Wildland fire prevention: the impact of the Modifying Industrial Operations Protocol on the growth of industrial forestry-caused wildland fires in Ontario, Canada
Kevin Granville
A Department of Mathematics and Statistics, University of Windsor, Windsor, ON, Canada.
B Department of Statistical and Actuarial Sciences, University of Western Ontario, London, ON, Canada.
C Department of Statistics and Actuarial Science, University of Waterloo, Waterloo, ON, Canada.
D Ontario Ministry of Natural Resources and Forestry, Aviation, Forest Fire and Emergency Services, Dryden, ON, Canada.
International Journal of Wildland Fire 31(9) 825-834 https://doi.org/10.1071/WF22074
Submitted: 4 November 2021 Accepted: 19 July 2022 Published: 15 August 2022
© 2022 The Author(s) (or their employer(s)). Published by CSIRO Publishing on behalf of IAWF. This is an open access article distributed under the Creative Commons Attribution-NonCommercial-NoDerivatives 4.0 International License (CC BY-NC-ND)
Abstract
Background: Industrial forestry operations in Ontario, Canada, may be restricted to reduce the risk of wildland fires. This is currently done according to the Modifying Industrial Operations Protocol (MIOP), which was implemented in 2008 as a replacement for the Woods Modification Guidelines that had been in place since 1989. One of MIOP’s objectives is to limit the negative impact or damage caused by fires ignited by industrial forestry operations.
Aims: Treating the incremental growth between discovery and final sizes as a measure of suppression effectiveness, we aimed to characterise and contrast growth distributions for three successive time periods using data spanning 1976–2019 on Crown forest areas of Ontario.
Methods: Stratifying by first responding group (Ontario Ministry vs forestry personnel), we tested for evidence of changes in the growth distribution using the Kruskal–Wallis and Mann–Whitney U tests.
Key results: We found iterative improvements between successive time periods (Pre-Woods, then Woods Guidelines, then MIOP) in the growth distribution of fires first responded to by forestry personnel.
Conclusions: MIOP appears to be successfully limiting the negative impact of industrial forestry fires while increasing operational flexibility relative to the Woods Modification Guidelines.
Implications: MIOP has been implemented in a manner that still encourages safe operations while not contradicting this objective.
Keywords: empirical cumulative distribution function, fire growth, fire size, forest fire, initial response group, Kruskal–Wallis test, Mann–Whitney U test, regulations, wildfire risk mitigation.
Introduction
Wildland fires can occur where there are receptive fuels and a source of ignition. In Ontario, Canada, fires are ignited by lightning strikes (Wotton and Martell 2005) or human activities including, but not limited to, recreation, railways, arson, or industrial forestry (Woolford et al. 2021). Wildland fires are a natural part of Canada’s forests, with both negative impacts (e.g. to public health and human infrastructure) and benefits (e.g. healthy natural ecosystems, removal of hazardous fuels). For a thorough summary and discussion of wildland fire impacts and risk in Canada, see the recent review paper by Johnston et al. (2020) and the references therein. These impacts, in combination with highly variable fire numbers, locations, and sizes, create many challenging decisions (Boychuk et al. 2020) and demonstrate the necessity of strategic fire management to resolve these complex and interacting factors (Martell 2015).
Fire management agencies take a risk-based approach through the detection of new fires (McFayden et al. 2019), suppression of high-risk fires (Hirsch et al. 2004; Martell and Sun 2008), prevention of human-caused fires (Hesseln 2018), and mitigation of fire impacts (Beverly et al. 2020; FireSmart Canada 2022). Effective initial attack suppression systems are associated with an increased likelihood of fires remaining small (Arienti et al. 2006; Martell and Sun 2008; Cardil et al. 2019). However, less is known about the effectiveness of prevention efforts. which is required to make both operational (e.g. prevention actions on days with higher fire hazards) and strategic decisions (e.g. how much to invest in prevention vs response efforts).
