Linking crown fire likelihood with post-fire spectral variability in Mediterranean fire-prone ecosystems
José Manuel Fernández-Guisuraga
A
B
C
D
E
Abstract
Fire behaviour assessments of past wildfire events have major implications for anticipating post-fire ecosystem responses and fuel treatments to mitigate extreme fire behaviour of subsequent wildfires.
This study evaluates for the first time the potential of remote sensing techniques to provide explicit estimates of fire type (surface fire, intermittent crown fire, and continuous crown fire) in Mediterranean ecosystems.
Random Forest classification was used to assess the capability of spectral indices and multiple endmember spectral mixture analysis (MESMA) image fractions (char, photosynthetic vegetation, non-photosynthetic vegetation) retrieved from Sentinel-2 data to predict fire type across four large wildfires
MESMA fraction images procured more accurate fire type estimates in broadleaf and conifer forests than spectral indices, without remarkable confusion among fire types. High crown fire likelihood in conifer and broadleaf forests was linked to a post-fire MESMA char fractional cover of about 0.8, providing a direct physical interpretation.
Intrinsic biophysical characteristics such as the fractional cover of char retrieved from sub-pixel techniques with physical basis are accurate to assess fire type given the direct physical interpretation.
MESMA may be leveraged by land managers to determine fire type across large areas, but further validation with field data is advised.
Keywords: canopy fraction burned, crown fire, fire type, MESMA, Sentinel-2, spectral indices, spectral variability, surface fire.
Introduction
Wildfires are a frequent disturbance in terrestrial ecosystems of Mediterranean-type climate regions around the world (Catry et al. 2013; Xofis et al. 2020). In the western Mediterranean Basin, the abandonment of traditional extensive agricultural practises and forestry uses in past decades. Together with proliferation of unmanaged forest plantations and fire suppression policies, this has led to an extensive and continuous accumulation of dense and flammable fuels (Moreira et al. 2011; Fernandes 2013). In addition, anthropogenic climate change has increased the occurrence of prolonged droughts and heat waves (Tripathy et al. 2023), leading to dryness conditions of fuel conducive to extreme fire behaviour and intense crown fires (Dimitrakopoulos et al. 2007; Pickering et al. 2023). These events may have unprecedented ecological consequences (Lasslop et al. 2019) and associated losses of human lives, infrastructure and properties (Mansoor et al. 2022).
Extreme wildfire events involving crown fires usually occur under severe fire weather conditions, resulting in hazardous and erratic fire behaviour (Mitsopoulos and Dimitrakopoulos 2007) over a wide variety of forest ecosystems throughout the western Mediterranean Basin (Fernández-Guisuraga et al. 2019, 2023a). A crown fire in forest spreads much faster (up to two to four times) than a surface fire burning in the same conditions (Fernandes et al. 2004; Perrakis et al. 2023), and its control efforts by direct attack are ineffective (Erni et al. 2020; Frost et al. 2022). These characteristics, together with increased fire intensity and frequent long-range spotting (Albini et al. 2012), may entail a serious threat to property and life in the wildland–urban interface (Fiorini et al. 2023). Long-lasting effects of high-intensity crown fires may include near total tree mortality (Woolley et al. 2012), abrupt shifts in species composition and structure leading to alternative stable states threatening ecosystem resilience (Allen et al. 2002; Knox and Clarke 2012) and biogeochemical cycles (Varner et al. 2021). Such fire impacts reflect the type and extent of heat transfer processes to which vegetation and soils were exposed (Fernández-Guisuraga et al. 2023b). Altogether, predicting the occurrence of crown fire behaviour is of utmost importance for planning pre-fire fuel treatments and fire suppression strategies (Scott and Reinhardt 2001).
Wildfires rarely behave only as crown fires due to changing fuel, topography or fire weather and thus, the spatial variability in the fire type entails major implications for the structure, successional dynamics and functioning of fire-prone ecosystems (Erni et al. 2020; Pérez-Izquierdo et al. 2021; Taylor et al. 2021). Therefore, it is not only important to assess the likelihood of crown fire behaviour, but also the spatial variability of the fire type (e.g. surface, crown) after wildfire extinction. Such knowledge would be of value, for example, in (1) testing the accuracy of fire behaviour models (Alexander and Cruz 2012), (2) providing insights on pre-fire fuel conditions conducive to extreme fire behaviour and severe ecological impacts (Dimitrakopoulos et al. 2007), (3) evaluating fuel treatment effectiveness (Cruz et al. 2004; Hu et al. 2019), and (4) estimating post-fire ecosystem responses including delayed tree mortality (Shearman et al. 2023). Indeed, several authors suggested that physically meaningful variables of actual fire effects that can be used as a proxy for fire type, such as crown fraction burned (CFB), can be more readily translated into management applications (Woolley et al. 2012; Hood et al. 2018), as opposed to integrative fire severity indices (e.g. the Composite Burn Index; Key and Benson 2005) (Morgan et al. 2014; Fernández-Guisuraga et al. 2023c).
Field methods enable assessing the type of fire based on both direct observation of fire behaviour (Cruz et al. 2003) and crown scorch and consumption (e.g. Pollet and Omi 2002; Morgan et al. 2014), but are labour-intensive and unable to thoroughly cover extensively burned landscapes. In this sense, the synoptic nature of remote sensing earth observations may be more appropriate for wall-to-wall estimation of fire type in post-fire landscapes (Fernández-Guisuraga et al. 2023a). Most remote sensing-based research to date have been focused on assessing fire severity drivers (e.g. Parks et al. 2018; Fernández-Guisuraga et al. 2021, 2023d; Fernández-García et al. 2022) or on developing accurate wall-to-wall fire severity estimates (e.g. De Santis and Chuvieco 2007; Miller et al. 2009; Quintano et al. 2013; Fernández-Guisuraga et al. 2023a). Conventionally, multispectral remote sensing data acquired from broadband sensors have been used to compute spectral indices, such as the differenced Normalised Burn Ratio (dNBR; Key 2006) or its relativised variants (Miller et al. 2009; Parks et al. 2014), as a proxy for the spectral signal of fire effects through empirical models. In this context, many previous studies (e.g. Roy et al. 2006; Fernández-Manso et al. 2016; Delcourt et al. 2021) and operational programs such as the Monitoring Trends in Burn Severity (MTBS; Picotte et al. 2020) in the United States or the Rapid Damage Assessment (RDA) module of the European Forest Fire Information System (EFFIS), have extensively used spectral indices computed from Landsat and Sentinel-2 imagery to obtain wall-to-wall fire severity estimates. Physical-based models applied to broadband or narrowband multispectral data, such as multiple endmember spectral mixture analysis (MESMA; Roberts et al. 1998), have also been considered in previous research (e.g. Quintano et al. 2013, 2023; Meng et al. 2017) to decompose sub-pixel reflectance signal and retrieve the fractional cover at pixel level of post-fire ground components (e.g. char or green vegetation) representative of wildfire ecological effects (i.e. fire severity) on vegetation and soils. This advanced pixel unmixing technique is considered to be more robust, scalable and generalisable than spectral indices due to its physical basis (Quintano et al. 2013).
Previous studies have seldom considered the potential of post-fire spectral variability to provide fire type estimates, particularly in Mediterranean fire-prone ecosystems (Mitri and Gitas 2006; Collins et al. 2018). These studies leveraged either pixel-based or object-based classification schemes and spectral indices computed from multispectral satellite data for mapping fire type or predicting fire severity categories as proxies for fire type. Other authors used a change detection framework for identifying fire type from airborne laser scanning (ALS) data in Sierra Nevada, California (Hu et al. 2019). As a bi-temporal change-detection framework, this method requires the acquisition of pre- and post-fire ALS datasets, which is a constraint because of the limited ALS data availability (Fernández-Guisuraga et al. 2022), contrary to multispectral satellite data.
To the best of our knowledge, physical-based and generalisable remote sensing approximations applied to broadband multispectral data, together with extensive field assessments, have not been used to detect crown fire in post-fire environments. Accordingly, we explore for the first time in the literature the potential of multispectral satellite data and advanced pixel unmixing models to provide meaningful and generalisable fire type estimates in Mediterranean ecosystems. Specifically, we examined how the post-fire spectral signal variability of MESMA fraction images computed from Sentinel-2 multispectral data reflects the likelihood of distinct fire types (surface, intermittent crown, continuous crown fire; Forestry Canada Fire Danger Group 1992) based on CFB, using spectral indices as a benchmark. See Scott and Reinhardt (2001) and National Wildfire Coordinating Group (2023) for fire type definitions. We selected four wildfires for this purpose that burned different types of broadleaf and conifer forests across the western part of the Mediterranean Basin. We hypothesise that MESMA fraction images, specifically the char fraction, would outperform spectral indices due to their higher physical sense and the intrinsic relationship between CFB and char signal in post-fire landscapes.
