Perspectives and opinions from scientific leaders on the evolution of data-independent acquisition for quantitative proteomics and novel biological applications
Christie L. Hunter


A SCIEX, Redwood City, CA, USA.
B Buck Institute for Research on Aging, 8001 Redwood Boulevard, Novato, CA 94945, USA.
![]() Dr. Christie Hunter is the Chief Scientist, Application Development at SCIEX. Christie is focused on developing innovative MS workflows for the quantitative analysis of proteins and peptides, working in the SCIEX R&D department, and working collaboratively with researchers in the field. Over the years, she has developed workflows for MRM analysis of peptides, advanced data independent acquisition strategies, and most recently, ultra-high throughput quantification workflows for peptides/proteins using Acoustic Ejection Mass Spectrometry. Christie received her PhD in protein biochemistry from the University of British Columbia (Canada). |
![]() Dr. Joanna Bons is a postdoctoral fellow in the laboratory of Dr. Birgit Schilling at the Buck Institute for Research on Aging. After an engineer degree in Biotechnology, she joined the team of Dr. Christine Carapito at the BioOrganic Mass Spectrometry Laboratory in Strasbourg, France, where she specialized in quantitative mass spectrometry-based proteomics method development (SRM, PRM, DIA) for proteome quantification and characterization. She received her PhD in Analytical Chemistry in 2019, and then joined Dr. Birgit Schilling s laboratory. She focuses on developing and optimizing innovative DIA and targeted strategies for deciphering proteome and PTM remodeling in various collaborative projects, spanning neurodegenerative diseases, cancer, and metabolism dysfunction and diseases. |
![]() Dr. Birgit Schilling works at the Buck Institute for Research on Aging in the San Francisco Bay Area since 2000 as Professor and Director of the Mass Spectrometry Technology Center, specifically focusing on data-independent acquisition technologies and large-scale proteome quantification. Dr. Schilling received her PhD in Germany, and then moved to the University of California San Francisco (UCSF) as postdoctoral fellow. Dr. Schilling is interested in translational research and research that may aim towards therapeutic interventions to improve human aging or age-related diseases, specifically osteoarthritis and cancer. Dr. Schilling uses modern proteomic technologies to investigate mechanisms of aging, senescence and cancer, and using this knowledge to develop biomarkers and targets for interventions. |
Australian Journal of Chemistry 76(8) 379-398 https://doi.org/10.1071/CH23039
Submitted: 22 February 2023 Accepted: 22 May 2023 Published: 19 July 2023
© 2023 The Author(s) (or their employer(s)). Published by CSIRO Publishing. This is an open access article distributed under the Creative Commons Attribution-NonCommercial-NoDerivatives 4.0 International License (CC BY-NC-ND)
Abstract
The methodology of data-independent acquisition (DIA) within mass spectrometry (MS) was developed into a method of choice for quantitative proteomics, to capture the depth and dynamics of biological systems, and to perform large-scale protein quantification. DIA provides deep quantitative proteome coverage with high sensitivity, high quantitative accuracy, and excellent acquisition-to-acquisition reproducibility. DIA workflows benefited from the latest advancements in MS instrumentation, acquisition/isolation schemes, and computational algorithms, which have further improved data quality and sample throughput. This powerful DIA-MS scan type selects all precursor ions contained in pre-determined isolation windows, and systematically fragments all precursor ions from each window by tandem mass spectrometry, subsequently covering the entire precursor ion m/z range. Comprehensive proteolytic peptide identification and label-free quantification are achieved post-acquisition using spectral library-based or library-free approaches. To celebrate the > 10 years of success of this quantitative DIA workflow, we interviewed some of the scientific leaders who have provided crucial improvements to DIA, to the quantification accuracy and proteome depth achieved, and who have explored DIA applications across a wide range of biology. We discuss acquisition strategies that improve specificity using different isolation schemes, and that reduce complexity by combining DIA with sophisticated chromatography or ion mobility separation. Significant leaps forward were achieved by evolving data processing strategies, such as library-free processing, and machine learning to interrogate data more deeply. Finally, we highlight some of the diverse biological applications that use DIA-MS methods, including large-scale quantitative proteomics, post-translational modification studies, single-cell analysis, food science, forensics, and small molecule analysis.
Keywords: data-independent acquisition, food science, forensics, immunopeptidomics, ion mobility, machine learning, metabolomics, microflow chromatography, protein turnover, proteomics, quantification, reproducibility, single-cell proteomics.
Introduction
Liquid chromatography coupled to tandem mass spectrometry (LC–MS/MS) has evolved into a powerful tool to profile and quantify thousands of proteins, including post-translationally modified proteins and different proteoforms.[1] Indeed, the comprehensive, accurate, and reproducible measurements achieved by LC–MS/MS proteomics technology enable scientists to gain deep insights into biological systems. The three predominant MS acquisition workflows used today – data-dependent acquisition (DDA), data-independent acquisition (DIA), and targeted proteomics – are illustrated in Fig. 1. Workflows utilizing DDA have traditionally been applied for large-scale discovery-based proteomics that do not require any hypotheses or prior knowledge. DDA is considered a global discovery workflow used to identify proteins and proteoforms, and is conceptionally data-dependent as the mass spectrometer typically selects the top N most abundant precursor ions from an MS survey scan to be sequentially subjected to MS/MS acquisition within each scan cycle. Depending on scan speed, a fixed number of precursor ions can be sampled for MS/MS during each scan cycle leading to stochasticity, which can introduce MS/MS missing values in complex samples. The stochastic nature of DDA acquisitions reduces the acquisition-to-acquisition reproducibility; however, label-free DDA discovery experiments can still be employed for parallel analyte quantification, typically by extracting ion chromatograms at the MS1 level,[2,3] inferring precursor ions confidently identified from associated acquisitions (often referred to as MS1 quantification or label-free quantification, LFQ).
Principles of data-dependent acquisition, data-independent acquisition, and parallel reaction monitoring. The acquisition strategies of three mass spectrometry-based proteomic workflows, (a) data-dependent acquisition (DDA), (b) targeted parallel reaction monitoring (PRM), unscheduled (top) and scheduled (bottom), and (c) data-independent acquisition (DIA), are depicted. In DDA and PRM (also called MRMHR), single precursor ions are isolated within narrow quadrupole isolation windows (typically ~1 m/z), fragmented in the collision cell, and resulting fragment ions are analyzed in the second analyzer to collect MS/MS spectra. Thus, DDA generates MS/MS spectra for the top N selected precursor ions that are further used for identification, while PRM enables retrieval of highly quantitative information for analytes of interest. In DIA, all precursor ions contained in wider mass range windows are co-isolated and co-fragmented, and all resulting fragment ions are analyzed to collect convoluted MS/MS spectra. DIA combines the capabilities of both shotgun DDA and targeted PRM as MS/MS level information is collected over the entire mass range and chromatographic gradient, providing proteome-wide highly quantitative information.
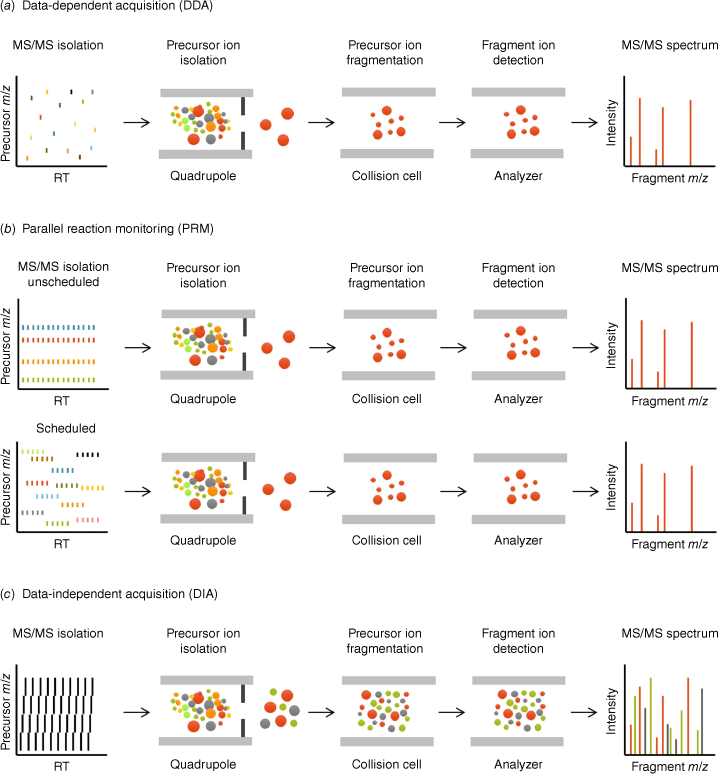
In contrast, targeted methods, including selected reaction monitoring (SRM)[4]/multiple reaction monitoring (MRM)[5,6] and parallel reaction monitoring (PRM),[7–9] are used for hypothesis-driven proteomics. The MS method is designed to target a list of selected peptides only, by sequentially selecting the precursor ions for MS/MS acquisition, often at a known (scheduled) retention time, for each scan cycle. These targeted methods can be developed on both low-resolution triple quadrupole MS systems (for SRM/MRM) or on high resolution/accurate mass systems (for PRM/MRMHR). MS2-generated fragment ion peak areas are finally used for quantification adding high selectivity to the targeted quantitative analysis with minimal missing values.[4,10]
The strengths of DDA workflows are the capability to provide a deep analysis of a sample in an unbiased manner (10 000s to 100 000s of peptides) and the ease of implementation. For targeted acquisition, highly accurate and sensitive quantification is achieved, which is very reproducible and robust across many samples; however, methods typically interrogate a more limited number of pre-selected peptides per acquisition (100s to 1000s of peptides).
The premise to combine the strengths of both strategies, DDA and targeted MS, has been fulfilled with the more recent emergence of data-independent acquisition (DIA), which has evolved into the third main workflow of MS proteomics. In 2012, Gillet et al. introduced label-free SWATH MS quantification,[11] which is also referred to as DIA-MS, and gained high interest in the field. With DIA-MS, researchers demonstrated the ability to quantify large numbers of proteins with quantification at the MS2 fragment ion level (high selectivity and specificity) with fewer missing values to achieve the quantitative reproducibility and specificity of targeted MS. Over the last decade, major advancements were further achieved due to instrumentation improvements, and many technical and computational innovations (Fig. 2). Many diverse studies have been performed analyzing a broad range of biological systems and samples.[12] To celebrate the power of DIA-MS, we interviewed renowned leaders in the field to discuss the development and perspectives of DIA-MS and selected powerful applications.
Milestones for the evolution of data-independent acquisition. The emergence and evolution of data-independent acquisition (DIA) has relied on striking developments and improvements regarding instrumentation for acquisition and online separation, as well as computation for data processing, including the more recent implementation of artificial intelligence strategies. The impact of these crucial steps for DIA analysis is discussed in this review.
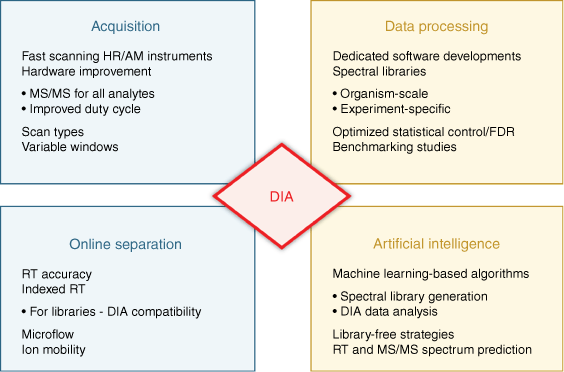
Why data-independent acquisition?
