (see the Data analysis section for further detail) | |
---|---|---|---|---|
Wet period fractional cover | Spring 2000 | 96 | Persistence of any grazing gradients following much above-average rainfall | |
Autumn 2001 | 94 | |||
Winter 2010 | 91 | |||
Autumn 2011 | 97 | |||
Autumn 2022 | 95 | |||
Dry period fractional cover | Winter 1999 | 33 | Used to calculate %CPL and R. Subtracted from corresponding wet-period images (above) to examine the scaled magnitude of vegetation response to above-average rainfall | |
Winter 2009 | 58 | |||
Winter 2020 | 6 | |||
DRCM | Spring 1991 | 9 | Spatially averaged ground-cover deficit and its change between successive periods of much below-average rainfall | |
Spring 1996 | 8 | |||
Spring 2004 | 0 | |||
Spring 2008 | 3 | |||
Winter 2020 | 6 |
The percentage rank of annual rainfall measured at the Alice Springs Airport is also reported (80 years of records). This rainfall is for the July–June period preceding, or in the same year as, the seasonal composite. Italicised numbers denote the latter case.
Data analysis
All spatial analyses were conducted using the ArcGIS software package (ArcGIS Desktop 10.8.2, Esri Australia, Brisbane QLD 4000. http://www.esri.com).
For 1991, the first dry year, pixel-level cover deficit values (ΔGC) were spatially averaged (i.e. ) for each land-type and paddock combination (terminology follows Bastin et al. 2012). Repeating the process for following dry years (Table 2) allowed the magnitude and direction of change in mean cover deficit to be calculated between dry years (i.e. ΔΔGC for pixels and for the mean of land systems or types within paddocks). Table 3 summarises indices and provides a guide to interpreting values.
Method | Index | Definition | Interpreting values | |
---|---|---|---|---|
DRCM: applied in very dry/drought years. Calculates a reference level of ground cover for each pixel. Cover deficit (ΔGC) is the difference between the actual and reference cover. | Spatially averaged cover deficit for an individual dry period (ΔGC is typically averaged for a land type within a paddock). | More negative values indicate that a landscape is in a poorer state because of grazing. | ||
Change in spatially averaged cover deficit between time1 and time2. | Positive values indicate improvement in state over time (positive trend). Negative values mean a declining trend. | |||
Grazing gradient analysis: response of vegetation cover to above-average rainfall relative to distance from water. | %CPL | Area-weighted index of the difference between full vegetation recovery and that which actually occurred. | Larger values are associated with reduced response of cover to rainfall and indicate a persistent grazing impact (poorer land condition). | |
For similar rainfall events, lower %CPL values over time should indicate that grazing impact on vegetation response has decreased. | ||||
R | The area-weighted ratio of vegetation-cover response on areas to a specified distance from water relative to more distant reference areas. | <1: grazed area had a lesser response than the benchmark; ~1: similar response on grazed and reference areas; >1: grazed area had a better response than the benchmark. | ||
Systematic change in R over time for land types within paddocks should indicate grazing-related trend in land condition. |
Statistics were calculated for the Muller One land system within the two tenure-types of the former pastoral lease, for calcareous shrubby grasslands and mulga shrublands in the original OMP paddock (Fig. 2), and for Crows Nest, No. 1 and Waterhouse paddocks that were formed within the original OMP paddock (see Fig. 3 for paddock locations). Crows Nest and No. 1 paddocks have the longest period of ground-based monitoring and a substantially altered distance-from-water geography. Distance from water has remained relatively stable in Waterhouse Paddock (Fig. 5).
Vegetation (or ground) cover in 200-m radial increments from water were spatially averaged for each land type (or land system) and paddock combination prior to and following each wet period (Table 2), to determine whether grazing gradients existed and persisted. The following two GGM indices were calculated: percentage cover production loss and a vegetation response ratio.
Where a wet-period gradient persisted, %CPL values (Table 3) were calculated on the basis of the distance to an appropriate inflexion point and the dry- to wet-period increase in mean cover as described by Bastin et al. (1993a). The inflexion point was the distance, when approaching water, beyond which wet-period average cover values markedly decreased. Identifying this point is assisted by progressively smoothing average cover values towards water (refer to Bastin et al. 1993a for details; also see Discussion).
Vegetation growth (%increase in cover) resulting from each wet period was adjusted for initial (dry-period) cover to give a standard measure of vegetation response. This scaling is necessary because the possible range of responses varies with initial cover; for example, an area with 10% cover before rainfall could possibly have an extra 90% cover after rain, whereas an area with 80% cover can have only an additional 20% cover. An area-weighted vegetation response ratio (Table 3) was then derived by comparing areas less than 3 km from water with benchmark areas further away (see Pickup et al. 1998 for detail). These authors used a 4-km distance for the benchmark but that was reduced to 3 km here because of the smaller paddocks now present on OMP.
R values were calculated for Muller One land system in the Owen Springs Reserve and original OMP paddock, and for calcareous shrubby grasslands and mulga shrublands in the original OMP paddock, and in the Crows Nest, No. 1 and Waterhouse paddocks.
Ground data
Pasture assessments at fixed sites within OMP paddocks were replicated at four distances from water (500 m, 1, 3 km, and also at 5 km in larger paddocks) in the calcareous shrubby grassland and mulga shrubland land types. Monitoring commenced in 2006 in No. 1 paddock and in 2007 in Crows Nest paddock, and then extended to all research paddocks in 2011. Sites were usually monitored in May–June of each year.
Pasture biomass (dry weight) and its composition (top four species), and percentage ground cover were estimated within a square-metre quadrat (40 samples per site). Biomass was calibrated using the double-sampling technique following BOTANAL methodology (Tothill et al. 1992).
