An evaluation of wildland fire simulators used operationally in Australia
P. Fox-Hughes
A
B
C
D
E
Abstract
Fire simulators are increasingly used to predict fire spread. Australian fire agencies have been concerned at not having an objective basis to choose simulators for this purpose.
We evaluated wildland fire simulators currently used in Australia: Australis, Phoenix, Prometheus and Spark. The evaluation results are outlined here, together with the evaluation framework.
Spatial metrics and visual aids were designed in consultation with simulator end-users to assess simulator performance. Simulations were compared against observations of fire progression data from 10 Australian historical fire case studies. For each case, baseline simulations were produced using as inputs fire ignition and fuel data together with gridded weather forecasts available at the time of the fire. Perturbed simulations supplemented baseline simulations to explore simulator sensitivity to input uncertainty.
Each simulator showed strengths and weaknesses. Some simulators displayed greater sensitivity to different parameters under certain conditions.
No simulator was clearly superior to others. The evaluation framework developed can facilitate future assessment of Australian fire simulators.
Collection of fire behaviour observations for routine simulator evaluation using this framework would benefit future simulator development.
Keywords: Australis, evaluation framework, fire behaviour modelling, fire simulation modelling, operational fire modelling, Phoenix, Prometheus, Spark.
Introduction
Fire is integral to the Australian landscape (Russell-Smith et al. 2007) but poses a hazard to life and property (Scott et al. 2013; Doerr and Santín 2016; EM-DAT 2018). Each year, fire burns part of the Australian landscape (Carmona-Moreno et al. 2005; Giglio et al. 2013), and occasional fire disasters have caused widespread destruction and many deaths (e.g. Stretton 1939; Cheney 1976; Brotak 1980; Miller et al. 1984; Mills 2005; Teague et al. 2010; Owens and O’Kane 2020; Tomašević et al. 2022). Fire and land management agencies attempt to manage fire in the landscape. Over the last two decades in particular, fire behaviour simulators, which model the spread and some other properties of fires, have become a tool to predict fire behaviour for that management (Finney 2004; Opperman et al. 2006; Haas et al. 2013). Simulator applications include estimating the likelihood of fire ignitions becoming difficult to control (Finney et al. 2011), predicting direction and rate of growth of going fires (Tymstra et al. 2010), assessing the impact of fuel reduction strategies on future fires (Ager et al. 2010; State Fire Management Council 2014) and identifying safe evacuation routes from wildfires (Ozaki et al. 2019). Owing to their quite recent introduction, wildland fire simulators lack standardisation, and new simulator versions have sometimes been introduced to operations without clear and comprehensive assessment of their suitability. Several simulators, and sometimes different versions of the same simulator, are used in wildfire management in Australia, often within one agency. Fire agencies have become concerned that they do not know whether the best simulator is used for any given application (Cruz et al. 2014).
Simulator evaluations have been reported from fire-prone regions globally. These studies, although often including multiple case studies, have tended to focus on individual simulators (e.g. Arca et al. 2007; Duff et al. 2013; Kelso et al. 2015; Miller et al. 2015; Giannaros et al. 2020), though there are exceptions. Opperman et al. (2006) examined simulators then available for their applicability in Australia and New Zealand, and Duff et al. (2018) compared versions of the Phoenix simulator. Some simulator evaluations have incorporated investigation of simulator input uncertainty (e.g. Bachmann and Allgöwer 2002; Fujioka 2002; Finney et al. 2011; Benali et al. 2016; Allaire et al. 2020, 2022; DeCastro et al. 2022) and variability (e.g. Hilton et al. 2015), whereas Cruz and Alexander (2013) dealt with modelling uncertainty in the fire behaviour models that underpin simulators. Pinto et al. (2016) used an ensemble of FARSITE instances to represent input uncertainty when generating probabilistic fire spread predictions for a fire in Portugal. Plucinski et al. (2017) presented a package, Amicus, aiming to standardise fire behaviour prediction by including an understanding of simulator limitations and expert knowledge. They also discussed a framework for uncertainty analysis. Penman et al. (2020) focussed on the impact of weather forecast error on two simulators, Phoenix and Spark, in order to avoid the potential for model bias to influence their results. The authors found considerable sensitivity on the part of simulators to variability in input weather parameters.
Approaches to simulator evaluation have used numerous performance metrics, some of which we note here. Filippi et al. (2014) addressed previous approaches to evaluation, including the Sørenson similarity index, Jaccard’s coefficient and Kappa statistics, but also advancing Arrival Time and Shape Agreement, in an effort to accommodate imprecision in underlying validation data. Duff et al. (2016) assessed Shape Deviation Index and Area Difference Index, as well as Jaccard’s coefficient and Sørenson similarity.
Efforts to formalise evaluation of fire simulation systems extend back at least to Rothermel and Rinehart (1983) for the Rothermel (1983) wildland fire spread model. Filippi et al. (2014) introduced a formal evaluation protocol that targeted dynamic properties of fires and fire simulations but noted that no single measure of simulator performance was likely to comprehensively capture simulator error characteristics. Recently, again, Duff et al. (2018) proposed an approach to evaluate relative performance of simulator versions using Area Difference Index.
To our knowledge, there has not been an attempt to date to evaluate multiple simulators for a large variety of case studies, taking uncertainty in input variables into account and visualising the results for different end-users. Here, we present a new evaluation that achieves those aims, using a framework that caters for a wide range of test cases and visualises simulator performance using methods new to this field. By ‘framework’, we mean a suite of software that automates the evaluation of fire simulators against a defined set of documented fire events. The framework acknowledges that different user groups may have different decision requirements in any evaluation process. Such users will include high level fire agency managers looking to improve agency predictive capability, fire behaviour analysts attempting to obtain the best possible simulations of fire behaviour during individual incidents, developers attempting to enhance simulator performance by improved algorithms and potentially other groups. Thus, a range of simulator performance metrics were used to accommodate the range of user requirements.
Such evaluation is important for fire authorities to understand the effectiveness of predictive tools at their disposal, and the circumstances in which simulators can be expected to perform well or poorly. Simulator evaluation, and documentation of the evaluation process, is important not only for fire behaviour analysts using simulators as a routine part of their work, but for senior fire agency managers making decisions about which simulators their organisations will use or support in development. It is also important for simulator developers to assist them in identifying the extent to which changes in simulator design improve on earlier efforts.
As noted, the framework developed to carry out evaluations consists of software for organising the execution of simulators in a manner mimicking operational use, including presenting them with input data with uncertainty estimates to assess the degree of simulator sensitivity to sometimes uncertain real-world input data, and computing metrics of simulator performance against fire spread observations. Finally, investigation of (relative) simulator performance was also identified as an important consideration for users. Plots were developed to assist in the analysis of evaluation metrics and to cater for different user needs, as noted above.
The work described in this paper was commissioned by the Australasian Fire and Emergency Service Authorities Council, AFAC, with the intention of better understanding the accuracy and sensitivities of fire simulators used in Australia at the time. It was also anticipated that the work undertaken would provide a mechanism for assessing fire simulators and simulator versions developed in the future.
AFAC requested the Australian Bureau of Meteorology undertake the assessment of fire simulator performance. The Bureau has a long involvement in fire weather forecasting and has worked closely with fire managers over many decades. In addition, fire managers wanted to make use of the Bureau’s extensive experience in running models of physical systems – albeit weather and environmental systems rather than fire, largely – and in verifying the performance of those models.
An initial workshop involving researchers and senior operational managers from fire and land management agencies around Australia established key requirements that users wanted addressed concerning simulator performance. The workshop also agreed on a broad approach to simulator assessment, and identified a number of test cases of fire events against which simulator performance would be evaluated.