Generating high-quality, full scan MS/MS data to identify components in biological matrices is key in most applications performed by mass spectrometry. Analyte fragment ions generated during MS/MS fragmentation provide crucial information about the structural composition of a compound, and typically MS/MS spectra provide enough information to determine the identity of molecules. In addition, the selection of a set of specific, determinant, and quantifiable fragment ions for a specific molecule can provide more specific quantitative information as compared to using the precursor ion signal alone (as achieved using SRM/MRM analysis). However, obtaining comprehensive MS/MS sampling in an untargeted way can be a challenge as these components often are present in highly complex matrices and exist across a wide abundance range.
In the early 2000s, Venable et al.[13] and Silva et al.[14] investigated initial DIA workflows; however, instrument limitations were experienced due to relatively low scan speeds and low fragment ion resolution of the MS technology at the time. Kitata et al. described a comprehensive overview into the early development of DIA-MS.[15]
Fast scanning, high resolution quadrupole-time-of-flight (QqTOF) instruments (100 Hz acquisition speed) enabled comprehensive MS/MS sampling in a LC-compatible cycle time, which enabled a major workflow advancement with the introduction of SWATH MS,[11] a DIA-MS method where full-scan MS/MS spectra were systematically acquired for all precursor ions by using wide mass windows of 25 m/z that are stepped across the entire MS1 mass range. Dr. Ruedi Aebersold (Box 1) describes the work that was on-going at the time and that led to the to the development of SWATH MS/DIA workflow.
Box 1. Question and answer. |
Q. What were the key technologies that led to the ‘light-bulb moment’ for the SWATH MS concept? |
A. Around 2010 when the SWATH MS technique was developed, we were using two bottom-up proteomic workflows in our group. The first was DDA-MS which we used for discovery-type measurements, i.e. to identify as many proteins per sample as possible. The second was targeted MS by SRM which we used for the reproducible measurement of sets of roughly 10–100 predetermined peptides across large sample sets.[16] We used SRM for biomarker validation studies and for the analysis of differentially perturbed sample sets in systems biology studies. To facilitate the tedious manual analysis of SRM data Lukas Reiter and Oliver Rinner had created the software tool mProphet[17] for assigning probabilities to the identification of peptides by SRM by scoring the peak groups generated by the detected transitions. |
For quite a while we had sought a way to combine the proteome coverage provided by DDA-MS with the reproducibility and quantitative accuracy provided by SRM. We were well aware of the possibility to concurrently fragment multiple peptides – in fact this is also what is happening with SRM – but we were worried whether specific peptides could be confidently identified from the convoluted fragment ion spectra. Hannes Röst generated a simulator (SRMCollider[18]) which allowed us to ask whether a peptide could be confidently identified from MS/MS spectra if 10, 100, 1000 or all peptides in a sample were concurrently fragmented as a function of MS resolution, dwell time, and chromatographic peak capacity. The simulations clearly indicated that confident peptide identifications could be achieved at the resolution achieved by the state-of-the-art mass spectrometers at the time if the selected precursor ions were filtered by retention time and a moderately constrained mass range. |
At a visit to SCIEX in Toronto I learned about the performance characteristics of their new instrument – the TripleTOF 5600 system – and it became immediately clear that this was the instrument that had the potential to cover the whole peptide population of a complex sample if time/mass range windows were recursively sampled. The idea of SWATH MS was born during this collaboration. Very quickly, Stephen Tate and the team at SCIEX implemented the instrument control software to test the concept on our TripleTOF 5600 system. To extract and score the tens of thousands of peak groups for targeted peptide identification Hannes Röst created the software tool OpenSWATH[19] that built on elements of the mProphet software. Even the earliest results made us very confident that the method would become very powerful.[11] After some optimization of data acquisition and data analysis steps, we were excited about the performance of the method in terms of protein identification, quantification, and run to run reproducibility – and given the impressive recent advances we are even more excited now. |
![]() |
The DIA scan type was quickly adopted by laboratories on fast-scanning QqTOF platforms, and then rapidly evolved on to different high-resolution instrument platforms as a comprehensive, label-free, quantitative proteomics workflow.[15]
What is DIA?
The key goal of DIA-MS is to acquire MS/MS spectra for all analytes across the precursor ion m/z range, regardless of analyte abundance, generating a digitized MS and MS/MS map of a given sample. To achieve this, the acquisition of MS/MS cannot be dependent on initial detection of MS1 precursor ions, such as in DDA. The main factors that limit DDA workflows are that low level precursor ions are often not detected in the MS1 scans due to noise or interferences in a complex matrix, or the MS system may not be able to sample all individual precursor ions within a defined cycle time. With the DIA-MS workflow described by Gillet et al.,[11] acquisition was performed by selecting wide MS1 isolation windows using the mass isolating Q1 quadrupole (25 m/z in this first report) to co-isolate and subsequently unbiasedly co-fragment all precursor ions present in each m/z window. Subsequently, the isolation windows were stepped across the entire MS1 mass range of expected tryptic peptide precursor ions (Fig. 3). Full scan MS/MS spectra were systematically acquired for each quadrupole isolation window, and the MS/MS accumulation time multiplied by the number of required Q1 windows determined the cycle time of the acquisition method. Fast scanning mass spectrometers were able to yield reasonable cycle times (2–3 s at the time) even with multiple MS1 windows (initially 32 windows[11]), and it was possible to obtain enough points across the chromatographic peak (7–10 points) for each analyte to achieve accurate quantification. In this manner, MS/MS was acquired on all precursor ions including the low abundant, low signal intensity precursor ions that are often missed during DDA in complex mixtures.
Fundamentals of the DIA workflow. (a) In DIA, the isolation quadrupole steps through a defined mass range with wider isolation windows. Isolation window sizes are scaled according to expected precursor ion density, using smaller windows in the mass regions of highest precursor ion density and larger windows in less populated mass regions to increase specificity and decrease MS/MS convolution. (b) Co-isolated precursor ions are fragmented to collect convoluted full scan MS/MS spectra, as detailed in Fig. 1. From the DIA-MS/MS spectra acquired for each isolation window, extracted ion chromatograms (XICs) are obtained for target fragment ions to generate peak groups, which are further scored using dedicated algorithms for identity confirmation and quantification.
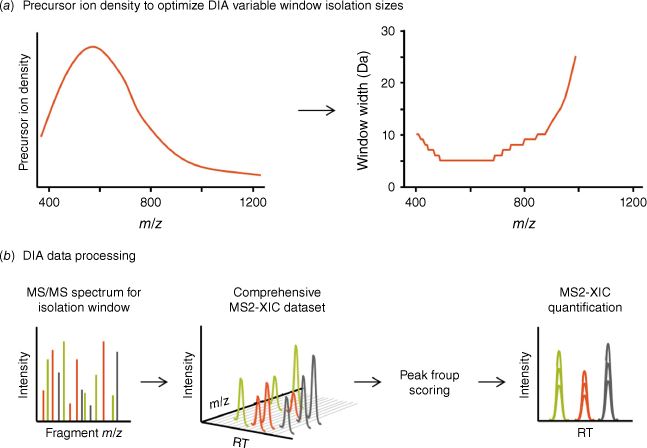
As the Q1 isolation window is wider, multiple precursor ions are fragmented in every MS/MS spectrum, challenging the interpretation of DIA-MS/MS spectra by traditional DDA search engine tools. Consequently, new data processing strategies were developed, which initially relied on targeted data extraction using experimentally generated spectral ion libraries, to identify and then quantify peptides from the MS/MS data. Spectral libraries generated from confidently identified peptides in DDA-MS/MS spectra[20,21] contained protein and peptide sequence information, retention time information (that could be normalized to indexed retention time peptides[22]) and peptide and fragment mass information. All of this information was then used to extract fragment ion chromatograms (XICs) for each peptide, at the expected retention time, creating overlapping XICs (Fig. 3b) or peptide peak groups. These peak groups were scored using sophisticated algorithms to confirm the identity of the peptides, then the area under the fragment ion peaks was used for quantification[11,19] in a similar manner to quantification from SRM/MRM data. As the MS/MS is acquired for all precursor ions in every sample acquisition, this led to a more consistent detection with far fewer missing values and more specific and accurate quantification of peptides acquisition-to-acquisition.
Workflow advances increase DIA proteome coverage
After the introduction of the DIA workflow for quantitative proteomics, researchers rapidly began to innovate upon this foundation. Following closely on the original work, two major steps forward occurred: (i) the creation of large spectral ion libraries[20,23] and (ii) the use of a greatly increased number of windows and variable-sized Q1 isolation windows.[24,25] Many research groups began to invest significant efforts into developing spectral libraries, typically by sub-fractionation of digested proteomes, analyzing them by DDA-MS, then identifying proteins/peptides using database search engines to assemble very large spectral ion libraries. Early examples were the generation of a Pan Human library,[23] which contained 139 449 proteotypic peptides and 10 316 proteins, covering 60% of the human proteome, and the Pan Yeast Library,[26] containing 66 007 unique modified peptides and 4596 unique protein isoforms. Additional comprehensive organism-scale spectral libraries have since been provided as public resources. For instance, the mouse MouseRefSWATH library[27] was built from various mouse organs and cell lines, and includes 167 138 proteotypic peptides and 10 597 proteins, covering 62% of the mouse proteome. Zhu et al.[28] published a newer DIA Pan Human Library generated from 16 human cancer tissue samples, and containing 242 476 peptides and 14 782 protein groups, that can support biomarker discovery and clinical studies. Importantly, while large-scale libraries tend to improve identification results, smaller sample-specific libraries might provide more accurate quantification.[29] Appropriate false discovery rate (FDR) control is crucial to obtain high quality and high confidence spectral libraries, and subsequently accurate quantification.
At the same time, the use of more and smaller m/z isolation windows was being explored to reduce MS/MS complexity and interferences, and improve confidence in peptide detection.[30] Use of variable-sized isolation windows where the window width is scaled inversely to the precursor ion density expected in that mass range (Fig. 3a) was helpful to improve specificity while maintaining the analysis of the full peptide m/z range. Typically, by using more, smaller windows with a LC-compatible cycle time, more peptides could be reliably quantified from a sample.[31] Another key innovation was the ability of mass spectrometers to collect full-scan high resolution MS/MS at fast acquisition rates (100 Hz acquisition speed), such that more isolation windows could be analyzed.
To investigate DIA-MS reproducibility and assay transferability, a large multi-laboratory quantitative proteomics study was implemented (see Box 2). The 11 international participating laboratories obtained highly similar results, confidently quantifying ~4000 human proteins by analyzing human cell lines using a 64-variable window DIA-MS protocol as described by Collins et al.[30]
Box 2. Question and answer. |
Q. A group of early adopting scientists from 11 international laboratories performed a cross laboratory study to assess the reproducibility and utility of DIA-MS. What were the key conclusions, highlights and gaps that were identified in this study? |
A. A large part of the motivation for this study came via work that the NCI-CPTAC consortium had published showing that reproducible and robust quantitative data could be produced across labs using the targeted proteomics approach selected reaction monitoring (SRM).[32] Conversely CPTAC among others had also shown that achieving such reproducibility by data-dependent acquisition (DDA) was much more challenging.[33] We felt that SWATH MS was achieving the level of data quality approaching that of SRM.[11] To assess this, we designed a study where benchmarking samples consisting of heavy isotope labelled peptides at varying concentrations in a complex cell lysate digest were distributed to 11 labs who were running SWATH MS.[30] The data were analyzed centrally, and we found that the results were very comparable across labs. This included both the linearity and dynamic range in the response curve of the spiked peptides, but also crucially the completeness of the quantitative matrix (i.e. rate of missing values) was a substantial improvement over what could be achieved by DDA-based methods. |
As we started to analyze bigger datasets with queries from bigger libraries[23] we realized that stronger statistical controls were required to keep the errors from inflating. This included calculating the false discovery rate (FDR) in different contexts and at the inferred protein level. We felt this warranted a second separate paper to accompany our multi-lab SWATH MS study that addressed this problem.[34] Our multi-lab study was strongly focused on data acquisition across labs but not data analysis. However, a parallel study (LFQbench[35]) involving many of the software developers in the DIA space showed using benchmarking samples that the various pipelines were converging well on similar answers. Further DIA benchmarking studies expanded on these ideas on different measurement platforms, using different sample types, and at larger scale.[36,37] My view is that the DIA community has to some extent been leading the way in such benchmarking studies for large-scale quantitative proteomics in recent years, and this effort has likely led to the strongly increased adoption and application of DIA that we have seen in the proteomics community and beyond. |
![]() |
Many additional strategies for DIA have emerged and were performed on various powerful high-resolution MS platforms, as reviewed by Kitata et al.[15] These innovations typically aimed to achieve deeper proteome coverage, higher reproducibly, and highly confident detection and quantification of peptides and proteins. One example was the coupling of DIA workflows with ion mobility in the form of field asymmetric ion mobility spectrometry (FAIMS)[38–40] or trapped ion mobility (TIMS)[41,42] to reduce spectral complexity and further enhance the specificity and sensitivity of DIA analysis. We reached out to Dr. Florian Meier-Rosar about his work in Dr. Matthias Mann’s laboratory on the impact of ion mobility separation with diaPASEF (PASEF = parallel accumulation serial fragmentation) on DIA data quality (Box 3).
