Above-ground carbon in mulga-dominated rangelands, Murchison Bioregion, Western Australia
Adrian Williams A * , Peter Russell B and David Blood CA
B
C
Abstract
The paper reports outcomes of surveys conducted between 2012 and 2014 in mulga (Acacia aneura) dominated arid rangelands of the Murchison Bioregion of Western Australia, approximately 470 km north-east of Perth, the State capital. The bioregion covers 281,200 km2. The purpose of the surveys was to measure the stocks of above-ground biomass (AGB) and sequestered organic carbon within 140 plots, each 25 m × 25 m. The resulting large database was interrogated to (1) find a relationship between AGB and contained carbon in trees and in shrubs, and (2) find plant metrics to predict AGB. At the plant level, analysis confirmed the finding that tree AGB was composed of a mean 47% carbon, with shrub AGB averaging 46%. This allowed biomass to be used as a surrogate for carbon in our further analysis. Tree canopy area was found to provide the best linear relationship with tree and shrub AGB. The linear relationships held true for individual plants across the survey sites and across the land systems involved. At the plot level, measures of AGB and carbon stocks varied across land systems. Because tree canopy can be measured by remote-sensing, the results of these field-only surveys indicated a potential for AGB and carbon in mulga-dominated rangelands to be assessed remotely.
Keywords: carbon estimation through remote sensing, carbon percentage of biomass, mulga rangelands, Murchison Bioregion, plant morphological metrics, total above ground biomass, tree and shrub biomass estimation, Western Australia.
Introduction
Interest in carbon farming to mitigate rising greenhouse-gas concentrations in the atmosphere and to diversify income streams has been growing for many years in the Western Australian (WA) rangelands. At the turn of the century, carbon sequestration on mine dumps as well as rangelands was being promoted (Williams 2000). Baumber et al. (2020) showed where carbon projects were located across Australia in early 2020 and, in addition to the CO2-mitigation benefit, emphasised the socio-ecological co-benefits that should improve the sustainability and resilience of rangeland communities and enterprises. The comprehensive paper by Nielsen et al. (2020) described the various pressures being placed on Australia’s rangelands, including its communities, industries, and environment, and described ways to address or mitigate the pressures. Russell-Smith et al. (2015) provided an example of how carbon markets are aiding sustainable land-management programs in fire-prone savanna in northern Australia. Others have lobbied for holistic approaches to carbon farming (Carbon Market Institute 2021).
Until recently, the main avenue for government funding of carbon farming initiatives in the rangelands specified the human-induced regeneration (HIR) method that concentrated on carbon sequestered in trees. However, this method was more suited to plantation forestry than the diverse forms of rangeland plants. The HIR method is being retired (Department of Climate Change, Energy, the Environment and Water 2023) in favour of a ‘whole of landscape’ approach, allowed under Section 6 of the Paris Agreement, and where carbon credits can be traded internationally. The new trading formats are aimed at both enhancing climate-mitigation ambitions at relatively low costs and channelling more climate finance into land use (von Unger and Emmer 2018), while developing improved standards, validation, and disclosure rules (Carbon Market Institute 2023).
In whatever way carbon-farming initiatives are established, they require reliable methods for assessment of initial and future carbon stocks, whether that be for trees alone or a whole of landscape approach. Because of the potential scale required for verification audits for future rangeland-based projects and the heterogeneity of the vegetation even within species, future, regular ground-truthing at plot level is likely to be limited to collecting further data to build models and relationships between remotely sensed vegetation metrics and carbon content. Models have been developed to estimate the volume increases of forests and woodland, but these projections have concentrated in the more productive, higher-rainfall parts of Australia (Turner et al. 1999; Paul et al. 2011). The WA rangelands may be less productive per square kilometre than are farming regions with higher rainfall, but they cover vast areas, and might be expected in the aggregate to be sequestering large quantities of carbon. Sequestration in selected parts of the rangelands might be enhanced with land-management techniques, such as those that detain rainfall and overland flow and increase stored soil moisture.
Grierson et al. (2000) compiled a review of unpublished biomass-related information from Western Australia, South Australia, New South Wales and Queensland that adds to public knowledge of relationships among plant morphological metrics, contained biomass and carbon. Yet, none of this seems to address mulga (Acacia aneura, Turland et al. 2018) rangelands in WA in the <300 mm mean annual rainfall zone. Specific to the WA rangelands, Adams et al. (2001) quantified stocks of biomass and carbon stored in types of Pilbara vegetation, including open mulga woodland. However, there is a lack of information for the mulga rangelands in WA south of the Pilbara and west and north of the Goldfields eucalyptus woodlands. In their fig. 21 of ‘The Distribution of Open Woodland across Australia by Net Primary Productivity’, Landsberg and Kesteven (2002) showed little data for this large area. The Western Australian Rangeland Monitoring System (WARMS) has been fully in place since 1993, but it concentrates on vegetation of height of <2.05 m, in the grazing/browsing zone (Russell 2007; Watson et al. 2007a). However, WARMS can be used to assess the health and recruitment of rangeland plants. Watson et al. (2007b) reported that between 1993 and 2005, most species present on WARMS sites, including mulga, showed increases in density and canopy area, and recruitment of new individuals was common. A Japanese project in the north-eastern Goldfields north of Leonora, WA, described by Yamada et al. (1999) and Taniguchi et al. (1998), has been productive in identifying relationships between plant metrics and biomass. Shiono et al. 2006) developed allometric equations for natural growth in a mulga woodland and for growth of planted Eucalyptus camaldulensis on a de-compacted (drilled and blasted), water-ponded, hard-pan area on an old stock route. Suganuma et al. (2006) examined areas of Halosarcia doleiformis (samphire) and developed allometric equations and relationships among canopy coverage, leaf area index and stand biomass.
The surveys reported in this paper have sought to extend knowledge of the relationships between above-ground biomass (AGB) and carbon on 625 m2 plots in the mulga-dominated WA rangelands of the Murchison region. The analysis aimed to elucidate reliable statistical relationships between biomass and plant morphological metrics for combined species of either trees or shrubs. Here we take tree species to be those with the potential to grow to more than 2 m in height, and shrub species to be those unlikely to reach 2 m. The intention was to provide data that will be of use in the development of future carbon modelling or for carbon assessment through remote-sensing techniques (e.g. Porfirio et al. 2020). In addition, ‘whole of landscape’ AGB has been estimated on a plot basis to identify site differences.