Fire prevention research covers a wide range of topics; for a recent and thorough review of the literature see Hesseln (2018). Outside of Canada, research has been conducted on the effectiveness of wildland fire prevention programs and education efforts to lower human-caused ignitions (Butry et al. 2010a, 2010b; Prestemon et al. 2010; Thomas et al. 2013; Abt et al. 2015; Butry and Prestemon 2019), as well as the effectiveness of fuel management and treatments in reducing the impact of wildland fires that do ignite (Scott 2006; Price and Bradstock 2012; Ruiz-Mirazo 2012; Marino et al. 2014; Varela et al. 2014; Labossière and McGee 2017), whereas in the Canadian context, there has been less research done on prevention. Some examples of literature about the government’s efforts on wildland fire prevention in Canada include the following: Cumming (2005), who investigated the impact of a 1983 change in Alberta’s management strategy for initial attacks on the probability of a fire escaping or becoming large in northern Alberta; Grunstra and Martell (2014), who provided an overview of the history of how policy and technological changes have reduced railway fire frequency; and Labossière and McGee (2017), who considered the impact of municipal government-level fire mitigation measures in the province of British Colombia. The need to expand Canadian wildland fire prevention research in general was recently noted in the Blueprint for Wildland Fire Science in Canada (Sankey 2018), which was developed by the government of Canada through extensive consultations as a guide to key research questions that need consideration in Canada. Consequently, our work is timely, as our analyses of the effectiveness of forestry regulations fall within this scope.
Some regulatory approaches are taken by Ontario’s Ministry of Natural Resources and Forestry (MNRF, hereafter the ‘Ministry’) to prevent human-caused fires. These include temporary fire bans in Restricted Fire Zones, within which it is illegal to have an outdoor fire with few exceptions. These kinds of fire bans are in put in place during high-hazard periods and generally target fires caused by residential or recreational activities such as campfires and grass burning. Another approach to prevention is through the Modifying Industrial Operations Protocol (MIOP). which aims to prevent and mitigate industrial forestry-caused (IDF) wildland fires. These IDF fires may be ignited by harvesting activities such as sparks from logging equipment, power saws, or hot work (among others).
Ontario has a large forest industry (Invest Ontario 2021). Both the forest industry and government have a mutual interest of ensuring forestry operations are conducted in a safe manner to limit the inherent wildland fire risk from IDF fires. This is crucial, not only in terms of ensuring the safety of industry workers and equipment, but also to protect natural resources and mitigate the risk of potential broader scale impacts should an IDF fire grow large. The Ministry released the Woods Modification Guidelines (Woods Guidelines) in 1989 to improve fire mitigation efforts for IDF fires, providing the forestry industry with guidance on how to modify operations daily, based on fire risk conditions. In our analyses, we refer to the time prior to 1989 as the Pre-Woods period. In consultation with the forest industry in 2003, the Ministry developed and tested a new set of regulations from 2004 to 2006. Those regulations eventually became the Modifying Industrial Operations Protocol (MIOP), which was partially implemented in 2007 and fully implemented across Ontario in 2008. MIOP was developed with the goal of meeting the following objectives (OMNR 2011):
Industrial operations are conducted in a manner that prevents wildfires from starting and does not increase the seasonal forest fire load;
Wildfires are detected and reported;
Industrial operators continue to work safely as long as possible as the fire danger increases; and
Employees are adequately trained to use available equipment to take safe action that will reduce the negative impact or damage from a fire, should one occur.
Due to the Crown Forest Sustainability Act (Canada, Government of Ontario 1994), adherence to MIOP is a legislative requirement of a sustainable forest license (SFL). The company that holds the SFL for a forest must produce a forest management plan that includes stipulations to follow MIOP through the defined fire season (1 April–31 October). When IDF fires do occur, they are most frequently first responded to by either the forestry personnel whose company holds the SFL for the use of the Crown land (whom we refer to as the licensee in our analyses), or by Ministry firefighters (e.g. to effectively handle larger or more active fires). To aid in licensee response to fires, MIOP regulations set minimums on quantities of and maximums on distances from fire suppression equipment (e.g. backpack pumps, fire equipment caches), which may vary depending on the deemed risk of an operation (OMNR 2011).
MIOP was designed to be less restrictive than the Woods Guidelines in terms of allowing operations to continue under increasing fire danger. However, as listed in the fourth objective of MIOP, it also requires that industry personnel be trained to limit fire impacts should a fire occur, and it also may shorten, further restrict, or even shut down operations when risk increases. The relationship between MIOP’s restrictions on hours of operation and operational risk of ignition, fire risk related to fuels and weather (summarised by a Fire Intensity Code), and the fire suppression training level of forestry personnel (‘Limited Operators’ vs ‘Trained and Capable’), is illustrated in Table 1.