Box 3. Question and answer. |
Q. How did the combination of DIA with ion mobility improve protein quantification? |
A. The potential of ion mobility separation to reduce the complexity of proteomics samples has long been recognized. With increased sensitivity and ease of implementation, as well as advances in bioinformatics, the latest generation of ion mobility devices has finally entered the main stage of proteomics in various workflows and instrument platforms. We focused primarily on the trapped ion mobility spectrometry (TIMS) technology, in which ions are captured and sequentially released to the downstream mass analyzer in narrow ion mobility peaks.[43] diaPASEF takes advantage of the correlation between mass and mobility to select peptide precursor ions for fragmentation as they are released from the TIMS device. This greatly enhances the ion utilization in DIA and positions both precursor and fragment ions precisely in a dense data cuboid spanned by m/z, retention time, and ion mobility. Ion mobility thus adds another criterion for the evaluation of peptide identifications and improves quantitative accuracy by reducing signal interferences. |
![]() |
Even 10+ years since the first publication of the SWATH MS/DIA-MS workflow,[11] researchers are continuing to evolve the MS acquisition strategies, leveraging the improving MS instrument functionality and sensitivity to further optimize DIA workflows. While DIA-MS typically consists of a stepwise selection of precursor ion isolation windows, a recently demonstrated acquisition functionality (Scanning SWATH DIA) relies on the continuous movement of the isolating quadrupole with the fragmentation of all precursor ions in each frame.[44] This acquisition mode was compatible with extremely fast gradients and provided significant gains in quantified precursor ions (~70%) for 10 μg human K562 cell line digestion compared to SWATH DIA performed on the same platform. More recently, new strategies coupling trapped ion mobility with better matched and synchronized quadrupole isolation windows have been developed (Synchro-PASEF) although benchmarking on complex proteomic samples has not yet been completed.[45] In another example using the increased MS/MS sensitivity afforded by the Zeno trap technology, the Zeno SWATH DIA workflow[46] enabled quantification of the same number of proteins from a 10× lower sample load of a human cell lysate, as compared to the SWATH DIA on the same platform.
Increased scan speed and chromatographic improvements to enable large cohort studies
During DIA-MS, MS/MS is not sequentially acquired on every coeluting precursor ion, thus method cycle times can be shortened, and the liquid chromatography (LC) gradients can be greatly reduced. This enabled the exploration of chromatographic strategies utilizing higher flow rates, including microflow rates (1–10 µL min–1)[47,48] and analytical flow rates up to 200–800 µL min–1.[44,49] Much faster gradient times, down to 5 min gradients, in combination with DIA performed on latest generation mass spectrometers has accelerated sample analysis such that 180 proteome maps can be collected per day.[44] With higher flow rates comes higher chromatographic robustness, which further enables the analysis of larger biological studies, such as the 1508 plasma samples analyzed by Bruderer et al.[50] While the sensitivity at higher flow rates can be slightly reduced,[51] there is often enough sample material available to scale up the amount of material injected. Faster acquisition times allow for higher throughput to perform larger, more statistically powered studies. We asked Dr. Bernhard Küster to discuss his journey of migrating his proteomic workflows to higher chromatographic flow rates (Box 4).
Box 4. Question and answer. |
Q. How has microflow chromatography influenced proteomic DIA-MS workflows, with its improved robustness and throughput? |
A. It is increasingly recognized that the performance of proteomic experiments not only relies on the speed and sensitivity of the mass spectrometers employed, but also the very substantial separation power provided by liquid chromatography.[52] While nanoLC has dominated the field for over two decades because it provides sensitivity, higher flow LC systems such as capillary-flow (capLC) and micro-flow (microLC) that achieve higher peptide separation power are emerging as viable alternatives for a range of applications.[53] Higher LC flow rates improve the robustness of separations (sharper chromatographic peaks and more reproducible retention times) and electrospray ionization. System dead volumes can also be bridged more rapidly than in nanoLC, in turn enabling higher sample throughput. However, this does come at the price of loss of sensitivity.[54] Hence, it can be anticipated that microLC will be most impactful in the analysis of protein expression (changes) for applications where sample quantities are not scarce (body fluids, full proteomes of tissues and cell lines). This enables the execution of very large-scale experiments comprising tens of thousands of experiments.[55] |
Integrating microLC separations into DIA workflows is conceptually very attractive. But it turns out to be a curse and a blessing at the same time. The narrow LC peaks and the higher ion current sampling of DIA offset much of loss of sensitivity compared to nanoLC or capLC. However, DIA comes with the need to sample the chromatographic peak multiple times (minimum of 7–10) for each DIA window in order to obtain robust quantification. At chromatographic peak widths of 2–5 s provided by microLC separations, this continues to challenge even the fastest current mass spectrometers that feature acquisition rates of 50–200 spectra per second.[56] |
![]() |
As mentioned, when adapting DIA methods for higher flow chromatography, it is important to adjust the acquisition method (number of isolation windows and time per window) to ensure the cycle time is appropriate for the narrower microflow peak (typically 7–10 points across the LC peak). Depending on the gradient length, sample complexity, and load, DIA method optimization should be performed to ensure highly quantitative data is obtained, as demonstrated by Sun et al.[31]
To generate statistically powered biomarker research datasets across the broad range of human diseases, it is critical to perform studies on large sample cohorts of 100s or 1000s of samples.[50,57] This can be achieved by using faster, more robust chromatography but also generating data on multiple instruments for increased study capacity. These larger studies pose multiple challenges: automating the preparation of biological samples such that it is reproducible over extended time periods, maintaining instrument performance to generate reproducible label-free quantification data sets over time, combining results obtained from various instruments and over an extended time period, and extracting significant, relevant biological information across a large study. The team at Children’s Medical Research Institute (CMRI) led by Dr. Phil Robinson has developed a full pipeline that integrated multiple mass spectrometers for the analysis of large cancer tissue cohorts (Box 5).
Box 5. Question and answer. |
Q. To analyze large tissue biopsy cohorts, what workflows, including data analysis strategies, have you implemented for maintaining reproducibility across multiple instruments across an extended time period? |
A. ProCan is a high throughput MS center focused on human cancer. At the outset we decided to base our approach on DIA technology because of the ability to digitize proteomes and to be able to mine and remine the data. True high throughput requires a sample preparation workflow that is rapid, has the fewest possible handling steps, and is fully automatable. It requires a fleet of harmonized LC–MS instruments capable of 24/7 operation with maximum up-time in a dedicated center. Finally, the generated data needs to be robust and reproducible down the years. We have found that DIA is an ideal technology to achieve these aims.[37] We developed short one pot sample processing with preparation times in under an hour that has now been used across our cohorts for years. The method is essentially universal and used for human cancer sections from fresh frozen OCT-embedded (FF-OCT), FFPE, or frozen cell line pellets. Using this, we have processed over 16 000 human cancer samples from 141 broad tumor types and almost 2000 cell line pellets from over 40 tumor types, producing over 61 000 MS runs in under 6 years. The consistent sample preparation method has been pivotal to long-term reproducibility. For example, we have been able to show using PCA that samples re-run after 1–3 years highly cluster with the original DIA-MS runs, providing evidence of a robust long-term stability of the acquired DIA data on our platform. Based on this, our goal is to continue to build our pan-cancer map into a knowledgebase of the human cancer proteome to be able to predict cancer type and tissue of origin of any sample, and towards the goal of predicting patient outcomes or guiding treatment decisions within specific classes of cancer.[58] |
![]() |
Data processing innovations further extend DIA workflows
In addition to the evolution and improvements for DIA-MS data acquisition, DIA data processing post-acquisition has continuously improved and contributed to large leaps in protein detection and more accurate protein quantification. Many different tools were developed over the years such as OpenSWATH,[19] DIA-NN,[59] MS Fragger,[60] and many others.[15] As DIA-MS/MS spectra are inherently complex and convoluted, dedicated algorithms for data extraction and peak group scoring are required for peptide identification and quantification.[11] Since the early tools, numerous algorithms, software tools, and library generation tools have been developed, thoroughly evaluated,[35,61,62] and summarized by Kitata et al.[15] Dr. Hannes Röst has been involved in developing algorithms for DIA data processing from the very earlier days, and we asked him to discuss the current situation (Box 6).
Box 6. Question and answer. |
Q. What is the state-of-the-art in terms of DIA data processing and reporting, and what are the remaining gaps to close? |
A. We have indeed come a long way since the very first studies and today we have a vibrant ecosystem of software that all support DIA data analysis both on the Desktop using a graphical user interface, as well as platforms that allow us to analyze tens of thousands of DIA runs on computing clusters or the cloud. Both open-source and commercial software are available, and more recently we have seen strong interest in using machine learning both for library generation as well as for raw data analysis. However, there are several areas of active research in DIA methods and software, where much fewer options are available for reliable data analysis. These areas include specialized workflows for enriched samples like pull-downs, non-tryptic peptides, as well as modified peptides (post-translational modifications, cross-linked peptides etc.). Highly interesting are small molecules and lipids where few software packages exist for targeted data extraction at large scale and many steps of our standard workflow (such as library generation or FDR control) need to be re-evaluated. Finally, exciting innovation in instrumentation creates novel opportunities for DIA, such as the recent development of diaPASEF which exploits the TIMS both as a trapping and separation device.[41] |
![]() |
As mentioned by Dr. Röst, more machine learning-based approaches[63–65] were implemented into DIA-MS processing tools, e.g. DIA-NN,[59] MaxDIA,[66] or commercial directDIA 2.0 modules embedded in Spectronaut (Biognosys),[67] which all result in a significant increase in protein/peptide identification and quantification accuracy. We asked Oliver Bernhardt to discuss how machine learning and artificial intelligence (AI) have impacted the interrogation of DIA data and interpretation of results (Box 7).