Selected ground data were compared with, and used to provide additional insight into, the spatial and temporal analyses of fractional cover.
Results
Stocking history of focal paddocks
Waterhouse, No. 1 and Crows Nest paddocks were destocked from mid-2002 until cattle were placed in Waterhouse paddock in mid-2005. Restocking of No. 1 and Crows Nest paddocks followed in mid-2007 (Fig. 7). Average stocking rates were similar among paddocks from the time that OMP commenced to mid-2021 (Table 4), although Waterhouse paddock was more heavily stocked between 2005 and 2010, and Crows Nest and No. 1 paddocks were 10% and 40% respectively more heavily stocked than was Waterhouse between 2014 and 2021 (Fig. 7).
Dynamic reference-cover method (DRCM)
At the first assessment in 1991, Muller One land system had similarly negative values for both the Owen Springs Reserve and the original OMP paddock (asterisked symbols in Fig. 8). Both areas showed similar recovery, on the basis of , almost 30 years later.
Scatterplot of land-type by paddock combinations ranked according to initial state (1991) and change in state to 2020 (). Data values are shown for land-system mapping (asterisk symbol and italicised area names) and for land types within OMP focal paddocks. Paddock by land-type values are plotted where more than 1 km2 of that land type is present. Area and paddock locations are shown in Figs 2 and 3.
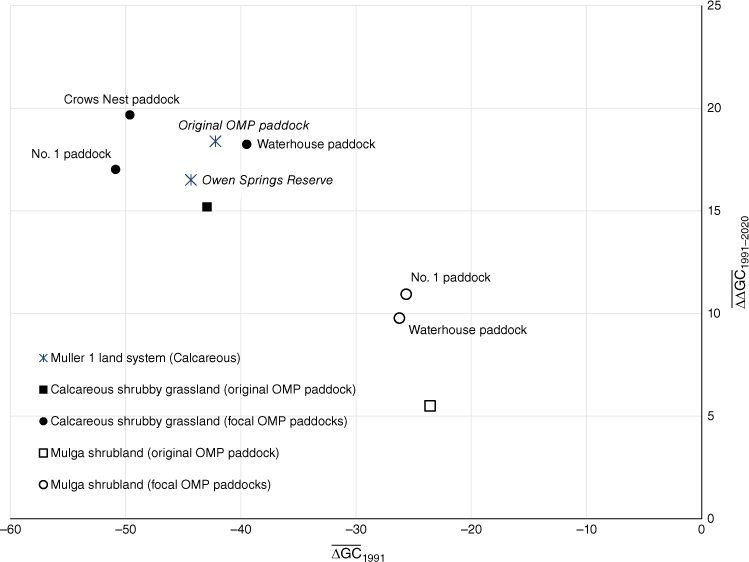
The ground-cover deficit for calcareous shrubby grasslands and the Muller One land system in the original OMP paddock plotted close together (Fig. 8) due to the spatial similarity between the land system and unit mapping (Fig. 6). Values of converged in 2004 (Fig. 9) after OMP had been completely destocked. Thereafter, ground‐cover deficit was mostly stable subsequently for the entire area of calcareous shrubby grassland.
Magnitude and direction of change in spatially averaged ground-cover deficit between 1991 and 2020 for calcareous shrubby grasslands in the original OMP and the three focal paddocks.
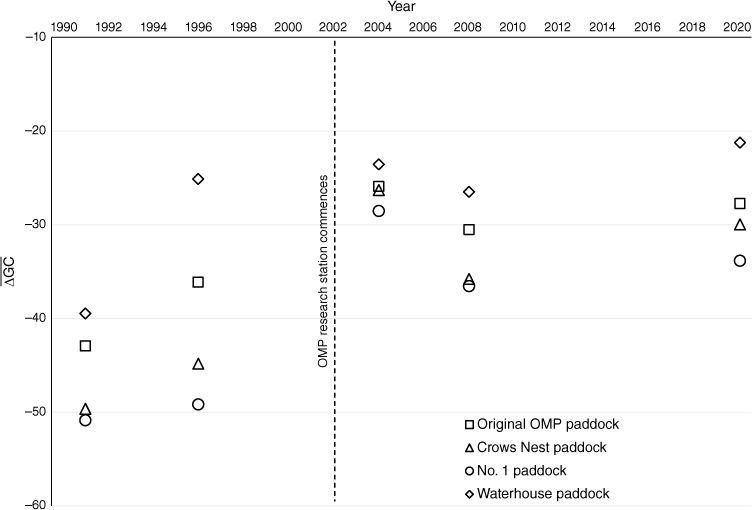
Initial ground-cover deficits for calcareous shrubby grasslands in the future No. 1 and Crows Nest paddocks were more negative than the average for the original OMP paddock (Fig. 9). After establishment, both paddocks had similar trajectories of change in ground-cover deficit, such that, by 2020, their values were similar to those for the original OMP paddock. Calcareous shrubby grasslands in Waterhouse paddock appeared, on average, not to have been as severely degraded on the basis of DRCM analysis (Fig. 9), with values becoming less negative by 1996 and then largely stabilising. However, there was considerable dichotomy in the spatial arrangement of ΔGC values in Waterhouse paddock; water-remote country in the south was less degraded in 1991 and had a larger increase in persistent ground cover to 2020 than had similar country closer to water (Fig. 10). This figure also shows where persistent ground cover increased in No. 1 and Crows Nest paddocks between 1991 and 2020 and where the response was minimal.
Pixel-level state (ΔGC1991) and change (ΔΔGC1991–2020) of calcareous shrubby grasslands in three focal paddocks on OMP. Darker blue areas correspond with a large negative ground-cover deficit in 1991 and limited change to 2020, lighter blue indicates a similarly negative cover deficit at the outset and considerable improvement by 2020, and green shows areas in a better state in 1991 that have also improved to 2020.