Ten historical fires were used to test the performance of four simulators used in Australia: Australis (Johnston et al. 2008), Phoenix (Tolhurst et al. 2008), Prometheus (Tymstra et al. 2010) and the Spark simulator framework (Hilton et al. 2015).
Australis was developed by the University of Western Australia. The Western Australian Department of Fire and Emergency Services’ ‘Landgate’ web interface http://aurora.landgate.wa.gov.au/home.php permitted Australis version 1.5.6 to run remotely on Landgate, with simulator output downloaded for evaluation.
Phoenix was developed by the University of Melbourne. The evaluation included four Phoenix versions: 4.06, 4.07, 4.08 and 5.00. Several Phoenix versions were used by operational staff in fire agencies at the commencement of the project, while version 5.00 represented a development version. It was unclear to fire agencies whether successive versions were superior to their predecessors, so all in use, or potentially in use, were submitted for evaluation. The simulator was run automatically in ‘batch’ mode, enabling a large number of simulations to be included.
Prometheus, the Canadian Wildland Fire Growth Simulator Model, was developed by the Canadian Forest Service and is supported by Alberta Agriculture and Forestry. Version 6.1.0.7 was included in the evaluation project, downloaded from the agency website http://firegrowthmodel.ca/. The simulator was run manually only for one case study, the Wuthering Heights fire in Tasmania, owing to limited availability of base fuel layer inputs in the required format. Tasmanian fire agencies have adapted Prometheus fuel and moisture parameters to permit its use in that jurisdiction.
Spark simulator framework, version 0.8.0, was used to develop a set of fire spread simulators workflows, called Basic, Vesta and McArthur, where ‘workflow’ here is used to describe a fire behaviour model running within the Spark framework. These varied in the selection of fire spread models and the detail of fuel modelling included in the workflow. For grass fuels, ‘Basic’ used the CSIRO grassland meter unmodified; ‘Vesta’ varied the model based on condition (natural, grazed, eaten out); and ‘McArthur’ varied the model based on condition and a shape constraint applied to fire flank and backing spread. The very recent development of Spark at the time of this evaluation meant that it had not yet been configured for operational use anywhere in Australia, so these workflows were developed by New South Wales Rural Fire Service (NSW RFS) for evaluation. They were reviewed by CSIRO Spark developers, then run automatically.
Details of the fire behaviour models and fire propagation methods used in each simulator are provided in Table 1. Description of the implementation is available in Bureau of Meteorology (2017).
Simulator | Fire model(s) | Fire propagation | |
---|---|---|---|
Australis | Several vegetation-specific models
| Cellular automata | |
Phoenix | Composition of:
| Huygens’ Principle (Knight and Coleman 1993) | |
Prometheus | Canadian Forest Fire Behaviour Prediction system (Forestry Canada 1992), modified for Tasmanian fuel types | Huygens’ Principle | |
Spark | Models are user-defined. For this project:
| Level set methods |
This paper focuses on describing the results obtained in one case study event, and illustrates the application of the evaluation framework with example data and plots from that case study. Also, we offer some observations on the effectiveness of the framework. Full results of the evaluation are available in Bureau of Meteorology (2017), together with greater detail of the evaluation framework.
Evaluation method
As noted above, at the commencement of the project, senior operational fire managers from most Australian state and territory fire authorities attended a workshop with project staff to discuss project implementation. Participants agreed that evaluation of a range of test cases was an appropriate way of assessing simulator performance. Fire agency staff selected 10 historical fire events for analysis, across a range of climates and vegetation types, in which fire spread was not suppressed during the analysis period. The latter constraint was added to ensure that the simulators were assessed simply and fairly, i.e. without having to engage suppression sub-models that may have confounded the analysis of their basic operation. Case study baseline conditions are summarised in Table 2. Limited availability of observed fire behaviour data constrained the number of possible case studies. To approximate conditions agreed in the initial workshop, a fairly unsuppressed fire run of 6–12 h was required, from either a known ignition point or perimeter, along with the corresponding final fire perimeter for the simulation period. For each case study, fire agencies supplied data layers required to run simulators, including fuel and topography, as well as fire observations. Fire agency staff were also asked during the initial project scoping workshop which aspects of fire behaviour simulators were important to them. Their answers informed the development of the simulator evaluation framework. The fire agency staff required knowledge on:
which simulators perform best, both overall and in individual cases;
simulator accuracy across a range of output attributes (bearing, rate of spread, burnt area); and
sensitivity of simulators to variations and uncertainty in input parameters.
Fire case study (state) | Simulation start | Ignition | ADFD weather (forecast local time (hours) and location) | Dominant fuel and final fire area | |
---|---|---|---|---|---|
Simulation end (local time; hours) | |||||
Ballandean (Qld) | 27/10/14 12:38 | Point ignition | 04:00 26/10/14 | >1000 ha | |
28/10/14 16:40 | −28.8060, 151.8691 | −28.8060, 151.8691 | Grass/forest | ||
Cobbler Road (NSW) | 08/01/13 15:54 | Point ignition | 06:00 07/01/13 | 14,000 ha | |
08/01/13 20:04 | −34.8361, 148.4197 | −34.8361, 148.4197 | Grass | ||
North Grampians (Vic.) | 17/01/14 01:00 | Polygon | 16:00 16/01/14 | 52,000 ha | |
17/01/14 10:00 | −36.9701, 142.4191 | Forest/grass | |||
Pinery (SA) | 25/11/15 12:00 | Point ignition | 04:00 24/11/15 | 82,000 ha | |
26/11/15 00:00 | −34.3069, 138.4225 | −34.3100, 138.4200 | Crop/grass | ||
Sampson Flat (SA) | 02/01/15 12:20 | Point ignition | 05:00 01/01/15 | 12,500 ha | |
02/01/15 18:00 | −34.7469, 138.7955 | −34.7452, 138.7977 | Forest | ||
State Mine (NSW) | 16/10/13 12:00 | Point ignition | 17:00 15/10/13 | >55,000 ha | |
16/10/13 16:23 | −33.4366, 150.1605 | −33.4366, 150.1605 | Forest/heath | ||
Wambelong (NSW) | 12/01/13 09:50 | Point ignition | 05:00 11/01/13 | 56,280 ha | |
13/01/13 14:35 | −31.2768, 148.9698 | −31.2768, 148.9698 | Forest | ||
Waroona (WA) | 06/01/16 06:30 | Point ignition | 04:00 05/01/16 | 69,165 ha | |
06/01/16 14:50 | −32.8900, 116.1700 | −32.8899, 116.1842 | Forest | ||
Wuthering Heights (Tas.) | 26/01/16 18:26 | Polygon | 16:00 26/01/16 | 21,970 ha | |
27/01/16 10:49 | −41.1367, 144.7762 | Forest/moor | |||
Wye River (Vic.) | 25/12/15 11:21 | Polygon | 21:00 24/12/15 | >50,000 ha | |
25/12/15 18:00 | −38.5950, 143.9000 | Forest/coastal |
Note: all simulation times are listed in local time. ADFD is Australian Digital Forecast Database, the source of publicly available gridded weather forecasts used to provide simulator weather input data.