Box 7. Question and answer. |
Q. How have innovations in machine learning and artificial intelligence helped DIA data, and how close are we to extracting all data stored in high-quality DIA files? |
A. The advent of AI in proteomics has certainly changed the landscape of data processing in MS-proteomics. Workflows that would have been unthinkable just a few years back suddenly do not only become viable but are what pushes the field further and further. I feel like the landmark work on Prosit (an artificial neural network architecture for high-accuracy predictions of retention times and fragment ion intensities)[68] is to a large extent responsible for this AI-proteomics gold rush that we are currently observing. It cemented the idea of AI assisted data analysis workflows in the minds of the wider community and sparked many interesting spin-off ideas. How far are we to extracting all data? I think we, as a community, have made tremendous gains in the last couple of years in explaining more and more of the observable ion currents. It is, however, evident when looking at all measured MS1 features that we are only scratching the surface at the moment. Getting better quantification from those hard to identify signals will be the next big challenge. Personally, I hope we don’t reach the point where we have extracted ‘all’ data any time soon as it would make my job suddenly a lot less interesting. |
![]() |
Where do we go next for DIA data processing and protein quantification? Are there more biological insights to be gained if we rethink the way we roll up transition-level information to peptide-level information to the protein level, to better represent post-translational modifications (PTMs) and other proteoforms, such as splice variants and protein degradation products? The mapDIA tool[69] performs DIA data normalization, filtering, and differential protein statistical analysis at the fragment level, taking advantage of the repeated transition and peptide measurements obtained for each protein with DIA-MS. However, there are still challenges when considering peptide quantification and concerns as to whether peptide quantification results should be used to infer protein quantification due to many different proteoforms and PTMs present in the peptide data. Plubell et al. discuss this in detail providing deeper insights into proteoform quantification.[70]
Biological applications featuring the power of DIA-MS
Because of the high quality and reproducible quantification achieved using DIA-MS, this strategy has been applied to a plethora of areas, including proteome characterization, biomarker research, and drug discovery in large-scale clinical and disease studies. Protein quantification by DIA provided insights into many disease areas, such as neurobiology,[71–73] cancer research,[58,74–76] drug-metabolizing enzymes and transporters,[77,78] virology,[42,79,80] and many others. Increasingly, DIA is used to quantify specific PTM changes during biological processes or during the manifestation and progression of diseases. Here, we asked several scientists to discuss why they have adopted the DIA-MS approach for their work.
Proteomic workflows have been broadly used for PTM analysis and more recently DIA-MS has become more prevalent; however, there are some inherent challenges, such as PTM site localization[81] and low stoichiometry of many PTMs. Modifications are often highly dynamic and transient, occur with low occupancy, and small abundance changes can lead to impactful biological signaling and trigger functional changes of proteins. PTM enrichment strategies,[82,83] such as IMAC[84] or using antibody-based enrichments,[85,86] have been coupled with DIA analysis to decrease sample complexity and increase the relative abundance of the modified peptide forms. However, these strategies typically lead to limited sample material after PTM enrichment. Labile PTM groups may result in neutral losses during MS analysis, and some PTMs with small mass increments may lead to DIA co-isolation of both the modified and the unmodified precursor ions in the same MS1 m/z window. Drs. Jennifer Van Eyk and Justyna Fert-Bober (Box 8) developed a novel DIA-MS workflow for the study of citrullination which is a particularly challenging PTM to study due to a resulting mass change of +~1 Da for arginine residues.
Box 8. Question and answer. |
Q. How have you leveraged DIA for the study of citrullination, where the mass change is +~1 Da? |
A. Protein citrullination, an enzymatically produced PTM, is the result of deimination of the side chain of arginine. This irreversible PTM converts the guanidium group of the arginine residue into an ureido group resulting in the production of the non-standard amino acid, citrulline, release of ammonia and the subsequent loss of a positive charge, and a monoisotopic mass increase of +0.984016. The mass increment of 0.9840 Da compared with the unmodified arginine is small and in fact identical to the frequently occurring deamidation of the amino acid asparagine (Asn/N) and glutamine (Gln/Q) residues, leading to serious ambiguity in database searches and reports. We use DIA-MS to allow us to exploit the retention time increase that occurs consistently with each citrullinated residue within a peptide at the MS/MS spectra level to ensure the correct site localization of this PTM with or without a neutral loss.[87] To increase observation of this rare PTM, we developed tissue-specific citrullination enriched peptide libraries by up-regulating expression of the enzyme peptidylarginine deiminase (PAD) that generates the citrullination modification, and use this to search our DIA-MS data.[88] Finally, we developed an analytical data processing pipeline to help the broader community to obtain high-confidence site specific citrullination data.[87] These DIA-MS tools have allowed us to describe the landscape of protein citrullination more comprehensively in heart disease,[89,90] inflammation,[91] and brain injury.[92,93] |
![]() |
![]() |
DIA data processing often relies on the generation of experimental or in silico MS/MS spectral libraries;[81] however, this presents a unique challenge when studying PTMs. Dr. Birgit Schilling, among others,[94] has been involved in developing alternative and efficient strategies for PTM analysis by DIA-MS and discusses how they enable comprehensive and accurate PTM identification, site localization, and quantification to be acheived (Box 9).
Box 9. Question and answer. |
Q. Which recent data processing innovations have made DIA-MS PTM studies more straightforward? |
A. I think DIA is an extremely powerful tool specifically for analysis of post-translational modifications and for addressing the specific challenges that come with mass spectrometric PTM analysis. DIA-MS ensures that all PTM-containing peptide isomers are monitored and sampled for MS/MS, ensuring that lower abundant isoforms are not missed because they were below a DDA sampling threshold or dynamically excluded during DDA. DIA-MS for PTMs foremost allows for much more accurate PTM site localization. Post-acquisition extraction of MS/MS fragment ions for each PTM containing peptide isomer allows for assessment of the presence and absence of isomer distinguishing fragment ions, and these are subsequently scored to determine PTM site localization, followed by quantification. For identification, site localization, and quantification of PTM sites, we use Spectronaut, which provides efficient site localization scores, quantification, and visualization. Additional visualization and quantification of PTM-containing peptides is often performed in Skyline.[95] As PTM protocols typically contain some type of enrichment for the PTM-containing peptides, PTM workflows often yield very small amounts of input material. Meyer et al.[96] employed a library-free workflow via DIA-Umpire[97] and Skyline[95] in order to use the same DIA-MS workflow to identify the PTM containing peptides, to build a spectral library, and to quantify. Recent work by Bekker-Jensen et al.[94] and Bons et al.[86] used Spectronaut to employ similar library-free workflows (directDIA) for PTM quantification for phospho proteomics and for succinylome/malonylome analysis, respectively. |
![]() |
Understanding biology goes beyond the characterization of the primary protein structures and how the total abundance of a single protein changes. How proteins fold, change in structure, form complexes with other partners, and the dynamics of these changes are also extremely important in order to gain a full picture of a biological process. Another important aspect of protein dynamics is protein turnover, which is multi-faceted because it includes both the rates of synthesis and degradation of a protein. In addition, the protein changes can be correlated with the transcript changes to build a more refined picture; however, this has been a challenging aspect for multi-omics studies. This is an area in which Dr. Yansheng Liu investigated applications of DIA-MS for innovative new protein turnover workflows (Box 10).
Box 10. Question and answer. |
Q. What were the critical attributes of the DIA generated proteomics data that made analysis at the proteoform level more powerful? |
A. DIA-MS provides several extraordinary attributes for quantifying protein turnover rates at the proteome scale using, e.g. the dynamic SILAC (or pSILAC) approach. First, the high reproducibility of DIA ensures that much fewer missing values are generated in the pSILAC experiments, which almost always include multiple samples due to the usage of a time course design. This advantage translates to a deeper profiling of proteome turnover. Second, the heavy and light (H/L) ratios in pSILAC (or SILAC) can be quantified at the peptide fragment level with almost 10 times more quantitative features than the traditional MS1-based approach. Third, DIA embraces the retention time (RT)-associated properties for peptide identification and, therefore, better supports the differentiation between peptide variants, enabling the correlation analysis between proteoforms and spliced transcripts at the isoform level. Finally, DIA-MS offers great flexibility for data mining. For example, we have applied the inverted spike-in workflow (ISW), an algorithm previously developed for labeling MRM analysis, to analyze pSILAC-DIA data,[98] which significantly improved the peptide detection rate at the early time points in the pSILAC experiment. |
![]() |
Some biological processes are driven by peptides, such as hormones[99] and neuropeptides,[100] and are associated with cellular signaling[101] and immune response.[102] MS workflows have been used extensively in such studies. One very important field of peptide research is immunopeptidomics, which aims to decipher the complexity and dynamism of peptide ligands presented by human leukocytes antigen (HLA) complexes at the cell surface of antigen-presenting cells to elicit an immune response. Dr. Anthony Purcell has been a pioneer in this field and was an early adopter of DIA to deeply characterize these challenging peptide samples (Box 11).
Box 11. Question and answer. |
Q. What has been a recent application of DIA in this field that has been particularly exciting? |
A. Studying the processing and presentation of viral antigens not only helps us to identify the specific targets of antiviral T cells but to also understand fundamental processes in all cells that can be harnessed for immunotherapy and vaccine design. Together with my colleagues, Nathan Croft and David Tscharke, we used MRM-based studies of the kinetic appearance of a few select T cell epitopes on the surface of vaccinia virus (VACV) infected cells.[103] Here we observed that a number of these epitopes appeared in parallel to or even before the mature viral antigen could be detected in the cells. This provided biochemical evidence for the existence of defective ribosomal initiation products (DRiPS) that were initially proposed to explain the rapid presentation of viral antigens to CD8+ T cells.[104] The advent of DIA-MS presented us with a chance to look more globally at antigen expression and the kinetics of antigen presentation and, when applied to VACV infected cells, allowed a more system wide study of viral antigen presentation[105] and T cell epitope immunogenicity.[106] These studies indicated three kinetic classes of antigens – DriPs, those derived from rapidly degraded mature antigen, and those generated from longer lived antigen that appear later during infection. This really demonstrated how the immune system had evolved to sense the viruses very early during infection allowing rapid elimination of infected cells. Fast forward a few years and the list of such non-canonical sources and translational errors that give rise to antigens have grown, opening up systematic studies of novel cancer antigens and vaccine targets in infectious diseases like influenza and SARS CoV-2. |
![]() |
Beyond traditional proteomics
As the DIA technique became more accepted in the proteomics field, highly specialized applications emerged. The development of protein therapeutics is a relatively new area in the pharmaceutical field. These drugs are typically manufactured using a process that greatly differs from small molecule drug production workflows, as it relies on protein expression systems and large cell culture workflows using bioreactors.[107] Purification of the protein drug from cell culture fluids typically involves multiple steps to achieve a final drug product with the highest purity possible. Impurities, including contaminating proteins or host cell proteins (HCPs), need to be reduced to as few as possible. This creates many different challenges for quality control of drug manufacturing and characterization of the final drug product. Dr. Christine Carapito was interested in how DIA might be applied to this problem and worked with pharmaceutical companies to investigate best practices (Box 12).
Box 12. Question and answer. |
Q. How did the DIA methodology enable you to overcome the challenges of profiling and quantifying low abundant host cell proteins? |
A. Indeed, characterizing HCP impurities remaining in therapeutic proteins such as monoclonal antibodies (mAbs) turned out to be the most challenging proteomics question I had to face. The use of DIA methods on various instruments including Q-TOFs and Q-Orbitraps allowed us to reach the sensitivity and quantification accuracy/precision of targeted methods (SRM or PRM) down to sub-ppm level HCPs, while providing the global profiling of HCPs.[108,109] The use of MS2 data is highly beneficial to achieve non-interfered signal and thus more robust quantification in matrices holding such an extreme dynamic range (six logs between the mAb and HCP in final drug substances). Finally, the recent promising improvements of library-free DIA data processing (including AI-based predictions) constitute a real asset for HCP characterization especially for end-product characterization and quality control for which producing cell lines may not be available to generate experimental libraries. |
![]() |
Experimental spectral libraries are now available for use in DIA studies across an increasing number of species, as summarized in Kitata et al.,[15] and have been made available for download from the SWATHAtlas repository (http://www.swathatlas.org/).[110] This expanded availability of libraries, as well as workflows for the generation of experimental libraries,[20] allowed DIA-MS to be used in an increasing number of application areas beyond the study of human biology. The study of the proteins in organisms key to the food supply, both for humans and other animals, is an important research area in food science and the use of DIA techniques in the area was pioneered by Dr. Michelle Colgrave of Commonwealth Scientific and Industrial Research Organisation (CSIRO, Australia) (Box 13).