Methods
Field methods
The surveys were conducted on three rangeland properties in the Murchison Bioregion of WA (Environment Australia 2000), approximately 470 km north–north-east of Perth, the WA State capital. The Bioregion has been described by the Department of Climate Change, Energy, the Environment and Water (nd). The largest town in the area is Mount Magnet. Its mean annual rainfall over the period 1898–2010 was 239.1 mm (range: 73.4–642 mm). Rain can occur in all months, but is usually highest in late summer, autumn and winter (Bureau of Meteorology, Government of Australia 2023). These open, arid, mulga-dominated rangelands contain a range of Acacia and other species over a mixed shrub understorey with ephemeral, seasonally opportunistic, herbs and grasses. The latter comprise a very small proportion of the biomass. The region has been grazed for over 100 years, mainly by sheep, but also by native and feral herbivores as water resources developed. Recently, cattle have replaced sheep. Prior to the advent of pastoralism, the area was occupied by nomadic Aboriginal peoples for thousands of years. Fig. 1. provides views of these rangelands.
The properties in the surveys were the former Muggon pastoral station (now a conservation reserve), including 80 plots, and Meka and Yoweragabbie pastoral stations, with 30 plots each. The area and survey locations are shown on Fig. 2, and the properties, land systems, numbers of plots and survey dates are given in Table 1. Muggon and Meka were surveyed a second time to collect additional data on ‘standing dead’ trees.
Survey site | Land system | Plots (n) | Survey date | Re-survey date | |
---|---|---|---|---|---|
Muggon | Boulder = B | 33 | April–May 2012 | July 2014 | |
Muggon | Holmwood = H | 27 | April–May 2012 | July 2014 | |
Muggon | Sherwood = S | 20 | April–May 2012 | July 2014 | |
Meka | Yanganoo = Y | 30 | May 2013 | July 2014 | |
Yoweragabbie | Woodline = W | 26 | August 2014 | ||
Yoweragabbie | Sherwood = S | 4 | August 2014 |
The surveys were located on five land systems. A definition of a land system is warranted. A land system is defined as an area, or group of areas, throughout which a recurring pattern of geology, topography, soils, and vegetation can be recognised, and these reflect land capability (Christian and Stewart 1946). Twelve suitable land systems, mostly mulga-dominated, were initially identified, on which biomass characterisation studies could be undertaken. The selection identified land systems that (a) were extensive in the pastoral zone and (b) had moderate to high future carbon sequestration potential. A two-dimensional matrix was constructed to identify land systems that best met these two important criteria. The owners and managers of the pastoral stations on which these land systems occurred were then approached to ascertain their interest in hosting the surveys. Three properties, namely Muggon, Meka and Yoweragabbie, were most amenable, resulting in a final selection of five land systems, namely Boulder (B), Holmwood (H) and Sherwood (S) land systems on Muggon, Yanganoo (Y) land system on Meka and Sherwood (S) and Woodline (W) land systems on Yoweragabbie. Importantly, Muggon had been de-stocked for approximately 10 years in preparation for it becoming a formal conservation reserve. Sherwood land system occurred on two stations, Yoweragabbie and Muggon, some 250 km apart. It was anticipated that would allow spatio-temporal comparison to be undertaken.
Descriptions of the named land systems in the survey areas are provided in Table 2 (after Curry et al. 1994). (See also Payne et al. (1987, 1998) and Tille (2006, pp. 82–86).)
Land system | Description | |
---|---|---|
Boulder (B) | Granitic and stony mulga (Acacia aneura) shrubland or mixed mulga–snakewood (A. xiphophylla) shrubland distributed on plateaux, stony slopes, elevated plains, lower saline plains and drainage floors. Land units are: (1) low hills and breakaways (10%); (2) stony plains (60%); (3) sand sheets (5%); (4) saline lower plains (20%); (5) drainage floors (5%). | |
Holmwood (H) | Bluebush (Maireana pyramidata) and saltbush (Atriplex bunburyana or A. vesicaria) with tall shrubs up to 6 m (mainly Acacia xiphophylla and A. ramulosa) on lower saline plains, alluvial plains, low breakaways, plateaux and footslopes, stony plains and sandy banks. Land units are: (1) low breakaways, plateaux and footslopes (10%); (2) sandy banks (5%); (3) saline stony plains (10%); (4) alluvial plains (35%); (5) lower saline plains (40%). | |
Sherwood (S) | Granitic mulga (Acacia aneura) shrubland with A. grasbyi, A. tetragonophylla and A. victoriae with low storey comprising bluebush, saltbush or mixed halophytic shrubs, mainly on gently undulating plains and drainage interfluves. Land units are: (1) plateaux edges, buttes and breakaways (10%); (2) low hills, rises and tor fields (5%); (3) footslope plains (10%); (4) sandy alluvial fans (5%); (5) gritty-surfaced plains on granite (15%); (6) stony plains and drainage interfluves (45%); (7) drainage tracts and channels (10%). | |
Woodline (W) | Extensive hardpan mulga shrubland with tracts of mulga grove woodlands and sandplain acacia shrubland dominated by mulga (Acacia aneura) or wanyu (A. ramulosa). Land units are: (1) sandplains (10%); (2) hardpan plains (80%); (3) drainage tracts (10%). | |
Yanganoo (Y) | Extensive hardpan mulga (Acacia aneura) shrubland plains including mulga groves, with A. pruinocarpa, A. ramulosa and Eucalyptus dichromophloia, and low storey halophytic and non-halophytic shrubs. Land units are: (1) sand plains (2%); (2) wanderrie banks (10%); (3) hard-pan plains (80%); (4) mulga groves (4%); (5) drainage tracts (4%). |
On the first survey (Muggon), plot locations were pre-determined at approximately 500 m intervals along selected, accessible transects, partitioned across each of the three land systems to capture biomass variability. At Meka and Yoweragabbie, plot sites avoided infrastructure, 4 km piospheres around water points and integrated with station management where possible. As an example, at Meka, plots were partitioned between proposed grazing and non-grazing trial areas. At Yoweragabbie plots were allocated to each of the two chosen land systems according to their extent on the property. For both Meka and Yoweragabbie, the assigned number of plots for each grazing trial area, land system, etc. were distributed using a random coordinate generator within ArcGIS (GIS mapping software for stratified simple random sampling, De Gruijter et al. 2005). Within each land system are multiple land units. These are more detailed subdivisions of the landscape, characterised by landform with regolith terms such as hard pan, sand sheet and drainage tract. Accordingly, vegetation assemblages vary considerably among land units within a land system. Although each land unit is well described (landform, soil, and vegetation) and the proportion of each land unit within a land system is recorded in the Rangeland Survey Technical Bulletins published by the Department of Agriculture, Western Australia (e.g. Curry et al. 1994, see also Table 2 above), land-unit maps are not published. This means that it is very difficult to locate survey plots proportionally across land units. In the surveys reported here, for each plot, the applicable land unit was identified in the field and recorded. Overall, fortuitously, the plots within each of the five surveyed land systems were distributed approximately in proportion to their areal extent. (For more information on the land systems and land units chosen for the survey, please refer to Curry et al. 1994. In that publication, see pp. 78–79 for specific land units in Boulder land system, pp. 126–128 for land units in Holmwood land system, pp. 204–207 for land units in Sherwood land system, pp. 242–243 for land units in Woodline land system and pp. 262–264 for land-unit information on Yanganoo land system.) In the field, plot locations were shifted only if (a) access by 4WD vehicle was unreasonably difficult, (b) the location was clearly in the adjacent land system as a result of mapped land-system boundary error, or (c) unmapped infrastructure or disturbed vegetation (e.g. new powerline easement) was close to the proposed survey site.
