Long-term monitoring and modelling of pasture regeneration and water quality from a Bothriochloa pertusa site in the Great Barrier Reef catchments
Chris Stokes

A CSIRO, Townsville, Qld 4810, Australia.
B CSIRO, Brisbane, Qld 4068, Australia.
The Rangeland Journal 45(1) 12-26 https://doi.org/10.1071/RJ22067
Submitted: 30 November 2022 Accepted: 11 April 2023 Published: 24 May 2023
© 2023 The Author(s) (or their employer(s)). Published by CSIRO Publishing on behalf of the Australian Rangeland Society. This is an open access article distributed under the Creative Commons Attribution-NonCommercial-NoDerivatives 4.0 International License (CC BY-NC-ND)
Abstract
There has been considerable investment in grazing land management in Australia’s Great Barrier Reef catchments targeted at improving off-site runoff and water quality. This study used a systems modelling framework to analyse an 18-year monitoring data set from an Indian couch (Bothriochloa pertusa) dominated hillslope flume site in the Burdekin catchment, Australia. The analyses show important functional differences in B. pertusa pastures, relative to the native tussock pastures they replaced, with implications for how they are monitored and managed: (1) rates of landscape recovery were far slower than rates of degradation. In the practical time frames of most land managers, transition to a B. pertusa state is effectively irreversible, so historical management practices will have to adapt; and (2) transformations in pasture composition and structure can lead to misinterpretation of monitored trends in ground cover (a common proxy for land condition). At 50% pasture cover, B. pertusa pastures have only 33% of the biomass of local tussock pastures. Where B. pertusa invasions are occurring, inferences from positive associations between changes in cover and landscape function can break down: increases in ground cover can instead be accompanied by decreases in biomass, productivity, and runoff retention. The paper contributes an initial calibration of the GRASP pasture grazing systems model for a transformed B. pertusa site, capturing the observed relationships between grazing management, pasture biomass, ground cover and water quality. This serves as a starting point from which these landscapes, of growing extent and concern in Great Barrier Reef catchments, can be better represented in monitoring and modelling assessments, and for revised management options to be explored. The calibrated model was able to accurately represent long-term average runoff and sediment yield (both within 2% of observed) but was only able to adequately represent the year-to-year variation in runoff (not sediment).
Keywords: GRASP, grazing management, ground cover, hillslope soil erosion, native pasture, rangeland monitoring, runoff, state and transition, systems modelling.
Introduction
Excess sediments and associated nutrients from agricultural areas are having a detrimental impact on Australia’s Great Barrier Reef (GBR) (Furnas 2003; De’ath and Fabricius 2010; Humanes et al. 2017; Lambert et al. 2021), with sediment exports estimated to be at least five times greater than before European settlement (Mariotti et al. 2020). Livestock grazing is the dominant land use (≈70%) in the catchments draining to the GBR (Lewis et al. 2021), and improvements in the amount and quality of ground cover and pasture biomass has been demonstrated to improve hillslope scale runoff and water quality (Roth 2004; Bartley et al. 2006; Silburn et al. 2011). However, while models and remotely sensed estimates of ground cover are now widely used to infer the long-term effects of improved grazing land management on water quality in GBR catchments (Ghahramani et al. 2020; McCloskey et al. 2021), there remains a gap in published data on these links, and the effectiveness of models in representing them.
One data gap of particular concern is the implications of the widespread invasion of the exotic stoloniferous grass Bothriochloa pertusa (‘Indian couch’), which is displacing native tussock grass pastures in many parts of the GBR catchments, fundamentally transforming their ecological function and responses to grazing (Kutt and Fisher 2011; Kutt and Kemp 2012). The conversion from tussock- to B. pertusa-dominated pastures typically occurs following long-term moderate to heavy grazing of fragile land types (O’Reagain et al. 2018), and coincides with areas prone to sediment loss (McKeon et al. 2004). B. pertusa now covers large areas of grazing land (Lebbink 2021; Lebbink et al. 2022; Lebbink and Fensham 2023) (Fig. 1), but the implications of this physiognomic transformation for water quality are not clear. (Botanical names throughout this paper follow the Australian Plant Names Index (APNI 2022)).
Map of Bothriochloa pertusa distribution, in relation to Great Barrier Reef catchments, based on herbarium, observation and survey records. Source data: Karfs et al. 2009, ALA (2022), AVH (2022).
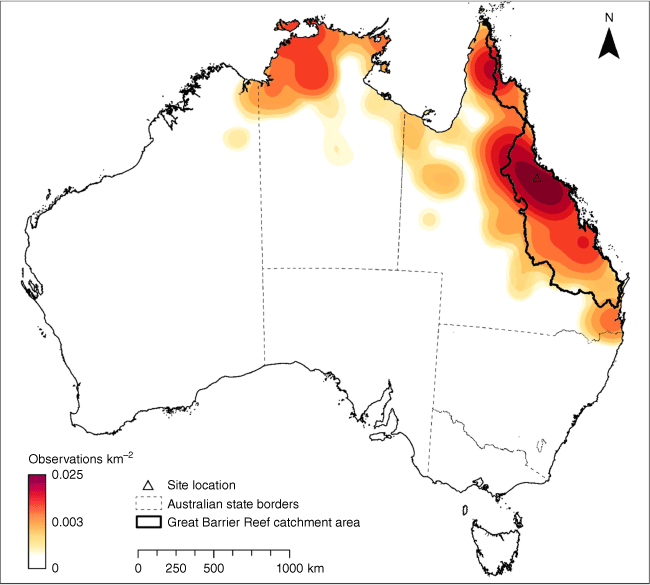
Various grazing land management practices have been used in efforts to reverse this change, including approaches that focus on reducing stocking rates to achieve appropriate levels of pasture utilisation and wet season resting (Ash et al. 2011; Hall et al. 2014; Hunt et al. 2014), and rotational grazing systems that often involve grazing areas for shorter durations at higher intensity (Briske et al. 2011; Teague et al. 2013; Gosnell et al. 2020). Regardless of the method employed, the aim is to restore previous levels of pasture cover, biomass, and land condition, with the perception that this will also reduce runoff and improve off-site water quality. Because semi-arid systems can take 10–20 years or more to respond to improved management (O’Reagain et al. 2011; Bartley et al. 2014; Koci et al. 2020) and these changes are costly to measure, paddock and catchment scale hydrological models are frequently used to estimate and infer the long term effects of management changes on future offsite water quality (Carroll et al. 2012). Land condition is defined here as the capacity of a landscape to retain water, produce biomass, cycle nutrients and support both biodiversity and livestock production.
Few hydrological data sets are available for northern Australian grazing lands to test the links between metrics of improved land condition, runoff, and water quality. Where suitable runoff data exist, most studies were conducted under controlled conditions involving small exclosures or complete cattle removal (McIvor et al. 1995; Scanlan et al. 1996; Silburn et al. 2011), and very few studies have been conducted under commercial grazing conditions. Moreover, while hundreds of calibration data sets have been collected to parameterise pasture components of models such as GRASP for tussock pastures (Day et al. 1997; McKeon et al. 2010), there are fewer calibrated runoff responses, and there are no readily available models that have been parameterised for stoloniferous lawn-forming pastures like B. pertusa.
There is therefore a need for data that can quantify the links between grazing management, pasture responses, land condition, runoff, and water quality in GBR catchments, particularly for expanding B. pertusa landscapes. This paper aims to address this need in two ways. First, it presents 18 years of flume and pasture measurements from monitoring a B. pertusa dominated hillslope located on a commercial grazing property in northeast Australia. Secondly, the GRASP grazing systems model was used to both: provide a rigorous systems framework for quantifying and interpreting trends in land condition and water quality over the course of the study; and test the suitability of the calibrated model to support future work in better representing the influence of B. pertusa in GBR catchments. GRASP is commonly used as a pasture growth and rangeland systems tool (Rickert and McKeon 1988; Owens et al. 2003) to simulate the effects of weather and management on pasture growth, livestock production, land condition, runoff, and erosion. Demonstrating the veracity of the GRASP model components in a site dominated by B. pertusa, will enhance the use of GRASP and related models in assessing water quality outcomes in GBR catchments.