Material and methods
Study sites
We selected four wildfires that affected forests, shrublands and grasslands in the western Mediterranean Basin (north-western and central Spain) under extreme fire weather conditions in the summer seasons between 2017 and 2022 (Fig. 1), with unprecedented prolonged droughts and heat waves in the months prior to the wildfires. The sites encompass wide environmental gradients are in Table 1. We chose as target conifer and broadleaf forests within the wildfires. Maritime pine (Pinus pinaster Ait.) dominated conifer forests in lowlands, whereas Scots pine (Pinus sylvestris L.) dominated in highlands. Broadleaf forests were mainly dominated by Pyrenean oak (Quercus pyrenaica Willd.) and holm oak (Quercus ilex L.). Wildfires were selected on the basis of available canopy fraction burned (CFB) data acquired by the same observers.
Wildfire | Sierra de Cabrera | Villapadierna | Navalacruz | Sierra de la Culebra | |
---|---|---|---|---|---|
Location | NW Spain | NW Spain | C Spain | W Spain | |
Wildfire size (ha) | 9940 | 82 | 24,444 | 28,046 | |
Wildfire date | 21 August 2017 | 22 August 2019 | 14 August 2021 | 11 June 2022 | |
Elevation range (m) | 836–1938 | 922–1027 | 939–2157 | 747–1205 | |
Slope range (%) | 0–149 | 6–27 | 0–649 | 0–155 | |
Average annual precipitation (mm) | 850 | 761 | 758 | 750 | |
Average annual temperature (°C) | 9.0 | 10.7 | 9.6 | 11Confusion matrix and accuracy metrics | |
Plant communities (# plots) | Qp (21) | Qp (15) Pp (5) Ps (5) | Qi (8) Ps (18) | Qp (9) Qi (14) Ps (21) Pp (13) | |
Pre-fire Sentinel-2 image date | 13 August 2017 | 15 August 2019 | 9 August 2021 | 26 May 2022 | |
Post-fire Sentinel-2 image date | 2 September 2017 | 30 August 2019 | 8 ctober 2021 | 15 July 2022 |
Location of Sierra de Cabrera (a), Villapadierna (b), Navalacruz (c), and Sierra de la Culebra (d) wildfires in the western part of the Mediterranean Basin (north-western and central Spain). A Sentinel-2 false colour composite (R = band 12; G = band 8A; B = band 4) is displayed at the background.
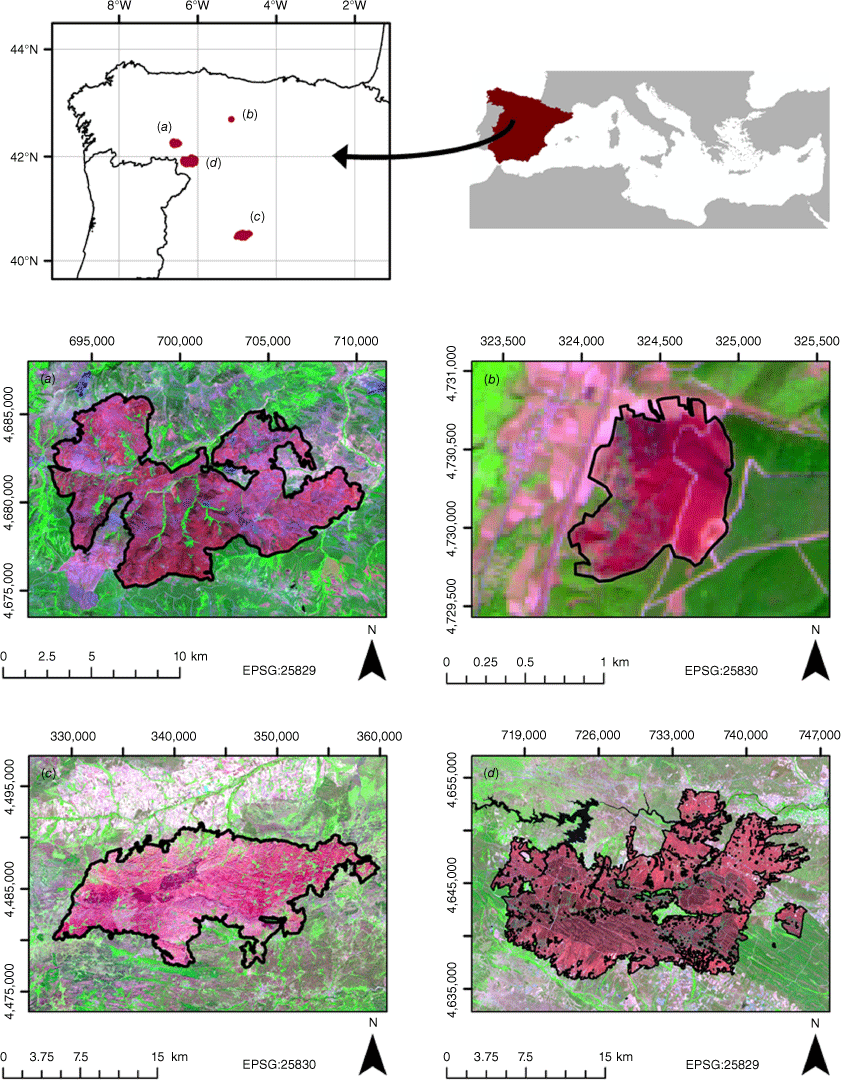
Field data
Plots of 20 m × 20 m were randomly established in burned areas (ensuring a minimum distance between plots of 100 m) within 2 months after fire, being homogeneous in terms of the dominant species (maritime pine, Scots pine, holm oak, and Pyrenean oak) (Table 1). The plots were located using a high-accuracy GPS receiver (RMSEX,Y < 1 m). In each plot (n = 129; Table 1), we measured the CFB as the proportion of burned crown (charred or consumed foliage, twigs and branches; Varner et al. 2021) with respect to all tree crowns in the plot (Cruz and Alexander 2017), which is indicative of the most probable fire type for crowning-susceptible vegetation (Cruz et al. 2003). To validate a measurement, the consensus of two observers was required (Fernández-Guisuraga et al. 2023a). Fire type in each field plot was classified according to three CFB thresholds: (1) surface fire (CFB < 0.1); (2) intermittent crown fire (0.1 ≤ CFB ≤ 0.89); and (3) continuous crown fire (CFB > 0.89) (Forestry Canada Fire Danger Group 1992).
Remote sensing data
Multispectral data acquired from the multispectral instrument (MSI) onboard Sentinel-2 satellite of the (European Space Agency; ESA) Copernicus program were used to compute spectral indices and MESMA fraction images. Sentinel-2 provides multiresolution data (10, 20, 60 m) across visible (VIS; 4 bands), red edge (RE; 3 bands), near infrared (NIR; 3 bands) and short-wave infrared (SWIR; 3 bands) regions. Pre-fire and post-fire Sentinel-2 Level-2A scenes (orthorectified, surface reflectance product) covering the wildfires were downloaded from the Copernicus Open Access Hub (https://scihub.copernicus.eu) for dates as close as possible to the start and end date of the wildfires based on the availability of cloud-free imagery (Table 1). The 10-m bands were downsampled to a spatial resolution of 20 m through nearest neighbour interpolation. The bands at 60 m were discarded for the MESMA procedure because of their susceptibility to atmospheric effects and thus the absence of interpretable surface reflectance data (Jia et al. 2016).
Remote sensing data processing
We calculated the most commonly used bi-temporal, absolute spectral index in the literature; i.e. the dNBR (Eqns 1 and 2), from using bands 8a (NIR) and 12 (SWIR) of pre- and post-fire Sentinel-2 scenes. We also calculated a relativised spectral index; i.e. the Relativised Burn Ratio (RBR; Parks et al. 2014) (Eqn 3), for the higher potential it can offer in burned landscapes with heterogeneous vegetation composition, and in areas with sparse vegetation (Miller and Thode 2007). We discarded the commonly-used Relative dNBR index (RdNBR; Miller et al. 2009) because of potentially anomalous values as a consequence of the numerical instability of the index when pre-fire NBR displays zero or negative values (Parks et al. 2014).
Sentinel-2 dNBR and RBR values were extracted for each plot of 20 m × 20 m by averaging the values of a systematically sampled grid of 20 points with 2-m spacing within each plot in order to account for the mismatch between the Sentinel-2 grid and the plot extent (Fernández-Guisuraga et al. 2022).
The MESMA algorithm was implemented using VIPER tools ver. 2.1 software (Roberts et al. 2019). The initial phase of the procedure involved the identification of candidate endmember (i.e. basic ground components) spectra to build a spectral library and execute the spectral unmixing process on the post-fire Sentinel-2 scenes. This phase is critical, as the precise selection of endmember spectral signatures has a high impact on the accuracy of the MESMA algorithm output (Tompkins et al. 1997).