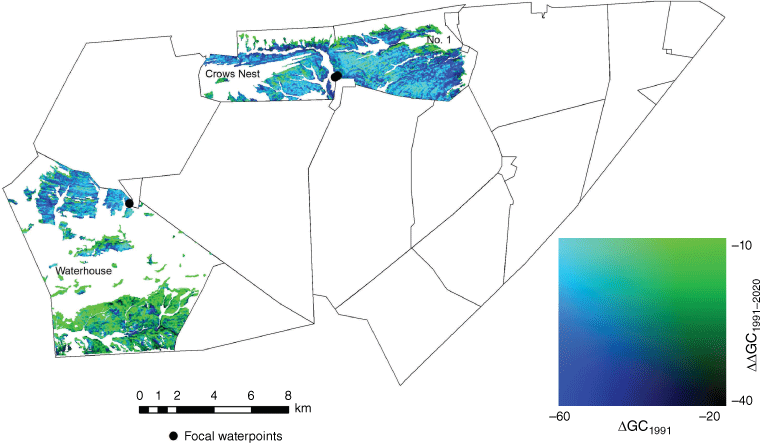
Ground-cover deficit was less negative for mulga shrublands in the original OMP paddock in 1991 than for the calcareous shrubby grassland land type (Fig. 8). Mulga shrublands then had a reduced magnitude of change over the 30 years to 2020.
Grazing-gradient methods
Calcareous country on the Owen Springs Reserve and the original OMP paddock had high %CPL values in 2000 (Table 5), indicating a more severe grazing impact at the outset of this analysis. Further rainfall in 2001 reduced the %CPL on the Owen Springs Reserve and this was maintained following subsequent wet periods.
Tenure | Land system/type | Wet period | %CPL | Inflexion point | ||
---|---|---|---|---|---|---|
Distance from water (km) | Mean %cover | |||||
Owen Springs Reserve | Muller One land system | 2000 | 12.8 | 6.2 | 61.1 | |
2001 | 8.4 | 6.2 | 72.8 | |||
2010 | 3.7 | 1.8 | 58.9 | |||
2011 | 4.3 | 1.8 | 78.6 | |||
2022 | 5.6 | 2.8 | 58.4 | |||
Original OMP paddock | 2000 | 15.5 | 4.4 | 61.8 | ||
2001 | 16.7 | 4.2 | 72.4 | |||
2010 | 7.6 | 1.8 | 59.1 | |||
2011 | 4.6 | 1.8 | 80.8 | |||
2022 | 5.8 | 1.8 | 66.6 | |||
Original OMP paddock | Calcareous shrubby grassland land type | 2000 | 19.7 | 5.8 | 62.3 | |
2001 | 17.5 | 4.4 | 70.8 | |||
2010 | 31.2 | 6.8 | 66.9 | |||
2011 | 6.3 | 1.4 | 79.6 | |||
2022 | 11.2 | 1.4 | 64.5 | |||
Mulga shrubland land type | 2000 | 6.9 | 1.0 | 65.8 | ||
2001 | 4.3 | 1.0 | 76.3 | |||
2010 | 3.0 | 1.2 | 68.0 | |||
2011 | 3.5 | 1.2 | 85.5 | |||
2022 | 4.0 | 1.2 | 75.2 | |||
Waterhouse paddock | Calcareous shrubby grassland | 2000 | 20.7 | 5.4 | 61.3 | |
2001 | 19.8 | 5.4 | 71.6 | |||
2010 | 22.6 | 6.6 | 68.9 | |||
2011 | 11.4 | 6.6 | 83.9 | |||
2022 | 26.0 | 6.6 | 74.2 | |||
Mulga shrubland | 2000 | 16.3 | 3.8 | 66.5 | ||
2001 | 14.6 | 3.8 | 74.4 | |||
2010 | 10.2 | 3.6 | 69.2 | |||
2011 | 4.5 | 1.2 | 83.2 | |||
2022 | 7.0 | 2.8 | 76.5 | |||
No. 1 paddock | Calcareous shrubby grassland | No distinct grazing gradient following any wet period and %CPL not calculated | ||||
Mulga shrubland | Only a small area (3.3 km2) which is beyond 4.5 km from water. %CPL not calculated | |||||
Crows Nest paddock | Calcareous shrubby grassland | No distinct grazing gradient following any wet period and %CPL not calculated | ||||
Mulga shrubland | A very small area (0.2 km2) that is beyond 4.5 km from water. %CPL not calculated |
Parameters used to calculate %CPL values (distance to inflexion point and mean percentage cover at that distance) are also specified.
Based on the more precise land-unit mapping, calcareous shrubby grasslands in the original paddock had high %CPL values until 2010 and reduced values thereafter, implying that landscapes subject to historical degradation were recovering. However, this was not consistent for the entire OMP area; within the Waterhouse paddock and using the current distance-from-water layer, %CPL values were higher in all years except 2010, indicating that recent grazing management was maintaining longstanding degradation.
Inflexion points were close to water in the mulga shrublands of the original OMP paddock and this produced small and generally stable %CPL values. A contributing factor may have been the relative abundance of calcareous country within grazing range of water (Table 1), meaning that cattle preferentially grazed this land type before using the mulga shrublands. It was a different case for Waterhouse paddock, where the small grazing area of calcareous shrubby grassland was likely to mean that cattle preferentially grazed the shrubland country. Apart from 2011, inflexion points in Waterhouse paddock were more distant from water, a situation similar to the calcareous shrubby grassland, and produced higher %CPL values than those of the original OMP paddock. Another contributing factor until 2010 was the higher stocking rate (Fig. 7), most likely requiring cattle to walk further from water. The recovery of %CPL in 2011, facilitated by further high rainfall, an increased watered area and an associated reduced stocking rate, appeared to be temporary on the basis of the elevated 2022 %CPL value. This suggests that mulga shrublands surrounding waterpoints in the western part of the original OMP paddock may have been more affected by past grazing than is indicated by the average for the paddock.