Methods
Study site
This study was carried out on a ‘typical’ hillslope within Virginia Park station, a commercial cattle grazing property in the Burdekin catchment, Queensland, Australia (−19.89°, 146.54°) (Fig. 1). The main drainage system on the property is Weany Creek. The area is representative of the highly erodible granodiorite (‘gold-fields’) country between the cities of Townsville and Charters Towers. The site is a 1.2 ha hillslope within a larger 625 ha paddock, and was chosen because it has been studied and characterised in detail from research spanning more than 30 years (Gardener et al. 1990; Leuning et al. 2005; Bartley et al. 2007; Koci et al. 2020, 2021). The key landscape and hillslope attributes are summarised in Table 1.
Attribute | Description or value |
---|---|
Geology | Granodiorite |
Soil type | Chromosol, sodosol |
Land type, bioregion | Einasleigh Uplands |
Vegetation – overstory | Dominated by Ironbark, bloodwood communities (Queensland Regional Ecosystem 9.12.1), tree basal area 5.1 m2 ha−1 |
Vegetation – grasses | Dominated by B. pertusa |
Long term average rainfall | 634 mm |
Slope gradient | 3.9% |
Slope length | 250 m |
Hillslope area | 11 930 m2 |
The dominant land use has been beef cattle grazing for over 100 years. Prior to 1988, pastures at Virginia Park were dominated by tall native perennial grasses, Heteropogon contortus, Dichanthium fecundum, and native legumes, with the upper story consisting primarily of narrow-leafed ironbark (Eucalyptus crebra) and red bloodwood (Corymbia erythrophloia). However, heavy grazing by Bos indicus cattle between 1979 and 1988 resulted in a significant change in the pasture composition, where the dominant species shifted to B. pertusa, annual grasses, non-legume dicots, and legumes (Gardener et al. 1990).
This study covered the period from 2003 to 2021. At the beginning of the project, during a drought, the ground cover and pasture biomass levels were on average 63% and 350 kg of dry matter per hectare (kg DM ha−1), respectively, well below that considered ‘sustainable’ in terms of long term grazing management for this soil type (Ash et al. 2001). Prior to the study (1986–2002), the stocking rate was about 0.25 AE ha−1, whereas in the first 10 years of the study stocking rates averaged about 0.1 AE ha−1, where one animal equivalent (AE) is measured relative to a 450 kg steer at maintenance (McLean and Blakeley 2014). Previous publications documented the data outputs from this site after 6 and 10 years, respectively (Bartley et al. 2010, 2014). After the formal research project finished, stocking rates varied between years, approximated as light stocking rates from 2013 to 2016 (0.1 AE ha−1, except for complete destocking in 2014) and heaving stocking rates in the five remaining years (about 0.5 AE ha−1 in 2012–2013 and 2017–2020).
Vegetation monitoring
Land condition on the flume hillslope was measured using a range of techniques over the 18-year measurement period (Table 2). Measurements were made at the end of the wet season and end of the dry season each year. Between 2003 and 2016, land condition was measured across the hillslope on an 8 × 4 m grid. Between 2020 and 2021, ten 100-m transects were each sampled with ten equidistant 1 × 1 m quadrats. Sampling followed the BOTANAL calibrated visual estimation method of Tothill et al. (1992). Measurements included pasture composition (by species and functional group), pasture standing biomass, percentage pasture cover (associated with standing pasture biomass), percentage ground cover (predominantly pasture cover plus a small (≈5%) area of non-overlapping litter cover) and grass basal area. Between 2016 and 2020 field data were collected from an adjacent hillslope in the same paddock (within 500 m, sharing the same land management and comparable MODIS ground cover). Calibrated MODIS pasture and ground cover estimates (Guerschman 2020) were used when field measurements were not conducted.
Method | Time frame applied |
---|---|
End of dry season surveys | 2003–2016; 2020–2021 |
End of wet season surveys | 2003–2007; 2020–2021 |
Calibrated MODIS fractional cover components | 2001–2002; 2008–2016 |
End of dry and end of wet surveys from adjacent hillslope | 2016–2019 |
Remote sensing of ground cover
MODIS (Moderate Resolution Imaging Spectroradiometer) satellite data were calibrated against field measurements (Table 2) to derive estimates of pasture cover and ground cover (R2 = 0.88). High resolution (≤10 m) satellite images (Quickbird, Worldview and Sentinel-2) were analysed and calibrated using the PD54 cover method (Pickup et al. 1993) and used to map the distribution of ground cover for 7 years (2003–2007, 2009–2010).
Rainfall corrected trends in ground cover were derived from Landsat images using the dynamic reference cover method (DRCM) outlined in Bastin et al. (2012). This approach identified minimally disturbed, grazed, non-riparian reference areas in Landsat images to benchmark changes in cover through time. There may be systematic biases in the Landsat estimates of ground cover, particularly in areas dominated by B. pertusa (Bastin et al. 1996; Wilkinson et al. 2014a, 2014b; Bartley et al. 2022).
Rainfall, runoff and sediment yield monitoring
Most rain falls between December and April, but occasional out of season events occur, and therefore rainfall and runoff data extend from July 1 to June 30 (used here to define the ‘water year’, each referenced by its starting year).
To measure water and sediment runoff, a large Parshall flume was installed at the bottom of the hillslope. Details of the data logger, associated instrumentation and data processing can be found in Bartley et al. (2006). Annual percentage runoff was calculated as the total annual runoff divided by total rainfall for that water year. Water sampling was stratified by flow depth, and collected samples were used to represent total suspended solids (TSS) concentrations between 2002 and 2013. In the rare case that samples were not collected for an event, an average TSS concentration from the wet season was applied. In 2014 a turbidity sensor was installed in the flume, and a turbidity-TSS relationship (R2 = 0.91) was developed, which allowed continuous sediment loads to be derived when runoff occurred.
Collected water samples were analysed for TSS concentration (considered to represent the silt (0.002–0.063 mm) and clay (<0.002 mm) sediment fractions). Bedload samples (generally between 0.063 and 8 mm) were collected manually from bedload traps but these represent <1% of total load (Bartley et al. 2006), and the data were not used in calculating fine sediment loads. When both concentration and discharge data were available, annual sediment loads were estimated by summing the event loads using the arithmetic mean approach (Letcher et al. 1999). When sediment concentration data were unavailable for an entire event, average values for that wet season were applied.
Modelling approach using GRASP
This study used GRASP (Cedar version 2.1.0), a deterministic, point-based, daily time step, native/naturalised pasture grazing systems model, developed to support research and decision making in semi-arid and tropical grasslands (Rickert and McKeon 1988; McKeon et al. 1990; Owens et al. 2003). The model and parameterisation of its key functions are described by Littleboy and McKeon (1997), with components of the model covering water balance, pasture dynamics, grazing management, runoff and soil loss. GRASP focuses on capturing and representing the gross long-term ‘average’ system behaviours that are of most relevance to property- and catchment-scale management decisions.
Data on the site soil profile (Queensland Government 2022) were used to directly estimate GRASP parameters related to rooting layer depths and water-holding characteristics of each layer (Table 3). Previous pasture measurements were used to directly calculate important parameters related to pasture growth, such as sward nitrogen, maximum pasture growth, and the relationship between pasture cover and biomass. Direct measurements for some other key parameters related to leaf senescence and defoliation were unavailable, and were instead calibrated by selecting pasture parameters that gave a good fit between modelled and observed pasture biomass. The model used daily rainfall measured at the flume site augmented with Charters Towers gap-filled historical weather station data from the SILO online repository (Jeffrey et al. 2001) (Table 3). Model parameters were set to represent the broad shifts in stocking rates described previously in the site description: the intention was not to mimic the full detail of month-to-month changes in stocking rate, but to capture periods of major differences in management. The model was reset to observed pasture states wherever observations were available, which had the effect of limiting the cumulative propagation of small errors that could cause progressive drift of the pasture component of the model from observations over 20 years.