To build the spectral library, we first extracted candidate endmembers from Sentinel-2 scenes, including several land cover classes representative of the study site, and second, we identified the optimum endmembers to build the definitive spectral library. We chose photosynthetic vegetation (PV), non-photosynthetic vegetation and soil (NPVS), and char as endmembers to unmix Sentinel-2 scenes following previous post-fire assessments (e.g. Quintano et al. 2013, 2023; Fernández-Manso et al. 2019). The candidate endmembers were manually delineated inside regions of interest for each class (Quintano et al. 2023). Polygon delineation outside the fire scar was assisted using as reference orthophotos from Spanish Aerial Ortho-photography National Plan (PNOA) at a spatial resolution of 50 cm, the Spanish Forest Map, and true (4-3-2) and false (12-8A-4) colour composites of post-fire Sentinel-2 scenes. Spectral signatures were checked to verify that they had the expected shape for the ground covers of interest. Char spectral signatures were extracted from homogeneous polygons within the fire scar using Sentinel-2 colour composites and reviewing the expected char spectral signature (Fernández-Manso et al. 2019). We then built the definitive spectral library by a semi-automatic process. We first implemented the Iterative Endmember Selection (IES; Schaaf et al. 2011; Roth et al. 2012) algorithm to identify the most relevant endmembers of the different land cover classes of interest based on the maximisation of the Kappa index. Following this, we manually included endmembers not selected by the automatic IES algorithm, but where (1) Minimum Average Spectral Angle (MASA; Dennison et al. 2004), (2) Count-based Endmember Selection Index (CoB; Roberts et al. 2003), and (3) Endmember Average RMSE (EAR; Dennison and Roberts 2003) indices reflected a high endmember representativeness (Quintano et al. 2013). An additional endmember per class was included in the definitive spectral library if it jointly exhibits the lowest MASA index value, the highest CoB index value, and the lowest EAR index value.
Once the definitive spectral library was established, the endmembers were hierarchically organised using a multilevel fusion procedure and thus at different levels of complexity (Roberts et al. 2003). The process of spectral unmixing executed on Sentinel-2 post-fire scenes was then iterative because it is necessary to adjust the maximum number of endmembers considered in each model, and their optimum spectral signatures, until the imposed restrictions (i.e. fraction values between −0.10 and 1.10, shade fraction values between 0.00 and 1.00, maximum allowed RMSE equal to 0.025 and maximum 5% of unclassified pixels) were fulfilled, following previous research (Quintano et al. 2013, 2023; Fernández-Manso et al. 2019). Finally, the fraction images (i.e. char, PV and NPVS) were shade-normalised to remove the shade endmember influence (Roberts et al. 2019). For more detailed information on the whole MESMA procedure see Quintano et al. (2023).
Fractional cover by shade-normalised image fractions was then extracted for each 20 m × 20 m plot following the same procedure as for spectral indices.
Data analyses
First, we assessed the differences in CFB, spectral index values (i.e. dNBR and RBR), and fractional cover by MESMA image fractions (i.e. char, PV and NPVS) between conifer and broadleaf forest ecosystems using Mann–Whitney U tests after evidencing non-compliance with parametric test assumptions. The statistical significance of the differences was determined at the 0.05 level. The Kruskal–Wallis test was used to assess statistical differences in spectral indices and image fractions between fire types (surface, intermittent, crown), followed by a pairwise Wilcoxon test.
Second, a Random Forest (RF; Breiman 2001) classification algorithm was used to assess the capability of spectral indices (univariate model) and MESMA image fractions (multivariate model) to predict fire type and link crown fire likelihood with post-fire spectral variability in conifer and broadleaf forests separately. RF classification algorithm was selected due to the absence of assumptions about the distribution of the response variable and its capacity to unravel complex, non-linear relationships between the dependent variable and predictors (Rodriguez-Galiano et al. 2012; Wang et al. 2019). The ntree RF hyperparameter was set to 2000 for ensuring prediction stability (Probst and Boulesteix 2018). The optimum value of the mtry RF hyperparameter was found through tuning experiments consisting of repeated 10-fold cross-validation. RF classification performance was assessed through the confusion matrix averaged across 10-fold cross validation resamples. The following accuracy metrics were computed: overall accuracy (OA; %), Kappa index, user’s accuracy (UA; %), and producer’s accuracy (PA; %) for each fire type category. Variable importance in multivariate RF models (i.e. those calibrated with MESMA fraction images) was calculated using the mean decrease in accuracy (MDA; %) metric. We also computed partial dependence plots depicting continuous crown fire likelihood in a centred logit scale. Finally, we fitted a RF model using global data (conifer and broadleaf forests pooled together) to test the generalisation ability of MESMA image fractions and the best-performing spectral index. RF model objects were used to generate wall-to-wall predictions (i.e. fire type maps) for the broadleaf and conifer forests within the Sierra de la Culebra wildfire scar (Fig. 1), selected because it is one of the largest wildfires ever recorded in Spain and has a large area occupied by conifer and broadleaf forests. The Spanish Forest Map derived from the fourth Spanish National Forest Inventory was used to delimit the broadleaf and conifer stands.
All analyses were conducted in R (R Core Team 2021).
Results
The CFB of conifer forests was significantly larger than that of broadleaf forests (Mann–Whitney P-value < 0.01) in the study sites (Fig. 2). Char fractional cover estimated from MESMA was significantly higher in conifer than in broadleaf forests (P-value < 0.01), with NPVS fraction exhibiting the opposite behaviour (Fig. 2). Both spectral indices (i.e. dNBR and RBR) and the GV MESMA fraction did not significantly differ (P-value > 0.05) between forest types (Fig. 2).
Boxplots depicting the relationship of canopy fraction burned (CFB), spectral indices (dNBR and RBR) and MESMA image fractions (char; GV, green vegetation; NPVS, non-photosynthetic vegetation and soil) with ecosystem type (BF, broadleaf forests; Cf, conifer forests). Mann–Whitney U test results are shown in the upper part of the plots.
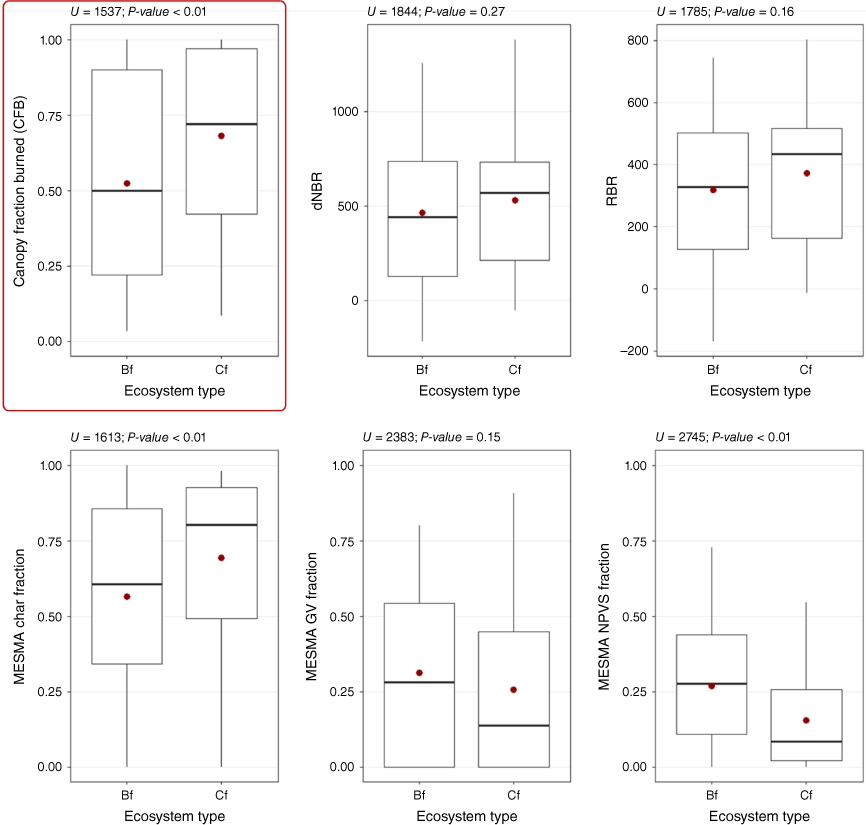
All spectral indices and MESMA fractions differed significantly between fire types (χ > 46.96; P-value < 0.001), with a significant increase from surface to crown fires in the case of spectral indices and the char MESMA fraction, and the opposite behaviour in the case of the GV and NPVS fractions (Fig. 3). The strongest relationship with fire type corresponded to the char MESMA fraction.
Boxplots depicting the relationship of spectral indices (dNBR and RBR) and MESMA image fractions (char; GV, green vegetation; NPVS, non-photosynthetic vegetation and soil) with fire type. We show Kruskal–Wallis test results in the upper part of the plots. Lowercase red letters denote significant differences between fire types at P = 0.05.