Box 13. Question and answer. |
Q. How have DIA-MS workflows been critical to success in the food sciences? |
A. Proteomics is commonly applied to clinical and medicinal science. But to me, food is the number one medicine and protein is a critical macronutrient. Proteins fuel our bodies, bioactive proteins provide immunity or other health benefits (cardiovascular, antimicrobial, and more) but some proteins are also ‘villains’. These antinutritive proteins can inhibit food digestion, cause intolerance or even life-threatening allergy. My research has used DIA to not only characterize food ingredients, exploring genetically diverse inputs (different crop varieties) to find the ones that are rich in bioactives, but absent or low in antinutritionals – this informs our breeding programs to create the ingredients of the future to improve our health status. We also deploy the same DIA technology to measure the impact of food processing (fermentation, formulation) on these proteins and ask can we maintain the hero proteins while destroying the villains – you can think of DIA as the ‘cape’ that our proteomics teams wear allowing us to fly through the protein universe on a quest to deliver optimized nutrition for all! |
![]() |
Many of these non-human studies that require MS-based proteomics analysis rely on the core laboratories of universities or research institutes, which support researchers with a broad range of analytical technologies.[111] Adoption of DIA into a mass spectrometric core facility faced hurdles, mainly due the challenge of library creation. Many studies received by core labs are performed using non-human or non-mouse organisms which previously required additional DDA acquisition time to build custom libraries. Dr. Brett Phinney discusses his experiences in bringing DIA into his core facility in Box 14.
Box 14. Question and answer. |
Q. From a proteomics core facility perspective, what have been the past barriers and what are current strengths of DIA for a busy core lab? |
A. In my opinion, the barrier to using DIA in a core facility has always been the lack of easy-of-use and robust software and reliance on spectral libraries. Both are not really problems anymore. Now that sensitivity of DIA library-free approaches has matched, or in most cases, exceeds DDA, there is virtually no downside to doing DIA in a core facility. Sensitivity is better, quantification is ‘night and day’ better, and it’s just as easy to analyze a wide variety of samples from different organisms. We have used DIA on everything from a 250K year old mammoth tooth, potato pollen, COVID nasal swabs, pig and bull sperm, turnips, bok-choy, and cricket protein extract! |
![]() |
The field of lipidomics has been exploring comprehensive MS/MS acquisition strategies for infusion-based shotgun lipidomics workflows. On earlier generation instruments, the MS/MS was interrogated for key fragments diagnostic of specific lipid classes (multi-precursor ion scanning[112]), then advancements in MS instrumentation allowed for full scan MS/MS interrogation providing lipid class and molecular species information (Infusion MS/MSAll[113]). Increasingly, DIA-MS was explored for the quantification of small molecular weight exogenous and endogenous molecules in various matrices;[114–116] however, broad adoption was challenged by limited by lack of: (i) software tools, (ii) libraries with broad analyte coverage, and (iii) quantitative standards as Dr. Bruno Manadas discusses (Box 15).[117]
Box 15. Question and answer. |
Q. Why has the use of DIA in metabolomics been lagging behind the adoption in proteomics? |
A. In my opinion, one of the issues with performing DIA for metabolomics is the inconsistency of the intensities of the different fragments. In our hands, the development of targeted approaches for peptide quantification (MRM or DIA) has less variability than the same procedures adapted for metabolites. We reject more transitions when developing MRM methods for metabolites because they lack reproducibility as compared to peptide MRM development. Furthermore, for peptide analysis, we usually apply rolling collision energy (CE), calculated considering the mass-to-charge ratio and the charge state of the peptides.[118] The weak peptidic bond results in more reproducible fragmentation spectra due to the highly optimized CE. This is much less predictable for metabolites, so we use a large CE ramp or spread (CES) applied around a centered CE.[119] This strategy is not currently standardized between labs, highlighting a challenge and a need for the metabolomics workflow. |
We have developed our metabolite spectral databases on pure compounds to use for processing the DIA data. But we found that fragmentation spectra produced from pure compounds can differ from the spectra observed in a complex biological matrix acquired using our standard gradients. This, in turn, results in more challenging requirements for the software tools to perform unbiased metabolite identification from DIA data files. There is still a long way to go until DIA can be applied to metabolomics as broadly as with proteomics, but the foundations are being created. |
![]() |
Xenobiotic screening methods using DIA provides the added value of providing both quantitative information on known target compounds and acquiring information on many other unknown or unexpected compounds.[116] This is key in forensic work to fully unlock the secrets of every sample, ensuring no analytes are missed during the analysis and enabling retrospective investigations to monitor emerging trends,[120] as Dr. Alex Krotulski discusses in Box 16.
Box 16. Question and answer. |
Q. What attributes of DIA made it compelling for forensic testing applications? |
A. DIA provides more certainty and reliability when acquiring data on a HRMS platform, aspects that are critical to both forensic analysis and research. Specifically, correct development and implementation of DIA methods ensure that data will be acquired and available for scientific review, and further exploration, regardless of sample type and drugs and/or metabolites present. This data includes the MS/MS fragment spectrum, which is a critical piece to the puzzle and is the data element with the highest specificity acquired during analysis. DIA approaches lend themselves better to our strategies of sample-mining and data-mining, prospective and retrospective approaches, respectively, that allow our laboratory to monitor and surveille drug markets and their latest emerging changes. For our program, it is critically imperative that MS/MS data is acquired during analysis, for both drugs we know about and those we don’t, because our identification must be of high certainty and quality – DDA methods, even when using a target list, do not provide this same level of comprehensive data acquisition which renders the final data file less useful than DIA data files. DIA ensures peace of mind in the laboratory that is necessary for the current advance state of modern forensic toxicology. |
![]() |
High sensitivity DIA for single cell analysis
Recent technology improvements providing a large increase in MS sensitivity and miniaturization of sample preparation led to the possibility to analyze increasingly smaller amounts of input material, where today laboratories report to successfully identify ~1000–2000 proteins per cell,[121,122] recently reaching ~3000 protein groups quantified from a single mouse lung epithelial cell.[123] The first reports employed DDA workflows and isobaric tagging, such as SCoPE-MS,[124] but recently laboratories explored whether DIA might be a useful strategy.[125,126] Dr. Nikola Slavov speaks about some of the latest workflow innovations using DIA for single cell analysis in Box 17.
Box 17. Question and answer. |
Q. What drove the desire to move your single cell workflow to using DIA with non-isobaric tags for the study of single cell proteomics? |
A. The depth of single-cell proteomics by DDA is limited in large part by the inability to analyze all detectable precursor ions with the required long accumulation times as discussed by Slavov.[127] DIA overcomes this problem since it allows obtaining MS2 fragmentation spectra from all detectable peptide features even when using long ion accumulation times. This makes it attractive for analyzing small samples, such as single cells. Indeed, sensitive MS analysis detects over 60 000 peptide-like precursor ions from a single human cell, and parallel isolation and fragmentation of precursor ions may allow analyzing all of them at the MS2 level.[128] |
DIA allows for efficient multiplexing using non-isobaric mass tags, which traditionally has undermined depth of coverage by DDA. Such tags should be much easier and cheaper to design and manufacture than isobaric tags. Thus, we expect these tags to substantially increase the throughput and accessibility of sensitive protein analysis. Extrapolation of our 3-plexDIA results to a 100-plexDIA predicts the feasibility of analyzing the proteomes of about 5000 cells per day using a single MS instrument. This possibility of multiplicative scaling is discussed by Slalov.[129] |
![]() |
Obtaining the highest duty cycle on MS/MS fragment ions is critical for high sensitivity applications like single-cell proteomics or spatial proteomics. Recently, a further adaptation to the diaPASEF workflow, termed Slice-PASEF, was developed to enable more refined optimization between sensitivity and specificity by dividing each PASEF frame (PASEF = parallel accumulation serial fragmentation) into several quadrupole isolation windows.[130] This method provided increases in quantified proteins (52%) compared to original diaPASEF technology, even at very low sample loads (10 ng of human K562 digest) and can be applied in combination with microflow chromatography.
Conclusions/future perspectives
The steady improvement in speed and sensitivity of mass spectrometric instrumentation combined with the numerous advancements in data analysis have enabled DIA workflows to quantify more and more proteins from every sample using much less biological material. Today, highly informative proteomic maps can be rapidly generated using DIA, providing more proteins and peptides quantified with more confidence in the results, as Dr. Christie Hunter explains below (Box 18).
Box 18. Question and answer. |
Q. What has impressed you most in the technological developments for DIA workflows? |
A. This DIA journey that our proteomics community has been on for the last decade has been one of creativity, innovation, and collaboration. The progress made in terms of number of proteins quantified per unit time and amount of sample used has been phenomenal! In the early days of DIA, quantification of ~4000 proteins from 1 µg of human cell lysate using a 120 min nanoflow gradient was consistently achievable across labs.[30] With the increased speed and sensitivity of the high resolution mass spectrometers, we are now quantifying many more proteins from less sample material and performing sample analysis much faster for larger studies. Today we can quantify over 6000 proteins from a human cell lysate from 10× less material (62.5 ng of a human cell lysate) using a six-fold faster microflow gradient (20 min),[46] or quantify ~5000 proteins from 10 μg in a 5 min analytical flow gradient.[44] We even routinely talk about LC–MS methods in terms of samples per day (SPD), coined for the fast microflow gradients we now use routinely.[131] Obtaining deep proteome maps across 1000s of samples in a study is now truly a reality, the age of clinical and translational proteomics is finally here! |
![]() |
The unique and powerful capability of DIA-MS to generate digitalized maps of samples that can be interrogated retrospectively and indefinitely had made this approach highly valuable for many study types, from the study of human cancer[75] to the monitoring of illegal drug trends.[120] In proteomics research, DIA-MS has been used to characterize many aspects of the proteome, from splice variants and post-translational modifications to elucidating protein complexes, and quantify protein abundance, all driving better annotation of proteomes.
Workflow refinements, such as adding additional separation strategies, for example ion mobility or FAIMS, or improvements in scan dimensions like Scanning SWATH, will further reduce spectral complexity and improve detection confidence and quantitative accuracy in DIA-MS data. Advances in machine learning have provided significant improvements in the information mined from DIA datasets,[59] in terms of proteins confidently detected and quantified, and there are likely more advances to come here.
Study of the human proteome is a key component of precision medicine research, driving the need for obtaining deeper single shot proteomes with very complete quantitative data matrices that can be mined for biological insight. Performing larger studies with better population representation and longitudinal sampling where possible[132] is another step towards personalized medicine but requires analytical approaches with higher throughput and high reproducibility. DIA-MS is uniquely poised to play a major role in this important research, as demonstrated by a steadily growing number of large‐scale clinical proteomics studies.
Data availability
No novel primary data are presented in this review. For access to data discussed in this review, refer to the original publication.
Acknowledgements
The authors truly thank all the interviewees, who have kindly participated in this review.