Attribute | Frequency | Units | Data source |
---|---|---|---|
Data inputs | |||
Soil layer depth | Layers to 1.1 m | cm | Local field measurements |
Air dry moisture | Layers to 1.1 m | mm mm−1 | Local field measurements |
Wilting point | Layers to 1.1 m | mm mm−1 | Local field measurements |
Field capacity | Layers to 1.1 m | mm mm−1 | Local field measurements |
Maximum sward nitrogen | Early wet season | kg ha−1 | Local field measurements |
Rainfall | Daily | mm | Local weather station (or SILO) |
Max temperature | Daily | °C | Local weather station (or SILO) |
Min temperature | Daily | °C | Local weather station (or SILO) |
Vapour pressure deficit | Daily | hPa | SILO (Charters Towers) |
Pan evaporation | Daily | mm | SILO (Charters Towers) |
Solar radiation | Daily | MJ m−2 | SILO (Charters Towers) |
Time series observations (for calibrating matched data outputs) | |||
Pasture biomass | Twice yearly | kg ha−1 | Local field measurements |
Pasture cover | Twice yearly | m2 m−2 | Local field measurements + remote sensing |
Ground cover | Twice yearly | m2 m−2 | Local field measurements + remote sensing |
Runoff | Daily (annual totals) | mm | Flume monitoring site |
Total suspended sediment | Daily (annual totals) | t ha−1 | Flume monitoring site |
GRASP contains several different sub-model options for representing runoff. Of these, the ‘curve number’ sub-model was used because it provided the best fit in a previous analysis of the first 10 years of Virginia Park data, and because it allowed explicit testing of whether ground cover affected runoff. Curve numbers range between 30 (maximum infiltration) and 100 (an impermeable surface with maximum runoff). The modified implementation of the curve number model in GRASP (Owens et al. 2003) allows specifying different curve numbers for 0 and 100% ground cover (and then interpolating a curve number for intervening levels of cover). Calibration involved running factorials of these two parameters with progressively finer resolution picking the combination that gave the highest Pearson correlation between modelled and observed annual runoff until the deviation of the modelled average was within 2% of the observed value for the study period.
The sub-model for fine sediment yield was parameterised similarly to runoff, using the Rose sub-model option (Rose 1985), which has parameters for slope angle, sensitivity of soil loss to ground cover, and bare soil efficiency of entrainment.
Results
Changes in pasture composition
Although originally dominated by tussock grasses (with no B. pertusa), the site had been transformed by invasion of B. pertusa by 2002 when the flume was installed and a period of reduced grazing pressure implemented (Fig. 2). Such B. pertusa transformations are characteristic of heavy grazing over long periods, and are typical of State 3 and 4 grazing systems within state and transition models for this region (McIvor and Scanlan 1994; Ash et al. 2011). While the B. pertusa invasion was rapid (replacing most tussock grasses within 9 years), the altered state has proven very resistant to improvements in grazing management, with only slow and limited recovery of native tussock grasses over the subsequent 20 years (Fig. 2).
Changes in relative species composition of the pasture layer at the study site measured at the end of the dry season (as percentages of total biomass). Data points for 1979 and 1988 are from previous studies at the site, predating the installation of the flume (Gardener et al. 1990).
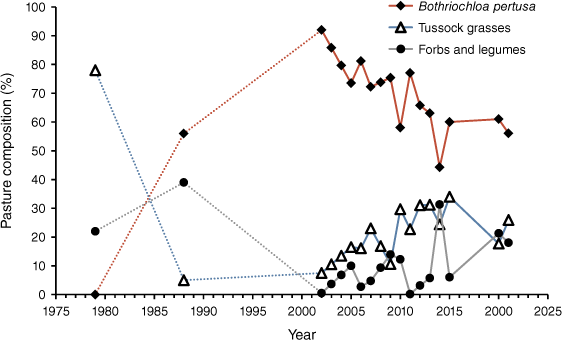
Changes in ground cover
Ground cover at the start of the wet season is particularly important for water quality outcomes, as is the spatial arrangement of the vegetation, where cover lower on the hillslope is more important for trapping sediment (Bartley et al. 2010). Both the amount and spatial arrangement of end of dry season ground cover varied during the study (Fig. 3).
Remotely sensed ground cover on the hillslope flume site in 2003, 2007, 2010, 2015 and 2020. The missing area in 2010 was due to cloud interference.
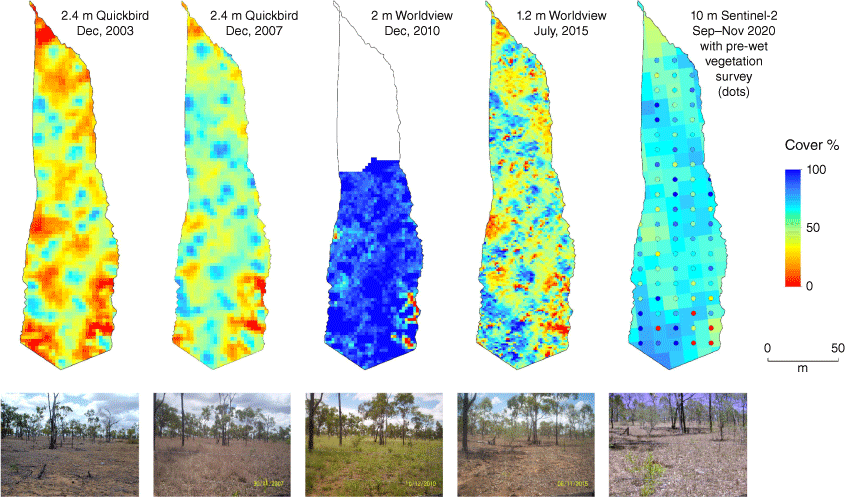
The weather-corrected DCRM data show a slight trend of increasing ground cover over time (not statistically significant, P = 0.134) (Fig. 4). Increases in ground cover occur not only from management practices, but also from changes in pasture composition (as discussed in the next section).
Modelled vs observed pasture biomass and cover
GRASP modelling reflected the observed fluctuations in pasture biomass at the study site over the duration of the investigation, however, forced resets to observations were required to correct for the gradual accumulation of errors (Fig. 5). Ultimately, the pasture component of the modelling was mainly used to interpolate the vegetation dynamics between observations (Fig. 5) to provide the daily ground cover data required by the runoff and soil loss components of GRASP.
Fluctuations in pasture biomass over the duration of the investigation at the Virginia Park flume site. The dashed line is the unconstrained estimate from the GRASP model with approximated adjustments to stocking rates to represent periods of substantial differences in management. Field observations are marked by diamonds, and the solid line is where the model was used to interpolate between observations.
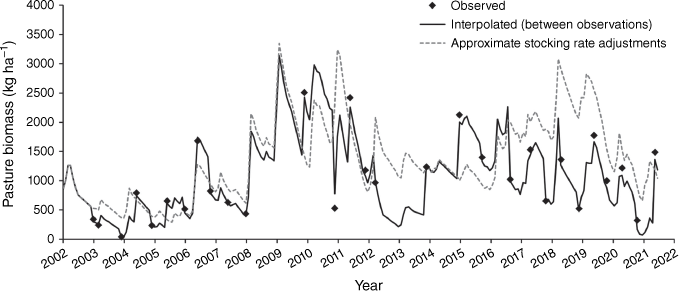
Pasture cover has a strongly asymptotic relationship to pasture biomass. The structure of pasture vegetation at the site was very different from typical tussock pastures in northern Australia because of the creeping, stoloniferous growth form of the dominant B. pertusa. B. pertusa has much less biomass for the same amount of pasture cover relative to a tussock grass (Fig. 6, where, at 50% pasture cover, the B. pertusa pasture had only a third of the biomass expected for tussock pastures in the region). This structural change in vegetation has important implications for how satellite and field-based measures of pasture are interpreted, as demonstrated in Fig. 7, showing that pasture biomass would be greatly over-estimated if it were assumed to be a typical tussock pasture rather than calibrated for B. pertusa. Although pasture cover is easier to measure, biomass is a better indicator of functional land condition both in terms of water quality (the amount of pasture material that obstructs overland flow of water) (Roth 2004) and productivity (the amount of forage and protein-building nitrogen available for livestock to consume). Inferences from pasture cover alone in B. pertusa dominated landscapes would therefore tend to give overoptimistic estimates about land condition and trend.