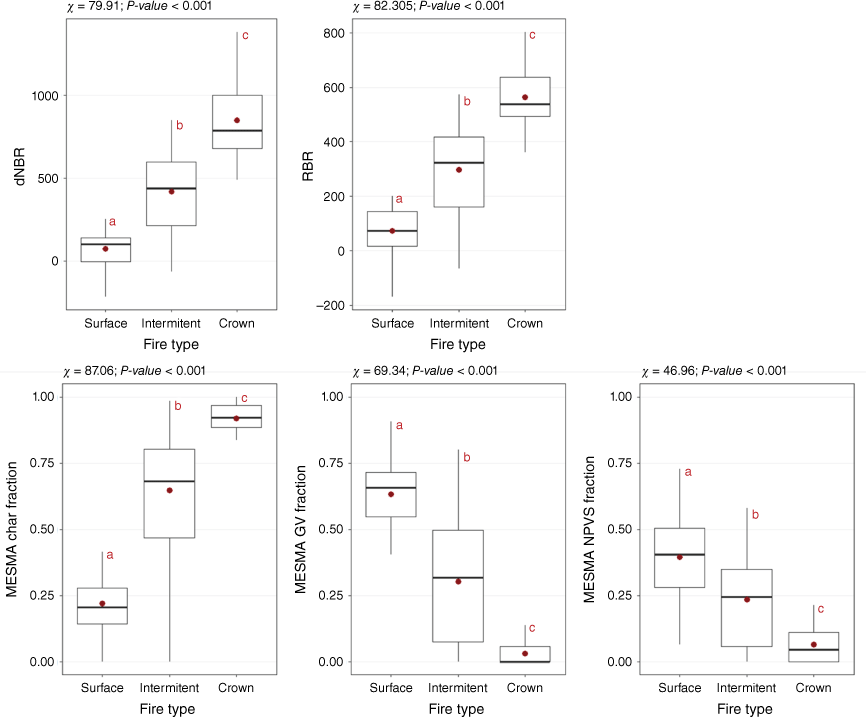
In broadleaf forests, the highest performance in fire type classification was obtained by the multivariate RF model calibrated from MESMA fractions (OA = 82.09% and Kappa index = 0.71). The Producer’s and User’s accuracy of the model were balanced and no remarkable confusion was observed among all classes (Table 2). Classification accuracy was notably lower for the spectral indices (OA < 71.96% and Kappa index < 0.55), particularly for the dNBR (Table 2). The greatest confusion was observed for both indices between surface and intermittent crown fires, and between intermittent and continuous crown fires. Continuous crowning was never misclassified as a surface fire for all remote sensing products (Table 2).
Fire type | Ground truth | ||||
---|---|---|---|---|---|
Surface | Intermittent | Crown | |||
Predicted (dNBR) | Surface | 9 | 6 | 0 | |
Intermittent | 7 | 22 | 6 | ||
Crown | 0 | 5 | 12 | ||
PA (%) | 56.25 | 66.67 | 66.67 | ||
UA (%) | 60.00 | 62.86 | 70.59 | ||
OA (%) | Kappa | ||||
64.18 | 0.42 | ||||
Predicted (RBR) | Surface | 10 | 5 | 0 | |
Intermittent | 6 | 24 | 5 | ||
Crown | 0 | 4 | 13 | ||
PA (%) | 62.50 | 76.67 | 72.22 | ||
UA (%) | 71.43 | 67.65 | 81.25 | ||
OA (%) | Kappa | ||||
71.86 | 0.55 | ||||
Predicted (MESMA fractions) | Surface | 12 | 2 | 0 | |
Intermittent | 4 | 27 | 2 | ||
Crown | 0 | 4 | 16 | ||
PA (%) | 75.00 | 81.82 | 88.89 | ||
UA (%) | 85.71 | 81.82 | 80.00 | ||
OA (%) | Kappa | ||||
82.09 | 0.71 |
In conifer forests, the overall accuracy provided by spectral indices and MESMA fractions in the RF fire type classification, as well as the Producer’s and User’s accuracy of the models (Table 3), followed the same pattern as in broadleaf forests (MESMA accuracy > RBR > dNBR). The very low confusion rate in the MESMA-based classification is worth noting (Table 3).
Fire type | Ground truth | ||||
---|---|---|---|---|---|
Surface | Intermittent | Crown | |||
Predicted (dNBR) | Surface | 5 | 5 | 0 | |
Intermittent | 3 | 21 | 8 | ||
Crown | 0 | 3 | 17 | ||
PA (%) | 62.50 | 72.41 | 68.00 | ||
UA (%) | 50.00 | 65.63 | 85.00 | ||
OA (%) | Kappa | ||||
69.36 | 0.50 | ||||
Predicted (RBR) | Surface | 6 | 5 | 0 | |
Intermittent | 2 | 22 | 6 | ||
Crown | 0 | 2 | 19 | ||
PA (%) | 75.00 | 75.86 | 76.00 | ||
UA (%) | 54.55 | 73.33 | 90.47 | ||
OA (%) | Kappa | ||||
75.81 | 0.61 | ||||
Predicted (MESMA fractions) | Surface | 6 | 0 | 0 | |
Intermittent | 2 | 25 | 3 | ||
Crown | 0 | 4 | 22 | ||
PA (%) | 75.00 | 86.21 | 88.00 | ||
UA (%) | 100.00 | 83.33 | 84.62 | ||
OA (%) | Kappa | ||||
85.48 | 0.76 |
All remote sensing products performed better in conifer (OA = 76.88% ± 8.11% and Kappa = 0.62 ± 0.13) than in broadleaf (OA = 72.71% ± 8.99% and Kappa = 0.56 ± 0.15) forests.
The likelihood of continuous crowning relative to the spectral variability of burned areas captured by the MESMA fractions was very similar in conifer and broadleaf forests (Fig. 4). Occurrence of continuous crowning is linked in both ecosystems to post-fire char fractions greater than 0.5, the maximum likelihood being reached when char fraction is equal to 0.8. When GV and NPVS fractions are greater than 0.25–0.30, the likelihood of continuous crowning is very low. The MESMA char fraction was the most important variable to explain fire-type likelihood in broadleaf and conifer forests (Fig. 4). Consistency of continuous crowning likelihood between spectral indices and ecosystem types was lower than in the case of MESMA fractions (Fig. 4). dNBR values above 500 seem to be associated with maximum crowning probability in conifer forests, while in broadleaf forests the probability continues to increase progressively above such dNBR threshold. The same behaviour in both forest types was observed with RBR values above 300.
Partial dependence plots depicting the relationship in broadleaf and conifer forests between crown fire likelihood and the variability of MESMA image fractions and spectral indices in the Random Forests (RF) classification algorithm. The red line is a LOESS smooth curve. Mean decrease in accuracy (MDA;%) metric is shown for each MESMA image fraction (multivariate RF models).
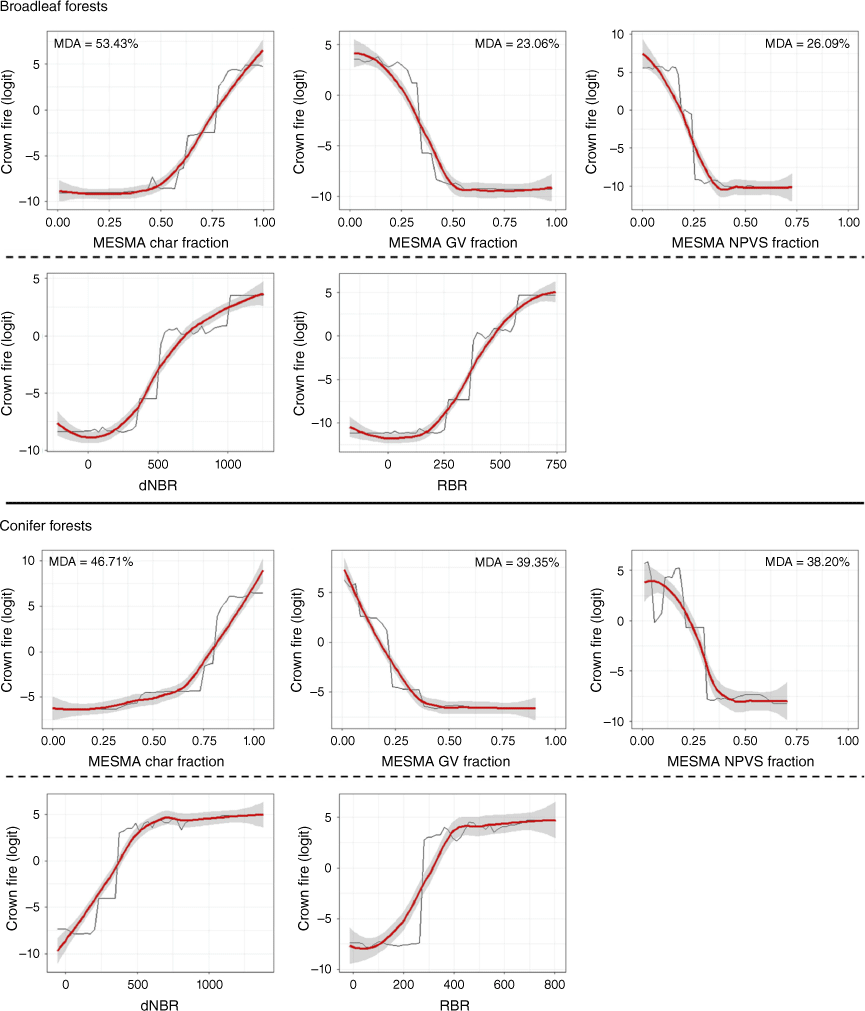
When pooling data from conifer and broadleaf forests, the RBR index shows poor generalisation in fire type estimates (OA = 62.88% and Kappa index = 0.42) (Table 4) when comparing results with RF classification models by single ecosystems (OA = 73.84% ± 2.79% and Kappa = 0.58 ± 0.04) (Tables 2 and 3), with even greater underestimation of continuous crowning and higher confusion between surface and intermittent crown fires. Conversely, the performance loss of the global RF model was negligible (OA = 82.95% and Kappa index = 0.73 vs OA = 83.79% ± 2.40% and Kappa = 0.74 ± 0.04) when calibrated with MESMA fractions (Table 4).