References
1 Aebersold R, Mann M. Mass-spectrometric exploration of proteome structure and function. Nature 2016; 537(7620): 347-55.
| Crossref | Google Scholar |
2 Schilling B, Rardin MJ, MacLean BX, Zawadzka AM, Frewen BE, Cusack MP, et al. Platform-independent and label-free quantitation of proteomic data using MS1 extracted ion chromatograms in skyline: application to protein acetylation and phosphorylation. Mol Cell Proteomics 2012; 11(5): 202-14.
| Crossref | Google Scholar |
3 Cox J, Hein MY, Luber CA, Paron I, Nagaraj N, Mann M. Accurate proteome-wide label-free quantification by delayed normalization and maximal peptide ratio extraction, termed MaxLFQ. Mol Cell Proteomics 2014; 13(9): 2513-26.
| Crossref | Google Scholar |
4 Picotti P, Aebersold R. Selected reaction monitoring-based proteomics: workflows, potential, pitfalls and future directions. Nat Methods 2012; 9(6): 555-66.
| Crossref | Google Scholar |
5 Anderson L, Hunter CL. Quantitative mass spectrometric multiple reaction monitoring assays for major plasma proteins. Mol Cell Proteomics 2006; 5(4): 573-88.
| Crossref | Google Scholar |
6 Zhang H, Liu Q, Zimmerman LJ, Ham A-JL, Slebos RJC, Rahman J, et al. Methods for peptide and protein quantitation by liquid chromatography-multiple reaction monitoring mass spectrometry. Mol Cell Proteomics 2011; 10(6): M110.006593.
| Crossref | Google Scholar |
7 Gallien S, Duriez E, Crone C, Kellmann M, Moehring T, Domon B. Targeted proteomic quantification on quadrupole-orbitrap mass spectrometer. Mol Cell Proteomics 2012; 11(12): 1709-23.
| Crossref | Google Scholar |
8 Peterson AC, Russell JD, Bailey DJ, Westphall MS, Coon JJ. Parallel reaction monitoring for high resolution and high mass accuracy quantitative, targeted proteomics. Mol Cell Proteomics 2012; 11(11): 1475-88.
| Crossref | Google Scholar |
9 Schilling B, MacLean B, Held JM, Sahu AK, Rardin MJ, Sorensen DJ, et al. Multiplexed, Scheduled, High-Resolution Parallel Reaction Monitoring on a Full Scan QqTOF Instrument with Integrated Data-Dependent and Targeted Mass Spectrometric Workflows. Anal Chem 2015; 87(20): 10222-9.
| Crossref | Google Scholar |
10 Doerr A. Targeted proteomics. Nat Methods 2009; 7(1): 34.
| Crossref | Google Scholar |
11 Gillet LC, Navarro P, Tate S, Röst H, Selevsek N, Reiter L, et al. Targeted data extraction of the MS/MS spectra generated by data-independent acquisition: a new concept for consistent and accurate proteome analysis. Mol Cell Proteomics 2012; 11(6): O111.016717.
| Crossref | Google Scholar |
12 Meyer JG, Schilling B. Clinical applications of quantitative proteomics using targeted and untargeted data-independent acquisition techniques. Expert Rev Proteomics 2017; 14(5): 419-29.
| Crossref | Google Scholar |
13 Venable JD, Dong MQ, Wohlschlegel J, Dillin A, Yates JR. Automated approach for quantitative analysis of complex peptide mixtures from tandem mass spectra. Nat Methods 2004; 1(1): 39-45.
| Crossref | Google Scholar |
14 Silva JC, Denny R, Dorschel CA, Gorenstein M, Kass IJ, Li GZ, et al. Quantitative proteomic analysis by accurate mass retention time pairs. Anal Chem 2005; 77(7): 2187-200.
| Crossref | Google Scholar |
15 Kitata RB, Yang JC, Chen YJ. Advances in data-independent acquisition mass spectrometry towards comprehensive digital proteome landscape. Mass Spectrom Rev 2022; e21781.
| Crossref | Google Scholar |
16 Picotti P, Rinner O, Stallmach R, Dautel F, Farrah T, Domon B, et al. High-throughput generation of selected reaction-monitoring assays for proteins and proteomes. Nat Methods 2010; 7(1): 43-6.
| Crossref | Google Scholar |
17 Reiter L, Rinner O, Picotti P, Hüttenhain R, Beck M, Brusniak MY, et al. mProphet: automated data processing and statistical validation for large-scale SRM experiments. Nat Methods 2011; 8(5): 430-5.
| Crossref | Google Scholar |
18 Röst H, Malmström L, Aebersold R. A computational tool to detect and avoid redundancy in selected reaction monitoring. Mol Cell Proteomics 2012; 11(8): 540-9.
| Crossref | Google Scholar |
19 Röst HL, Rosenberger G, Navarro P, Gillet L, Miladinović SM, Schubert OT, et al. OpenSWATH enables automated, targeted analysis of data-independent acquisition MS data. Nat Biotechnol 2014; 32(3): 219-23.
| Crossref | Google Scholar |
20 Schubert OT, Gillet LC, Collins BC, Navarro P, Rosenberger G, Wolski WE, et al. Building high-quality assay libraries for targeted analysis of SWATH MS data. Nat Protoc 2015; 10(3): 426-41.
| Crossref | Google Scholar |
21 Ludwig C, Gillet L, Rosenberger G, Amon S, Collins BC, Aebersold R. Data-independent acquisition-based SWATH-MS for quantitative proteomics: a tutorial. Mol Syst Biol 2018; 14(8): e8126.
| Crossref | Google Scholar |
22 Escher C, Reiter L, MacLean B, Ossola R, Herzog F, Chilton J, et al. Using iRT, a normalized retention time for more targeted measurement of peptides. Proteomics 2012; 12(8): 1111-21.
| Crossref | Google Scholar |
23 Rosenberger G, Koh CC, Guo T, Röst HL, Kouvonen P, Collins BC, et al. A repository of assays to quantify 10,000 human proteins by SWATH-MS. Sci Data 2014; 1: 140031.
| Crossref | Google Scholar |
24 Zhang Y, Bilbao A, Bruderer T, Luban J, Strambio-De-Castillia C, Lisacek F, et al. The Use of Variable Q1 Isolation Windows Improves Selectivity in LC-SWATH-MS Acquisition. J Proteome Res 2015; 14(10): 4359-71.
| Crossref | Google Scholar |
25 Schilling B, Gibson BW, Hunter CL. Generation of High-Quality SWATH® Acquisition Data for Label-free Quantitative Proteomics Studies Using TripleTOF® Mass Spectrometers. Methods Mol Biol 2017; 1550: 223-33.
| Crossref | Google Scholar |
26 Selevsek N, Chang CY, Gillet LC, Navarro P, Bernhardt OM, Reiter L, et al. Reproducible and consistent quantification of the Saccharomyces cerevisiae proteome by SWATH-mass spectrometry. Mol Cell Proteomics 2015; 14(3): 739-49.
| Crossref | Google Scholar |
27 Krasny L, Bland P, Burns J, Lima NC, Harrison PT, Pacini L, et al. A mouse SWATH-mass spectrometry reference spectral library enables deconvolution of species-specific proteomic alterations in human tumour xenografts. Dis Model Mech 2020; 13(7): dmm044586.
| Crossref | Google Scholar |
28 Zhu T, Zhu Y, Xuan Y, Gao H, Cai X, Piersma SR, et al. DPHL: A DIA Pan-human Protein Mass Spectrometry Library for Robust Biomarker Discovery. Genomics Proteomics Bioinformatics 2020; 18(2): 104-19.
| Crossref | Google Scholar |
29 Barkovits K, Pacharra S, Pfeiffer K, Steinbach S, Eisenacher M, Marcus K, et al. Reproducibility, Specificity and Accuracy of Relative Quantification Using Spectral Library-based Data-independent Acquisition. Mol Cell Proteomics 2020; 19(1): 181-97.
| Crossref | Google Scholar |
30 Collins BC, Hunter CL, Liu Y, Schilling B, Rosenberger G, Bader SL, et al. Multi-laboratory assessment of reproducibility, qualitative and quantitative performance of SWATH-mass spectrometry. Nat Commun 2017; 8(1): 291.
| Crossref | Google Scholar |
31 Sun R, Hunter C, Chen C, Ge W, Morrice N, Liang S, et al. Accelerated Protein Biomarker Discovery from FFPE Tissue Samples Using Single-Shot, Short Gradient Microflow SWATH MS. J Proteome Res 2020; 19(7): 2732-41.
| Crossref | Google Scholar |
32 Addona TA, Abbatiello SE, Schilling B, Skates SJ, Mani DR, Bunk DM, et al. Multi-site assessment of the precision and reproducibility of multiple reaction monitoring-based measurements of proteins in plasma. Nat Biotechnol 2009; 27(7): 633-41.
| Crossref | Google Scholar |
33 Tabb DL, Vega-Montoto L, Rudnick PA, Variyath AM, Ham A-JL, Bunk DM, et al. Repeatability and reproducibility in proteomic identifications by liquid chromatography–tandem mass spectrometry. J Proteome Res 2010; 9(2): 761-76.
| Crossref | Google Scholar |
34 Rosenberger G, Bludau I, Schmitt U, Heusel M, Hunter CL, Liu Y, et al. Statistical control of peptide and protein error rates in large-scale targeted data-independent acquisition analyses. Nat Methods 2017; 14(9): 921-7.
| Crossref | Google Scholar |
35 Navarro P, Kuharev J, Gillet LC, Bernhardt OM, MacLean B, Röst HL, et al. A multicenter study benchmarks software tools for label-free proteome quantification. Nat Biotechnol 2016; 34(11): 1130-6.
| Crossref | Google Scholar |
36 Xuan Y, Bateman NW, Gallien S, Goetze S, Zhou Y, Navarro P, et al. Standardization and harmonization of distributed multi-center proteotype analysis supporting precision medicine studies. Nat Commun 2020; 11(1): 5248.
| Crossref | Google Scholar |
37 Poulos RC, Hains PG, Shah R, Lucas N, Xavier D, Manda SS, et al. Strategies to enable large-scale proteomics for reproducible research. Nat Commun 2020; 11(1): 3793.
| Crossref | Google Scholar |
38 Bekker-Jensen DB, Martínez-Val A, Steigerwald S, Rüther P, Fort KL, Arrey TN, et al. A Compact Quadrupole-Orbitrap Mass Spectrometer with FAIMS Interface Improves Proteome Coverage in Short LC Gradients. Mol Cell Proteomics 2020; 19(4): 716-29.
| Crossref | Google Scholar |
39 Tognetti M, Sklodowski K, Müller S, Kamber D, Muntel J, Bruderer R, et al. Biomarker Candidates for Tumors Identified from Deep-Profiled Plasma Stem Predominantly from the Low Abundant Area. J Proteome Res 2022; 21(7): 1718-35.
| Crossref | Google Scholar |
40 Reilly L, Peng L, Lara E, Ramos D, Fernandopulle M, Pantazis CB, et al. A fully automated FAIMS-DIA proteomic pipeline for high-throughput characterization of iPSC-derived neurons [Preprint]. bioRxiv 2021; 2021.11.24.469921.
| Crossref | Google Scholar |
41 Meier F, Brunner AD, Frank M, Ha A, Bludau I, Voytik E, et al. diaPASEF: parallel accumulation-serial fragmentation combined with data-independent acquisition. Nat Methods 2020; 17(12): 1229-36.
| Crossref | Google Scholar |
42 Mun DG, Vanderboom PM, Madugundu AK, Garapati K, Chavan S, Peterson JA, et al. DIA-Based Proteome Profiling of Nasopharyngeal Swabs from COVID-19 Patients. J Proteome Res 2021; 20(8): 4165-75.
| Crossref | Google Scholar |
43 Meier F, Park MA, Mann M. Trapped Ion Mobility Spectrometry and Parallel Accumulation-Serial Fragmentation in Proteomics. Mol Cell Proteomics 2021; 20: 100138.
| Crossref | Google Scholar |
44 Messner CB, Demichev V, Bloomfield N, Yu JSL, White M, Kreidl M, et al. Ultra-fast proteomics with Scanning SWATH. Nat Biotechnol 2021; 39(7): 846-54.
| Crossref | Google Scholar |
45 Skowronek P, Krohs F, Lubeck M, Wallmann G, Itang ECM, Koval P, et al. Synchro-PASEF Allows Precursor-Specific Fragment Ion Extraction and Interference Removal in Data-Independent Acquisition. Mol Cell Proteomics 2023; 22(2): 100489.