Simulator input sensitivity is important for several reasons. Most data available from firegrounds are necessarily imprecise and sparse and may not reflect conditions across an entire active fire. It is important for users to understand how such imprecision and inaccuracy may affect simulator behaviour, and whether, for example, it might be advisable, or even necessary, to run several simulator instances to capture output uncertainty, given known uncertainties in input parameters. Note that such simulator sensitivity may be appropriate if fire behaviour is similarly sensitive to those inputs. These requirements dictated the specific methods used to investigate simulator performance. Archived official weather forecast grids from the Australian Digital Forecast Database (ADFD, Bureau of Meteorology 2015) were used as weather inputs for all cases. ADFD grids are produced from numerical weather predictions edited by forecasters and are available throughout Australia, providing a representation of average weather parameters over 6 km grid cells (3 km over Victoria and Tasmania) at the times of the case studies. To produce hourly input weather time series, ADFD grids were sampled at the ignition point or, if a case involved prediction from an existing fire, the centroid of the observed fire perimeter. This was undertaken to provide the most representative weather for the initial fire point or perimeter. The derived weather stream dataset for each case study comprised on-the-hour forecast values of temperature, relative humidity, wind speed and wind direction, together with 3-hourly drought factor (DF, an indication of the proportion of forest fuel available to burn; McArthur 1967) and weekly updated observations of grassland curing, depending on the nature of the vegetation burnt. Data from the three nearest automatic weather stations (AWS) were compared against ADFD grids to estimate weather parameter uncertainty.
Simulators were tested using data from the selected events, using baseline data provided by agencies; no attempt was made to tune results by modifying local fuel or weather inputs as would usually occur when using simulators operationally, except in one case study to explore the effects of changing clearly incorrect forecast weather. Where included, suppression modules were deactivated.
This approach was used to best represent the real-world usage of simulators. Users wanted, for example, to know which simulator(s) best reproduce fire behaviour, irrespective of inputs that may not be completely accurate. To have provided ‘perfect’ inputs would not have achieved this aim, even though it would have isolated potential model errors from the possibility of input errors affecting simulator outputs.
Input variables were perturbed independently to explore sensitivity of simulators to each input. Simulator outputs were compared against available fire observations using evaluation metrics that relate to spatial verification of the fire boundary, targeting area, bearing and distance of forward spread, and overlap between observed and simulated burnt area. Uncertainties in fuel type, fuel load and weather were quantified using a data reliability assessment defined by Cruz et al. (2012).
We estimated uncertainties in weather data from weather forecast errors determined around the time and place of each case study, using AWS observations as noted above. This local ‘error climatology’ approach matched ADFD grid cells with AWS observations (Bridge 2015) in the vicinity of each case study (e.g. Fig. 1) to create error histograms from which perturbations were determined (Pinto et al. 2016).
(a) The Blue Mountains, New South Wales, showing the best-guess ignition location for the State Mine fire and the location of three AWSs. (b) ADFD temperature forecast ‘error climatology’ derived from AWSs in the vicinity of the State Mine fire of October 2013.
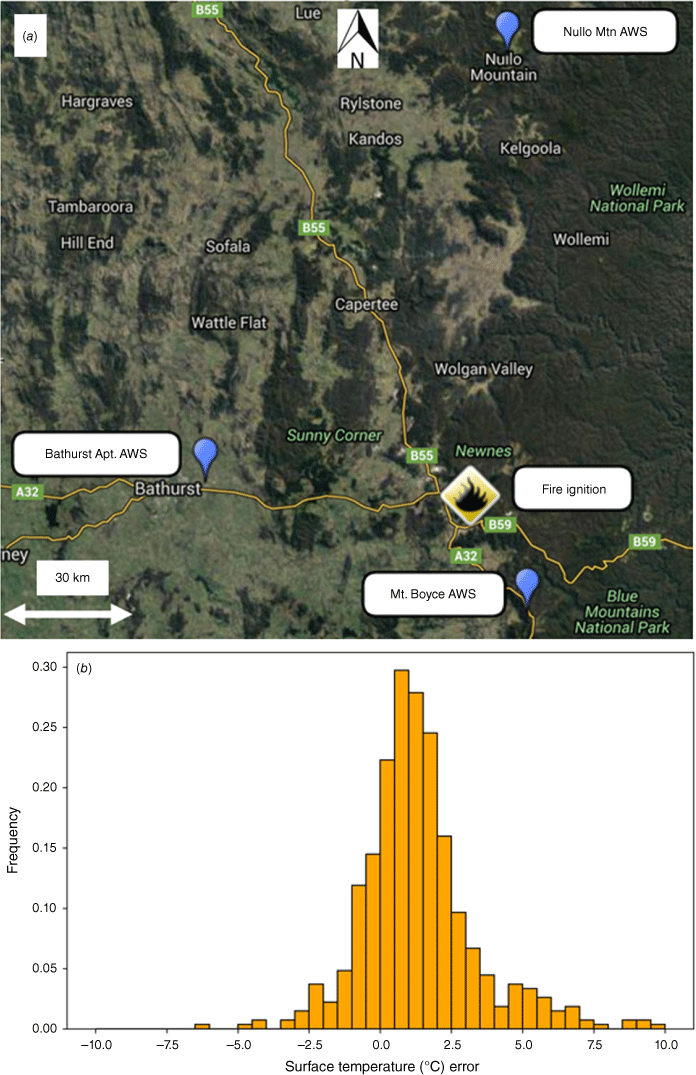
Perturbations were also applied to baseline fuel load for each case study using linear sampling in the range of ±20%, based on NSW RFS estimation. Cloud, DF and curing perturbations were made by linearly sampling in the range ±10%, based on fire weather meteorologist estimation. Ignition time perturbations were made by linearly sampling within a ±30 min window of best guess ignition time, based on NSW RFS estimation. Ignition location perturbations were made by randomly sampling within a 200 m radius of the ‘best guess’ ignition location; this range was determined arbitrarily. Some 19 perturbations were applied, yielding 20 input values, including the baseline value, for each input variable. In our application of the framework, Spark and Phoenix simulators were run 201 times for each case study (20 simulations for each of the 10 input variables perturbed plus a baseline simulation) but Prometheus and Australis simulations had to be performed manually, resulting in fewer perturbed runs (16 for each case study for Australis, 16 for one – Tasmanian – case study for Prometheus). Also, fewer input variables could feasibly be perturbed for those simulators. The alternative was not to include Australis and Prometheus in the evaluation, which was not desirable.
Details of the computing infrastructure built to run the evaluation framework are available in the project final report (Bureau of Meteorology 2017). Briefly, the evaluation was divided into four general reproducible workflows to be applied for each experiment: input data preparation, comparison data (observation) normalisation, simulator execution and summarisation.
Two simulators (Phoenix and Spark) were run automatically using the Simulator execution workflow. Prometheus (in the Tasmanian case, where fuel state data were available) and Australis were run manually outside of the Simulator execution workflow, as noted, with outputs returned and ingested into the summarisation workflow to allow evaluation using standard metrics.
Simulators ran using the standardised inputs and produced simulated fire boundaries. Metrics were generated to assess simulator accuracy and sensitivity. Parameters considered in the sensitivity analysis included wind speed, wind direction, temperature, humidity, ignition location, ignition time, DF, curing, cloud and fuel layer, where simulators permitted varying these parameters.
Given user interest expressed in the initial workshop in accurate prediction of fire spread, bearing and area burnt, we adopted four metrics in the evaluation framework. Jaccard’s coefficient is commonly known as the threat score (TS) in weather warning evaluation and is defined as:
where H (hits) is the area correctly identified as burnt, FA (false alarms) the area incorrectly identified as burnt and M (misses) the area not predicted to burn but that was burnt (Fig. 2). A good TS is closer to 1 whereas a poor TS is near 0.
Schematic diagram showing how the evaluation metrics are calculated. The diagram depicts an idealised observed (red) and simulated (blue) fire. A common ignition location is indicated by the black cross. Overlapping (H, hits) and non-overlapping (M, misses; FA, false alarms) components of the simulated and observed fires are used for calculation of the threat score. Bearing of the simulated and observed forward spread of the fire is determined using a binned circular weighting approach (described in the text). Bearing error is the difference between simulated bearing bin (blue sector) and observed bearing bin (red sector). Forward spread error is determined from the difference between the observed and simulated maximum fire extent in the direction of the observed and simulated bearing bins, respectively. Burnt area error is the difference between the total burnt area within the simulated fire perimeters and the total burnt area within the observed fire perimeter.