Contrasting relationships between pasture cover and biomass for pastures dominated by Bothriochloa pertusa (diamonds: end of dry season observations at Virginia Park; solid line: calibrated curve in the form of the equation used in GRASP) and typical tussock grasses in the region (dashed line: parameterised curve for tussock grasses in the region).
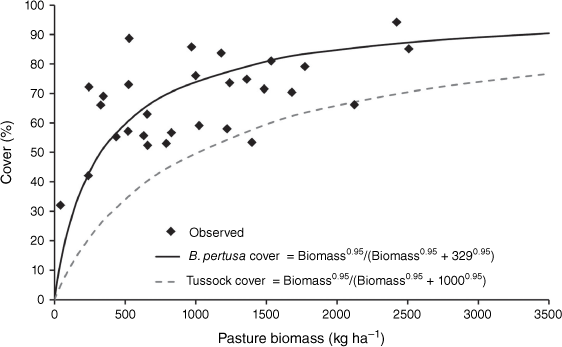
Fluctuations in biomass for Bothriochloa pertusa pastures at the study site for observed data (diamonds) with modelled interpolations (black line) compared to the biomass that would be implied from observed pasture cover alone if pastures were assumed to have the typical structure of tussock grasses (dashed line: calculated using the inverse relationship from Fig. 6 for pasture cover-vs-biomass in tussock pastures).
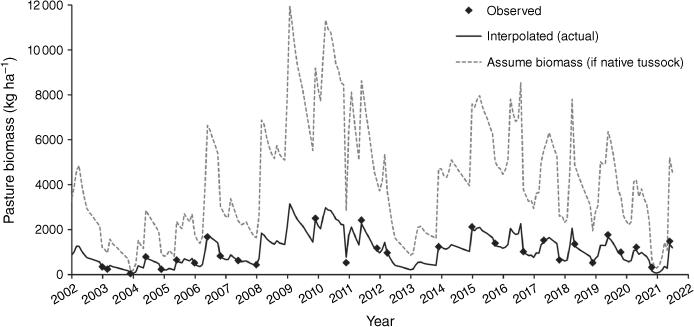
Hillslope rainfall, runoff, sediment concentrations and sediment yields
The long-term average measured rainfall for the site was 634 mm year−1. The lowest annual rainfall over the study period was 245 mm (2003), the highest 1308 mm (2008) (shown with coincident ground cover data in Fig. 8a). The measurements of runoff (Fig. 8b) and total suspended sediment concentrations (Fig. 8c) over the same period combine to generate fine sediment loads (Fig. 8d).
(a) Annual rainfall and end of dry season ground cover (%) (calibrated MODIS estimate); (b) annual runoff (mm); (c) TSS concentrations (mg L−1); and (d) fine sediment yield (t ha−1) for the flume site from 2003 to 2020. Red bars/plots represent years when cover <70% and blue bars/plots when cover >70%. All years are numbered as the starting year of that ‘water year’ (July–June). Box plots for (c) show upper quartile, median and lower quartile; whisker lines show maximum and minimum; and points show outliers.
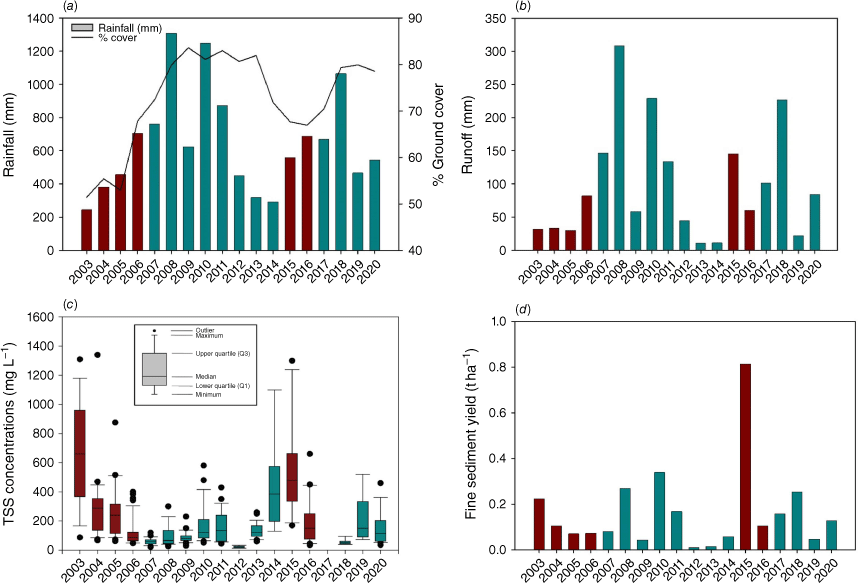
The data provide support for a previously documented functional water quality target for end of dry season ground cover of ≈70% (Sanjari et al. 2009; Bartley et al. 2014): summarising the data in Fig. 8 as the averages for years when ground cover was below the 70% threshold vs averages when the 70% threshold was exceeded showed that despite rainfall (506 mm vs 718 mm) and runoff (64 mm vs 115 mm) being substantially higher in years with >70% cover, total sediment yield (0.23 t ha−1 vs 0.13 t ha−1) and TSS concentration (318 mg L−1 vs 119 mg L−1) were reduced. However, in the 2015/2016 wet season, a single event produced 90% of the annual hillslope sediment loss when 101 mm of rain fell in an hour on the 1st of March 2016. The 70% ground cover target for this site is higher than the 40% target recommended in the region for sites with a larger proportion of tussock grasses (Ash et al. 2001).
There was a strong linear correlation between the interannual variations in runoff and rainfall (R2 = 0.87) (Fig. 9a), and a moderate relationship between the interannual variations in percent runoff and fine sediment yield (R2 = 0.47). Using multiple linear regression, including rainfall and ground cover, the addition of ground cover improved the prediction of annual hillslope runoff marginally (R2 = 0.90).
(a) Linear relationship between annual rainfall (mm) and runoff (mm) for the 18-year record at the flume site; and (b) power curve relationship between end of dry season ground cover (calibrated MODIS estimate) and the median annual measured TSS concentration. Outliers in (b) are associated with large rainfall (high intensity) events.
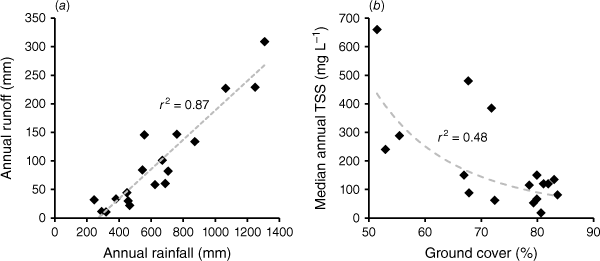
Despite a moderate relationship between median TSS concentration and ground cover (R2 = 0.48) (Fig. 9b), the average annual ground cover alone was not particularly useful at predicting year-to-year variation in sediment yield (t ha−1) (R2 < 0.2) due to the dominant influence of weather and interactions between the variables within and between years.
Modelled runoff and sediment
The calibrated GRASP curve number sub-model for runoff matched both the long-term observed average annual runoff and provided a reasonable approximation of year-to-year variations (Pearson r = 0.61) (Fig. 10a). The curve numbers that gave the best fit were 39.8 for 100% cover and 82.2 for 0% cover. This demonstrates that, despite its relatively low biomass, there was still a strong relationship between temporal changes in ground cover and runoff for B. pertusa pastures. The calibrated GRASP soil-loss sub-model matched the long-term average annual fine sediment yield but did not capture the fine-scale detailed processes that give rise to annual variations in soil loss (r = −0.09) (Fig. 10b).
Calibrations of the (a) runoff and (b) soil-loss sub-models in GRASP for annual observations vs modelled estimates. These calibrations are for year-to-year variations at a single site. The dotted line is the 1:1 relationship. Averages for annual runoff were 93.5 ± 20.1 (observed) and 95.1 ± 26.7 mm (modelled). Average annual sediment yields were 0.169 ± 0.043 (observed) and 0.168 ± 0.117 t ha−1 (modelled). Annotated biases express the modelled averages and standard errors (s.e.) as percentages of the observed values.