| Crossref | Google Scholar |
46 Wang Z, Mülleder M, Batruch I, Chelur A, Textoris-Taube K, Schwecke T, et al. High-throughput proteomics of nanogram-scale samples with Zeno SWATH MS. Elife 2022; 11: e83947.
| Crossref | Google Scholar |
47 Vowinckel J, Zelezniak A, Bruderer R, Mülleder M, Reiter L, Ralser M. Cost-effective generation of precise label-free quantitative proteomes in high-throughput by microLC and data-independent acquisition. Sci Rep 2018; 8(1): 4346.
| Crossref | Google Scholar |
48 Zelezniak A, Vowinckel J, Capuano F, Messner CB, Demichev V, Polowsky N, et al. Machine Learning Predicts the Yeast Metabolome from the Quantitative Proteome of Kinase Knockouts. Cell Syst 2018; 7(3): 269-83.e6.
| Crossref | Google Scholar |
49 Messner CB, Demichev V, Wendisch D, Michalick L, White M, Freiwald A, et al. Ultra-High-Throughput Clinical Proteomics Reveals Classifiers of COVID-19 Infection. Cell Syst 2020; 11(1): 11-24.e4.
| Crossref | Google Scholar |
50 Bruderer R, Muntel J, Müller S, Bernhardt OM, Gandhi T, Cominetti O, et al. Analysis of 1508 Plasma Samples by Capillary-Flow Data-Independent Acquisition Profiles Proteomics of Weight Loss and Maintenance. Mol Cell Proteomics 2019; 18(6): 1242-54.
| Crossref | Google Scholar |
51 Covey TR, Schneider BB, Javaheri H, LeBlanc JCY, Ivosev G, Corr JJ, et al. ESI, APCI, and MALDI a Comparison of the Central Analytical Figures of Merit: Sensitivity, Reproducibility, and Speed. In: Cole RB, editor. Electrospray and MALDI Mass Spectrometry. John Wiley & Sons, Ltd; 2010. pp. 441–90.
52 Shishkova E, Hebert AS, Coon JJ. Now, More Than Ever, Proteomics Needs Better Chromatography. Cell Syst 2016; 3(4): 321-4.
| Crossref | Google Scholar |
53 Bian Y, The M, Giansanti P, Mergner J, Zheng R, Wilhelm M, et al. Identification of 7000–9000 Proteins from Cell Lines and Tissues by Single-Shot Microflow LC–MS/MS. Anal Chem 2021; 93(25): 8687-92.
| Crossref | Google Scholar |
54 Bian Y, Zheng R, Bayer FP, Wong C, Chang YC, Meng C, et al. Robust, reproducible and quantitative analysis of thousands of proteomes by micro-flow LC–MS/MS. Nat Commun 2020; 11(1): 157.
| Crossref | Google Scholar |
55 Bian Y, Bayer FP, Chang YC, Meng C, Hoefer S, Deng N, et al. Robust Microflow LC-MS/MS for Proteome Analysis: 38000 Runs and Counting. Anal Chem 2021; 93(8): 3686-90.
| Crossref | Google Scholar |
56 Bian Y, Gao C, Kuster B. On the potential of micro-flow LC-MS/MS in proteomics. Expert Rev Proteomics 2022; 19(3): 153-64.
| Crossref | Google Scholar |
57 Messner CB, Demichev V, Wang Z, Hartl J, Kustatscher G, Mülleder M, et al. Mass spectrometry-based high-throughput proteomics and its role in biomedical studies and systems biology. Proteomics 2023; 23: e2200013.
| Crossref | Google Scholar |
58 Boys EL, Liu J, Robinson PJ, Reddel RR. Clinical applications of mass spectrometry-based proteomics in cancer: where are we? Proteomics 2023; 23: e2200238.
| Crossref | Google Scholar |
59 Demichev V, Messner CB, Vernardis SI, Lilley KS, Ralser M. DIA-NN: neural networks and interference correction enable deep proteome coverage in high throughput. Nat Methods 2020; 17(1): 41-4.
| Crossref | Google Scholar |
60 Kong AT, Leprevost FV, Avtonomov DM, Mellacheruvu D, Nesvizhskii AI. MSFragger: ultrafast and comprehensive peptide identification in mass spectrometry-based proteomics. Nat Methods 2017; 14(5): 513-20.
| Crossref | Google Scholar |
61 Gotti C, Roux-Dalvai F, Joly-Beauparlant C, Mangnier L, Leclercq M, Droit A. Extensive and Accurate Benchmarking of DIA Acquisition Methods and Software Tools Using a Complex Proteomic Standard. J Proteome Res 2021; 20(10): 4801-14.
| Crossref | Google Scholar |
62 Fröhlich K, Brombacher E, Fahrner M, Vogele D, Kook L, Pinter N, et al. Benchmarking of analysis strategies for data-independent acquisition proteomics using a large-scale dataset comprising inter-patient heterogeneity. Nat Commun 2022; 13(1): 2622.
| Crossref | Google Scholar |
63 Xu LL, Young A, Zhou A, Röst HL. Machine Learning in Mass Spectrometric Analysis of DIA Data. Proteomics 2020; 20(21–22): e1900352.
| Crossref | Google Scholar |
64 Bouwmeester R, Gabriels R, Van Den Bossche T, Martens L, Degroeve S. The Age of Data-Driven Proteomics: How Machine Learning Enables Novel Workflows. Proteomics 2020; 20(21–22): e1900351.
| Crossref | Google Scholar |
65 Meyer JG. Deep learning neural network tools for proteomics. Cell Rep Methods 2021; 1(2): 100003.
| Crossref | Google Scholar |
66 Sinitcyn P, Hamzeiy H, Salinas Soto F, Itzhak D, McCarthy F, Wichmann C, et al. MaxDIA enables library-based and library-free data-independent acquisition proteomics. Nat Biotechnol 2021; 39(12): 1563-73.
| Crossref | Google Scholar |
67 Bruderer R, Bernhardt OM, Gandhi T, Miladinović SM, Cheng LY, Messner S, et al. Extending the limits of quantitative proteome profiling with data-independent acquisition and application to acetaminophen-treated three-dimensional liver microtissues. Mol Cell Proteomics 2015; 14(5): 1400-10.
| Crossref | Google Scholar |
68 Gessulat S, Schmidt T, Zolg DP, Samaras P, Schnatbaum K, Zerweck J, et al. Prosit: proteome-wide prediction of peptide tandem mass spectra by deep learning. Nat Methods 2019; 16(6): 509-18.
| Crossref | Google Scholar |
69 Teo G, Kim S, Tsou CC, Collins B, Gingras AC, Nesvizhskii AI, et al. mapDIA: Preprocessing and statistical analysis of quantitative proteomics data from data independent acquisition mass spectrometry. J Proteomics 2015; 129: 108-20.
| Crossref | Google Scholar |
70 Plubell DL, Käll L, Webb-Robertson BJ, Bramer LM, Ives A, Kelleher NL, et al. Putting Humpty Dumpty Back Together Again: What Does Protein Quantification Mean in Bottom-Up Proteomics? J Proteome Res 2022; 21(4): 891-8.
| Crossref | Google Scholar |
71 Li KW, Gonzalez-Lozano MA, Koopmans F, Smit AB. Recent Developments in Data Independent Acquisition (DIA) Mass Spectrometry: Application of Quantitative Analysis of the Brain Proteome. Front Mol Neurosci 2020; 13: 564446.
| Crossref | Google Scholar |
72 Tshilenge K-T, Aguirre CG, Bons J, Basisty N, Song S, Rose J, et al. Proteomic Analysis of Huntington’s Disease Medium Spiny Neurons Identifies Alterations in Lipid Droplets. Mol Cell Proteomics 2023; 22(5): 100534.
| Crossref | Google Scholar |
73 Karayel O, Virreira Winter S, Padmanabhan S, Kuras YI, Vu DT, Tuncali I, et al. Proteome profiling of cerebrospinal fluid reveals biomarker candidates for Parkinson’s disease. Cell Rep Med 2022; 3(6): 100661.
| Crossref | Google Scholar |
74 Krasny L, Huang PH. Data-independent acquisition mass spectrometry (DIA-MS) for proteomic applications in oncology. Mol Omics 2021; 17(1): 29-42.
| Crossref | Google Scholar |
75 Bons J, Pan D, Shah S, Bai R, Chen-Tanyolac C, Wang X, et al. Data-independent acquisition and quantification of extracellular matrix from human lung in chronic inflammation-associated carcinomas. Proteomics 2022; 23: e2200021.
| Crossref | Google Scholar |
76 Gonçalves E, Poulos RC, Cai Z, Barthorpe S, Manda SS, Lucas N, et al. Pan-cancer proteomic map of 949 human cell lines. Cancer Cell 2022; 40(8): 835-49.e8.
| Crossref | Google Scholar |
77 Nakamura K, Hirayama-Kurogi M, Ito S, Kuno T, Yoneyama T, Obuchi W, et al. Large-scale multiplex absolute protein quantification of drug-metabolizing enzymes and transporters in human intestine, liver, and kidney microsomes by SWATH-MS: comparison with MRM/SRM and HR-MRM/PRM. Proteomics 2016; 16(15–16): 2106-17.
| Crossref | Google Scholar |
78 Li J, Smith LS, Zhu HJ. Data-independent acquisition (DIA): An emerging proteomics technology for analysis of drug-metabolizing enzymes and transporters. Drug Discov Today Technol 2021; 39: 49-56.
| Crossref | Google Scholar |
79 DeBoer J, Wojtkiewicz MS, Haverland N, Li Y, Harwood E, Leshen E, et al. Proteomic profiling of HIV-infected T-cells by SWATH mass spectrometry. Virology 2018; 516: 246-57.
| Crossref | Google Scholar |
80 Lozano C, Grenga L, Gallais F, Miotello G, Bellanger L, Armengaud J. Mass spectrometry detection of monkeypox virus: Comprehensive coverage for ranking the most responsive peptide markers. Proteomics 2023; 23(2): e2200253.
| Crossref | Google Scholar |
82 Doll S, Burlingame AL. Mass spectrometry-based detection and assignment of protein posttranslational modifications. ACS Chem Biol 2015; 10(1): 63-71.
| Crossref | Google Scholar |
83 Brandi J, Noberini R, Bonaldi T, Cecconi D. Advances in enrichment methods for mass spectrometry-based proteomics analysis of post-translational modifications. J Chromatogr A 2022; 1678: 463352.
| Crossref | Google Scholar |
84 Kitata RB, Choong WK, Tsai CF, Lin PY, Chen BS, Chang YC, et al. A data-independent acquisition-based global phosphoproteomics system enables deep profiling. Nat Commun 2021; 12(1): 2539.
| Crossref | Google Scholar |
85 Christensen DG, Meyer JG, Baumgartner JT, D’Souza AK, Nelson WC, Payne SH, et al. Identification of Novel Protein Lysine Acetyltransferases in Escherichia coli. mBio 2018; 9(5): e01905-18.
| Crossref | Google Scholar |
86 Bons J, Rose J, Zhang R, Burton JB, Carrico C, Verdin E, et al. In-depth analysis of the Sirtuin 5-regulated mouse brain malonylome and succinylome using library-free data-independent acquisitions. Proteomics 2023; 23(3–4): e2100371.
| Crossref | Google Scholar |
87 Fert-Bober J, Venkatraman V, Hunter CL, Liu R, Crowgey EL, Pandey R, et al. Mapping Citrullinated Sites in Multiple Organs of Mice Using Hypercitrullinated Library. J Proteome Res 2019; 18(5): 2270-8.
| Crossref | Google Scholar |
88 Stachowicz A, Sundararaman N, Venkatraman V, Van Eyk J, Fert-Bober J. pH/Acetonitrile-Gradient Reversed-Phase Fractionation of Enriched Hyper-Citrullinated Library in Combination with LC–MS/MS Analysis for Confident Identification of Citrullinated Peptides. Methods Mol Biol 2022; 2420: 107-26.