In the field, 25 m × 25 m plots were laid out orientated north–south. Plot size was designed to sample examples of most tree and shrub species within the surrounding landscape in that land unit, while being logistically appropriate. The plot perimeter was conveniently 100 m long, facilitating an assessment of ground-cover types (trees, shrubs, litter, standing dead material) and percentages of cover and bare soil by using the line-intercept method (Canfield 1941). The total length of each cover type was summed. Because the total length of the plot boundary was 100 m, the total length of each cover type conveniently converted to % cover.
Plot litter was collected from six 1 m × 1 m quadrats. Two quadrats were chosen to represent light, medium and heavy litter loads. The litter from each quadrat was weighed separately and bagged. Later, the litter mean dry weights of the light, medium and heavy litter categories would be multiplied by their percentage plot cover (assessed by the boundary survey) and the results summed to arrive at an estimate of total plot litter. This approach is consistent with that of McKenzie et al. (2000).
Grasses made up an insignificant and highly variable portion of plot biomass. Many plots contained no grasses. Because grass productivity and size in these rangelands are highly dependent on seasonal conditions, the grass component was ignored.
All other perennial plant species within each plot (trees, shrubs, and standing-dead material) were identified using Mitchell and Wilcox (1994) and listed using nomenclature according to Turland et al. (2018). The plants of each species were allocated a size category (small, medium, large) by visual appraisal on the basis of knowledge of the species’ growth potential. The numbers of plants of each species in each size category were recorded. Plots were photographed and GPS located.
Subsets of plants were chosen for destructive sampling and their morphological measurements (height, canopy and basal stem diameter) were recorded. Plant canopy was recorded at its maximum canopy width (W1) × orthogonal width (W2), as used by Holm et al. (1984), who reported use of maximum canopy width and its orthogonal width as repeatable metrics for tree canopy. Here, we modelled each tree as an ellipse and calculated canopy area as:
On trees and larger shrubs, crown break (distance from the ground to the lowest leaves) and diameter of stems at 1.3 m above ground (diameter at breast height, DBH) were initially recorded. However, because of the high variability in growth forms, even within species, these last two measurements were not found to be useful and were discontinued during the first survey (Muggon).
Each survey aimed to destructively sample up 20 individuals of tree and shrub species; however, this was not always achieved. Sampling aimed to collect a range of plant sizes of each sampled species. Numbers of plants destructively sampled on each plot varied. No more than three individual trees or shrubs of any species were destructively sampled from any one plot. The actual number sampled per plot depended on the number of each species present. We left at least two plants of each species to foster plot rehabilitation.
Above-ground parts of plants were destructively sampled by cutting as close to ground level as possible. Samples were divided into sections of crown (branches of <2.0 cm in diameter), live stem and standing-dead material. Smaller plants were not subdivided in this way but were included in the crown category only. The whole of the crown, stem and standing dead material of each destructively sampled plant was weighed (field fresh weight). Smaller plants were weighed in toto and bagged in pre-weighed calico bags. The material from larger plants was subsampled, aiming for samples of 2 kg. At Yoweragabbie, whole standing dead plants were sampled, as opposed to dead components of living plants or fallen dead material that became part of the litter layer. Standing dead trees may contribute significantly to plot biomass and carbon content in mulga rangeland environments (McKenzie et al. 2000; Sinclair 2004). These were measured in the same way as living plants. Biomass and carbon (BC) data and allometrics of standing dead trees and shrubs from the Yoweragabbie survey were used in the calculation of BC in standing dead trees and shrubs recorded on the Muggon and Meka plots in the re-surveys of July 2014.
Laboratory preparation and analysis of biomass samples
Plant samples were analysed by the ChemCentre, Perth. Following milling, sample moisture content was measured (by percentage loss of weight after oven drying to a constant weight at 70°C) to calculate dry biomass. Organic carbon in the dry biomass was measured by weight loss on ignition.
Data analysis
Data for destructively sampled plants were calculated from the field and laboratory results. These derived data included canopy, stem, dead and total AGB and carbon per plant. Other data included values for plant height, plant canopy area and basal stem diameter. For multi-stemmed individuals, one equivalent stem diameter, De, was calculated as the quadratic mean of diameters, as follows:
Following laboratory analysis, the mean biomass of litter from each of the three categories [light (L), medium (M) and heavy (H)] was multiplied by the plot area and divided by the percentage of the boundary survey each litter type covered to estimate plot litter biomass for each category. The categories were summed to give total plot litter weight (kg plot−1).
Similar calculation strings were used to calculate plot litter carbon.
An initial analysis compared AGB with carbon content for all trees and all shrubs in each survey. Having found strong linear correlations between AGB and carbon content, further analyses focussed on finding relationships between AGB and plant morphological metrics to ascertain relationships, aggregated for individual stations and individual land systems.