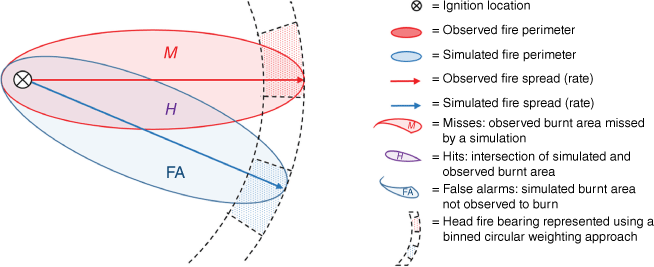
The bearing error is computed as the simulated bearing minus observed head fire direction (e.g. Duff et al. 2012). The range is [0, 180] degrees with a good bearing error being closer to zero. Bearing error may point to errors in simulator inputs including wind direction, fuel mapping, topography or disruptions.
The average forward rate of head fire spread is given by the distance travelled by the head fire during a defined time interval. Various metrics have been employed to measure the difference between simulated and observed rates of spread (e.g. Cruz and Alexander 2013; Cruz et al. 2015). Here, we use forward spread error. This is the difference between the simulated and observed head fire spread – a distance rather than a speed. Converting between these two metrics is trivial as they differ only by a scaling factor. Forward spread errors can be expected to relate to the one-dimensional fire models underpinning the simulators and the weather and fuel inputs that drive them. However, unless the fuel and topography in the fire landscape are homogeneous, this depends on an accurate fire bearing. A good forward (rate of) spread error is close to zero.
The burnt area error is simulated minus observed total burnt area (compared graphically by Cui and Perera 2010). Positive (negative) burnt area errors represent over (under)-prediction. Small values are desirable. Absolute values of error can be important operationally because they correlate to damage, CO2 emissions and suppression effort. However, absolute errors may be misleading when comparing fires that differ greatly in size. An alternative, relative error measure is the ratio of simulated to observed total burnt area. However, a 100% overprediction has different operational implications if the real fire is 3 ha or 3000 ha. The choice of metric depends on user need. Burnt area errors can arise from underlying model errors, and input fuel- and weather-related errors. These evaluation metrics are summarised for convenience in Table 3.
Metric | Citation | Comments on representation in citations | |
---|---|---|---|
Threat score | Filippi et al. (2014), Duff et al. (2016) | As Jaccard’s coefficient | |
Bearing error | Duff et al. (2012) | As orientation error | |
Forward spread error | Cruz and Alexander (2013) | Discussed, without being explicitly defined | |
Burnt area error | Cui and Perera (2010) | As Simulation Error Index |
Discriminating between false alarms and misses, and hence information on simulator over- and under-prediction, can be achieved with two auxiliary measures:
These are, respectively, identical to the definitions of ‘recall’ and ‘precision’ in Duff et al. (2016). In addition, SR is equal to 1– FA. TS, PoD and SR can be represented on a categorical performance diagram, described later. Of these metrics, TS and SR penalise false alarms, TS and PoD penalise misses, and all are insensitive to correct negatives. This is desirable, as the metrics are then independent of fire domain area, which can be defined arbitrarily. This problem besets Cohen’s kappa coefficient (Finney 2000; Filippi et al. 2014; Duff et al. 2016).
High-level summaries of relative simulator accuracy for the baseline runs are obtained by comparing outputs from each metric using a technique developed for evaluation of climate models (Gleckler et al. 2008). Simulator performance relative to other simulators is colour-coded so that poor performance of a simulator is displayed in these ‘Gleckler plots’ as progressively deeper shades of pink as performance deteriorates and progressively deeper shades of green as performance improves. White denotes performance close to the median of simulator performance.
Results
First, we present results for one case study, the State Mine Fire (NSW), to illustrate evaluation outputs and demonstrate how these metrics are used to address the user requirements identified in the Evaluation Methods section.
Of the 10 case studies in the evaluation project, the State Mine Fire was chosen because quite frequent fire boundary observations were available. This was not always the case with other fire case study events owing to firefighting resource limitations. Additionally, simulator performance was broadly similar for the State Mine Fire. Across all cases studies, no simulator performed markedly better than others but, in some cases, one or other simulator appeared superior on account of the specific details of the case. We wanted to avoid any perceptions that one simulator was clearly superior to others in the case study we presented. We also present summary results and plots for Spark and Phoenix simulators to illustrate the capacity of the evaluation framework to provide an overview and intercomparison of simulator performance. Comprehensive results and interpretation for all case studies are available in Bureau of Meteorology (2017).
Figs 3–5 address the user requirements for better understanding of simulator relative performance and accuracy. Baseline results for the State Mine Fire case for each simulator (Fig. 3, yellow) compared with the observed fire boundary at the same time (orange) permit subjective visual assessment of baseline simulation results, providing users with an indication of relative simulator performance and accuracy compared with observed conditions. Rate of spread is overestimated by all simulators, and forecast bearing differs from observed fire behaviour for most simulators, resulting in little overlap. The uniform overestimation of rate of spread suggests that the input weather forecast data were inaccurate. This is perhaps unsurprising. Forecast data were obtained from routine grids at 6 km horizontal resolution and the fire occurred in complex terrain. Such routine data were used to ensure consistency between case studies, as noted above. Also of interest is the fire aspect ratio, with a range of length to width ratios between simulators.
Baseline simulations for the State Mine Fire, showing the predicted fire perimeter from different simulators in yellow and the observed fire perimeter in orange.
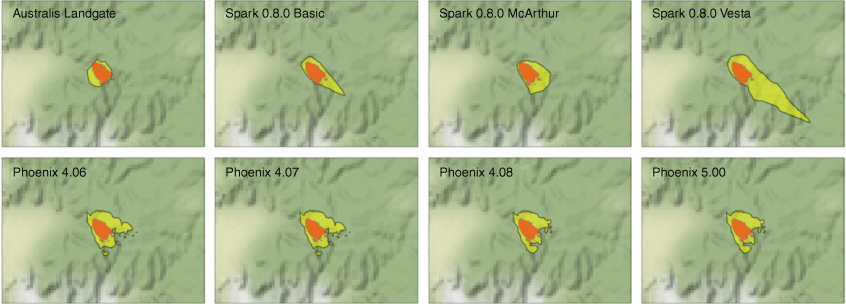
Example relative comparisons for the State Mine baseline fire case study for (a) Threat score, and (b) Bearing error, showing the performance of each simulator coloured by the distance from median simulator performance (in white). Green (pink) colours indicate relatively better (worse) accuracy in comparison with other simulators. Absolute metric values for the worst, median and best-performing simulator are also provided in inset text in the colour legends.
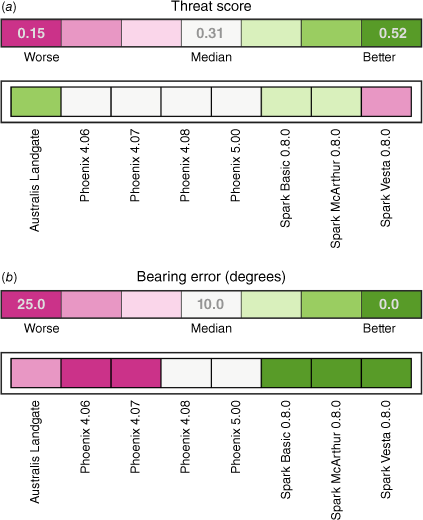
Categorical performance diagram for the State Mine fire case study, providing a direct visual comparison of simulator performance. Dashed lines show constant values of Threat Score, with better performance towards the top right corner. Overprediction of fire spread appears above the diagonal and underpredicted results appear below the diagonal.