Fire type | Ground truth | ||||
---|---|---|---|---|---|
Surface | Intermittent | Crown | |||
Predicted (RBR) | Surface | 13 | 13 | 0 | |
Intermittent | 8 | 40 | 15 | ||
Crown | 3 | 9 | 28 | ||
PA (%) | 55.56 | 64.52 | 65.12 | ||
UA (%) | 53.57 | 62.50 | 70.00 | ||
OA (%) | Kappa | ||||
62.88 | 0.42 | ||||
Predicted (MESMA fractions) | Surface | 18 | 3 | 0 | |
Intermittent | 6 | 51 | 5 | ||
Crown | 0 | 8 | 38 | ||
PA (%) | 75.00 | 82.26 | 88.37 | ||
UA (%) | 85.71 | 82.26 | 82.61 | ||
OA (%) | Kappa | ||||
82.95 | 0.73 |
These results are consistent with wall-to-wall fire type maps computed from RF model objects at landscape scale in broadleaf and conifer forests within the Sierra de la Culebra wildfire (Fig. 5). The area of continuous crowning is much smaller in RBR than in MESMA estimates due to the high confusion between intermittent and continuous crown fire types in the former product. Wall-to-wall estimates of the RF models calibrated in conifer and broadleaf forests separately are quite consistent with those of the RF model calibrated from global data in the case of MESMA fractions. In contrast, wall-to-wall estimates of RBR-based models are much less consistent between the two model calibration strategies.
Location of the field plots and wall-to-wall fire type predictions in broadleaf and conifer forests within the Sierra de la Culebra wildfire (RF model in conifer and broadleaf forests separately). We also present the map for the RF model calibrated from global data (conifer and broadleaf forests pooled together).
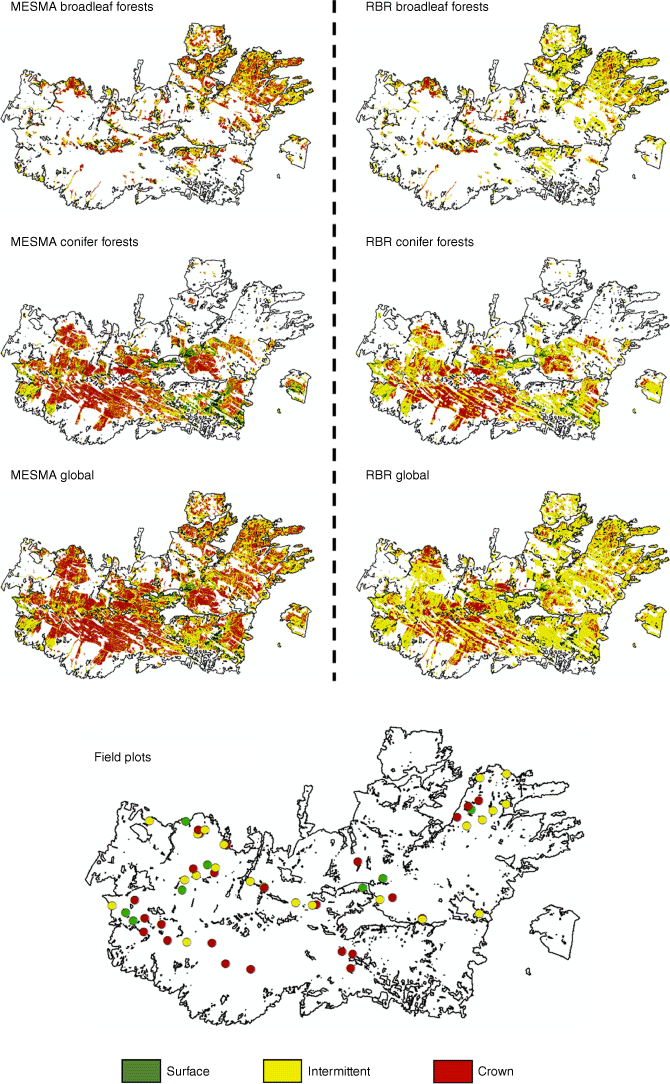
Discussion
Despite the extensive literature on fire severity assessments through remote sensing techniques using integrative field measurements (e.g. Fernández-Guisuraga et al. 2023a; Miller et al. 2023) or physically meaningful variables such as crown scorch height or crown consumption (e.g. Lentile et al. 2009; Lydersen et al. 2016; Arkin et al. 2023), the potential of physically-based and generalisable remote sensing approximations to provide wall-to-wall fire type estimates representative of wildfire behaviour is examined for the first time in this study. Our results showed the importance of generalisable remote sensing techniques to procure accurate crown fire likelihood estimates that align with post-fire land management needs in Mediterranean burned landscapes (Keeley 2009). This is particularly relevant because, although fire severity is closely related to fire behaviour (Finney 2005), the latter is what really determines the effectiveness of fire suppression efforts and size-dependent fire impacts (Fernandes et al. 2010).
Typically, remote sensing techniques with a physical basis, including MESMA, perform better than empirical methods based on spectral indices for retrieving biophysical properties of burned landscapes (e.g. De Santis and Chuvieco 2007; Fernández-Guisuraga et al. 2021, 2023a; Quintano et al. 2023). Accordingly, MESMA image fractions also procured here a remarkably higher performance than spectral indices in the fire type RF classifier, particularly when pooling field data from several ecosystems. Mitri and Gitas (2006) used an object-based classification relying on spectral indices computed from a post-fire IKONOS scene, together with object contextual information, for mapping fire type within a single, small wildfire that affected a Mediterranean pine forest in Greece. The authors reported a classification accuracy (overall accuracy and Kappa index) comparable to that obtained here with MESMA fractions. However, they did not consider intermittent crown fire in the fire type classification, which may be responsible for high patchiness of fire effects over the landscape (Scott and Reinhardt 2001) and thus for mixed spectral responses. In contrast to our study, only one ecosystem type within a single wildfire was examined, and it has been previously reported that spectral indices (1) suffer from generalisation issues between different vegetation types and environmental conditions due to the lack of physics in the retrieval of fire effects (e.g. De Santis and Chuvieco 2007; Lentile et al. 2009; Fernández-Guisuraga et al. 2023a), and (2) have suboptimal sensitivity to complex spectral responses (e.g. Roy et al. 2006; Mallinis et al. 2018).
Despite this, the higher accuracy of MESMA image fractions versus spectral indices used as benchmark within the same experimental design for estimating fire-type spatial variability may be related to the sound physical meaning of sub-pixel image analysis techniques in post-fire environments (Quintano et al. 2013). In this context, capturing the variability in the fire type spectral signal can be a subpixel matter when using moderate spatial resolution multispectral imagery (Quintano et al. 2013; Fernández-Manso et al. 2019), such as Sentinel-2. Specifically, the high spatial variability in surface and canopy fuels expected in Mediterranean fire-prone ecosystems (Fernández-Guisuraga et al. 2023a), together with fine-scale variations in terrain and fire weather, may result in fire behaviour alternation between different fire types at small spatial scales (Scott and Reinhardt 2001). MESMA image fractions also represent intrinsic biophysical characteristics of burned landscapes (i.e. char or photosynthetic vegetation), not only a secondary proxy for these constituents. Indeed, the SWIR region of broadband remote sensing data involved in dNBR and thus RBR calculation is not as sensitive to the spectral variability of char, ash and soil, nor to their complex mixture in post-fire scenarios as traditionally assumed (see Lentile et al. 2009), unlike the NIR region to vegetation vigour or amount (Hudak et al. 2007). Several authors also stated that spectral indices such as dNBR were originally conceived to map burned areas, and not to estimate the variability in their biophysical properties (Roy et al. 2006).
The ability of MESMA to resolve complex and mixed spectral responses of scorched and burned canopies due to the use of full available spectra (Quintano et al. 2023), rather than a limited number of bands and thus spectral information as in the case of spectral indices (Mallinis et al. 2018), may have prevented the high confusion between fire types and the underestimation of continuous crowning. This effect, evident in dNBR and RBR estimates and highly dependent on vegetation type (Lentile et al. 2009), was probably also attributable to the SWIR reflectance saturation and steady NIR reflectance decrease at high char and ash cover (Soverel et al. 2010). Indeed, high crown fire likelihood in conifer and broadleaf forests is linked to a post-fire MESMA char fractional cover of about 0.8 (Fig. 4), close to the CFB defining a continuous crown fire (CFB > 0.89). Consistent with previous fire severity research (Tane et al. 2018; Fernández-Manso et al. 2019), the char fraction was the most important ground constituent to retrieve fire type likelihood in broadleaf and conifer forests.
The better performance of MESMA-based models (and spectral indices) in conifer than in broadleaf forests may be associated with the typical lower canopy closure in Mediterranean conifer forests than in broadleaf forests (e.g. García et al. 2010; Sheffer et al. 2015), which may cause the char spectral signal from the lower canopy strata to be better captured by the sensor. This behaviour has been previously reported by Gibson et al. (2020) in Mediterranean, semi-arid and subtropical burned areas of eastern Australia. The authors evidenced that high canopy closure may result in the underestimation of the fire severity spectral signal sensed by moderate spatial resolution satellites. Conifer forests were more prone to elevated CFB and thus to high-intensity crown fires than broadleaf forests according to fire hazard generic expectations and previous reports of crowning potential in the Mediterranean Basin (Fernandes 2009; Fernandes et al. 2010; Fernández-Guisuraga et al. 2023c), and in other biomes elsewhere (e.g. Scott and Reinhardt 2001; Epting and Verbyla 2005). This may be linked to higher accumulation of flammable litter and fuel loading of fine-fuel rich species in the understory of unmanaged Mediterranean conifer forests than in those dominated by broadleaf species, coupled with increased vertical fuel continuity and ladder fuels (Safford et al. 2012; Fernández-Guisuraga et al. 2021).