Muller One land system on the Owen Springs Reserve had the most dynamic R (Fig. 11a). Starting with a similar ground-cover response on grazed and benchmark areas in 2001, the formerly grazed country then showed strong recovery compared with the reference in 2011. This pattern was reversed in 2022 when the response close to former waterpoints was less than that for more-distant areas. Ground-cover response was similar throughout on grazed and benchmark areas of the land system in the original OMP paddock.
Vegetation response ratios (R) for three wet periods: (a) Muller One land system in the Owen Springs Reserve and original OMP paddock of the former Owen Springs pastoral lease; and (b) calcareous shrubby grasslands in the original OMP and three focal paddocks. For (a) and the original OMP paddock in (b), R was calculated using the original distance-from-water configuration (Fig. 5, top panel). This was also the case for the 1999–2001 analyses of focal paddocks in (b). Thereafter, the current paddock and waterpoint infrastructure was used (Fig. 5, lower panel). All benchmark areas are >3 km from water.
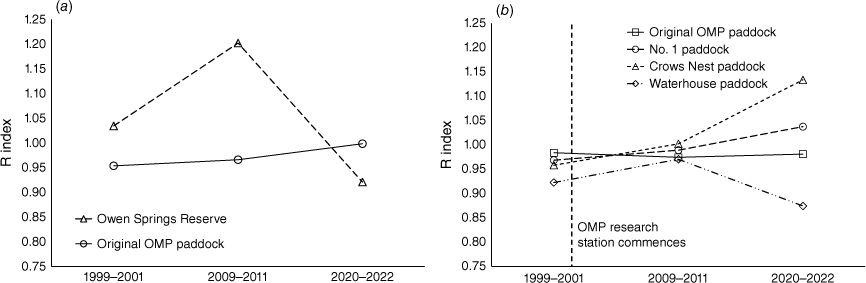
R values for the more precisely mapped calcareous shrubby grasslands in the original OMP paddock were close to one for all wet periods (Fig. 11b). Ground-cover response closer to water in Waterhouse paddock was generally less than that on benchmark areas (Fig. 11b), particularly in 2022. In contrast, there were consistent, if gradual, increases in R through time in the other two focal paddocks, indicating increased ground-cover response closer to the currently used waterpoints. However, it is unlikely that ground cover on assigned benchmark areas in both paddocks responded to their full potential because they were formerly adjacent to presently closed waterpoints.
There was little difference in R values throughout for mulga shrublands in the original OMP or Waterhouse paddocks, implying no adverse grazing impact on cover response (data not shown).
Comparison with ground data
Annual, quadrat-based estimates of ground cover averaged across three to eight sites in the calcareous shrubby grasslands of the three focal paddocks were almost universally less than the contemporaneous, remotely sensed average for the entire land type in each paddock (data not shown, Pearson correlation coefficients for individual paddocks all ≥0.90, P < 0.01). Human assessments of cover are commonly smaller than satellite-based assessments (Trevithick and Scarth 2013), but this does not alter the relative proportions of the human-observed cover and biomass; the issue will not be further addressed here.
The biomass of buffel grass (Cenchrus ciliaris) progressively dominated pasture composition between 2013 and 2019 in Crows Nest and No. 1 paddocks (Fig. 12, top graph). Much of this population died in 2019 and 2020 (Chris Materne, pers. obs.), and its subsequent decay meant that estimated ground cover decreased to slightly more than that present in 2009. Biennial Enneapogon species, forbs and native annual grasses then comprised a greater proportion of standing biomass (and ground cover) as calcareous shrubby grasslands in these two paddocks responded to above-median rainfall in 2021–22. Enneapogon species were the dominant grasses by biomass in Waterhouse paddock between 2011 and 2018 (Fig. 12, bottom). Buffel grass briefly dominated in 2019 but then declined in abundance as in the other focal paddocks.
Annual composition, by dry-weight biomass, of two dominant grasses, other grasses and forbs estimated at calcareous shrubby grassland sites in the Crows Nest and No. 1 paddocks (top) and Waterhouse paddock (bottom). Estimated mean ground cover (±standard error) at each assessment is also shown. Sites in Waterhouse paddock were not monitored in 2016.