![]() |
Recently, Granville et al. (2022) examined the impact of industrial forestry prevention efforts on the frequency of IDF fires in Ontario using a case-crossover approach. Although MIOP has increased flexibility in operating hours, they concluded that there was no evidence of an increased probability of IDF fire occurrences. Here, our interest is to examine the impact of such prevention efforts on another characteristic of IDF fires. Specifically, our goal is to determine whether these prevention efforts aimed specifically at reducing the risk of IDF fires in Ontario may have influenced suppression effectiveness, focusing on the growth distributions of IDF fires.
Here, growth refers to the incremental change in size from discovery size to final size – this differs from past research modelling fire size distributions, such as studies involving the fitting of parametric models including those following the power law (Malamud et al. 1998; Cui and Perera 2008; Hantson et al. 2015; Wang et al. 2020) or assuming other distributional families (Cumming 2001; Reed and McKelvey 2002; Schoenberg et al. 2003; Marchal et al. 2017; Maffei and Menenti 2019) – specifically, we characterise the growth distributions during the MIOP, Woods Guidelines and Pre-Woods time periods, stratifying by whether the fire was first responded to by the licensee or the Ministry. Then, we employ statistical methods to test each initial response group for evidence of differences in growth across these time periods. In what follows, we find evidence of less growth between successive time periods for fires that were first responded to by forestry personnel, and no evidence of a change in distribution is detected for those fires first responded to by Ministry. First, we elaborate on the methods used for our empirical study.
Methods
Data
We analyse historical wildland fire records in Ontario, provided to us by the Ministry of Natural Resources and Forestry. This archive contains an array of information describing these fires, including but not limited to the spatial coordinates of where a fire was ignited, when a fire was ignited, reported, attacked, and extinguished, the weather and fuel conditions at the time and location of ignition, the cause of ignition, the estimated size at discovery, and the final size. Given our focus, we restrict our analysis solely to IDF fires. Our study period is 1976–2019. (Data from 2020 onwards were omitted due to potential confounding effects of the covid-19 pandemic.) During this 44-year window, a total of 1175 of the 61 862 wildland fires that occurred in Ontario were IDF fires ignited by the industrial forestry industry. Because MIOP is only enforced during the operational fire season (1 April–31 October), we omitted 14 data points corresponding to IDF fires ignited outside of these months. Furthermore, because our interest lies in distributions of IDF fires first responded to by Ministry or licensee groups, we filtered out those IDF fires responded to by others, reducing our data set to 983 fires.
Ontario’s Crown forest is separated into several forest management units (FMUs); see Fig. 1. These FMUs are used to aid in the organisation and enforcement of approved forest management plans. Consequently, we restrict our study region to the Crown forest areas of Ontario where forest operations are carried out under a forest management plan and MIOP is enforced. Removing IDF fires that were not ignited on Crown land brings us to our final count of 934 fires, of which 142 occurred under MIOP, 507 occurred under Woods Guidelines, and 285 occurred during Pre-Woods. Among these are three IDF fires that were recorded as being ignited on Crown land but were outside of our study region. However, we elected to retain them as they were within 5 km of the boundary, and it was reasonable to assume they had minor errors in their recorded positions because harvesting would not occur where they were reported to have ignited (one in Lake Superior and two in Wabakimi Provincial Park). Additionally, two of the 934 IDF fires had recorded final sizes that were smaller than their discovery sizes. However, because discovery size is estimated, their discovery sizes were adjusted to equal their final sizes, resulting in zero growth for such fires.
Because MIOP underwent a phased implementation, we make use of the FMUs to distinguish between IDF fires ignited under MIOP or Woods Guidelines during years when both were being enforced in different parts of the province. In 2006 and 2007, MIOP was tested in two and six FMUs respectively. There were no IDF fires ignited within these two MIOP FMUs in 2006, so all IDF fires ignited in 2006 are classified as having occurred under the Woods Guidelines. Conversely, in 2007, there was a mix of IDF fires ignited within Woods Guidelines and MIOP FMUs, as visualised in Fig. 1, that we have classified accordingly. Note that FMUs do change over time, and one of the MIOP FMUs from 2007 has since been merged with another FMU that had operated under Woods Guidelines that year. No IDF fires were ignited in either of those areas in 2007, so it was unnecessary to obtain the exact spatial boundaries from that year to aid in classification. However, we use an intermediate shade of grey in Fig. 1 to represent the fact that there was a mix of both sets of regulations across that area in 2007. Because MIOP was fully implemented in 2008, all IDF fires from 2008 onwards are considered to have been ignited under MIOP.