MESMA fraction images provided not only more accurate fire type estimates than spectral indices in broadleaf and conifer forests, but also featured greater generalisation ability. This could be related to the analogy between the variation in crown scorch and consumption estimated through MESMA fraction images and the fire type definition (CFB) used in the field. In addition, the variability of the background reflectance signal corresponding to bare soil can affect the discrimination of ecological fire effects and generalisation ability when relying on spectral indices (Meng et al. 2017; Fernández-Guisuraga et al. 2023a). Conversely, the endmember collection of all representative ground constituents at each site to build the definitive spectral library in the MESMA algorithm could be accounted for the minimisation of the background influence in the estimation of fire effects (Quintano et al. 2023).
Overall, the results of this study could be leveraged by land managers to reliably infer tree damage, mortality and ecosystem responses in Mediterranean post-fire landscapes, and develop accordingly appropriate post-fire management plans and restoration strategies. In particular, the potential implementation of physical-based algorithms such as advanced spectral mixture models in consolidated geospatial processing platforms in the cloud; e.g. Google Earth Engine (GEE; Gorelick et al. 2017), could emerge as a valuable resource for minimising data acquisition and processing efforts in wildfire management (Costa-Saura et al. 2022). Moreover, future research could leverage the potential of recently-available spectroscopic spaceborne data together with advanced image analysis techniques like MESMA (Quintano et al. 2023) to determine whether wall-to-wall fire behaviour estimates reported here can be further refined. Recently, the use of unmanned aerial vehicles (UAVs) has enabled accurate predictions of physically meaningful variables as proxies for fire effects at the level of individual trees (Moran et al. 2022; Arkin et al. 2023), and can therefore be a reliable tool in future research to characterise the fire type particularly when fire effects vary greatly at fine spatial scales.
Conclusions
We examined for the first time the potential of broadband satellite data to provide meaningful fire type estimates representative of wildfire behaviour in Mediterranean ecosystems. A key result of this study is that intrinsic biophysical characteristics of burned landscapes, such as the fractional cover of char or photosynthetic vegetation, retrieved from sub-pixel image analysis techniques with a physical basis, are more accurate at assessing fire type (e.g. surface or crown fire), given the direct physical interpretation, than commonly-used spectral indices. For example, post-fire char fraction estimates computed by MESMA can be used by forest managers directly to estimate the CFB and thus determine fire type in distinct ecosystems; i.e. without the need for calibration with field data, unlike spectral indices. Such estimates would enable evaluating both the performance of fire behaviour models and pre-fire treatments as moderators of extreme fire behaviour.
Data availability
The data that support this study will be shared upon reasonable request to the corresponding author.
Conflicts of interest
Paulo M. Fernandes is an Associate Editor of International Journal of Wildland Fire, and was blinded from the review process and was not involved at any stage in the editing of this manuscript.
Declaration of funding
This study was financially supported by the Spanish Ministry of Science and Innovation in the framework of LANDSUSFIRE project (PID2022-139156OB-C21) within the National Program for the Promotion of Scientific-Technical Research (2021–2023), and with Next-Generation Funds of the European Union (EU) in the framework of the FIREMAP project (TED2021-130925B-I00); by the Regional Government of Castile and León in the framework of the WUIFIRECYL project (LE005P20); and by Portuguese funds through FCT- Portuguese Foundation for Science and Technology, project UIDB/04033/2020 (DOI: 10.54499/UIDB/04033/2020). José Manuel Fernández-Guisuraga was supported by a Ramón Areces Foundation postdoctoral fellowship. Alfonso Fernández-Manso and Carmen Quintano were supported as research visitors at VIPER Lab. (University of California, Santa Barbara) by a Spanish Education Ministry grant (Salvador de Madariaga program, codes PRX22/00307 and PRX22/00305, respectively).
References
Albini FA, Alexander ME, Cruz MG (2012) A mathematical model for predicting the maximum potential spotting distance from a crown fire. International Journal of Wildland Fire 21, 609-627.
| Crossref | Google Scholar |
Alexander ME, Cruz MG (2012) Interdependencies between flame length and fireline intensity in predicting crown fire initiation and crown scorch height. International Journal of Wildland Fire 21, 95-113.
| Crossref | Google Scholar |
Allen CD, Savage M, Falk DA, Suckling KF, Swetnam TW, Schulke T, Stacey PB, Morgan P, Hoffman M, Klingel JT (2002) Ecological restoration of southwestern ponderosa pine ecosystems: a broad perspective. Ecological Applications 12, 1418-1433.
| Crossref | Google Scholar |
Arkin J, Coops NC, Daniels LD, Plowright A (2023) A novel post-fire method to estimate individual tree crown scorch height and volume using simple RPAS-derived data. Fire Ecology 19, 17.
| Crossref | Google Scholar | PubMed |
Breiman L (2001) Random forests. Machine Learning 45, 5-32.
| Crossref | Google Scholar |
Catry FX, Pausas JG, Moreira F, Fernandes PM, Rego F (2013) Post-fire response variability in Mediterranean Basin tree species in Portugal. International Journal of Wildland Fire 22, 919-932.
| Crossref | Google Scholar |
Collins L, Griffioen P, Newell G, Mellor A (2018) The utility of Random Forests for wildfire severity mapping. Remote Sensing of Environment 216, 374-384.
| Crossref | Google Scholar |
Costa-Saura JM, Bacciu V, Ribotta C, Spano D, Massaiu A, Sirca C (2022) Predicting and mapping potential fire severity for risk analysis at regional level using Google Earth engine. Remote Sensing 14, 4812.
| Crossref | Google Scholar |
Cruz MG, Alexander ME (2017) Modelling the rate of fire spread and uncertainty associated with the onset and propagation of crown fires in conifer forest stands. International Journal of Wildland Fire 26, 413-426.
| Crossref | Google Scholar |
Cruz MG, Alexander ME, Wakimoto RH (2003) Assessing the probability of crown fire initiation based on fire danger indices. The Forestry Chronicle 79, 976-983.
| Crossref | Google Scholar |
Cruz MG, Alexander ME, Wakimoto RH (2004) Modeling the likelihood of crown fire occurrence in conifer forest stands. Forest Science 50, 640-658.
| Google Scholar |
Delcourt CJF, Combee A, Izbicki B, Mack MC, Maximov T, Petrov R, Rogers BM, Scholten RC, Shestakova TA, van Wees D, Veraverbeke S (2021) Evaluating the differenced normalized burn ratio for assessing fire severity using Sentinel-2 imagery in northeast Siberian larch forests. Remote Sensing 13, 2311.
| Crossref | Google Scholar |
Dennison PE, Roberts DA (2003) Endmember selection for mapping chaparral species and fraction using Multiple Endmember Spectral Mixture Analysis. Remote Sensing of Environment 87, 123-135.
| Crossref | Google Scholar |
Dennison PE, Halligan KQ, Roberts DA (2004) A comparison of error metrics and constraints for multiple endmember spectral mixture analysis and spectral angle mapper. Remote Sensing of Environment 93, 359-367.
| Crossref | Google Scholar |
De Santis A, Chuvieco E (2007) Burn severity estimation from remotely sensed data: Performance of simulation versus empirical models. Remote Sensing of Environment 108, 422-435.
| Crossref | Google Scholar |
Dimitrakopoulos AP, Mitsopoulos ID, Raptis DI (2007) Nomographs for predicting crown fire initiation in Aleppo pine (Pinus halepensis Mill) forests. European Journal of Forest Research 126, 555-561.
| Crossref | Google Scholar |
Epting J, Verbyla D (2005) Landscape-level interactions of prefire vegetation, burn severity, and postfire vegetation over a 16-year period in interior Alaska. Canadian Journal of Forest Research 35, 1367-1377.
| Crossref | Google Scholar |
Erni S, Wang X, Taylor S, Boulanger Y, Swystun T, Flannigan M, Parisien MA (2020) Developing a two-level fire regime zonation system for Canada. Canadian Journal of Forest Research 50, 259-273.
| Crossref | Google Scholar |
Fernandes PM (2009) Combining forest structure data and fuel modelling to assess fire hazard in Portugal. Annals of Forest Science 66, 415.
| Crossref | Google Scholar |
Fernandes PM (2013) Fire-smart management of forest landscapes in the Mediterranean basin under global change. Landscape and Urban Planning 110, 175-182.
| Crossref | Google Scholar |
Fernandes PAM, Loureiro CA, Botelho HS (2004) Fire behaviour and severity in a maritime pine stand under differing fuel conditions. Annals of Forest Science 61, 537-544.
| Crossref | Google Scholar |
Fernandes PM, Luz A, Loureiro C (2010) Changes in wildfire severity from maritime pine woodland to contiguous forest types in the mountains of northwestern Portugal. Forest Ecology and Management 260, 883-892.