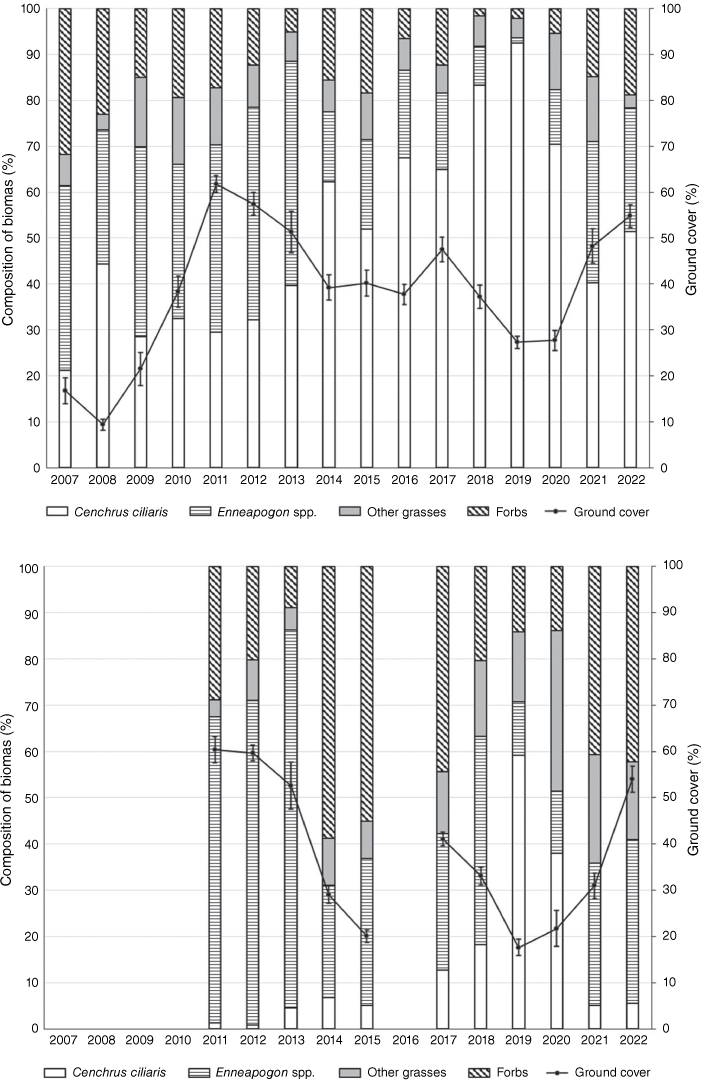
Discussion
The DRCM has demonstrated its utility in quantifying the recovery of a degraded calcareous landscape over a 20–30-year period. Performance of GGMs was more mixed; both the %CPL and R indices quantified recovery of ground cover close to waterpoints after large rainfalls at some locations, whereas elsewhere, and at other times, index values appear unrelated. Within the Owen Springs Reserve, destocking by 2002 facilitated improvement of seasonal ground cover over the next 20 years. Between the very dry years of 1991 and 2020, an initially highly negative ground-cover deficit for Muller One land system was considerably reduced (Fig. 8, ). Reduced %CPL values between 2001 and 2011 suggest that much of the improved ground-cover response occurred during the consecutive wet years of 2010 and 2011. A maximum R value was also recorded for the 2009–11 wet-period (Fig. 11a) where ground-cover response on limestone plains surrounding previously stocked waterpoints exceeded that on more distant benchmark areas. The marginally increased %CPL and reduced R values following the most recent growth event suggest that there were persistent grazing impacts surrounding some former waterpoints that suppressed ground-cover response after single years of above-average rainfall, although these values may also have been mediated by the differences in timing and the amount of rainfall.
Under controlled grazing with stocking rates less than half that which prevailed in the original OMP paddock, there were similarities in some indicator values describing improvement in seasonally interpreted ground-cover dynamics. values for both Muller One land system and calcareous shrubby grasslands plotted close to that of the Owen Springs Reserve. Most of the improvement in had occurred by 2004 (Fig. 9), which we attribute to negligible pasture utilisation following rabbit haemorrhagic disease (Edwards et al. 2002) and complete destocking. For the pastorally more preferred calcareous land types, a mostly stable since 2004 (Fig. 9) likely indicated that any increase in persistent ground cover, including an observed small increase in woody plant cover, largely compensated for that component consumed through grazing. Comparing the three focal paddocks, the value of 2.3 for Waterhouse paddock contrasted with slightly negative for No. 1 and Crows Nest paddocks (−5.3 and −3.7 respectively) and is explained by the greater proportion of calcareous shrubby grasslands in the former paddock that is far from water and less grazed (Table 1). No. 1 paddock was in a slightly poorer state than was Crows Nest in 2020 ( of −33.9 and −30.0 respectively) and this may have been partly attributable to its higher stocking rate preceding that dry year (Fig. 7, Table 4).
The ability of DRCM to quantify the grazing-related trend in this study was similar to that in Queensland’s tropical savanna woodlands where there was broad agreement between values and ground-based assessments of land-condition change made for commercial beef properties (Bastin et al. 2012). In the same region and based partly on DRCM, Bartley et al. (2023) also found that ungrazed and well managed grazed areas had similar recovery of ground cover.
Initial (2001) %CPL values of calcareous country at both land-system and land-type scale in the original OMP paddock were higher than that for the Reserve, indicating a smaller wet-period response. These differences contrasted with the ordering of index values for Muller One land system 13 years earlier when CPL for the Reserve was 18 percentage points higher (Bastin et al. 1993b). The reason for the improved wet-period cover response on the Reserve in 2001 is unclear but may have been attributable to destocking being more advanced on that part of the Owen Springs lease. Thereafter, %CPL values for both the Reserve and original OMP paddock mostly trended down (2011 and 2022), indicating that ground-cover response to above-average rainfall was approaching expected values. The elevated %CPL for calcareous shrubby grasslands on the latter area in 2022 probably relates to more precise mapping of calcareous country, observed widespread death of perennial grasses in preceding drier years and increased cattle numbers on part of the former paddock from 2015 in a deliberately higher stocked treatment. The high %CPL value for Waterhouse paddock in 2010 corresponded with a stocking rate similar to that of the Owen Springs era (Fig. 7) and this may explain the lack of recovery to this point. The stocking rate was substantially reduced in the following year by changing the waterpoint stock accessed, which increased the 4-km watered area from 7% to 28% of the paddock. High pasture growth and 3% utilisation of that growth (C. Materne, unpublished data (or similar)) contributed to the CPL value halving in 2011. In summary, the performance of the %CPL index in quantifying grazing-related trend based on wet-period response of ground cover builds on its previously demonstrated utility for ranking the severity of grazing impacts following a single large rainfall event (Bastin et al. 1993a).