All plants including the standing dead trees and shrubs were included in calculating the plot biomass and carbon totals (whole of landscape). Only live plants were included in the derivation of relationships between plant metrics and biomass.
Plant size classes of destructively sampled plants within species (large, medium, small) were calculated on the basis of individual plant biomass. Plant biomass records of individuals within each species were sorted from largest to smallest. The standard deviation of species biomass was calculated, and the plant samples were divided into three categories by using one standard deviation as the boundary between small and medium plants, and two standard deviations as the boundary between medium and large plants. The mean values of the AGB and carbon content for plants in each size category were calculated species by species. AGB and carbon for standing-dead material of different sizes was calculated in a similar way. This was in preparation for calculating the total AGB and carbon content for each plot (whole of landscape).
To estimate AGB and carbon at plot level, all species present in the field were listed along with the number of individuals within each size class, including standing dead individuals. These were multiplied by the mean AGB and carbon for their species and size category, and those results were summed to provide the total AGB and carbon for each species. Species totals were summed to provide AGB and carbon totals for the whole plot. The procedure was repeated for each plot.
For each survey, tables were produced listing AGB and carbon values for tree, shrub, standing-dead, and litter totals for all plots and overall plot totals for AGB and carbon in kg plot−1 and t ha−1. Using these tables for AGB and carbon contents, the proportions of plot AGB and Carbon contributed by different components were calculated.
Results
Plant level
Summary results for trees and shrubs across all surveys are provided at Table 3.
Tree survey | Land systems | Number of trees | Number of species | Tree biomass mean (kg) | Tree carbon mean (kg) | Carbon % of biomass | Range % of biomass | s.d. | |
---|---|---|---|---|---|---|---|---|---|
All | 379 | 45.96 | 21.90 | 47.3 | 43.8–51.7 | 1.2 | |||
Meka | Y | 115 | 6 | 52.94 | 25.02 | 46.9 | 44.7–49.2 | 0.8 | |
Muggon | B, H, S | 177 | 17 | 28.62 | 13.53 | 47.1 | 43.8–51.7 | 1.2 | |
Yoweragabbie | S, W | 87 | 5 | 72.00 | 34.79 | 48.3 | 45.4–50.8 | 1.0 |
Shrub survey | Land systems | Number of shrubs | Number of species | Shrub biomass mean (kg) | Shrub carbon mean (kg) | Carbon % of biomass | Range % of biomass | s.d. | |
---|---|---|---|---|---|---|---|---|---|
All | 544 | 1.73 | 0.82 | 46.1 | 23.4–52.0 | 2.8 | |||
Meka | Y | 146 | 10 | 2.10 | 1.00 | 46.1 | 29.7–48.8 | 1.8 | |
Muggon | B, H, S | 329 | 24 | 1.24 | 0.58 | 45.7 | 23.4–52.0 | 3.3 | |
Yoweragabbie | S, W | 69 | 4 | 3.28 | 1.58 | 47.8 | 45.5–49.0 | 0.6 |
Land systems: B, Boulder; H, Holmwood; S, Sherwood; W, Woodline; Y, Yanganoo.
Combining the results from the various tree species and then all shrub species supplied ample numbers of individual trees and shrubs to achieve meaningful results from all three surveys, as recommended by Paul et al. (2016).
The close agreement between AGB and carbon quantity and carbon percentage of biomass shows that it is possible to use biomass as a surrogate to estimate carbon values for trees and, separately, for shrubs.
Relationships between plant biomass and measured parameters were examined for trees and shrubs. The strongest relationships found were linear relationships between biomass and plant canopy area (Figs 3, 4). In forestry allometrics, transformations of stem diameter and height are most often used as predictor variables to model (transformed) AGB; log-transforms are commonly used assuming exponential relationships.
Relationships between tree canopy area, tree height and basal stem diameter and tree biomass (tree species combined) for each survey (x axis = canopy area (m2), height (m) or basal diameter (cm); y axis = tree biomass (kg)), Changes in N in Yoweragabbie are due to one missing basal diameter. Land system(s) LS(s): Boulder (B), H = Holmwood (H), S = Sherwood (S), W = Woodline (W), and Y = Yanganoo (Y).
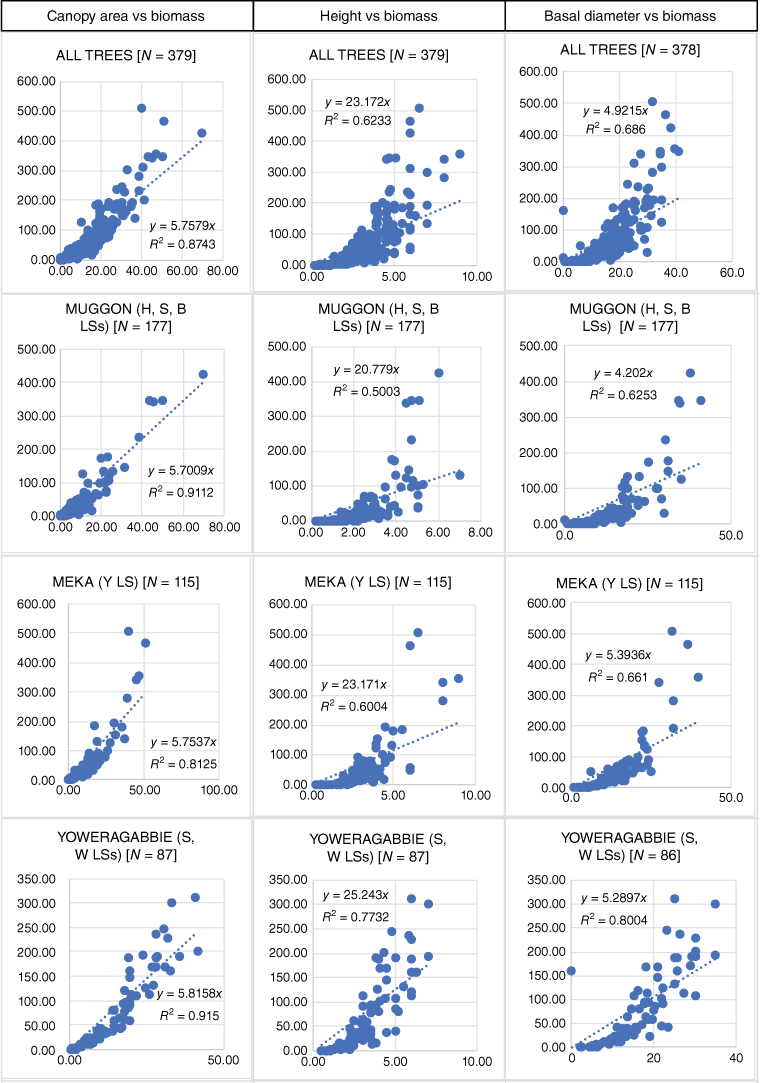
Relationships between shrub canopy area, shrub height and basal stem diameter and shrub biomass (shrub species combined) for each survey (x axis = canopy area (m2), height (m) or basal diameter (cm); y axis = tree biomass (kg)). Changes in N in Meka owing to one missing basal diameter. Land system(s) LS(s): Boulder (B), Holmwood (H), Sherwood (S), Woodline (W), and Yanganoo (Y).