Testing for differences in IDF fire growth distributions
To test for the presence of distributional changes across time periods, we make use of the Kruskal–Wallis (KW) test (Kruskal and Wallis 1952). This is a non-parametric procedure that can test the null hypothesis that two or more samples are from the same distribution. It allows for the simultaneous consideration of our three samples of observed IDF fire growth values from the MIOP, Woods Guidelines, and Pre-Woods time periods. If the KW test rejects its null hypothesis, then this is evidence that at least one time period had a different distribution of growth from the others. Details on how to conduct the KW test appear in Appendix A.
Although the KW test can identify the presence of differences, it does not indicate which specific distributions are different. Should a KW test find evidence of differences, we next employ the Mann–Whitney U (MWU) test (Mann and Whitney 1947) in a pairwise fashion to directly test one time period against another. These tests link well with each other, because the MWU test is equivalent to the KW test for two samples, so it is testing using similar approaches whether two samples are from the same or different distributions. To account for the fact that conducting multiple comparisons increases the likelihood of incorrectly rejecting a true null hypothesis, we adjust the pairwise MWU P-values using the conservative Bonferroni adjustment (e.g. Goeman and Solari 2014) to avoid an inflated type I error rate. In our case, because we require a set of three pairwise tests, this adjustment multiplies our P-values by a factor of three (i.e. the number of tests). Should an adjusted P-value still be small enough, we can safely conclude that there is sufficient evidence to reject a null hypothesis and declare that two time periods’ growth distributions are different.
Empirical cumulative distribution functions
Should the MWU test conclude that two growth distributions are different, visual inspections of empirical estimates of the distributions can be employed to identify which time period tended to observe ‘bigger’ or ‘smaller’ amounts of growth. We make use of histograms and empirical cumulative distribution functions (ECDFs) for this purpose.
An ECDF is a function describing a sample of data that when evaluated at a value x, is equal to the proportion of observations in the sample whose values are less than or equal to x. If we let x1, x2, …, xN denote the sample of N growth values for a given prevention time period and define the indicator function
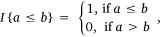
then we may formally express the ECDF evaluated at x, FN(x), for that time period as
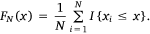
Suppose that GM(x) is the ECDF for a second time period having M observations. If FN(y) > GM(y) for some number y, this means that a larger proportion of the fires from the first time period experienced growth that was less than or equal to y. If this inequality holds true over most of the entire range of observed growth values, then we can conclude that the first time period tended to have smaller sizes of growth.
Software
The statistical software R (R Core Team 2021) was used to compute all calculations and create all visualisations. The functions kruskal.test() and pairwise.wilcox.test() from the package ‘stats’ were used to conduct the KW and pairwise MWU tests respectively. The function ecdf(), also from the ‘stats’ package, was used to calculate ECDFs, whereas ggplot() and other functions found within the ‘tidyverse’ group of packages were used to plot the histograms and ECDFs in Fig. 2 as well as the scatter plots in Fig. 3. In addition to ‘tidyverse’, the package ‘sf’ was required to manage the spatial data and geometries used to create Fig. 1.
Results
Testing for differences in growth distributions
The results from the KW test appear in Table 2. For IDF fires first responded to by the Ministry, the P-value is very large, implying that there is no evidence of any changes in the growth distribution. In contrast, for those IDF fires first responded to by a licensee group, there is very strong evidence (i.e. a very small and significant P-value) to suggest that there has been at least one such change in the growth distribution across the time periods of our study.
![]() |
The results of the MWU tests for differences in the distributions of licensee-responded IDF fires are also presented in Table 2. The P-values for these tests were modified using the Bonferroni adjustment to account for multiple comparisons. The adjusted P-values are all still small (i.e. P-value <0.05), with the P-value resulting from the test of MIOP vs Pre-Woods (P-value <0.001) notably being several orders of magnitude smaller than the others (P-value = 0.028 for MIOP vs Woods Guidelines; P-value = 0.013 for Woods Guidelines vs Pre-Woods). As such, we have evidence of changes in the growth distribution between each time period, and in particular, the evidence of differences between the Pre-Woods and MIOP is very strong.