R values did not suggest any consistent improvement, over time, in ground-cover response surrounding waterpoints compared with benchmark areas (Fig. 11). This is an equivocal result; either grazing management has enabled similar responses to, and beyond, 3 km from water (a desirable outcome), or the R values are an aberration because calcareous country beyond 3 km was an inappropriate benchmark for calculating R. The latter conclusion is supported by the earliest grazing-gradient analyses showing inflexion points extending to at least 4 km from water (Table 5). Moreover, the presence of persistent grazing effects beyond 3 km from water were likely to mean that R values between 0.95 and 1.05 indicated a similar ground-cover response close to, and further from, waterpoints, and we suggest that the small differences are largely attributable to differences in rainfall. Extending the benchmark to >4 km from water, as used by Pickup et al. (1998), remained inconclusive, with R stable at 0.97 for the three dry–wet period responses.
DRCM analysis is pixel-based, meaning that ΔGC and ΔΔGC calculations are minimally affected by changed locations of fences and waterpoints, and by existing spatial patterns of degradation related to historic grazing. Images such as Fig. 10, replicated over time, may guide grazing management at paddock and property level, suggesting, for example, relocating waterpoints and implementing additional land-management practices to increase forage supply.
Cover dynamics for mulga shrublands were suppressed relative to calcareous shrubby grasslands, partly owing to the dampening effect of higher woody cover. Moreover, mulga shrublands can be less preferred by livestock when grassland and floodplain forage is available (Low 1972).
The spread of C. ciliaris in the eastern part of OMP, and particularly Crows Nest and No. 1 paddocks (Fig. 12, top), probably contributed to reduced %CPL values for calcareous shrubby grasslands in the area of the original OMP paddock in 2011 (Table 5). Subsequently, estimated ground cover decreased in the dry period 2018–20 and, by 2021 (Chris Materne, pers. obs.), many grass tussocks were dead and disappearing through decay or termite consumption. Thereafter, much of the 2020–22 wet-period response was from seedling establishment, with forbs and annual grasses comprising almost one-third of the pasture biomass. This compositional change was likely to be a contributing factor to the increased %CPL values in 2022 (Table 5).
Calculating DRCM ‘reference cover’ is numerically intensive and has been provided as a complimentary service by the Queensland Government’s Remote Sensing Centre. Operationally, from this point, deriving statistical summaries ( and ) is straightforward, meaning that the types of results presented here (Figs 8, 9) can readily be reproduced for other areas. Users should be aware that, because no prior stratification is applied, landscape heterogeneity may affect the calculation of pixel-level reference cover (Bastin et al. 2014; Beutel et al. 2021) and consequent cover deficit (ΔGC). This may mean that , as an indicator of condition state, is sometimes arbitrary. However, landscape heterogeneity is stable over time, ensuring that change in mean cover deficit between sequences of dry years () reliably indicates change owing to grazing.
Contrasting with DRCM analysis, the design of GGMs means that there are practical limitations to their application. These include small paddocks and closely spaced waterpoints where grazing-related patterns of increasing cover with distance from water are unlikely to be fully expressed (Pickup et al. 1994). Such is the case in No. 1 and Crows Nest paddocks, where it was not possible to calculate %CPL values (Table 5). A further complication is altered grazing infrastructure where pre-existing spatial patterns of degradation are present. Spatially averaged ground cover declined substantially to ~2 km from currently used waterpoints in both paddocks, also shown by the ground data in No. 1 paddock, with no trend then apparent in remotely sensed ground cover to ~6 km (data not shown). The initial decline is characteristic of a composite grazing gradient (Pickup and Chewings 1994) and is often associated with less palatable herbage species close to water; this was the case in No. 1 paddock in 2011 with unpalatable grasses and forbs comprising 15% of total biomass at 0.5-km sites and 22% at 1-km sites. The absence of a remotely sensed cover gradient beyond 2 km may have been due in part to the persistence of historic trends in ground cover radiating from previously stocked waterpoints as reported by Pickup and Chewings (1994). Similarly, continuing substantial ground-cover deficit in the northern part of Waterhouse paddock (Fig. 10) indicated that there is longstanding pasture degradation surrounding the waterpoint.
Operationally, GGMs are best applied to large paddocks with stable waterpoint locations. There is some subjectivity in assigning the inflexion point prior to calculating %CPL and, to minimise that, we followed the criteria of Bastin et al. (1993a). We were conservative in all cases by selecting inflexion values closer to, rather than further from, water. Additionally, %CPL values were partly dependent on the magnitude of wet-period rainfall and the resultant dry- to wet-period increase in ground cover. Single years of above-average rainfall (2000 and 2010) generally produced a higher %CPL value than did consecutive wet years (2001 and 2011, Table 5). The R index appeared to be of questionable value. Muller One land system within 3 km of formerly stocked waterpoints on the Owen Springs Reserve had a much superior ground-cover response to rainfall in 2011 than did benchmark country further from water (Fig. 11). Elsewhere, and for other wet periods, its performance was patchy. Our assessment is that GGMs provide an objective means for assessing the long-term effects of grazing but they cannot remove all of the climatic variation associated with differing wet periods.
Conclusions
Our ability to objectively monitor grazing impact in the Australian arid zone is assisted by retrospectively examining change in remotely sensed ground cover following sequences of very low and exceptionally high rainfall. In this study, the DRCM demonstrated an increased level of persistent ground cover in calcareous shrubby grasslands over a 30-year period. The performance of GGM-derived indices was more variable, principally owing to smaller paddocks, an altered distance-from-water geography overlaid on pre-existing degradation and inappropriate benchmarks for the R index. Despite these limitations, both methods can assist in objectively judging the long-term sustainability of grazing practices because they can be used in contrasting seasonal conditions.