| Crossref | Google Scholar |
Fernández-García V, Beltrán-Marcos D, Fernández-Guisuraga JM, Marcos E, Calvo L (2022) Predicting potential wildfire severity across Southern Europe with global data sources. Science of the Total Environment 829, 154729.
| Crossref | Google Scholar | PubMed |
Fernández-Guisuraga JM, Suárez-Seoane S, García-Llamas P, Calvo L (2021) Vegetation structure parameters determine high burn severity likelihood in different ecosystem types: a case study in a burned Mediterranean landscape. Journal of Environmental Management 288, 112462.
| Crossref | Google Scholar |
Fernández-Guisuraga JM, Suárez-Seoane S, Calvo L (2019) Modeling Pinus pinaster forest structure after a large wildfire using remote sensing data at high spatial resolution. Forest Ecology and Management 446, 257-271.
| Crossref | Google Scholar |
Fernández-Guisuraga JM, Marcos E, Suárez-Seoane S, Calvo L (2022) ALOS-2 L-band SAR backscatter data improves the estimation and temporal transferability of wildfire effects on soil properties under different post-fire vegetation responses. Science of the Total Environment 842, 156852.
| Crossref | Google Scholar | PubMed |
Fernández-Guisuraga JM, Calvo L, Quintano C, Fernández-Manso A, Fernandes PM (2023a) Fractional vegetation cover ratio estimated from radiative transfer modeling outperforms spectral indices to assess fire severity in several Mediterranean plant communities. Remote Sensing of Environment 290, 113542.
| Crossref | Google Scholar |
Fernández-Guisuraga JM, Marcos E, Calvo L (2023b) The footprint of large wildfires on the multifunctionality of fire-prone pine ecosystems is driven by the interaction of fire regime attributes. Fire Ecology 19, 32.
| Crossref | Google Scholar |
Fernández-Guisuraga JM, Fernandes PM, Marcos E, Beltrán-Marcos D, Sarricolea P, Farris M, Calvo L (2023c) Caution is needed across Mediterranean ecosystems when interpreting wall-to-wall fire severity estimates based on spectral indices. Forest Ecology and Management 546, 121383.
| Crossref | Google Scholar |
Fernández-Guisuraga JM, Martins S, Fernandes PM (2023d) Characterization of biophysical contexts leading to severe wildfires in Portugal and their environmental controls. Science of the Total Environment 875, 162575.
| Crossref | Google Scholar |
Fernández-Manso A, Fernández-Manso O, Quintano C (2016) SENTINEL-2A red-edge spectral indices suitability for discriminating burn severity. International Journal of Applied Earth Observation and Geoinformation 50, 170-175.
| Crossref | Google Scholar |
Fernández-Manso A, Quintano C, Roberts DA (2019) Burn severity analysis in Mediterranean forests using maximum entropy model trained with EO-1 hyperion and LiDAR data. ISPRS Journal of Photogrammetry and Remote Sensing 155, 102-118.
| Crossref | Google Scholar |
Finney MA (2005) The challenge of quantitative risk analysis for wildland fire. Forest Ecology and Management 211, 97-108.
| Crossref | Google Scholar |
Fiorini C, Craveiro HD, Santiago A, Laím L, Simões da Silva L (2023) Parametric evaluation of heat transfer mechanisms in a WUI fire scenario. International Journal of Wildland Fire 32, 1600-1618.
| Crossref | Google Scholar |
Frost SM, Alexander ME, Jenkins MJ (2022) The application of fire behavior modeling to fuel treatment assessments at Army Garrison Camp Williams, Utah. Fire 5, 78.
| Crossref | Google Scholar |
García M, Riaño D, Chuvieco E, Danson FM (2010) Estimating biomass carbon stocks for a Mediterranean forest in central Spain using LiDAR height and intensity data. Remote Sensing of Environment 114, 816-830.
| Crossref | Google Scholar |
Gibson R, Danaher T, Hehir W, Collins L (2020) A remote sensing approach to mapping fire severity in south-eastern Australia using sentinel 2 and random forest. Remote Sensing of Environment 240, 111702.
| Crossref | Google Scholar |
Gorelick N, Hancher M, Dixon M, Ilyushchenko S, Thau D, Moore R (2017) Google Earth Engine: Planetary-scale geospatial analysis for everyone. Remote Sensing of Environment 202, 18-27.
| Crossref | Google Scholar |
Hood SM, Varner JM, van Mantgem P, Cansler CA (2018) Fire and tree death: understanding and improving modeling of fire-induced tree mortality. Environmental Research Letters 13, 113004.
| Crossref | Google Scholar |
Hu T, Ma Q, Su Y, Battles JJ, Collins BM, Stephens SL, Kelly M, Guo Q (2019) A simple and integrated approach for fire severity assessment using bi-temporal airborne LiDAR data. International Journal of Applied Earth Observation and Geoinformation 78, 25-38.
| Crossref | Google Scholar |
Hudak AT, Morgan P, Bobbitt MJ, Smith AMS, Lewis SA, Lentile LB, Robichaud PR, Clark JT, McKinley RA (2007) The relationship of multispectral satellite imagery to immediate fire effects. Fire Ecology 3, 64-90.
| Crossref | Google Scholar |
Jia K, Liang S, Gu X, Baret F, Wei X, Wang X, Yao Y, Yang L, Li Y (2016) Fractional vegetation cover estimation algorithm for Chinese GF-1 wide field view data. Remote Sensing of Environment 177, 184-191.
| Crossref | Google Scholar |
Keeley JE (2009) Fire intensity, fire severity and burn severity: a brief review and suggested usage. International Journal of Wildland Fire 18, 116-126.
| Crossref | Google Scholar |
Key CH (2006) Ecological and sampling constraints on defining landscape fire severity. Fire Ecology 2, 34-59.
| Crossref | Google Scholar |
Key CH, Benson N (2005) Landscape assessment: Ground measure of severity, the Composite Burn Index; and remote sensing of severity, the Normalized Burn Ratio. In ‘FIREMON: Fire Effects Monitoring and Inventory System’. General Technical Report RMRS-GTR-164. (Eds DC Lutes, RE Keane, JF Caratti, CH Key, NC Benson LJ Gangi) pp. CD:LA1–LA51. (USDA Forest Service, Rocky Mountain Research Station: Ogden, UT)
Knox KJE, Clarke PJ (2012) Fire severity, feedback effects and resilience to alternative community states in forest assemblages. Forest Ecology and Management 265, 47-54.
| Crossref | Google Scholar |
Lasslop G, Coppola AI, Voulgarakis A, Yue C, Veraverbeke S (2019) Influence of fire on the carbon cycle and climate. Current Climate Change Reports 5, 112-123.
| Crossref | Google Scholar |
Lentile LB, Smith AMS, Hudak AT, Morgan P, Bobbitt MJ, Lewis SA, Robichaud PR (2009) Remote sensing for prediction of 1-year post-fire ecosystem condition. International Journal of Wildland Fire 18, 594-608.
| Crossref | Google Scholar |
Lydersen JM, Collins BM, Miller JD, Fry DL, Stephens SL (2016) Relating fire-caused change in forest structure to remotely sensed estimates of fire severity. Fire Ecology 12, 99-116.
| Crossref | Google Scholar |
Mallinis G, Mitsopoulos I, Chrysafi I (2018) Evaluating and comparing Sentinel 2A and Landsat-8 Operational Land Imager (OLI) spectral indices for estimating fire severity in a Mediterranean pine ecosystem of Greece. GIScience & Remote Sensing 55, 1-18.
| Crossref | Google Scholar |
Mansoor S, Farooq I, Kachroo MM, Mahmoud AED, Fawzy M, Popescu SM, Alyemeni MN, Sonne C, Rinklebe J, Ahmad P (2022) Elevation in wildfire frequencies with respect to the climate change. Journal of Environmental Management 301, 113769.
| Crossref | Google Scholar | PubMed |
Meng R, Wu J, Schwager KL, Zhao F, Dennison PE, Cook BD, Brewster K, Green TM, Serbin SP (2017) Using high spatial resolution satellite imagery to map forest burn severity across spatial scales in a Pine Barrens ecosystem. Remote Sensing of Environment 191, 9534-109.
| Crossref | Google Scholar |
Miller JD, Thode AE (2007) Quantifying burn severity in a heterogeneous landscape with a relative version of the delta normalized burn ratio (dNBR). Remote Sensing of Environment 109, 66-80.
| Crossref | Google Scholar |
Miller JD, Knapp EE, Key CH, Skinner CN, Isbell CJ, Creasy RM, Sherlock JW (2009) Calibration and validation of the relative differenced Normalized Burn Ratio (RdNBR) to three measures of fire severity in the Sierra Nevada and Klamath Mountains, California, USA. Remote Sensing of Environment 113, 645-656.
| Crossref | Google Scholar |
Miller CW, Harvey BJ, Kane Van R, Moskal LM, Alvarado E (2023) Different approaches make comparing studies of burn severity challenging: a review of methods used to link remotely sensed data with the Composite Burn Index. International Journal of Wildland Fire 32, 449-475.