| Crossref | Google Scholar |
89 Fert-Bober J, Giles JT, Holewinski RJ, Kirk JA, Uhrigshardt H, Crowgey EL, et al. Citrullination of myofilament proteins in heart failure. Cardiovasc Res 2015; 108(2): 232-42.
| Crossref | Google Scholar |
90 Romero V, Fert-Bober J, Nigrovic PA, Darrah E, Haque UJ, Lee DM, et al. Immune-mediated pore-forming pathways induce cellular hypercitrullination and generate citrullinated autoantigens in rheumatoid arthritis. Sci Transl Med 2013; 5(209): 209ra150.
| Crossref | Google Scholar |
91 Stachowicz A, Pandey R, Sundararaman N, Venkatraman V, Van Eyk JE, Fert-Bober J. Protein arginine deiminase 2 (PAD2) modulates the polarization of THP-1 macrophages to the anti-inflammatory M2 phenotype. J Inflamm (Lond) 2022; 19(1): 20.
| Crossref | Google Scholar |
92 Jin Z, Fu Z, Yang J, Troncosco J, Everett AD, Van Eyk JE. Identification and characterization of citrulline-modified brain proteins by combining HCD and CID fragmentation. Proteomics 2013; 13(17): 2682-91.
| Crossref | Google Scholar |
94 Bekker-Jensen DB, Bernhardt OM, Hogrebe A, Martinez-Val A, Verbeke L, Gandhi T, et al. Rapid and site-specific deep phosphoproteome profiling by data-independent acquisition without the need for spectral libraries. Nat Commun 2020; 11(1): 787.
| Crossref | Google Scholar |
95 MacLean B, Tomazela DM, Shulman N, Chambers M, Finney GL, Frewen B, et al. Skyline: an open source document editor for creating and analyzing targeted proteomics experiments. Bioinformatics 2010; 26(7): 966-8.
| Crossref | Google Scholar |
96 Meyer JG, Mukkamalla S, Steen H, Nesvizhskii AI, Gibson BW, Schilling B. PIQED: automated identification and quantification of protein modifications from DIA-MS data. Nat Methods 2017; 14(7): 646-7.
| Crossref | Google Scholar |
97 Tsou CC, Avtonomov D, Larsen B, Tucholska M, Choi H, Gingras AC, et al. DIA-Umpire: comprehensive computational framework for data-independent acquisition proteomics. Nat Methods 2015; 12(3): 258-64.
| Crossref | Google Scholar |
98 Salovska B, Zhu H, Gandhi T, Frank M, Li W, Rosenberger G, et al. Isoform-resolved correlation analysis between mRNA abundance regulation and protein level degradation. Mol Syst Biol 2020; 16(3): e9170.
| Crossref | Google Scholar |
99 Foreman RE, George AL, Reimann F, Gribble FM, Kay RG. Peptidomics: A Review of Clinical Applications and Methodologies. J Proteome Res 2021; 20(8): 3782-97.
| Crossref | Google Scholar |
100 DeLaney K, Buchberger AR, Atkinson L, Gründer S, Mousley A, Li L. New techniques, applications and perspectives in neuropeptide research. J Exp Biol 2018; 221(Pt 3): jeb151167.
| Crossref | Google Scholar |
101 Lyapina I, Ivanov V, Fesenko I. Peptidome: Chaos or Inevitability. Int J Mol Sci 2021; 22(23): 13128.
| Crossref | Google Scholar |
102 Becker JP, Riemer AB. The Importance of Being Presented: Target Validation by Immunopeptidomics for Epitope-Specific Immunotherapies. Front Immunol 2022; 13: 883989.
| Crossref | Google Scholar |
103 Croft NP, Smith SA, Wong YC, Tan CT, Dudek NL, Flesch IEA, et al. Kinetics of antigen expression and epitope presentation during virus infection. PLoS Pathog 2013; 9(1): e1003129.
| Crossref | Google Scholar |
104 Yewdell JW, Antón LC, Bennink JR. Defective ribosomal products (DRiPs): a major source of antigenic peptides for MHC class I molecules? J Immunol 1996; 157(5): 1823-6.
| Crossref | Google Scholar |
105 Croft NP, de Verteuil DA, Smith SA, Wong YC, Schittenhelm RB, Tscharke DC, et al. Simultaneous Quantification of Viral Antigen Expression Kinetics Using Data-Independent (DIA) Mass Spectrometry. Mol Cell Proteomics 2015; 14(5): 1361-72.
| Crossref | Google Scholar |
106 Croft NP, Smith SA, Pickering J, Sidney J, Peters B, Faridi P, et al. Most viral peptides displayed by class I MHC on infected cells are immunogenic. Proc Natl Acad Sci U S A 2019; 116(8): 3112-7.
| Crossref | Google Scholar |
107 Bracewell DG, Francis R, Smales CM. The future of host cell protein (HCP) identification during process development and manufacturing linked to a risk‐based management for their control. Biotechnol Bioeng 2015; 112(9): 1727-37.
| Crossref | Google Scholar |
108 Husson G, Delangle A, O’Hara J, Cianferani S, Gervais A, Van Dorsselaer A, et al. Dual Data-Independent Acquisition Approach Combining Global HCP Profiling and Absolute Quantification of Key Impurities during Bioprocess Development. Anal Chem 2018; 90(2): 1241-7.
| Crossref | Google Scholar |
109 Pythoud N, Bons J, Mijola G, Beck A, Cianférani S, Carapito C. Optimized Sample Preparation and Data Processing of Data-Independent Acquisition Methods for the Robust Quantification of Trace-Level Host Cell Protein Impurities in Antibody Drug Products. J Proteome Res 2021; 20(1): 923-31.
| Crossref | Google Scholar |
110 Schubert OT, Mouritsen J, Ludwig C, Röst HL, Rosenberger G, Arthur PK, et al. The Mtb proteome library: a resource of assays to quantify the complete proteome of Mycobacterium tuberculosis. Cell Host Microbe 2013; 13(5): 602-12.
| Crossref | Google Scholar |
111 Kockmann T, Trachsel C, Panse C, Wahlander A, Selevsek N, Grossmann J, et al. Targeted proteomics coming of age – SRM, PRM and DIA performance evaluated from a core facility perspective. Proteomics 2016; 16(15–16): 2183-92.
| Crossref | Google Scholar |
112 Ejsing CS, Duchoslav E, Sampaio J, Simons K, Bonner R, Thiele C, et al. Automated identification and quantification of glycerophospholipid molecular species by multiple precursor ion scanning. Anal Chem 2006; 78(17): 6202-14.
| Crossref | Google Scholar |
113 Simons B, Kauhanen D, Sylvänne T, Tarasov K, Duchoslav E, Ekroos K. Shotgun Lipidomics by Sequential Precursor Ion Fragmentation on a Hybrid Quadrupole Time-of-Flight Mass Spectrometer. Metabolites 2012; 2(1): 195-213.
| Crossref | Google Scholar |
114 Hopfgartner G, Tonoli D, Varesio E. High-resolution mass spectrometry for integrated qualitative and quantitative analysis of pharmaceuticals in biological matrices. Anal Bioanal Chem 2012; 402(8): 2587-96.
| Crossref | Google Scholar |
115 Bonner R, Hopfgartner G. SWATH data independent acquisition mass spectrometry for metabolomics. Trends Analyt Chem 2019; 120: 115278.
| Crossref | Google Scholar |
116 Klont F, Jahn S, Grivet C, König S, Bonner R, Hopfgartner G. SWATH data independent acquisition mass spectrometry for screening of xenobiotics in biological fluids: Opportunities and challenges for data processing. Talanta 2020; 211: 120747.
| Crossref | Google Scholar |
117 Raetz M, Bonner R, Hopfgartner G. SWATH-MS for metabolomics and lipidomics: critical aspects of qualitative and quantitative analysis. Metabolomics 2020; 16(6): 71.
| Crossref | Google Scholar |
118 Martins-Marques T, Anjo SI, Pereira P, Manadas B, Girão H. Interacting Network of the Gap Junction (GJ) Protein Connexin43 (Cx43) is Modulated by Ischemia and Reperfusion in the Heart. Mol Cell Proteomics 2015; 14(11): 3040-55.
| Crossref | Google Scholar |
119 Mendes VM, Coelho M, Manadas B. Untargeted Metabolomics Relative Quantification by SWATH Mass Spectrometry Applied to Cerebrospinal Fluid. In: Santamaría E, Fernández-Irigoyen J, editors. Cerebrospinal Fluid (CSF) Proteomics: Methods and Protocols. New York, NY: Springer New York; 2019. pp. 321–36.
120 Krotulski AJ, Varnum SJ, Logan BK. Sample Mining and Data Mining: Combined Real-Time and Retrospective Approaches for the Identification of Emerging Novel Psychoactive Substances. J Forensic Sci 2020; 65(2): 550-62.
| Crossref | Google Scholar |
121 Furtwängler B, Üresin N, Motamedchaboki K, Huguet R, Lopez-Ferrer D, Zabrouskov V, et al. Real-Time Search-Assisted Acquisition on a Tribrid Mass Spectrometer Improves Coverage in Multiplexed Single-Cell Proteomics. Mol Cell Proteomics 2022; 21(4): 100219.
| Crossref | Google Scholar |
122 Schoof EM, Furtwängler B, Üresin N, Rapin N, Savickas S, Gentil C, et al. Quantitative single-cell proteomics as a tool to characterize cellular hierarchies. Nat Commun 2021; 12(1): 3341.
| Crossref | Google Scholar |
123 Fulcher JM, Markillie LM, Mitchell HD, Williams SM, Engbrecht KM, Moore RJ, et al. Parallel measurement of transcriptomes and proteomes from same single cells using nanodroplet splitting. bioRxiv 2022; 2022.05.17.492137.
| Crossref | Google Scholar |
124 Budnik B, Levy E, Harmange G, Slavov N. SCoPE-MS: mass spectrometry of single mammalian cells quantifies proteome heterogeneity during cell differentiation. Genome Biol 2018; 19(1): 161.
| Crossref | Google Scholar |
125 Petrosius V, Schoof EM. Recent advances in the field of single-cell proteomics. Transl Oncol 2023; 27: 101556.
| Crossref | Google Scholar |
126 Brunner AD, Thielert M, Vasilopoulou C, Ammar C, Coscia F, Mund A, et al. Ultra-high sensitivity mass spectrometry quantifies single-cell proteome changes upon perturbation. Mol Syst Biol 2022; 18(3): e10798.
| Crossref | Google Scholar |
127 Slavov N. Driving Single Cell Proteomics Forward with Innovation. J Proteome Res 2021; 20(11): 4915-8.
| Crossref | Google Scholar |
128 Derks J, Slavov N. Strategies for Increasing the Depth and Throughput of Protein Analysis by plexDIA. J Proteome Res 2023; 22: 697-705.
| Crossref | Google Scholar |
129 Slavov N. Framework for multiplicative scaling of single-cell proteomics. Nat Biotechnol 2022; 41: 23-4.
| Crossref | Google Scholar |
130 Szyrwiel L, Sinn L, Ralser M, Demichev V. Slice-PASEF: fragmenting all ions for maximum sensitivity in proteomics [Preprint]. bioRxiv 2022; 2022.10.31.514544.
| Crossref | Google Scholar |
131 Bache N, Geyer PE, Bekker-Jensen DB, Hoerning O, Falkenby L, Treit PV, et al. A Novel LC System Embeds Analytes in Pre-formed Gradients for Rapid, Ultra-robust Proteomics. Mol Cell Proteomics 2018; 17(11): 2284-96.
| Crossref | Google Scholar |
132 Chen R, Snyder M. Promise of personalized omics to precision medicine. Wiley Interdiscip Rev Syst Biol Med 2013; 5(1): 73-82.
| Crossref | Google Scholar |