Data availability
The remote sensing and spatial data used in this analysis are public domain. The stocking rate data are the property of the NT Department of Industry, Tourism and Trade. Contact the corresponding author for further information.
Declaration of funding
The Australian Government provided $50 000 to the NT Government in 2004–06 to assist in setting up the four-paddock rotation on Old Man Plains. The NT Department of Industry, Tourism and Trade funded Cowley and Materne. Bastin and Friedel did not receive any specific funding for this research.
Acknowledgements
We thank Dan Tindall, the Remote Sensing Sciences team in the Queensland Department of Environment and Science and the Joint Remote Sensing Research Program for supplying DRCM images on request. Coral Allan, numerous other staff of the NT Department of Industry, Tourism and Trade and volunteers assisted in collecting the field data and their contributions are acknowledged. We thank John Ludwig and two anonymous reviewers whose comments have improved this manuscript.
References
Bartley R, Abbott BN, Ghahramani A, Ali A, Kerr R, Roth CH, Kinsey-Henderson A (2023) Do regenerative grazing management practices improve vegetation and soil health in grazed rangelands? Preliminary insights from a space-for-time study in the Great Barrier Reef catchments, Australia. The Rangeland Journal 44, 221-246.
| Crossref | Google Scholar |
Bastin GN, Pickup G, Chewings VH, Pearce G (1993a) Land degradation assessment in central Australia using a grazing gradient method. The Rangeland Journal 15, 190-216.
| Crossref | Google Scholar |
Bastin G, Scarth P, Chewings V, Sparrow A, Denham R, Schmidt M, O’Reagain P, Shepherd R, Abbott B (2012) Separating grazing and rainfall effects at regional scale using remote sensing imagery: a dynamic reference-cover method. Remote Sensing of Environment 121, 443-457.
| Crossref | Google Scholar |
Bastin G, Denham R, Scarth P, Sparrow A, Chewings V (2014) Remotely-sensed analysis of ground-cover change in Queensland’s rangelands, 1988–2005. The Rangeland Journal 36, 191-204.
| Crossref | Google Scholar |
Beutel TS, Trevithick R, Scarth P, Tindall D (2019) VegMachine.net. Online land cover analysis for the Australian rangelands. The Rangeland Journal 41, 355-362.
| Crossref | Google Scholar |
Beutel TS, Shepherd R, Karfs RA, Abbott BN, Eyre T, Hall TJ, Barbi E (2021) Is ground cover a useful indicator of grazing land condition? The Rangeland Journal 43(1), 55-64.
| Crossref | Google Scholar |
Dube OP, Pickup G (2001) Effects of rainfall variability and communal and semi-commercial grazing on land cover in southern African rangelands. Climate Research 17(2), 195-208.
| Crossref | Google Scholar |
Edwards GP, Dobbie W, Berman DM (2002) Population trends in European rabbits and other wildlife of central Australia in the wake of rabbit haemorrhagic disease. Wildlife Research 29(6), 557-565.
| Crossref | Google Scholar |
Foran BD, Low WA, Strong BW (1985) The response of rabbit populations and vegetation to rabbit control on a calcareous shrubby grassland in central Australia. Australian Wildlife Research 12(2), 237-247.
| Crossref | Google Scholar |
Graetz RD, Pech RP, Davis AW (1988) The assessment and monitoring of sparsely vegetated rangelands using calibrated Landsat data. International Journal of Remote Sensing 9, 1201-1222.
| Crossref | Google Scholar |
Hodder R, Low W (1976) Grazing distribution of free-ranging cattle at three sites in the Alice Springs district, central Australia. The Rangeland Journal 1(2), 95-105.
| Crossref | Google Scholar |
Kumar S, Purohit H, Mathur M, Bastin G, Friedel M (2015) Assessment of impact of drought on desert vegetation through remote sensing. Annals of Arid Zone Research 54(3&4), 63-70.
| Google Scholar |
Low WA (1972) Community preference by free ranging shorthorns in the Alice Springs district. Proceedings Australian Society of Animal Production 9, 381-386.
| Google Scholar |
Ludwig JA, Bastin GN, Chewings VH, Eager RW, Liedloff AC (2007) Leakiness: a new index for monitoring the health of arid and semiarid landscapes using remotely sensed vegetation cover and elevation data. Ecological Indicators 7, 442-454.
| Crossref | Google Scholar |
McGregor F, Bastin GN, Chewings VH (2001) Rangeland assessment on the Barkly Tablelands of Australia’s Northern Territory. In ‘People and Rangelands, Building the Future. Proceedings of the 6th International Rangeland Congress’. (Eds D Eldridge, D Freudenberger) pp. 497–498. (VI International Rangeland Congress: Townsville, Qld, Australia)
McLennan S, McLean I, Paton C (2020) Re-defining the animal unit equivalence (AE) for grazing ruminants and its application for determining forage intake, with particular relevance to the northern Australian grazing industries. Final Report B.NBP.0375. Meat and Livestock Australia, Sydney, NSW, Australia.