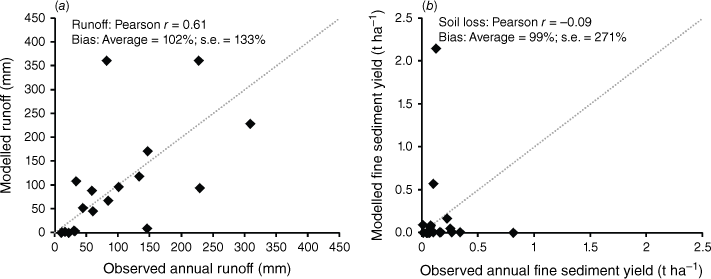
Discussion
Land condition and water quality trends
Rainfall, and associated runoff, was the dominant, natural perturbation driving year-to-year variation in sediment loads (Fig. 9). A combination of rainfall amount and the number of large (>50 mm h−1) rainfall events accounts for most of the short-term fluctuations in erosion in these systems (Dunkerley 2021), while the modifying influence of grazing management on runoff is more difficult to detect. However, the systems modelling analysis, by accounting for the complex landscape interactions, detected the influence of ground cover on runoff, as evidenced by the large differences in curve number between 0 and 100% cover. While ground cover has a relatively minor influence on the proportion of rainfall that becomes runoff at the annual time scale, it also affects suspended sediment (TSS) concentration, and thus has been shown to be an important parameter for predicting sediment loads at both hillslope (Tiwari et al. 2020) and larger catchment scales (Kuhnert et al. 2012). In fact, Kuhnert et al. (2012) found that TSS concentrations at the Burdekin catchment scale (end of system, Inkerman Bridge) decreased 2.1% for each 1% increase in ground cover, e.g. if ground cover improves by ≈10%, then TSS concentration reduces by ≈20%. Data presented here provide further evidence that ground cover does influence sediment concentrations, particularly when cover falls below 70%. In very wet years, high intensity rainfall events become more influential as the wet season progresses and can generate large amounts of runoff, regardless of the amount of cover, as the soil profile is saturated (Bartley et al. 2006; Jarihani et al. 2017). Cover is likely to exert a stronger influence on sediment loads in lower rainfall years and at the start of the wet season, or when comparing nearby properties that experience similar weather but have contrasting management.
Other plant attributes such as pasture biomass and plant basal area are likely better predictors of infiltration, and thus runoff, particularly in B. pertusa dominated rangelands (Roth 2004). There has been some recent progress in estimating biomass using remote sensing (Chen et al. 2021), however, the methods for hindcasting ground cover data using these methods and linking to historical runoff data are limited, and current approaches cannot adequately differentiate between pasture species (e.g. tussock vs stoloniferous grasses). In this regard, the data show important differences in the pasture cover–biomass relationships for pastures dominated by B. pertusa vs tussock grasses (Fig. 6), where B. pertusa pastures have far less biomass at the same cover than the pastures they replace. This would lead to overoptimistic assessments of land condition and trend (including the inferred sediment yields for a given observed ground cover) if changes in pasture composition and physiognomy are not considered. As B. pertusa expands in many grazed parts of the GBR catchments, traditional cover-based approaches to monitoring the influence of grazing land management on land condition and water quality could be compromised.
Long-term monitoring revealed several other insights into the altered functioning of transformed B. pertusa landscapes, with implications for management. Data show strong asymmetry in the rates of landscape degradation and recovery, where tussock grass regeneration rates have been far slower than their displacement rate by B. pertusa (Fig. 2). At best, there are long time lags between improved land management practices and water quality outcomes for the GBR; at worst, B. pertusa lawns are very resistant to change, and represent a transition to a relatively stable new pasture state (McIvor and Scanlan 1994; Ash et al. 2011) that needs to be managed differently to the native tussock pastures it replaced. The asymmetry in recovery vs degradation also has important implications for managing risks of adjusting stocking rates relative to (uncertain) future pasture growth, since any degradation from setting a stocking rate adjustment too high would require multiple years of intervention (with reduced stocking) for recovery. Conservative stocking rate strategies are therefore particularly favoured in land types with such asymmetric risks associated with irreversible transitions.
Comparison with other rangeland areas in the GBR catchments
The 18-year annual average fine sediment yield was ≈0.16 t ha−1 year−1, which is summarised alongside other hillslope fine sediment yields measured at grazing sites in the GBR catchments in Table 4. Given the variability in slope, rainfall, vegetation cover, grazing intensities and size of the hillslopes, it is not necessarily appropriate to compare these data, as factors such as plot area are known to affect hillslope sediment yield calculations (Ludwig et al. 2007). However, this summary does highlight how variable measured hillslope sediment yields can be between sites and years.
Catchment | Location | Length of data set (years) | Approximate grazing intensity | Fine sediment yield (t ha−1 year−1) | Reference |
---|---|---|---|---|---|
Burdekin | Upper Burdekin | ≈18 | Range ≈0.08 beasts ha−1 to ≈0.25 beasts ha−1 | 0.16 t ha−1 year−1 | This study |
Range 0.009–0.8 t ha−1 year−1 | |||||
Burdekin | Upper Burdekin | ≈10 | Range ≈0.08 beasts ha−1 to ≈0.25 beasts ha−1 | Range 0.004–≈4.0 t ha−1 year−1 (hillslopes with scald/bare areas) | Bartley et al. (2014) |
Burdekin | Upper Burdekin | ≈7 | Range ≈0.25 beasts ha−1 to ≈0.4 beasts ha−1 | 1.32 t ha−1 year−1 | Hawdon et al. (2008) |
Range 0.33–2.30 t ha−1 year−1 | |||||
Burdekin | Upper Burdekin | ≈3 | Low 0.1 beasts ha−1 to High 0.5 beasts ha−1 | 1.08 t ha−1 year−1 | McIvor et al. (1995) |
Range 0.12–1.96 t ha−1 year−1 | |||||
Burdekin | Upper Burdekin | ≈6 | No grazing + grazing, no stocking rates provided | Range ≈0.00–≈1.00 t ha−1 year−1 (total load, not fine sediment load) | Scanlan et al. (1996) |
Fitzroy | Dawson | ≈25 | Managed at industry recommended stocking rate with utilisation of no less than 1000 kg ha−1 of pasture available at any time | 0.12 t ha−1 year−1 | Elledge and Thornton (2017) |
Fitzroy | Nogoa | ≈7 | No grazing + grazing, no stocking rates provided | Range ≈0.00–≈18.00 t ha−1 year−1 (yield varied with cover and soil type, with highest yields for sandstone and mudstone with 0% cover). Noting slopes were steeper at ≈15% | Silburn (2011a) |
Murray-Darling Basin | Treverton Creek | 6 | Comparison of time-controlled (TC) and continuous (Cont) grazing. Stocking rate (SR) for TC = ≈12.6 DSE ha−1 for 12 days then 100 days rest. Cont SR = 1.6 DSE ha−1 | Range 0.44–2.06 t ha−1 year−1 | Sanjari et al. (2009) |
DSE, Dry sheep equivalent.
Erosion rates derived using cosmogenic beryllium (10Be isotope) suggest that the pre-grazing baseline representing the long-term average erosion rate (≈100 to >10 000 years) for this site is likely to be ≈0.27 t ha−1 year−1 (Bartley et al. 2015). Therefore, the contemporary ≈18-year average erosion rate from this flume hillslope site is within the range of tolerable or acceptable erosion rates (Montgomery 2007; Bui et al. 2011). However, data presented in Table 4 demonstrate that many hillslopes in the GBR catchments, including adjacent hillslopes on this property, have average hillslope soil erosion rates several times higher than this baseline value, particularly where there are large bare or scalded areas void of vegetation (Bartley et al. 2014).
Silburn et al. (2011) captured many of the key factors driving hillslope erosion rates in the rangelands draining to the GBR, including the significance of the 50–70% ground cover threshold required to reduce hillslope soil erosion and runoff, the difference between erosion rates predicted from stoloniferous and tussock grasses, the dominance of low-cover bare patches and large rainfall events on erosion rates, and the time lags associated with improved ground cover and reduced erosion rates.