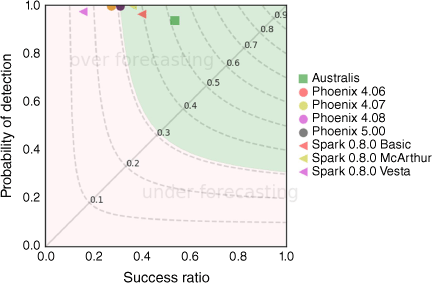
Fig. 4 displays a Gleckler plot for the State Mine fire simulations, specifically addressing the user question of which simulator performs best relative to others. Phoenix versions show similar performance for TS while Australis has the best TS (Fig. 4a), in part because the total area burnt is similar between modelled and observed cases (Fig. 3), but potentially also because the Australis version tested had wind directions fixed to compass points. However, the Bearing Error for Australis is relatively poorer than that of Spark and later Phoenix versions.
A categorical performance diagram (Roebber 2009) displays absolute, rather than relative, TS values (Fig. 5), providing users with an overview of objective simulator performance against observed fire behaviour, in addition to an indication of relative performance. The vertical axis shows PoD, the horizontal axis shows SR and dashed lines the TS, with the latter increasing towards the top right corner of the diagram. Overprediction of fire spread appears above the diagonal and underpredicted results appear below the diagonal. The superior TS of Australis in this example is clear, as is the substantial area error above the diagonal representing overprediction. The high PoD but relatively lower SR for all other simulators objectively highlight the false alarms evident from Fig. 3.
To address the third user requirements specified in the evaluation Methods section, better understanding of simulator sensitivity to variability and uncertainty in input parameters, a visual assessment can again be used to explore sensitivity across all input variables amenable to perturbation. For example, maps for each simulator in Fig. 6 display the effect of varying wind direction within the estimated error distributions for ADFD weather forecast grids, based on the error climatologies derived using data from nearby AWS. Again, baseline results for each simulator are in yellow, compared with the observed fire boundary at the final time step shown in orange. The area burnt in the simulations by 1, 10 and 19 or more of the 20 simulations with perturbed wind direction inputs is indicated by the dotted, dashed-dotted and solid thick black boundaries, respectively. These results show high sensitivity to uncertainty in the wind direction, giving a wide range of forward spread bearings. For most tested simulators, however, the solid black line includes most of the observed fire boundary; thus, 19 of the 20 perturbed simulations included most of the observed burnt area. Note, again, that the requirement to manually input data into Australis did not permit its evaluation in this fashion. Its baseline results are included in Fig. 6 for reference.
As in Fig. 3 but also showing effect of perturbing the wind direction input to each simulator. The simulated area burnt by at least 1, 10 and 19 of the 20 simulations with different wind direction inputs is indicated by the dotted, dashed-dotted and solid thick black lines, respectively. No wind perturbations were applied to the Australis simulator.
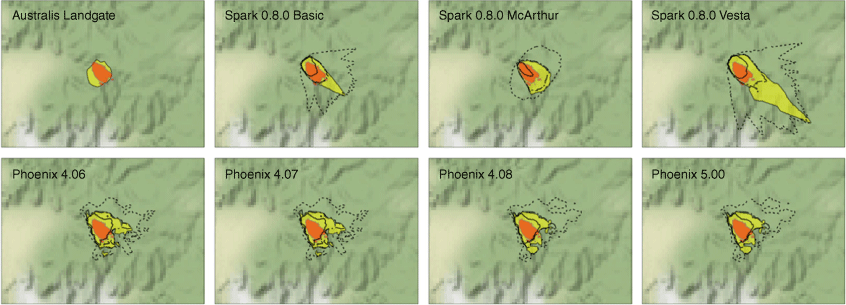
These sensitivity results can also be viewed for each input variable in terms of relative simulator performance. Display of relative performance specifically addresses user requirements to identify the best simulator for any case study. Fig. 7 shows the relative inter-comparison of simulators by varying input parameters, using a modified Hinton diagram. Box size indicates the level of sensitivity of the simulator output for each variable. Colours again identify the relative accuracy of simulators. Here, the median metric value of the perturbed simulations for each simulator is compared with the median metric value for the perturbed simulations for all simulators. White indicates a near-median result, pink indicates results worse than 25th percentile and green indicates a simulator performed better than 75th percentile of assessed simulators.
Modified Hinton diagram for the State Mine Fire case study, showing the sensitivity and accuracy of simulators to variations of input parameters. The box size indicates the sensitivity of the Threat Score to perturbations in corresponding parameters. Green (pink) colours indicate relatively better (worse) accuracy in comparison with other simulators. Absolute metric values for the worst, median and best-performing simulator are also provided in parentheses.
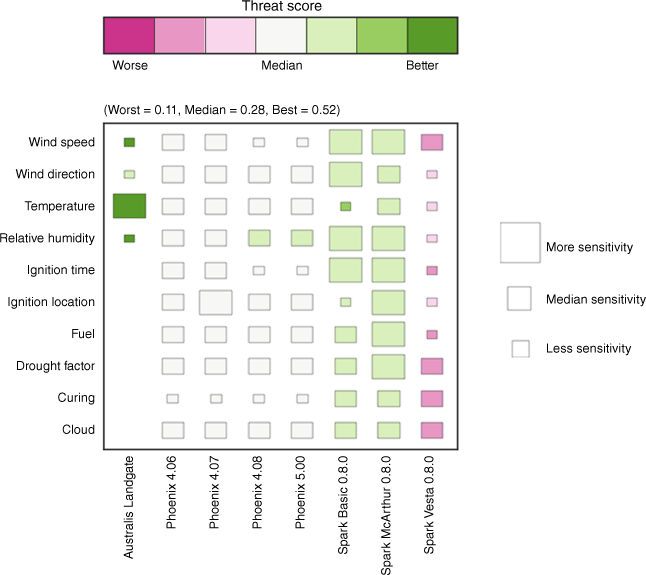
Sensitivity results for Australis should be interpreted with care. Instance simulations were run manually, then inserted into the evaluation with a smaller sample size and fewer variables, as noted. Manual operation was required because Australis codebase was not available for automation. Although far fewer instances (17) could be run than for Phoenix and Spark versions (201 each), it was considered valuable to include Australis results here to highlight how simulator differences affected the operation of this evaluation framework and how it was sufficiently flexible to include diverse simulators, and to provide an indication of the relative performance of simulators used operationally in Australia.
Absolute sensitivity performance can also be visualised using the categorical performance diagram shown in Fig. 5. Given the larger number of runs when including the perturbations for each input variable, there are more results to display, often resulting in clusters or ‘snakes’ of points for each simulator. Although users did not specifically request an indication of absolute performance in the evaluation of simulators, as noted above, this type of evaluation permits an assessment of the degree to which simulators differ in their performance, as well as their departure from a perfect (i.e. identical to that observed) output. Fig. 8 shows an example for perturbations to wind direction input, again for the State Mine Fire.
Performance diagram for the State Mine fire for all simulators including perturbed wind direction inputs as well as the baseline input.