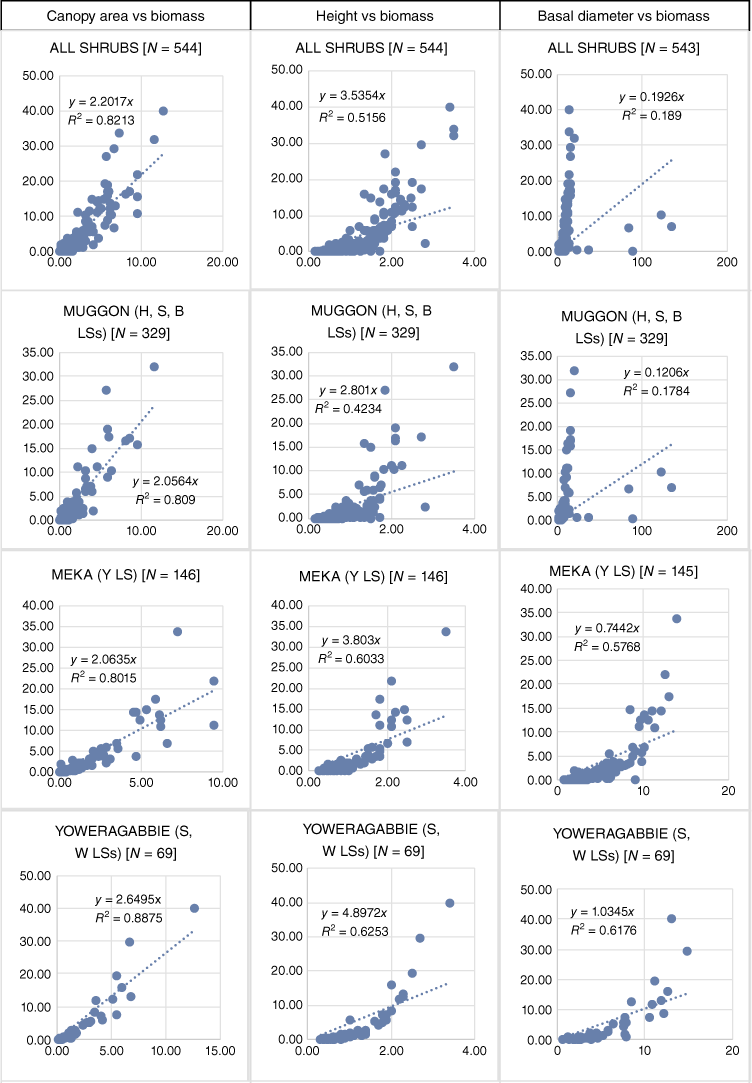
Fig. 3 shows graphically the relationships between tree canopy area and AGB for all 379 trees and for each survey area. Trend lines have been forced through the origin. Relationships between tree height and AGB and basal diameter and AGB are included for comparison. Canopy area and tree AGB show strong relationships (r2 values 0.8–0.9), which appear acceptably linear, noting the expected truncation of errors at low values. Residual plots show errors consistent with linear models for canopy-area values above 12.
For trees, the relationship of AGB with basal diameter appears exponential Fig. 3, and log transformations were applied for further analysis. For trees, relationships with height show no clear pattern; for shrubs, both diameter and height show no clear pattern (Fig. 4).
For the trees, log-transformed AGB and basal-diameter plots showed strong linear patterns, consistent with the exponential model. In the transformed space, r2 values were similar to those noted for untransformed canopy area (above). Because canopy area is measurable from remote-sensing, and stem diameter is not, we have focussed on canopy area as the most prospective predictor of AGB in the subsequent sections of this paper.
Fig. 4 shows a similar series of relationships between shrub canopy area and shrub AGB. Again, the metric of the shrub canopy area has strong and acceptably linear relationships with AGB.
The equations in the form y = A × x shown in Figs 3 and 4 are quite consistent across the land systems that were surveyed. For trees, the constant (A) varied between only 5.70 and 5.82, and for the shrubs the range was 2.06 and 2.65. This would suggest a general relationship across the trees and across the shrubs in this mulga-dominated area, particularly when recording large plant populations.
Plot level
Data for means of total plot AGB, plot tree AGB, plot shrub AGB, plot standing dead AGB, plot litter AGB and tree AGB as a percentage of total plot AGB across the land systems in all surveys are shown in Table 4. Tree AGB was the largest component of Plot AGB from plots aggregated to LS level.
Land system | Boulder | Holmwood | Sherwood | Woodline | Yanganoo | |
---|---|---|---|---|---|---|
Number of plots | 33 | 27 | 24 | 26 | 30 | |
Total plot biomass (P < 0.01) | 12.4a (11.2) | 17.9a (14.5) | 30.3a,b (28.7) | 54.5b (32.3) | 35.6a,b (21.1) | |
Tree plot biomass (P < 0.01) | 8.0a (9.3) | 11.5a (12.0) | 24.6a,b (28.0) | 47.5b (32.2) | 29.3a,b (19.2) | |
Shrub plot biomass (NS) | 1.7 (2.0) | 4.7 (4.9) | 2.6 (2.4) | 1.4 (1.6) | 2.8 (3.1) | |
Standing dead plot biomass (P < 0.01) | 1.7a (2.2) | 0.7a (0.7) | 2.4a (3.7) | 4.8b (4.5) | 2.4a,b (2.0) | |
Litter plot biomass (NS) | 1.1 (1.5) | 1.0 (1.1) | 0.7 (0.6) | 0.6 (0.6) | 1.1 (1.0) | |
Tree AGB as % of total plot AGB (P < 0.01) | 52.0a (29.7) | 45.7a (32.6) | 66.5a,b (26.6) | 83.8b (14.2) | 78.8a,b (16.6) |
Standard deviations in parentheses. Superscripts a and b indicate statistical differences at the p < 0.01 level.