Model calibration and evaluation
Given the large natural variability represented in hillslope erosion measurements in grazed rangelands (Table 4), and the complex interactions and feedbacks among variables, it is difficult to attribute measured changes in hillslope sediment yields to changed grazing management in real time. Robust models are therefore very useful for providing insights and scenario analysis into the potential effects of rangeland management interventions.
The main method for assessing investments in the GBR catchments is the SourceCatchments model (Wilkinson et al. 2014b; McCloskey et al. 2021). The key sub-modelling tool used to estimate sediment loss from grazed hillslopes within the SourceCatchments model is the Revised Universal Soil Loss Equation (RUSLE) (Renard et al. 1994; Rosewell and Loch 2002; Silburn 2011b). Numerous publications outline how the RUSLE is not necessarily suitable for rangeland environments (Weltz et al. 1998; Spaeth et al. 2003); primarily due to the higher levels of heterogeneity in soils and plant properties at the plot scale, relative to cropping landscapes (Nearing et al. 2011). Another challenge is that RUSLE does not explicitly consider runoff. GBR specific studies have also identified that the RUSLE generally over-predicts hillslope erosion (Hughes and Croke 2011; Brooks et al. 2014). Bartley et al. (2006), and other studies, have determined that biomass and the arrangement of ground cover are likely better predictors of runoff than average hillslope cover, although it is unlikely that these attributes will replace average ground cover in predictive models any time soon. Despite these findings, RUSLE continues to be used in the GBR due to its utility and ability to parameterise models that can analyse large spatial areas using sparse data sets. The RUSLE is considered broadly suitable as a prioritisation or ranking tool when used in a relative sense for comparing hillslope erosion rates between regions, acknowledging that there may be high prediction error at any one location (Alewell et al. 2019). However, this means that it may be problematic to apply the SourceCatchments model and associated tools in accounting and financial payment frameworks (e.g. Reef Credits). This is because these models have relatively high uncertainty, compared to on-ground measured data, when estimating runoff or sediment loss at small spatial scales. There is therefore a need to consider incorporating a broader suite of modelling tools for GBR rangelands, such as GRASP (or its component sub-models).
This study is the first to apply the GRASP model to a B. pertusa dominated commercial grazing property. When calibrated using local data on soils and pastures, GRASP performed well at representing long-term average runoff and sediment yield (both within 2% of observed; Fig. 10), but was only able to adequately represent the year-to-year variation in runoff (Pearson r = 0.61), and not fine scale variations in sediment. Erosion models (and complex full-systems models like GRASP) are more suited to representing long-term, broadscale average behaviours. The GRASP calibration in this paper represents a single data point (capturing long-term variation at a single spatial location). A larger number of water quality data sets, from sites with contrasting management and ground cover, would be required to properly evaluate how well GRASP and RUSLE (or other alternatives) can capture the effects of different grazing land management across the GBR catchments (at paddock to catchment scales).
The calibrated model provides valuable insights into what further large-scale encroachment of B. pertusa into existing tussock pastures would mean for GBR catchments. Given the strongly altered physiognomy when tussock pastures are replaced by B. pertusa (as characterised by the contrasting pasture cover–biomass curve relationships), a scaling factor may need to be considered when modelling water quality from un-calibrated B. pertusa dominated landscapes. These changes will have important implications for sites currently being modelled under the Paddock to Reef program (Carroll et al. 2012) given the increasing and pervasive presence of B. pertusa across large parts of the grazing areas draining to the GBR lagoon. The findings presented here will also improve the ability to model how landscape regeneration from changed grazing land management affects runoff and water quality, including being able to evaluate revised guidelines for safe stocking rates (that incorporate the consequences of the asymmetric rates of land degradation and recovery).
Conclusions
This study of a long-term monitoring site has shown major functional differences in B. pertusa pastures, relative to the native tussock pastures they replace, with important implications (both for beef production and GBR water quality) for how they are managed and modelled, and how monitoring data are interpreted:
Rates of regeneration in land condition are much slower than the rates at which B. pertusa initially replaced tussock grasses. In the practical time frames of most land managers (10–20 years), degradation to a B. pertusa state is effectively irreversible. The risks of ‘irreversible’ pasture transitions from management decisions when stocking rates are adjusted too high, suggests that conservative stocking rate strategies would be particularly favoured in these land types. Where transitions do occur, B. pertusa pastures need to be managed as a new land type, accounting for the functional changes that have occurred.
Changes in species composition and vegetation structure can lead to misinterpretation of monitored trends in ground cover (which is often used as an economical proxy for land condition). Where B. pertusa is invading, inferences from positive associations between changes in cover and landscape function can break down: increases in ground cover can instead be accompanied by decreases in biomass, productivity, and sediment retention.
The paper contributes an initial GRASP model calibration for a transformed B. pertusa site, capturing the observed relationships between grazing management, pasture biomass, ground cover and water quality. This serves as a starting point from which these landscapes, of growing extent and concern in GBR catchments, can be better represented in monitoring and modelling assessments, and for revised management options to be explored.
Data availability
The data will be made available on the CSIRO Data Access Portal (https://data.csiro.au/).
Acknowledgements
The research presented in this publication was funded by Meat and Livestock Australia, CSIRO, eWater CRC, Queensland Government Paddock to Reef Program, and the Great Barrier Reef Foundation; their support is gratefully acknowledged. We also thank Rob and Sue Bennetto on ‘Virginia Park’ Station for access to their property over 20 years to carry out this work. This site has received input from many research and support staff over two decades. Thanks to Christian Roth, Jeff Corfield, Peter Fitch, Rex Keen, David Fanning, Jamie Vleeshouwer, Joseph Kemei, Lindsay Whiteman, Scott Wilkinson, Brett Baker, Kahlytah Ahwang and Kahill Mitchell of CSIRO and the late Peter Allen. Thanks also to Queensland Government Paddock to Reef Soil Science Team for the collection of soil cores, and to Michelle Tink at the James Cook University TropWater Labs for water sample analysis and to Bob Shepherd for advice on stocking rate data. Acknowledgement is made to the Queensland Government who are the custodians of the GRASP code and provide ongoing model testing and improvement. Thankyou also to three anonymous reviewers, whose comments assisted in improving this manuscript.
References
ALA (2022) Atlas of Living Australia occurrence. (Ed. A.o.L. Australia). 10.26197/ala.679d0699-11f5-4d76-a11a-87c922e04622 [accessed 18 July 2022]
Alewell C, Borrelli P, Meusburger K, Panagos P (2019) Using the USLE: chances, challenges and limitations of soil erosion modelling. International Soil and Water Conservation Research 7(3), 203-225.
| Crossref | Google Scholar |
APNI (2022) Australian Plant Name Index. Available at https://www.anbg.gov.au/apni/index.html
Ash AJ, Corfield JP, McIvor JG, Ksiksi TS (2011) Grazing management in tropical savannas: utilization and rest strategies to manipulate rangeland condition. Rangeland Ecology & Management 64(3), 223-239.
| Crossref | Google Scholar |
AVH (2022) The Australasian Virtual Herbarium, Council of Heads of Australasian Herbaria. Available at https://avh.chah.org.au [accessed 18 July 2022]
Bartley R, Roth CH, Ludwig J, McJannet D, Liedloff A, Corfield J, Hawdon A, Abbott B (2006) Runoff and erosion from Australia’s tropical semi-arid rangelands: influence of ground cover for differing space and time scales. Hydrological Processes 20(15), 3317-3333.
| Crossref | Google Scholar |
Bartley R, Hawdon A, Post DA, Roth CH (2007) A sediment budget for a grazed semi-arid catchment in the Burdekin basin, Australia. Geomorphology 87(4), 302-321.
| Crossref | Google Scholar |
Bartley R, Corfield JP, Abbott BN, Hawdon AA, Wilkinson SN, Nelson B (2010) Impacts of improved grazing land management on sediment yields, Part 1: hillslope processes. Journal of Hydrology 389(3–4), 237-248.
| Crossref | Google Scholar |
Bartley R, Corfield JP, Hawdon AA, Kinsey-Henderson AE, Abbott BN, Wilkinson SN, Keen RJ (2014) Can changes to pasture management reduce runoff and sediment loss to the Great Barrier Reef? The results of a 10-year study in the Burdekin catchment, Australia. The Rangeland Journal 36(1), 67-84.