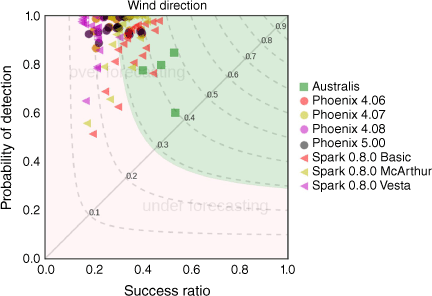
To provide a measure of overall simulator performance, i.e. across all case studies, metrics must be aggregated. We explored further extending the performance diagram. Whereas Fig. 8 shows, for all simulators, the results of simulations from one case study and perturbing one input variable (wind direction), Fig. 9 shows for Spark (left) and Phoenix (right) simulators the TS values for all simulations for all 10 case studies and all 10 perturbed input variables. In contrast to the performance diagram in Fig. 8 where data are represented as points, in Fig. 9, the larger number of data points are instead summarised by allocating them to bins. Interpretation of the diagram remains the same insofar as, ideally, most simulations would fall in the upper right bin, and a tendency to over (under)-predict fire spread results in high relative frequencies above (below) the diagonal.
A visual meta-analysis approach based on the performance diagram. The two diagonal lines enclose the ±50% over- and under-prediction region, i.e. where prediction bias is more acceptable. Left: all Spark simulators, all case studies. Right: all Phoenix simulators, all case studies. Darker (lighter) colours show higher (lower) proportions of the simulations.
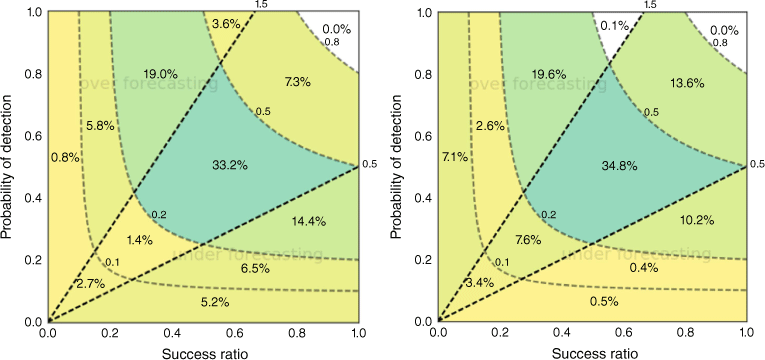
The above results indicate that different simulators, and different versions of the same simulator, perform better than others as conditions vary. This result carried through across case studies, with no simulator performing well against all, or even most, case studies. Given the varied and extreme nature of available case studies, and the small number available for this study, it is not possible to draw conclusions regarding simulator accuracy for specific fire types or weather conditions from this evaluation.
Discussion and summary
Evaluation is important both in absolute terms, for users to know the extent to which simulators can be expected to reproduce observed fire conditions, and in relative terms, to enable assessments of whether and by how much successive simulator versions improve over their predecessors. Our evaluation, described fully in Bureau of Meteorology (2017), highlights substantial differences in performance between simulator versions. It is also valuable to know how well simulators compare with other simulator implementations. The evaluation framework presented in association with case study results provides a tool for testing and verification of new simulator releases to assess operational impacts and risks as well as improvements.
We describe results of one case study, the State Mine Fire in NSW in October 2013. We also, however, demonstrate the information that can be gained from aggregating results of multiple case studies (Fig. 9). For the 10 cases we examined, no one simulator was universally superior to the others. The framework designed for this evaluation is sufficiently flexible to accommodate available case study verification data from a wide variety of perspectives. Such flexibility is valuable to account for differing requirements for simulator evaluation from a variety of user groups, and from the same users over time as their needs change and as simulators develop.
A set of spatial metrics based on simulated and observed fire perimeters was selected to answer the questions related to fire spread, bearing and area. These consisted of a summary metric, threat score and three diagnostic metrics (head fire spread error, head fire bearing area and burnt area error) enabling more detailed characterisation of simulation errors. Our choice of metrics is not prescriptive or comprehensive – additional metrics have been defined and used in the literature (e.g. Filippi et al. 2014; Kelso et al. 2015; Duff et al. 2016). Threat Score does not, for example, explicitly distinguish misses and false alarms. Were this a primary evaluation requirement, an alternative might be a combination of ‘probability of detection’ and ‘success ratio’ that form the axes of the categorical performance diagram (e.g. Fig. 8).
Also important was the gathering of input data (weather, fuel, topography) required to run simulators and observations of fire behaviour from historical wildland fires. Ideally, many wildfire cases would be available for this purpose. In reality, this is generally not the case. Although indicative, our dataset of 10 fire case studies was inadequate for drawing robust conclusions about simulator performance. High-quality field observations required for simulator evaluation are currently limited in type and quantity (Kelso et al. 2015; Filkov et al. 2018). Our observations were exclusively snapshots in time of fire perimeters obtained from fire agencies.
Uncertainty estimation for simulator inputs guides confidence in the results. We paid particular attention to uncertainty in weather data. The uncertainty estimates applied here and the one-at-a-time approach to sensitivity analysis are one way of catering for simulator input uncertainty. An alternative might employ a high-resolution weather ensemble forecasting system to provide a more dynamic and internally consistent picture of weather uncertainty. Such an approach may be favoured by fire behaviour analysts to estimate the probability of impact (e.g. Louis and Matthews 2015; Miller et al. 2015) and better inform risk-based decisions. The effect of (knowledge about) uncertainty in other inputs is also important. In particular, fuels may not be well characterised and variation in fuel between modelled and actual landscapes may substantially affect simulator outputs, as will variations in fuel load between modelled and actual fires, even if the fuel is well specified. We did not attempt to characterise errors arising from this source, and merely note that it is an additional contributing factor to background error in simulations.
Simulators were run for the historical fires and evaluation metrics calculated by comparing simulator predictions against observed fire behaviour. Our software of the framework developed for this purpose was implemented in the Python programming language with repeatability, reuse, flexibility and extensibility as key features. The intent of the framework development was that it would be available for simulator testing and comparison by the fire management community in Australia.
Visualisation of simulator evaluation metrics for analysis was the final component in the developed framework. We used several novel approaches to present results, including techniques drawn from weather and climate science, to address aspects of simulator performance important to a range of simulator users. Simple shaded plots provided a summary view in relative terms while performance diagrams provided greater detail. A modified Hinton diagram showed sensitivity to various perturbed simulator input variables and could be used as a means of comparing simulator performance across all case studies. As noted above, sensitivity to input parameters, or lack of it, is not in itself a useful characteristic of a simulator, unless actual fires are similarly sensitive. As users indicated initially, though, it is important to know whether a simulator is sensitive to sometimes imprecise or potentially inaccurate input data.
The current set of metrics and case studies formed an initial test bed for the fire research community, representing an example of a framework that could in future be routinely used to evaluate fire simulators prior to operational implementation. The metrics used address the questions about simulator performance initially posed by users, but other questions may arise over time, or on inspection of these and other case studies. In particular, users may wish to further investigate what constitutes a useful or good simulation. The currently used set of metrics provide simple, robust measures of aspects of simulator performance, such as the degree to which simulators burn the correct area, and simulate the rate at which fires progress. However, these measures were not able – using the available case study data – to distinguish whether any one simulator was superior to others. There may be other metrics that could be used in this framework to provide more nuanced information about simulator performance now or in future. In addition, it may be of value to weight particular measures more highly than others for some applications. Of course, a greater range of case study events would also be helpful in this respect.
Effort is required to collect routine measurements of fire behaviour to create a larger set of case studies to support future simulator development, testing and ongoing verification across a range of fire conditions and scenarios. This will permit more robust conclusions to be made and stratification of results by factors including fire danger rating or fuel type (Filkov et al. 2018). Extending observations to parameters including flame height and spotting distance would enable additional verification to be done using relevant metrics.
Ideally, all tested simulators should be subject to identical perturbations in this framework. We were unable to achieve this, as some tested simulators (Prometheus, Australis) could not be automated, and were therefore not subject to as extensive a range of testing as the remaining simulators.