| Crossref | Google Scholar |
Mitri GH, Gitas IZ (2006) Fire type mapping using object-based classification of Ikonos imagery. International Journal of Wildland Fire 15, 457-462.
| Crossref | Google Scholar |
Mitsopoulos ID, Dimitrakopoulos AP (2007) Canopy fuel characteristics and potential crown fire behavior in Aleppo pine (Pinus halepensis Mill) forests. Annals of Forest Science 64, 287-299.
| Crossref | Google Scholar |
Moran CJ, Hoff V, Parsons RA, Queen LP, Seielstad CA (2022) Mapping fine-scale crown scorch in 3D with remotely piloted aircraft systems. Fire 5, 59.
| Crossref | Google Scholar |
Moreira F, Viedma O, Arianoutsou M, Curt T, Koutsias N, Rigolot E, Barbati A, Corona P, Vaz P, Xanthopoulos G, Mouillot F, Bilgili E (2011) Landscape – wildfire interactions in southern Europe: implications for landscape management. Journal of Environmental Management 92, 2389-2402.
| Crossref | Google Scholar | PubMed |
Morgan P, Keane RE, Dillon GK, Jain TB, Hudak AT, Karau EC, Sikkink PG, Holden ZA, Strand EK (2014) Challenges of assessing fire and burn severity using field measures, remote sensing and modelling. International Journal of Wildland Fire 23, 1045-1060.
| Crossref | Google Scholar |
National Wildfire Coordinating Group (2023) Fire Behavior Field Reference Guide, PMS 437. Available at https://wwwnwcggov/publications/pms437 [accessed 14 October 2023]
Parks SA, Dillon GK, Miller C (2014) A New Metric for Quantifying Burn Severity: The Relativized Burn Ratio. Remote Sensing 6, 1827-1844.
| Crossref | Google Scholar |
Parks SA, Holsinger LM, Panunto MH, Jolly WM, Dobrowski SZ, Dillon GK (2018) High-severity fire: evaluating its key drivers and mapping its probability across western US forests. Environmental Research Letters 13, 044037.
| Crossref | Google Scholar |
Pérez-Izquierdo L, Clemmensen KE, Strengbom J, Granath G, Wardle DA, Nilsson MC, Lindahl BD (2021) Crown-fire severity is more important than ground-fire severity in determining soil fungal community development in the boreal forest. Journal of Ecology 109, 504-518.
| Crossref | Google Scholar |
Perrakis DDB, Cruz MG, Alexander ME, Hanes CC, Thompson DK, Taylor SW, Stocks BJ (2023) Improved logistic models of crown fire probability in Canadian conifer forests. International Journal of Wildland Fire 32, 1455-1473.
| Crossref | Google Scholar |
Pickering BJ, Bennett LT, Cawson JG (2023) Extending methods for assessing fuel hazard in temperate Australia to enhance data quality and consistency. International Journal of Wildland Fire 32, 1422-1437.
| Crossref | Google Scholar |
Picotte JJ, Bhattarai K, Howard D, Lecker J, Epting J, Quayle B, Benson N, Nelson K (2020) Changes to the Monitoring Trends in Burn Severity program mapping production procedures and data products. Fire Ecology 16, 16.
| Crossref | Google Scholar |
Pollet J, Omi PN (2002) Effect of thinning and prescribed burning on crown fire severity in ponderosa pine forests. International Journal of Wildland Fire 11, 1-10.
| Crossref | Google Scholar |
Probst P, Boulesteix AL (2018) To tune or not to tune the number of trees in Random Forest. Journal of Machine Learning Research 18, 1-18.
| Google Scholar |
Quintano C, Fernández-Manso A, Roberts DA (2013) Multiple Endmember Spectral Mixture Analysis (MESMA) to map burn severity levels from Landsat images in Mediterranean countries. Remote Sensing of Environment 136, 76-88.
| Crossref | Google Scholar |
Quintano C, Calvo L, Fernández-Manso A, Suárez-Seoane S, Fernandes PM, Fernández-Guisuraga JM (2023) First evaluation of fire severity retrieval from PRISMA hyperspectral data. Remote Sensing of Environment 295, 113670.
| Crossref | Google Scholar |
R Core Team (2021) ‘R: A language and environment for statistical computing.’ (R Foundation for Statistical Computing: Vienna, Austria) Available at https://wwwR-projectorg/
Roberts DA, Gardner M, Church R, Ustin S, Scheer G, Green RO (1998) Mapping chaparral in the Santa Monica Mountains using Multiple Endmember Spectral Mixture models. Remote Sensing of Environment 65, 267-279.
| Crossref | Google Scholar |
Roberts DA, Dennison PE, Gardner M, Hetzel Y, Ustin SL, Lee C (2003) Evaluation of the potential of Hyperion for fire danger assessment by comparison to the airborne visible/infrared imaging spectrometer. IEEE Transactions on Geoscience and Remote Sensing 41, 1297-1310.
| Crossref | Google Scholar |
Roberts DA, Halligan K, Dennison P, Dudley K, Somers B, Crabbe A (2019) Viper Tools User Manual, version 2.1. https://sites.google.com/site/ucsbviperlab/viper-tools
Rodriguez-Galiano VF, Ghimire B, Rogan J, Chica-Olmo M, Rigol-Sanchez JP (2012) An assessment of the effectiveness of a random forest classifier for land-cover classification. ISPRS Journal of Photogrammetry and Remote Sensing 67, 93-104.
| Crossref | Google Scholar |
Roth KL, Dennison PE, Roberts DA (2012) Comparing endmember selection techniques for accurate mapping of plant species and land cover using imaging spectrometer data. Remote Sensing of Environment 127, 139-152.
| Crossref | Google Scholar |
Roy DP, Boschetti L, Trigg SN (2006) Remote sensing of fire severity: assessing the performance of the normalized burn ratio. IEEE Geoscience and Remote Sensing Letters 3, 112-116.
| Crossref | Google Scholar |
Safford HD, Stevens JT, Merriam K, Meyer MD, Latimer AM (2012) Fuel treatment effectiveness in California yellow pine and mixed conifer forests. Forest Ecology and Management 274, 17-28.
| Crossref | Google Scholar |
Schaaf AN, Dennison PE, Fryer GK, Roth KL, Roberts DA (2011) Mapping plant functional types at multiple spatial resolutions using imaging spectrometer data. GIScience & Remote Sensing 48, 324-344.
| Crossref | Google Scholar |
Shearman TM, Varner JM, Hood SM, van Mantgem PJ, Cansler CA, Wright M (2023) Predictive accuracy of post-fire conifer death declines over time in models based on crown and bole injury. Ecological Applications 33, e2760.
| Crossref | Google Scholar | PubMed |
Sheffer E, Canham CD, Kigel J, Perevolotsky A (2015) Countervailing effects on pine and oak leaf litter decomposition in human-altered Mediterranean ecosystems. Oecologia 177, 1039-1051.
| Crossref | Google Scholar | PubMed |
Soverel NO, Perrakis DDB, Coops NC (2010) Estimating burn severity from Landsat dNBR and RdNBR indices across western Canada. Remote Sensing of Environment 114, 1896-1909.
| Crossref | Google Scholar |
Tane Z, Roberts D, Veraverbeke S, Casas Á, Ramirez C, Ustin S (2018) Evaluating endmember and band selection techniques for multiple endmember spectral mixture analysis using post-fire imaging spectroscopy. Remote Sensing 10, 389.
| Crossref | Google Scholar |
Taylor AH, Harris LB, Drury SA (2021) Drivers of fire severity shift as landscapes transition to an active fire regime, Klamath Mountains, USA. Ecosphere 12, e03734.
| Crossref | Google Scholar |
Tompkins S, Mustard JF, Pieters CM, Forsyth DW (1997) Optimization of endmembers for spectral mixture analysis. Remote Sensing of Environment 59, 472-489.
| Crossref | Google Scholar |
Tripathy KP, Mukherjee S, Mishra AK, Mann ME, Williams AP (2023) Climate change will accelerate the high-end risk of compound drought and heatwave events. Proceedings of the National Academy of Sciences 120, e2219825120.
| Crossref | Google Scholar | PubMed |
Varner JM, Hood SM, Aubrey DP, Yedinak K, Hiers JK, Jolly WM, Shearman TM, McDaniel JK, O’Brien JJ, Rowell EM (2021) Tree crown injury from wildland fires: causes, measurement and ecological and physiological consequences. New Phytologist 231, 1676-1685.
| Crossref | Google Scholar | PubMed |
Wang X, Gao X, Zhang Y, Fei X, Chen Z, Wang J, Zhang Y, Lu X, Zhao H (2019) Land-cover classification of coastal wetlands using the RF algorithm for Worldview-2 and Landsat 8 images. Remote Sensing 11, 1927.
| Crossref | Google Scholar |
Woolley T, Shaw DC, Ganio LM, Fitzgerald S (2012) A review of logistic regression models used to predict post-fire tree mortality of western North American conifers. International Journal of Wildland Fire 21, 1-35.
| Crossref | Google Scholar |
Xofis P, Tsiourlis G, Konstantinidis PA (2020) Fire Danger Index for the early detection of areas vulnerable to wildfires in the Eastern Mediterranean region. Euro-Mediterranean Journal for Environmental Integration 5, 32.
| Crossref | Google Scholar |