| Crossref | Google Scholar |
Bartley R, Croke J, Bainbridge ZT, Austin JM, Kuhnert PM (2015) Combining contemporary and long-term erosion rates to target erosion hot-spots in the Great Barrier Reef, Australia. Anthropocene 10, 1-12.
| Crossref | Google Scholar |
Bartley R, Abbott BN, Ghahramani A, Ali A, Kerr R, Roth CH, Kinsey-Henderson A (2022) Do regenerative grazing management practices improve vegetation and soil health in grazed rangelands? Preliminary insights from a space-for-time study in the Great Barrier Reef catchments, Australia. The Rangeland Journal 44, 221-246.
| Crossref | Google Scholar |
Bastin GN, Pickup G, Stanes J, Stanes A (1996) Estimating landscape resilience from satellite data and its application to pastoral land management. The Rangeland Journal 18(1), 118-135.
| Crossref | Google Scholar |
Bastin G, Scarth P, Chewings V, Sparrow A, Denham R, Schmidt M, O’Reagain P, Shepherd R, Abbott B (2012) Separating grazing and rainfall effects at regional scale using remote sensing imagery: a dynamic reference-cover method. Remote Sensing of Environment 121, 443-457.
| Crossref | Google Scholar |
Briske DD, Sayre NF, Huntsinger L, Fernandez-Gimenez M, Budd B, Derner JD (2011) Origin, persistence, and resolution of the rotational grazing debate: integrating human dimensions into rangeland research. Rangeland Ecology & Management 64(4), 325-334.
| Crossref | Google Scholar |
Brooks A, Spencer J, Borombovits D, Pietsch T, Olley J (2014) Measured hillslope erosion rates in the wet-dry tropics of Cape York, northern Australia: Part 2, RUSLE-based modeling significantly over-predicts hillslope sediment production. Catena 122, 1-17.
| Crossref | Google Scholar |
Bui EN, Hancock GJ, Wilkinson SN (2011) ‘Tolerable’ hillslope soil erosion rates in Australia: linking science and policy. Agriculture, Ecosystems & Environment 144(1), 136-149.
| Crossref | Google Scholar |
Carroll C, Waters D, Vardy S, Silburn DM, Attard S, Thorburn PJ, Davis AM, Halpin N, Schmidt M, Wilson B, Clark A (2012) A paddock to reef monitoring and modelling framework for the Great Barrier Reef: paddock and catchment component. Marine Pollution Bulletin 65(4–9), 136-49.
| Crossref | Google Scholar |
Chen Y, Guerschman J, Shendryk Y, Henry D, Harrison MT (2021) Estimating pasture biomass using Sentinel-2 imagery and machine learning. Remote Sensing 13(4), 603.
| Crossref | Google Scholar |
De’ath G, Fabricius K (2010) Water quality as a regional driver of coral biodiversity and macroalgae on the Great Barrier Reef. Ecological Applications 20(3), 840-850.
| Crossref | Google Scholar |
Dunkerley D (2021) Rainfall intensity in geomorphology: challenges and opportunities. Progress in Physical Geography: Earth and Environment 45(4), 488-513.
| Crossref | Google Scholar |
Elledge A, Thornton C (2017) Effect of changing land use from virgin brigalow (Acacia harpophylla) woodland to a crop or pasture system on sediment, nitrogen and phosphorus in runoff over 25 years in subtropical Australia. Agriculture, Ecosystems & Environment 239, 119-131.
| Crossref | Google Scholar |
Gardener CJ, McIvor J, Williams J (1990) Dry tropical rangelands: solving one problem and creating another. Proceedings of the Ecological Society of Australia 16, 279-286.
| Google Scholar |
Ghahramani A, Freebairn DM, Sena DR, Cutajar JL, Silburn DM (2020) A pragmatic parameterisation and calibration approach to model hydrology and water quality of agricultural landscapes and catchments. Environmental Modelling & Software 130, 104733.
| Crossref | Google Scholar |
Gosnell H, Grimm K, Goldstein BE (2020) A half century of Holistic Management: what does the evidence reveal? Agriculture and Human Values 37, 849-867.
| Crossref | Google Scholar |
Guerschman J (2020) ‘Fractional Cover - MODIS, CSIRO algorithm. Version 1.0.’ (Terrestrial Ecosystem Research Network (TERN)) Available at http://geonetwork.tern.org.au/geonetwork/srv/eng/catalog.search#/metadata/a6ef2480-e397-4c42-9706-3865dd6ca380 [accessed 18 September 2022]
Hall TJ, McIvor JG, Reid DJ, Jones P, MacLeod ND, McDonald CK, Smith DR (2014) A comparison of stocking methods for beef production in northern Australia: pasture and soil surface condition responses. The Rangeland Journal 36(2), 161-174.
| Crossref | Google Scholar |
Hughes AO, Croke JC (2011) Validation of a spatially distributed erosion and sediment yield model (SedNet) with empirically derived data from a catchment adjacent to the Great Barrier Reef Lagoon. Marine and Freshwater Research 62(8), 962-973.
| Crossref | Google Scholar |
Humanes A, Fink A, Willis BL, Fabricius KE, de Beer D, Negri AP (2017) Effects of suspended sediments and nutrient enrichment on juvenile corals. Marine Pollution Bulletin 125(1–2), 166-175.
| Crossref | Google Scholar |
Hunt LP, McIvor JG, Grice AC, Bray SG (2014) Principles and guidelines for managing cattle grazing in the grazing lands of northern Australia: stocking rates, pasture resting, prescribed fire, paddock size and water points – a review. The Rangeland Journal 36(2), 105-119.
| Crossref | Google Scholar |
Jarihani B, Sidle RC, Bartley R, Roth CH, Wilkinson SN (2017) Characterisation of hydrological response to rainfall at multi spatio-temporal scales in savannas of semi-arid Australia. Water 9, 540.
| Crossref | Google Scholar |
Jeffrey SJ, Carter JO, Moodie KB, Beswick AR (2001) Using spatial interpolation to construct a comprehensive archive of Australian climate data. Environmental Modelling & Software 16, 309-330.
| Crossref | Google Scholar |
Karfs RA, Abbott BN, Scarth PF, Wallace JF (2009) Land condition monitoring information for reef catchments: a new era. The Rangeland Journal 31, 69-86.
| Crossref | Google Scholar |
Koci J, Sidle RC, Kinsey-Henderson AE, Bartley R, Wilkinson SN, Hawdon AA, Jarihani B, Roth CH, Hogarth L (2020) Effect of reduced grazing pressure on sediment and nutrient yields in savanna rangeland streams draining to the Great Barrier Reef. Journal of Hydrology 582, 124520.
| Crossref | Google Scholar |
Koci J, Wilkinson SN, Hawdon AA, Kinsey-Henderson AE, Bartley R, Goodwin NR (2021) Rehabilitation effects on gully sediment yields and vegetation in a savanna rangeland. Earth Surface Processes and Landforms 46(5), 1007-1025.
| Crossref | Google Scholar |
Kuhnert PM, Henderson BL, Lewis SE, Bainbridge ZT, Wilkinson SN, Brodie JE (2012) Quantifying total suspended sediment export from the Burdekin River catchment using the Loads Regression Estimator tool. Water Resources Research 48, W04533.
| Crossref | Google Scholar |
Kutt AS, Fisher A (2011) Increased grazing and dominance of an exotic pasture (Bothriochloa pertusa) affects vertebrate fauna species composition, abundance and habitat in savanna woodland. The Rangeland Journal 33(1), 49-58.
| Crossref | Google Scholar |
Kutt AS, Kemp JE (2012) Native plant diversity in tropical savannas decreases when exotic pasture grass cover increases. The Rangeland Journal 34(2), 183-189.
| Crossref | Google Scholar |
Lambert V, Bainbridge ZT, Collier C, Lewis SE, Adams MP, Carter A, Saunders MI, Brodie J, Turner RDR, Rasheed MA, O’Brien KR (2021) Connecting targets for catchment sediment loads to ecological outcomes for seagrass using multiple lines of evidence. Marine Pollution Bulletin 169, 112494.