Although not identified as key questions or outcomes in this evaluation project, establishing performance criteria and defining national data standards for simulator inputs and fire observation data would clearly be highly beneficial for future simulator development. As evaluation becomes more standard in simulator implementation, it is likely that the sorts of questions users ask of the evaluation process will become more focussed on particular aspects of performance, leading to further improvement, analogous to the development and refinement of numerical weather prediction in recent decades.
Data availability statement
Data and software that support this study are not currently publicly available but may be shared upon reasonable request to the corresponding author on approval from the Australian Bureau of Meteorology and AFAC.
Declaration of funding
This work was funded by AFAC and the New South Wales Rural Fire Service, with publication funding assistance from the Australian Climate Service.
Acknowledgements
The authors are grateful to the model developers who shared and discussed the application of their products, and to Australian fire agencies and their staff for supply of data and support. Comments from the anonymous reviewers and journal editorial staff helped clarify and improve the presentation of the manuscript.
References
Ager AA, Vaillant NM, Finney MA (2010) A comparison of landscape fuel treatment strategies to mitigate wildland fire risk in the urban interface and preserve old forest structure. Forest Ecology and Management 259, 1556-1570.
| Crossref | Google Scholar |
Allaire F, Filippi J-B, Mallet V (2020) Generation and evaluation of an ensemble of wildland fire simulations. International Journal of Wildland Fire 29, 160-173.
| Crossref | Google Scholar |
Allaire F, Filippi J-B, Mallet V, Vaysse F (2022) Simulation-based high-resolution fire danger mapping using deep learning. International Journal of Wildland Fire 31(4), 379-394.
| Crossref | Google Scholar |
Anderson WR, Cruz MG, Fernandes PM, McCaw L, Vega JA, Bradstock RA, Fogarty L, Gould J, McCarthy G, Marsden-Smedley JB, Matthews S, Mattingley G, Pearce HG, van Wilgen BW (2015) A generic, empirical-based model for predicting rate of fire spread in shrublands. International Journal of Wildland Fire 24(4), 443-460.
| Crossref | Google Scholar |
Arca B, Duce P, Laconi M, Pellizzaro G, Salis M, Spano D (2007) Evaluation of FARSITE simulator in Mediterranean maquis. International Journal of Wildland Fire 16, 563-572.
| Crossref | Google Scholar |
Bachmann A, Allgöwer B (2002) Uncertainty propagation in wildland fire behaviour modelling. International Journal of Geographical Information Science 16(2), 115-127.
| Crossref | Google Scholar |
Benali A, Ervilha AR, Sá ACL, Fernandes PM, Pinto RMS, Trigo RM, Pereira JMC (2016) Deciphering the impact of uncertainty on the accuracy of large wildfire spread simulations. Science of the Total Environment 569–570, 73-85.
| Crossref | Google Scholar | PubMed |
Bridge C (2015) Why are temperature forecasts from the Australian Digital Forecast Database poorer on summer afternoons? Australian Meteorological and Oceanographic Journal 65(2), 176-194.
| Crossref | Google Scholar |
Brotak EA (1980) A comparison of the meteorological conditions associated with a major wildland fire in the United States and a major bushfire in Australia. Journal of Applied Meteorology and Climatology 19(4), 474-476.
| Crossref | Google Scholar |
Bureau of Meteorology (2015) Australian Digital Forecast Database (ADFD) User Guide. Available at http://www.bom.gov.au/catalogue/adfdUserGuide.pdf
Bureau of Meteorology (2017) Bushfire Predictive Services Final Report: An evaluation of fire spread simulators used in Australia. Report to NSW Rural Fire Service and Australasian Fire and Emergency Service Authorities Council. Available at http://www.bom.gov.au/research/publications/otherreports/FPS_Final_Report_v1.81_Evaluation_Of_Simulators_Release.pdf
Burrows ND, Ward B, Robinson A (2009) Fuel dynamics and fire spread in spinifex grasslands of the Western Desert. In ‘Proceedings of the Royal Society of Queensland: Bushfire 2006 Conference’, 6–9 June 2006, Brisbane, Qld. (Ed. C Tran.) pp. 69–76. (Royal Society of Queensland Inc.: St Lucia, Qld, Australia)
Carmona-Moreno C, Belward A, Malingreau JP, Hartley A, Garcia‐Alegre M, Antonovskiy M, Buchshtaber V, Pivovarov V (2005) Characterizing interannual variations in global fire calendar using data from Earth observing satellites. Global Change Biology 11(9), 1537-1555.
| Crossref | Google Scholar |
Cheney NP (1976) Bushfire disasters in Australia, 1945–1975. Australian Forestry 39(4), 245-268.
| Crossref | Google Scholar |
Cheney NP, Gould JS, Catchpole WR (1998) Prediction of fire spread in grasslands. International Journal of Wildland Fire 8(1), 1-13.
| Crossref | Google Scholar |
Cheney NP, Gould JS, McCaw WL, Anderson WR (2012) Predicting fire behaviour in dry eucalypt forest in southern Australia. Forest Ecology and Management 280, 120-131.
| Crossref | Google Scholar |
Cruz MG, Alexander ME (2013) Uncertainty associated with model predictions of surface and crown fire rates of spread. Environmental Modelling & Software 47, 16-28.
| Crossref | Google Scholar |
Cruz MG, Sullivan AL, Gould JS, Sims NC, Bannister AJ, Hollis JJ, Hurley RJ (2012) Anatomy of a catastrophic wildfire: the Black Saturday Kilmore East fire in Victoria, Australia. Forest Ecology and Management 284, 269-285.
| Crossref | Google Scholar |
Cruz MG, McCaw WL, Anderson WR, Gould JS (2013) Fire behaviour modelling in semi-arid mallee-heath shrublands of southern Australia. Environmental Modelling & Software 40, 21-34.
| Crossref | Google Scholar |
Cruz MG, Gould JS, Alexander ME, Sullivan AL, McCaw WL, Matthews S (2015) Empirical-based models for predicting head-fire rate of spread in Australian fuel types. Australian Forestry 78(3), 118-158.
| Crossref | Google Scholar |
Cui W, Perera AH (2010) Quantifying spatio-temporal errors in forest fire spread modelling explicitly. Journal of Environmental Informatics 16(1), 19-26.
| Crossref | Google Scholar |
DeCastro A, Siems-Anderson A, Smith E, Knievel JC, Kosović B, Brown BG, Balch JK (2022) Weather research and forecasting – fire simulated burned area and propagation direction sensitivity to initiation point location and time. Fire 5, 58.
| Crossref | Google Scholar |
Doerr SH, Santín C (2016) Global trends in wildfire and its impacts: perceptions versus realities in a changing world. Philosophical Transactions of the Royal Society. Series B, Biological Sciences 371(1696), 20150345.
| Crossref | Google Scholar | PubMed |
Duff TJ, Chong DM, Taylor P, Tolhurst KG (2012) Procrustes based metrics for spatial validation and calibration of two-dimensional perimeter spread models: a case study considering fire. Agricultural and Forest Meteorology 160, 110-117.
| Crossref | Google Scholar |
Duff TJ, Chong DM, Tolhurst KG (2013) Quantifying spatio-temporal differences between fire shapes: estimating fire travel paths for the improvement of dynamic spread models. Environmental Modelling & Software 46, 33-43.
| Crossref | Google Scholar |
Duff TJ, Chong DM, Tolhurst KG (2016) Indices for the evaluation of wildfire spread simulations using contemporaneous predictions and observations of burnt area. Environmental Modelling & Software 83, 276-285.