Paudel KP, Andersen P (2010) Assessing rangeland degradation using multi temporal satellite images and grazing pressure surface model in Upper Mustang, Trans Himalaya, Nepal. Remote Sensing of Environment 114, 1845-1855.
| Crossref | Google Scholar |
Pickup G (1989) New land degradation survey techniques for arid Australia. Problems and prospects. The Australian Rangeland Journal 11(2), 74-82.
| Crossref | Google Scholar |
Pickup G, Chewings VH (1994) A grazing gradient approach to land degradation assessment in arid areas from remotely-sensed data. International Journal of Remote Sensing 15, 597-617.
| Crossref | Google Scholar |
Pickup G, Chewings VH, Nelson DJ (1993) Estimating changes in vegetation cover over time in arid rangelands using Landsat MSS data. Remote Sensing of Environment 43(3), 243-263.
| Crossref | Google Scholar |
Pickup G, Bastin GN, Chewings VH (1994) Remote-sensing-based condition assessment for nonequilibrium rangelands under large-scale commercial grazing. Ecological Applications 4(3), 497-517.
| Crossref | Google Scholar |
Pickup G, Bastin GN, Chewings VH (1998) Identifying trends in land degradation in non-equilibrium rangelands. Journal of Applied Ecology 35, 365-377.
| Crossref | Google Scholar |
Scarth P, Röder A, Schmidt M (2010) Tracking grazing pressure and climate interaction – the role of Landsat fractional cover in time series analysis. In ‘Proceedings of the 15th Australasian Remote Sensing and Photogrammetry Conference (ARSPC)’. pp. 13–17. (Spatial Sciences Institute: Canberra, ACT, Australia)
Sparrow AD, Friedel MH, Tongway DJ (2003) Degradation and recovery processes in arid grazing lands of central Australia. Part 3. Implications at landscape scale. Journal of Arid Environments 55, 349-360.
| Crossref | Google Scholar |
Stafford Smith M, Pickup G (1990) Pattern and production in arid lands. Proceedings of the Ecological Society of Australia 16, 195-200.
| Google Scholar |
Tongway DJ, Sparrow AD, Friedel MH (2003) Degradation and recovery processes in arid grazing lands of central Australia. Part 1: Soil and land resources. Journal of Arid Environments 55, 301-326.
| Crossref | Google Scholar |
Trevithick R, Scarth P (2013) Estimating RUSLE C-factor values for Great Barrier Reef catchments using satellite derived ground cover estimates. In ‘MODSIM2013, 20th International Congress on Modelling and Simulation’, 1−6 December 2013, Adelaide, SA, Australia. (Eds J Piantadosi, RS Anderssen, J Boland) pp. 3246−3252. (The Modelling and Simulation Society of Australia and New Zealand Inc.)
Washington-Allen RA, Ramsey RD, Norton BE, West NE (1998) Change detection of the effect of severe drought on subsistence agropastoral communities on the Bolivian Altiplano. International Journal of Remote Sensing 19(7), 1319-1333.
| Crossref | Google Scholar |
Washington-Allen RA, Van Niel TG, Ramsey RD, West NE (2004) Remote sensing-based piosphere analysis. GIScience & Remote Sensing 41(2), 136-154.
| Crossref | Google Scholar |
Washington-Allen RA, West NE, Ramsey RD, Efroymson RA (2006) A protocol for retrospective remote sensing-based ecological monitoring of rangelands. Rangeland Ecology & Management 59(1), 19-29.
| Crossref | Google Scholar |
Wessels KJ, Prince SD, Malherbe J, Small J, Frost PE, VanZyl D (2007) Can human-induced land degradation be distinguished from the effects of rainfall variability? A case study in South Africa. Journal of Arid Environments 68(2), 271-297.
| Crossref | Google Scholar |
Wylie BK, Boyte SP, Major DJ (2012) Ecosystem performance monitoring of rangelands by integrating modeling and remote sensing. Rangeland Ecology & Management 65(3), 241-252.
| Crossref | Google Scholar |
Appendix 1.Calculating a standardised stocking rate for Owen Springs pastoral lease.
The Northern Territory Women’s Advisory Council (1991) reported ‘In 1988, the 1200 square mile property ran 5000 head and, Lizzy (owner, Mrs Elizabeth Milnes) proudly boasted, it was a very well-equipped, neat and tidy station’ (p. 87). Based on the following assumptions, we estimate that this number would have produced an effective stocking rate of ~12 AE/km2 on the Muller One land system.
Owen Springs was a beef breeding enterprise that opportunistically sold fat steers at 3–4 years old. In poorer-quality seasons, steers were turned off as stores. There was minimal sale of females. The reported 5000 head refers to branded cattle only. Minimal infrastructure for herd management meant that mustering efficiency was low, particularly in wet years when there was much surface water. There was no weaning but steers were shifted to a bullock paddock at ~2 years of age.
The herd structure and performance in an average-rainfall year was estimated to be as follows:
2060 predominantly Hereford breeders, 3 years and older with an average liveweight of 400 kg, 90% conception and ~60% calving. This equates to 2490 AE at an annualised rate of 1.21 AE/breeder (McLean and Blakeley 2014). We have not accounted for breeder mortality because these calculations are for 1 year, 1988.
1295 calves (animal equivalence included with breeders above).
1090 first-calf heifers to 2.5 years, 350–400 kg liveweight, 70% conception and 50% calving plus maiden heifers to 2 years, 300–350 kg liveweight, 60% conception and 40% calving. This converts to 1515 AE using an average of 1.39 AE/heifer.
490 steers, 1–3 years with a liveweight range of 300–500 kg, 580 AE (at 1.39 AE/steer on average).
75 herd bulls at an average of 1.35 AE each, 100 AE. There was an unknown additional number of mickey bulls.
On the basis of these assumptions, the herd size was ~5000 head translating to 4685 AE.
Most of the herd was run in two large paddocks, including the original OMP paddock. With complete open-range grazing, most of the grazing pressure was on Muller One land system most of the time (akin to Low 1972, and Hodder and Low 1976). We estimate that the effective watered area of this land system (240 km2; calculated as per the Methods section) carried 60% of the herd on an annualised basis. This equates to 11.7 AE/km2.