| Crossref | Google Scholar |
Lebbink G, Fensham R (2023) The ‘lawnification’ of Australia’s eastern grassy woodlands: the past, current and likely future spread of an invasive perennial grass, Bothriochloa pertusa. Biological Invasions 25, 1779-1794.
| Crossref | Google Scholar |
Lebbink G, Dwyer JM, Fensham RJ (2022) ‘Invasion debt’ after extensive land-use change: an example from eastern Australia. Journal of Environmental Management 302, 114051.
| Crossref | Google Scholar |
Leuning R, Cleugh HA, Zegelin SJ, Hughes D (2005) Carbon and water fluxes over a temperate Eucalyptus forest and a tropical wet/dry savanna in Australia: measurements and comparison with MODIS remote sensing estimates. Agricultural and Forest Meteorology 129(3–4), 151-173.
| Crossref | Google Scholar |
Lewis SE, Bartley R, Wilkinson SN, Bainbridge ZT, Henderson AE, James CS, Irvine SA, Brodie JE (2021) Land use change in the river basins of the Great Barrier Reef, 1860 to 2019: a foundation for understanding environmental history across the catchment to reef continuum. Marine Pollution Bulletin 166, 112193.
| Crossref | Google Scholar |
Littleboy M, McKeon G (1997) Subroutine GRASP: Grass production model, documentation of the Marcoola version of Subroutine GRASP. Appendix 2 of ‘Evaluating the risks of pasture and land degradation in native pasture in Queensland’. Final Project Report for Rural Industries and Research Development Corporation project DAQ124A. (Queensland Department of Natural Resources: Brisbane, Qld, Australia)
Ludwig JA, Bartley R, Hawdon AA, Abbott BN, McJannet D (2007) Patch configuration non-linearly affects sediment loss across scales in a grazed catchment in north-east Australia. Ecosystems 10(5), 839-845.
| Crossref | Google Scholar |
McCloskey GL, Baheerathan R, Dougall C, Ellis R, Bennett FR, Waters D, Darr S, Fentie B, Hateley LR, Askildsen M (2021) Modelled estimates of fine sediment and particulate nutrients delivered from the Great Barrier Reef catchments. Marine Pollution Bulletin 165, 112163.
| Crossref | Google Scholar |
McIvor JG, Scanlan JC (1994) State and transition models for rangelands. 8. A state and transition model for the Northern Speargrass Zone. Tropical Grasslands 28(4), 256-259.
| Google Scholar |
McIvor JG, Williams J, Gardener CJ (1995) Pasture management influences runoff and soil movement in the semi-arid tropics. Australian Journal of Experimental Agriculture 35(1), 55-65.
| Crossref | Google Scholar |
McKeon GM, Day KA, Howden SM, Mott JJ, Orr DM, Scattini WJ, Weston EJ (1990) Northern Australian Savannas: management for pastoral production. Journal of Biogeography 17(4–5), 355-372.
| Crossref | Google Scholar |
Montgomery DR (2007) Soil erosion and agricultural sustainability. Proceedings of the National Academy of Sciences 104(33), 13268-13272.
| Crossref | Google Scholar |
Nearing MA, Wei H, Stone JJ, Pierson FB, Spaeth KE, Weltz MA, Flanagan DC, Hernandez M (2011) A rangeland hydrology and erosion model. Transactions of the ASABE 54(3), 901-908.
| Crossref | Google Scholar |
O’Reagain P, Bushell J, Holmes B (2011) Managing for rainfall variability: long-term profitability of different grazing strategies in a northern Australian tropical savanna. Animal Production Science 51(3), 210-224.
| Crossref | Google Scholar |
Owens JS, Silburn DM, McKeon GM, Carroll C, Willcocks J, deVoil R (2003) Cover-runoff equations to improve simulation of runoff in pasture growth models. Australian Journal of Soil Research 41(8), 1467-1488.
| Crossref | Google Scholar |
Pickup G, Chewings VH, Nelson DJ (1993) Estimating changes in vegetation cover over time in arid rangelands using landsat MSS data. Remote Sensing of Environment 43, 243-263.
| Crossref | Google Scholar |
Queensland Government (2022) Virginia Park flume site soil morphology and chemistry. Available at https://resources.information.qld.gov.au/soils/reports/sites?project=P2REEF&site=1
Renard KG, Foster GR, Yoder DC, McCool DK (1994) RUSLE revisited: status, questions, answers, and the future. Journal of Soil and Water Conservation 49(3), 213-220.
| Google Scholar |
Rickert KG, McKeon GM (1988) Computer models of forage management on beef cattle farms. Mathematics and Computers in Simulation 30(1–2), 189-194.
| Crossref | Google Scholar |
Rose CW (1985) Developments in soil erosion and deposition models. In ‘Advances in soil science, vol. 2.’ (Ed. BA Stewart) pp. 1–64. https://doi.org/10.1007/978-1-4612-5088-3_1
Roth CH (2004) A framework relating soil surface condition to infiltration and sediment and nutrient mobilization in grazed rangelands of northeastern Queensland, Australia. Earth Surface Processes and Landforms 29, 1093-1104.
| Crossref | Google Scholar |
Sanjari G, Yu B, Ghadiri H, Ciesiolka CAA, Rose CW (2009) Effects of time-controlled grazing on runoff and sediment loss. Australian Journal of Soil Research 47(8), 796-808.
| Crossref | Google Scholar |
Scanlan JC, Pressland AJ, Myles DJ (1996) Run-off and soil movement on mid-slopes in North-east Queensland [Australia] grazed woodlands. The Rangeland Journal 18, 33-46.
| Crossref | Google Scholar |
Silburn DM (2011a) Hillslope runoff and erosion on duplex soils in grazing lands in semi-arid central Queensland. II. Simple models for suspended and bedload sediment. Soil Research 49(2), 118-126.
| Crossref | Google Scholar |
Silburn DM (2011b) Hillslope runoff and erosion on duplex soils in grazing lands in semi-arid central Queensland. III. USLE erodibility (K factors) and cover–soil loss relationships. Soil Research 49(2), 127-134.
| Crossref | Google Scholar |
Silburn DM, Carroll C, Ciesiolka CAA, deVoil RC, Burger P (2011) Hillslope runoff and erosion on duplex soils in grazing lands in semi-arid central Queensland. I. Influences of cover, slope, and soil. Soil Research 49(2), 105-117.
| Crossref | Google Scholar |
Spaeth KE, Pierson FB, Weltz MA, Blackburn WH (2003) Evaluation of USLE and RUSLE estimated soil loss on rangeland. Journal of Range Management 56(3), 234-246.
| Crossref | Google Scholar |
Teague R, Provenza F, Kreuter U, Steffens T, Barnes M (2013) Multi-paddock grazing on rangelands: why the perceptual dichotomy between research results and rancher experience? Journal of Environmental Management 128, 699-717.
| Crossref | Google Scholar |
Tiwari J, Yu B, Fentie B, Ellis R (2020) Probability distribution of groundcover for runoff prediction in rangeland in the Burnett–Mary Region, Queensland. The Rangeland Journal 42(2), 97-112.
| Crossref | Google Scholar |
Weltz MA, Kidwell MR, Fox HD (1998) Influence of abiotic and biotic factors in measuring and modeling soil erosion on rangelands: state of knowledge. Journal of Range Management 51(5), 482-495.
| Crossref | Google Scholar |
Wilkinson SN, Bastin G, Stokes CJ, Hawdon AA, Chewings VH, Kinsey-Henderson AE, Nicholas DM, Abbott BN, McKellar K, Kemei J (2014a) ‘Improving grazing management practices to enhance ground cover and reduce sediment loads.’ Report to the Reef Rescue Water Quality Research & Development Program. (Reef and Rainforest Research Centre Limited: Cairns, QLD, Australia)
Wilkinson SN, Dougall C, Kinsey-Henderson AE, Searle R, Ellis R, Bartley R (2014b) Development of a time-stepping sediment budget model for assessing land use impacts in large river basins. Science of the Total Environment 468–469, 1210-1224.
| Crossref | Google Scholar |