Interpreting test results with ECDFs
Fig. 2 visualises the distributions of our samples of IDF fire growth values using ECDFs. Histograms are also included to aid in interpretation. These plots are presented for each of the three time periods and both Ministry and licensee responding groups. One interesting feature of both the final size and discovery size empirical distributions are the heights of their initial jumps at 0.1 ha. This is a consequence of both rounding and digit preference. For example, any fire sizes below 0.1 ha were recorded as 0.1 ha in the historical fire archive. It is the most frequently observed value for both final and discovery sizes of IDF fires. Other sizes (e.g. multiples of 0.1, 0.5, or 1 ha) may also appear numerous times due to rounding and digit preference, albeit at a lower frequency, which results in varying sizes of vertical jumps in the ECDFs.
Given the KW and MWU tests’ P-values in Table 2, we concluded that there were no changes in growth distribution between time periods for IDF fires responded to by the Ministry while there were changes between each time period for those responded to by a licensee. It is therefore unsurprising that the three ECDF plots for Ministry-responded IDF fires experience large overlaps and do not contradict the possibility that they could be representing samples from the same distribution.
Conversely, the ECDF plots for each time period for licensee-responded IDF fires are all distinctly different, reflecting the conclusions of the MWU tests. For instance, 92.3, 78.7, and 65.4% of IDF fires experienced zero growth under MIOP, Woods Guidelines, and Pre-Woods respectively. This means that fires were approximately 2.77 and 4.50 times as likely to grow beyond their discovery sizes under Woods Guidelines and Pre-Regulations relative to what was observed to occur under MIOP. This results in a much larger vertical separation in the ECDFs, especially near smaller growth values. In fact, there is a clear separation between our ECDF plots with no intersections, with MIOP’s ECDF above the ECDF for the Woods Guidelines, which is itself above Pre-Woods’s ECDF. Consequently, IDF fires ignited under MIOP tended to have smaller values of growth than Woods Guidelines, which tended to have smaller values of growth than Pre-Woods.
Discussion
We investigated the relative impacts of MIOP, Woods Guidelines, and Pre-Woods as prevention efforts for IDF fires ignited on Crown land in Ontario over a 44-year period. We analysed suppression effectiveness, which was measured as the incremental change between discovery and final sizes (that we refer to as growth) of IDF fires. The majority of IDF fires are first responded to by either the Ministry or the licensee, so we conducted separate analyses for these two groups. To help justify our choice to analyse growth over final size, Fig. 3 presents a scatterplot of final vs discovery sizes of IDF fires broken down by response group and time period. Because some pairs of these sizes occur multiple times, the size of each point is scaled according to its observed frequency in the data. Points above the 45-degree line correspond to fires that grew beyond their discovery size before being declared as held. Within these plots, there is a notable decrease in variation of final size for fires responded to by a licensee when compared with those responded to by the Ministry, which may be related to the fact that Ministry personnel need to travel to the job site to suppress an IDF fire (allowing more time to grow before initial attack) and that a licensee group would be more inclined to call the Ministry for support to attack larger and/or more aggressive fires. As is immediately evident, it is frequently possible to explain much of a IDF fire’s final size based solely on its size when discovered. Because MIOP wants to limit the negative impact or damage of fires that ignite, and because it is logical to say that a fire discovered at 10 ha and kept at 10 ha was more successfully suppressed than a fire discovered at 1 ha that grew to 5 ha, we elected to measure suppression effectiveness by the incremental growth in fire size between when it is discovered and its final size. Simply put, incremental growth adjusts for the varying sizes at discovery of IDF fires.
The growth distributions for each combination of time period and response group are visualised in Fig. 2, and were tested for statistically significant differences across time periods using the Kruskal–Wallis test and pairwise Mann–Whitney U tests, where the latter was employed when the Kruskal–Wallis test returned evidence of at least one change in distribution. Both the Ministry and license groups are suppressing IDF fires that are ignited under similar circumstances (i.e. ignited in areas where harvesting is happening by a source related to forestry operations). However, their individual suppression capabilities differ. The Ministry has dedicated firefighters who have training and equipment capable of attacking large fires successfully. In contrast, forestry personnel are not as specialised in fire suppression and have their own suppression equipment on hand (e.g. water pumps, shovels). Therefore, regulations requiring suppression equipment to be available on the job site or for a certain percentage of forestry personnel to be trained in fire suppression (for the crew to be considered ‘Trained and Capable’ as opposed to ‘Limited Operators’, to potentially lessen MIOP’s restrictions on hours of operation) should only impact the suppression capabilities of the licensee.