Land-system level
There were clear differences in the productivity of the different land systems in these surveys (Table 4). Woodline land system, as surveyed, was the most productive, with the heaviest total AGB, tree biomass and total carbon. Sherwood and Yanganoo were measured as lower than Woodline, but similar to each other. Boulder and Holmwood were the least productive. This is reflected in the mean AGB of trees on the different land systems (Table 4). A small number of plots were located on treeless chenopod shrublands. All plots, including those that are treeless, were included in this assessment, to reflect a future broad-scale modelling or remote-sensing survey approach across large areas that will include treeless areas.
Discussion
The purpose of the surveys reported here was to assess the entire stocks of AGB and sequestered organic carbon in the trees, shrubs, ground litter and standing dead trees within a total of 140 plots, each 25 m × 25 m. This fits with a future ‘whole of landscape’ approach to carbon assessment. The collected data then allowed the testing of the concept that selected plant metrics could be used to estimate and monitor rangeland carbon stocks that might be used for approaches that both suit a whole of landscape or a Kyoto Protocol approach to carbon assessment (grasses were measured where encountered, but were ignored in the analysis owing to their opportunistic, ephemeral nature in this environment).
In setting out this work, we found few points of reference relating to the Murchison Biogeographical Region. A plot size of 25 m × 25 m seemed a good compromise between scale and work involved to assess total plot AGB and carbon. In addition, the 100 m long plot boundary suited assessment of percentages of different forms of plot [soil] cover. Destructive sampling was another compromise between data collection, work involved and site damage. It was not until after our survey that Paul et al. (2018) and Roxburgh et al. (2015) published their recommendations for keeping statistical errors to an acceptable level. Their analyses led to a recommendation to destructively sample 50 trees of each species, preferably more. Our target was 20 trees of individual species, and for many species, we fell short of that. In our survey areas, there was an insufficient number of trees of some species available. However, Paul et al. (2016) found that in developing allometrics, it was acceptable to combine metric data from different tree species within the same functional type. The total number of Acacia trees and trees of other species sampled in each of our surveys, when combined, more than met the 50-tree sample requirement. Roxburgh et al. (2015) suggested several tree individuals (as low as 17, but rising to 95 for some species) are required to achieve strong statistical allometric results. However, this related to algorithms based on stem diameter only.
The first aim was to confirm the relationship between biomass and carbon content. Overall, this held remarkably constant for both trees (mean of 47.3% carbon across 379 trees) and shrubs (mean of 46.1% carbon across 544 shrubs). Hence, plant biomass could be used as a reliable surrogate for carbon. Having confirmed that, the second aim was to find plant metrics that could predict biomass.
Tree and shrub metrics included plant height, crown break, basal stem diameter, stem diameter at 1.3 m above ground, and canopy measurements. Crown break was abandoned early in the first survey as being too highly variable to be of any use in the tree species we were measuring. Although AGB and carbon stocks in plantation timber have long been estimated from tree height and basal diameter or tree diameter at 1.3 m above the ground level, and although Witt et al. (2009) used these measurements in estimating tree biomass in mulga in Western Queensland, this was found not to be the case in stands of native trees in the areas we surveyed. Measurements incorporating the size of the canopy provided better results (Figs 3, 4).
This concurs with the findings of Adams et al. (2001) that biomass relates well to canopy measurements in the Pilbara region. Further agreement comes from Grierson et al. (2000) who found that for non-forest vegetation (<10% cover either for trees that have potential to reach 2–5 m, or any level of cover for plants not able to reach 2 m), the most useful statistical relationships are between the dimensions of crown, with or without leaves, and total biomass.
Airborne remote-sensing data from open woodland areas can now be automatically processed to measure tree heights and to define spatial objects such as tree crowns, from which dimension or area data can be obtained (e.g. Nguyen et al. 2022; and website: www.arborcarbon.com.au). These are operational technologies that allow rapid and efficient measurement of the canopy areas of all ‘tree objects’ over large areas. The results of our study suggest that linear relationships hold between canopy area and AGB at the tree level. If similar linear relationships exist for the remote-sensing data, then the error of an area’s AGB estimated from large numbers of trees will be very small. The airborne remote-sensing technologies further provide a practical means for consistent repeated measurement to enable monitoring of the tree canopy and dimensions (Fisher et al. 2016; Tu et al. 2019), and, hence, to quantify and, if desired, locate changes in tree AGB. It would be necessary in new areas to calibrate remotely derived measurements with tree AGB or carbon. For the mulga woodland area studied here, cross-calibration of remote and ground-based measurements of canopy area would be straightforward, and not require destructive sampling.
Tree AGB is the major component of total AGB in each land system (Table 4). Tree AGB is likely to respond to land-management changes such as fire control and methods to increase soil moisture intake and retention, as mentioned earlier, and tree-canopy changes are amenable to monitoring by ground survey or by remote-sensing. This all makes tree canopy an important part of future biomass and carbon monitoring.
The metrics and relationships in this paper have provided information relevant to development of methods for assessing and monitoring either tree AGB or carbon from tree canopy measurements. Since tree above-ground carbon is 47% of AGB, CO2 equivalents (carbon × 3.67) and Australian carbon-credit units (ACCUs) could be estimated from tree-canopy measurements in this and similar environments and similar land systems.
Land systems vary in their productivity, as can be seen from Table 4. The lowest mean tree biomass was found on the Boulder land system at 8.0 t ha−1, while Woodline land system was six times more productive in trees at 47 t ha−1. Assessing the productivity of land systems could become a guide to locating future carbon projects.
A reference point for comparison of these figures is the survey in the Pilbara region by Adams et al. (2001). There, the mean recorded values for biomass in mulga woodland was 18.8 t ha−1. The larger values of biomass (47 t ha−1) and carbon (47 t × 0.47 = 22 t C ha−1) found on Yoweragabbie Station are of particular interest because they agree with the largest findings by Adams et al. (2001) in the Pilbara and approach the Intergovernmental Panel on Climate Change-suggested default minimum value of 26 t C ha−1 for carbon-farming initiatives (Adams et al. 2001). Clearly, future carbon-farming initiatives in rangelands should carefully assess the productivity and rangeland condition of the land systems under consideration.