| Crossref | Google Scholar |
Duff TJ, Cawson JG, Cirulis B, Nyman P, Sheridan GJ, Tolhurst KG (2018) Conditional performance evaluation: using wildfire observations for systematic fire simulator development. Forests 9(4), 189-204.
| Crossref | Google Scholar |
EM-DAT (2018) The Emergency Events Database – Université Catholique de Louvain (UCL) - CRED, D. Guha-Sapir. (Brussels, Belgium) Available at www.emdat.be
Filippi J-B, Mallet V, Nader B (2014) Representation and evaluation of wildfire propagation simulations. International Journal of Wildland Fire 23, 46-57.
| Crossref | Google Scholar |
Filkov A, Duff T, Penman T (2018) Improving fire behaviour data obtained from wildfires. Forests 9, 81.
| Crossref | Google Scholar |
Finney MA, Grenfell IC, McHugh CW, Seli RC, Trethewey D, Stratton RD, Brittain S (2011) A method for ensemble wildland fire simulation. Environmental Modeling & Assessment 16, 153-167.
| Crossref | Google Scholar |
Fujioka FM (2002) A new method for the analysis of fire spread modeling errors. International Journal of Wildland Fire 11(4), 193-203.
| Crossref | Google Scholar |
Giannaros TM, Lagouvardos K, Kotroni V (2020) Performance Evaluation of an operational rapid response fire spread forecasting system in the southeast Mediterranean (Greece). Atmosphere 11, 1264.
| Crossref | Google Scholar |
Giglio L, Randerson JT, van der Werf GR (2013) Analysis of daily, monthly, and annual burned area using the fourth‐generation global fire emissions database (GFED4). Journal of Geophysical Research: Biogeosciences 118(1), 317-328.
| Crossref | Google Scholar |
Gleckler PJ, Taylor KE, Doutriaux C (2008) Performance metrics for climate models. Journal of Geophysical Research: Atmospheres 113(D6), D06104.
| Crossref | Google Scholar |
Haas JR, Calkin DE, Thompson MP (2013) A national approach for integrating wildfire simulation modeling into wildland urban interface risk assessments within the United States. Landscape and Urban Planning 119, 44-53.
| Crossref | Google Scholar |
Hilton JE, Miller C, Sullivan AL, Rucinski C (2015) Effects of spatial and temporal variation in environmental conditions on simulation of wildfire spread. Environmental Modelling & Software 67, 118-127.
| Crossref | Google Scholar |
Johnston P, Kelso J, Milne GJ (2008) Efficient simulation of wildfire spread on an irregular grid. International Journal of Wildland Fire 17(5), 614-627.
| Crossref | Google Scholar |
Kelso JK, Mellor D, Murphy ME, Milne GJ (2015) Techniques for evaluating wildfire simulators via the simulation of historical fires using the Australis simulator. International Journal of Wildland Fire 24, 784-797.
| Crossref | Google Scholar |
Knight I, Coleman J (1993) A fire perimeter expansion algorithm-based on Huygens wavelet propagation. International Journal of Wildland Fire 3(2), 73-84.
| Crossref | Google Scholar |
Louis S, Matthews S (2015) Fire spread prediction using a lagged weather forecast ensemble. In ‘Proceedings of the 21st International Congress on Modelling and Simulation’, 29 November–4 December 2015, Gold Coast, Australia. (Eds T Weber, MJ McPhee, RS Anderssen) pp. 236–242. (Modelling and Simulation Society of Australia and New Zealand)
Marsden-Smedley JB, Catchpole WR (1995) Fire behaviour modelling in Tasmanian buttongrass Moorlands. II. Fire behaviour. International Journal of Wildland Fire 5, 215-228.
| Crossref | Google Scholar |
Miller C, Hilton J, Sullivan A, Prakash M (2015) SPARK – A bushfire spread prediction tool. In ‘Proceedings of the International Symposium on Environmental Software Systems 2015: Environmental Software Systems. Infrastructures, Services and Applications’. (Eds R Denzer, RM Argent, G Schimak, J Hřebíček) pp. 262–271. (Springer: Cham, Switzerland) doi:10.1007/978-3-319-15994-2_26
Mills GA (2005) A re-examination of the synoptic and mesoscale meteorology of Ash Wednesday 1983. Australian Meteorological Magazine 54(1), 35-55.
| Google Scholar |
Opperman T, Gould J, Finney M, Tymstra C (2006) Applying fire spread simulators in New Zealand and Australia: results from an international seminar. In ‘Fuels Management – How to Measure Success: Conference Proceedings’, 28–30 March 2006, Portland, OR. RMRS-P-41. (Eds PL Andrews, BW Butler) pp. 201–212. (USDA Forest Service, Rocky Mountain Research Station: Fort Collins, CO)
Owens D, O’Kane M (2020) ‘Final Report of the NSW Bushfire Inquiry’. 466 p. (Sydney, Australia) Available at https://www.dpc.nsw.gov.au/assets/dpc-nsw-gov-au/publications/NSW-Bushfire-Inquiry-1630/Final-Report-of-the-NSW-Bushfire-Inquiry.pdf [accessed 1 February 2023]
Ozaki M, Aryal J, Fox-Hughes P (2019) Dynamic wildfire navigation system. ISPRS International Journal of Geo-Information 8, 194.
| Crossref | Google Scholar |
Penman TD, Ababei DA, Cawson JG, Cirulis BA, Duff TJ, Swedosh W, Hilton JE (2020) Effect of weather forecast errors on fire growth model projections. International Journal of Wildland Fire 29, 983-994.
| Crossref | Google Scholar |
Pinto RM, Benali A, Sá AC, Fernandes PM, Soares PM, Cardoso RM, Trigo RM, Pereira JM (2016) Probabilistic fire spread forecast as a management tool in an operational setting. SpringerPlus 5(1), 1205-1228.
| Crossref | Google Scholar | PubMed |
Plucinski MP, Sullivan AL, Rucinski CJ, Prakash M (2017) Improving the reliability and utility of operational bushfire behaviour predictions in Australian vegetation. Environmental Modelling & Software 91, 1-12.
| Crossref | Google Scholar |
Roebber PJ (2009) Visualizing multiple measures of forecast quality. Weather and Forecasting 24, 601-608.
| Crossref | Google Scholar |
Russell-Smith J, Yates CP, Whitehead PJ, Smith R, Craig R, Allan GE, Thackway R, Frakes I, Cridland S, Meyer MC, et al. (2007) Bushfires 'Down Under': patterns and implications of contemporary Australian landscape burning. International Journal of Wildland Fire 16(4), 361-377.
| Crossref | Google Scholar |
Stretton LEB (1939) ‘Report of the Royal Commission to inquire into the causes of and measures taken to prevent the bush fires of January, 1939 and to protect life and property and the measures to be taken to prevent bush fires in Victoria and to protect life and property in the event of future bush fires.’ (Government Printer: Melbourne, Vic.). Available at http://royalcommission.vic.gov.au/getdoc/fbe8a952-b2fc-4346-9c60-805faab437d9/TEN.028.001.0001.pdf
Teague B, McLeod R, Pascoe S (2010) ‘2009 Victorian Bushfires Royal Commission Final Report.’ (Parliament of Victoria) Available at http://royalcommission.vic.gov.au/finaldocuments/summary/PF/VBRC_Summary_PF.pdf
Tolhurst K, Shields B, Chong D (2008) Phoenix: development and application of a bushfire risk management tool. Australian Journal of Emergency Management 23(4), 47.
| Google Scholar |
Tomašević IČ, Cheung KKW, Vučetić V, Fox-Hughes P (2022) Comparison of wildfire meteorology and climate at the Adriatic Coast and southeast Australia. Atmosphere 13, 755.
| Crossref | Google Scholar |