The KW and pairwise MWU tests found evidence that the distribution of growth had changed between each time period for IDF fires first responded to by the licensee (Table 2). From visual inspection of the ECDFs in Fig. 2, we can conclude that this distribution has ‘improved’ in each successive time period, with IDF fires ignited under MIOP tending to experience the smallest growth values, followed by Woods Guidelines and then Pre-Woods. From Fig. 3, it is evident that under MIOP, licensee groups have only attacked IDF fires whose discovery sizes were around 1 ha or smaller. It is therefore possible that MIOP has succeeded in improving a licensee’s ability to attack smaller fires (due to a combination of training and access to appropriate suppression equipment). Assuming a licensee can respond to a smaller fire faster than the Ministry – which is reasonable because Ministry personnel would have to travel to the point of the fire whereas the licensee personnel are already in that area – these results are consistent with past findings studying initial attack success that found faster response times were associated with an increased probability of initial attack success, defined as a smaller fire not growing beyond an ‘escape’ threshold size (Arienti et al. 2006; Cardil et al. 2019). From the data available, it is unclear whether MIOP has improved a licensee’s ability to respond to fires discovered at larger sizes. Note that if we repeat this analysis for licensee-responded IDF fires with discovery sizes of 1 ha or less (i.e. omitting those fires with larger discovery sizes), the KW and pairwise MWU tests’ P-values increase slightly, but we still retain approximately the same levels of evidence and arrive at the same conclusions.
No differences in growth distribution were detected by the KW test for IDF fires first responded to by Ministry personnel (Table 2). There were also no notable differences in the Ministry’s ECDFs plotted in Fig. 2. This is not entirely surprising, because their suppression efforts would not feel the impact of MIOP’s regulations related to its fourth objective pertaining specifically to the capability of forestry personnel working on site. However, it is also important to note that this distribution has not become worse because of the increased flexibility in operating conditions resulting from MIOP’s third objective. It is most likely that either the possible allowance of working in riskier conditions has not resulted in the ignition of larger fires, or that advancements in the Ministry’s own suppression methods and equipment have been sufficient to counteract any such changes. Therefore, this flexibility appears to have been implemented in a reasonable and safe manner.
Other factors beyond response group and the set of operational regulations can impact IDF fire growth distributions. For instance, local weather and fuel buildup should influence both ignition probability and how fast an ignited fire may spread (and therefore how much it will grow). However, because MIOP takes both weather and fuel into consideration when determining a day’s restrictions (Table 1), we would not consider either as possible unmeasured confounders. Specifically, if MIOP tended to observe smaller amounts of growth due to operations often being conducted in ‘less risky’ situations, then this outcome reflects MIOP working as intended, because the licensee is incentivised to work in safer conditions to avoid restrictions on hours of operation, and thus can be credited as an impact of MIOP itself. Some other influential variables include the advancement of fire detection, suppression methods, and equipment, or changes in harvesting practices and equipment over time. Unfortunately, because many of these improvements would not be considered direct consequences of regulation changes, and because we have no controls for these in our tests, they may be considered potential confounders. As such, some improvements (or absence of worsening) over time that we detected may be in part due to these advancements rather than solely the regulations in isolation. Future work would benefit from considering statistical methods such as quantile regression, which could explicitly control for additional variables and be used to demonstrate a more causal relationship between the sets of regulations and changes in distribution.
Conclusions
Evidence suggests that IDF fires responded to by the licensee do not experience as much growth after discovery as often under MIOP relative to the previous prevention time period of Woods Guidelines, or the Pre-Woods time period. Conversely, there is no evidence of any changes in the distribution of IDF fire growth over the same time periods for fires responded to by the Ministry. This indicates that licensee group suppression efforts have become more effective alongside the enforcement of regulations, improving their ability to work safely and protect themselves and their equipment from the risk of accidental ignitions. Although advancements in technology and methodology are possible explanations for some of the improvements (or absence of worsening) over time, we nevertheless fail to observe evidence of these distributions worsening as a consequence of MIOP’s possible allowance of working in conditions with increased fire hazard compared with Woods Guidelines. Therefore, it would appear that MIOP’s flexibility in its restrictions of daily hours of operations (OMNR 2011) have been implemented in a manner that still encourages safe operations, and does not contradict its objective of limiting the negative impact of IDF fires that do ignite.