Permitting the calculation of below-ground biomass and carbon would add significantly to assessed biomass and carbon stocks. Paul et al. (2011) quoted a ratio of below-ground:above-ground biomass of 1:1.4. For example, with that inclusion, the mean tree above-ground values of 47 t ha−1 of biomass and 22 t C ha−1 for Yoweragabbie would become 80 t ha−1 of biomass and 37.5 t C ha−1 for mean combined above- and below-ground stocks.
Conclusions
One of the aims of these surveys has been to contribute to the development of protocols for efficiently estimating carbon stocks in the mulga rangelands. Clearly, future carbon-farming initiatives need robust, repeatable, economical methods to assess initial carbon stocks and monitor their changes. The data collected in these surveys showed that for the tree and shrub components, an approach based on remote-sensing is possible and practical with a minimum of time spent in ground-truthing. Of the plots we surveyed over the five land systems, trees made up 50–80% of the AGB (Table 4), and, therefore, probably 50–80% of the above-ground carbon stocks.
This study has identified plant morphological metrics suited to assessments of sequestered carbon stocks in mulga-dominated rangelands. These surveys have provided a range of statistical relationships between AGB and carbon and plant morphological metrics for individual plant species, standing dead material and litter on the studied land systems and pastoral stations.
It would be essential to undertake further verification studies in which rangeland AGB and carbon are first assessed using remote-sensing techniques, followed by ground survey to assess how close the remotely sensed values are to the values derived from ground-truthing. This would confirm the reliability of assessment by remote-sensing. Integration of outcomes of this work into the FULLCAM model (Department of Industry, Science, Energy and Resources (DISER) 2020) adopted by the Clean Energy Regulator for estimating above-ground carbon are recommended to improve accuracy of broad-scale carbon modelling.
These surveys have been performed in open mulga woodland. Even though this is a major vegetation type in the WA rangelands and other parts of Australia, other vegetation types should be assessed in similar ways. These should include those vegetation types that are thought to have the potential to sequester 26 t C ha−1 or more. The open eucalyptus woodland of the Western Australian Goldfields, and dense acacia woodlands of the Pilbara and Kimberley regions would be candidates for consideration.
Data availability
The data that support this study will be shared upon reasonable request to the corresponding author.
Declaration of funding
The work described in this paper was supported by the Western Australian State Government through the Royalties for Regions program. The surveys were commissioned and administered by Rangelands NRM (WA) as part of its Carbon Farming Awareness Program.
Acknowledgements
The authors acknowledge and thank the many people involved in these surveys. Jane Bradley as Rangelands Programme Manager of Rangelands NRM (WA) strongly supported the work. The property owners and managers Rob and Trish Grinham, Neil Grinham and Katherine Peat, and Jorgen Jensen provided access to their properties, support, local knowledge, accommodation, and hospitality. The field-survey personnel, including Frank and Freddie Ashburton, Jess Bibby, Indy Chambers, Kaz Collins (dec.), Mike Cully, Luce Dulau, Chris Graham, Victor Greenfield, Cole Hendrigan, Adele Kendle, Anna Lewis, Ostiane Massiani, Dave Nicholson, Tim Pope, Katie Shaw-Brown, Philip Tinker, Tim Wiley, and George Woolston, worked willingly often under difficult conditions, enabling the fieldwork to be completed expediently. David Galloway assisted in sourcing some of the field staff. The managers and staff of the ChemCentre, Perth, particularly Peter McCafferty, Katrina Walton, Jeremy Brown and Barry Price, provided strong support and expert, professional analytical work over several years. We are grateful to rangeland ecologists Don Burnside and Phil Thomas for their useful comments on an earlier draft of this paper. We thank Paul Novelly, Ian Watson and anonymous reviewers for their valuable and constructive comments and suggestions on earlier versions of the paper, and we are indebted to Jeremy Wallace for his guidance over statistical aspects of the later iterations.
References
Baumber A, Waters C, Cross R, Metternicht G, Simpson M (2020) Carbon farming for resilient rangelands: people, paddocks and policy. The Rangeland Journal 42, 293-307.
| Crossref | Google Scholar |
Bureau of Meteorology, Government of Australia (2023) BOM – Summary statistics MOUNT MAGNET. Available at http://www.bom.gov.au/climate/averages/tables/cw_007057.shtml [last updated 30 November 2010]
Canfield RH (1941) Application of the line interception method in sampling range vegetation. Journal of Forestry 39(4),.
| Google Scholar | PubMed |
Carbon Market Institute (2021) Blueprint for holistic approach to carbon farming. (Active Land Management & Agricultural Production (AL-MAP) Method). Available at https://carbonmarketinstitute.org/app/uploads/2021/08/AL-MAP-Method-Blueprint_final.pdf
Carbon Market Institute (2023) May 2023 update. Available at https://mailchi.mp/carbonmarketinstitute/critical-reforms-headline-busy-start-to-320734?e=eef365588a
Christian CS, Stewart GA (1946) General Report on Survey of Katherine-Darwin Region, Compiled in collaboration with L. C. Noakes and S. T. Blake. CSIRO Land Research Series No. 1, 1946. Available at https://hdl.handle.net/10070/674247
Department of Climate Change, Energy, the Environment and Water (2023) Independent Review of Australian Carbon Credit Units. Available at https://www.dcceew.gov.au/climate-change/emissions-reduction/independent-review-accus
Department of Climate Change, Energy, the Environment and Water (n.d.) Murchison Bioregion. Available at https://www.dcceew.gov.au/sites/default/files/env/resources/a8015c25-4aa2-4833-ad9c-e98d09e2ab52/files/bioregion-murchison.pdf
Department of Industry, Science, Energy and Resources (DISER) (2020) FullCAM Guidelines: requirements for using the Full Carbon Accounting Model (FullCAM) in the Emissions Reduction Fund (ERF) methodology determination Carbon Credits (Carbon Farming Initiative) (Human Induced Regeneration of a Permanent Even Aged Native Forest—1.1) Methodology Determination 2013, Version 3.0, 2020. Available at https://cer.gov.au/2020-fullcam-now-available
Fisher A, Day M, Gill T, Roff A, Danaher T, Flood N (2016) Large-area, high-resolution tree cover mapping with multi-temporal SPOT5 imagery, New South Wales, Australia. Remote Sensing 8, 515.
| Crossref | Google Scholar |
Holm AM, Curry PJ, Wallace JF (1984) Observer differences in transect counts, cover estimates and plant size measurements on range monitoring sites in an arid shrubland. The Rangeland Journal 6, 98-102.
| Crossref | Google Scholar |
Nguyen TT, Eslick H, Barber P, Harper R, Dell B (2022) Cooling effects of urban vegetation: the role of golf courses. Remote Sensing 14, 4351.
| Crossref | Google Scholar |
Nielsen UN, Stafford-Smith M, Metternicht GI, Ash A, Baumber A, Boer MM, Booth S, Burnside D, Churchill AC, El Hassan M, Friedel MH, Godde CM, Kelly D, Kelly M, Leys JF, McDonald SE, Maru YT, Phelps DG, Ridges M, Simpson G, Traill B, Walker B, Waters CM, Whyte AW (2020) Challenges, solutions and research priorities for sustainable rangelands. The Rangeland Journal 42, 359-373.
| Crossref | Google Scholar |
Paul KI, Roxburgh SH, Chave J, England JR, Zerihun A, Specht A, Lewis T, Bennett LT, Baker TG, Adams MA, Huxtable D, Montagu KD, Falster DS, Feller M, Sochacki S, Ritson P, Bastin G, Bartle J, Wildy D, Hobbs T, Larmour J, Waterworth R, Stewart HTL, Jonson J, Forrester DI, Applegate G, Mendham D, Bradford M, O’Grady A, Green D, Sudmeyer R, Rance SJ, Turner J, Barton C, Wenk EH, Grove T, Attiwill PM, Pinkard E, Butler D, Brooksbank K, Spencer B, Snowdon P, O’Brien N, Battaglia M, Cameron DM, Hamilton S, McAuthur G, Sinclair J (2016) Testing the generality of above-ground biomass allometry across plant functional types at the continent scale. Global Change Biology 22, 2106-2124.
| Crossref | Google Scholar | PubMed |
Paul KI, Radtke PJ, Roxburgh SH, Larmour J, Waterworth R, Butler D, Brooksbank K, Ximenes F (2018) Validation of allometric biomass models: how to have confidence in the application of existing models. Forest Ecology and Management 412, 70-79.
| Crossref | Google Scholar |
Porfirio LL, Antille DL, Watson I, Abbott BN, Bowman DMJS, Briggs PR, Canadell JG, Churchill AC, Donohue RJ, Guerschman JP, Haverd V, Hill MJ, Knauer J, Murphy BP, Paget M, Prior LD, Roxburgh SH, Williamson GJ (2020) Productivity and biomass of Australia’s rangelands: towards a national database. The Proceedings of the Royal Society of Queensland 128, 75-98.
| Crossref | Google Scholar |
Roxburgh SH, Paul KI, Clifford D, England JR, Raison RJ (2015) Guidelines for constructing allometric models for the prediction of woody biomass: how many individuals to harvest? Ecosphere 6(3), 38.
| Crossref | Google Scholar |
Russell PJ (2007) Assessing long-term change in rangeland ecological health using the Western Australian rangeland monitoring system. PhD Thesis, Curtin University, Perth, WA, Australia. Available at https://espace.curtin.edu.au/handle/20.500.11937/1598
Russell-Smith J, Yates CP, Edwards AC, Whitehead PJ, Murphy BP, Lawes MJ (2015) Deriving multiple benefits from carbon market-based savanna fire management: an Australian example. PLoS One 10(12), e0143426.
| Crossref | Google Scholar | PubMed |
Shiono K, Suganuma H, Abe Y, Tanouchi H, Utsugi H, Saito M, Takahashi N, Kojima T, Yamada K (2006) Biomass growth estimation of an afforestation site and natural forests in an arid land of Western Australia. Journal of Arid Land Studies 15(4), 251-254.
| Google Scholar |
Sinclair R (2004) Persistence of dead trees and fallen timber in the arid zone: 76 years of data from the T.G.B. Osborn Vegetation Reserve, Koonamore, South Australia. The Rangeland Journal 26, 111-122.
| Crossref | Google Scholar |
Suganuma H, Abe Y, Utsugi H, Tanouchi H, Kojima T (2006) Shrub-land biomass estimation method for application to remote sensing. Journal of Arid Land Studies 15(4), 259-262.
| Google Scholar |
Taniguchi M, Abe Y, Yamada K, Kojima T, Williams A (1998) Possibility of large scale afforestation in arid lands as a measure against increases in CO2 concentration. Journal of Arid Land Studies 7S, 249-252.
| Google Scholar |
Tu Y-H, Johansen K, Phinn S, Robson A (2019) Measuring canopy structure and condition using multi-spectral UAS imagery in a horticultural environment. Remote Sensing 11, 269.
| Crossref | Google Scholar |
Turland NJ, Wiersema JH, Barrie FR, Greuter W, Hawksworth DL, Herendeen PS, Knapp S, Kusber W-H, Li D-Z, Marhold K, May TW, McNeill J, Monro AM, Prado J, Price MJ, Smith GF (Eds) (2018) ‘International Code of Nomenclature for algae, fungi, and plants (Shenzhen Code) adopted by the Nineteenth International Botanical Congress’. Shenzhen, China, July 2017. Regnum Vegetabile 159. (Koeltz Botanical Books: Glashütten, Germany) 10.12705/Code.2018
Watson IW, Novelly PE, Thomas PWE (2007a) Monitoring changes in pastoral rangelands – The Western Australian Rangeland Monitoring System (WARMS). The Rangeland Journal 29(2), 191-205.
| Crossref | Google Scholar |
Watson IW, Thomas PWE, Fletcher WJ (2007b) The first assessment, using a rangeland monitoring system, of change in shrub and tree populations across the arid shrublands of Western Australia. The Rangeland Journal 29, 25-37.
| Crossref | Google Scholar |
Yamada K, Kojima T, Abe Y, Williams A, Law J (1999) Carbon sequestration in an arid environment near Leonora, Western Australia. Journal of Arid Land Studies 9(2), 143-151.
| Google Scholar |