Data availability
The datasets used for our analyses are subject to copyright by the Queen’s Printer for Ontario as represented by the Ontario Ministry of Natural Resources and Forestry, and were used under licence.
Conflicts of interest
The authors declare no conflicts of interest.
Declaration of funding
This research did not receive any specific funding.
Acknowledgements
We acknowledge the support of the Natural Sciences and Engineering Research Council of Canada (NSERC), the Institute for Catastrophic Loss Reduction, and the Ontario Ministry of Natural Resources and Forestry (MNRF), who also provided data for this study. We thank the following individuals from the MNRF for their contributions of helpful guidance and advice: P. Chandler, D. Johnson, D. Johnston, M. Pistilli and L. Watkins. We also thank S. Y. Cao for her assistance in formatting the manuscript in preparation for submission.
References
Abt KL, Butry DT, Prestemon JP, Scranton S (2015) Effect of fire prevention programs on accidental and incendiary wildfires on tribal lands in the United States. International Journal of Wildland Fire 24, 749–762.| Effect of fire prevention programs on accidental and incendiary wildfires on tribal lands in the United States.Crossref | GoogleScholarGoogle Scholar |
Arienti MC, Cumming SG, Boutin S (2006) Empirical models of forest fire initial attack success probabilities: the effects of fuels, anthropogenic linear features, fire weather, and management. Canadian Journal of Forest Research 36, 3155–3166.
| Empirical models of forest fire initial attack success probabilities: the effects of fuels, anthropogenic linear features, fire weather, and management.Crossref | GoogleScholarGoogle Scholar |
Beverly JL, Leverkus SER, Cameron H, Schroeder D (2020) Stand-level fuel reduction treatments and fire behaviour in Canadian boreal conifer forests. Fire 3, 35
| Stand-level fuel reduction treatments and fire behaviour in Canadian boreal conifer forests.Crossref | GoogleScholarGoogle Scholar |
Boychuk D, McFayden CB, Evens J, Shields J, Stacey A, Woolford DG, Wotton M, Johnston D, Leonard D, McLarty D (2020) Assembling and customizing multiple fire weather forecasts for burn probability and other fire management applications in Ontario, Canada. Fire 3, 16
| Assembling and customizing multiple fire weather forecasts for burn probability and other fire management applications in Ontario, Canada.Crossref | GoogleScholarGoogle Scholar |
Butry DT, Prestemon JP (2019) Economics of WUI/Wildfire Prevention and Education. In ‘Encyclopedia of Wildfires and Wildland–Urban Interface (WUI) Fires’. (Eds SL Manzello) pp. 1–8. (Springer: Cham, Switzerland)
| Crossref |
Butry DT, Prestemon JP, Abt KL (2010a) Optimal timing of wildfire prevention education. WIT Transactions on Ecology and the Environment 137, 197–206.
| Optimal timing of wildfire prevention education.Crossref | GoogleScholarGoogle Scholar |
Butry DT, Prestemon JP, Abt KL, Sutphen R (2010b) Economic optimisation of wildfire intervention activities. International Journal of Wildland Fire 19, 659–672.
| Economic optimisation of wildfire intervention activities.Crossref | GoogleScholarGoogle Scholar |
Cardil A, Lorente M, Boucher D, Boucher J, Gauthier S (2019) Factors influencing fire suppression success in the province of Quebec (Canada). Canadian Journal of Forest Research 49, 531–542.
| Factors influencing fire suppression success in the province of Quebec (Canada).Crossref | GoogleScholarGoogle Scholar |
Cui W, Perera AH (2008) What do we know about forest fire size distribution, and why is this knowledge useful for forest management. International Journal of Wildland Fire 17, 234–244.
| What do we know about forest fire size distribution, and why is this knowledge useful for forest management.Crossref | GoogleScholarGoogle Scholar |
Cumming SG (2001) A parametric model of the fire-size distribution. Canadian Journal of Forest Research 31, 1297–1303.
| A parametric model of the fire-size distribution.Crossref | GoogleScholarGoogle Scholar |
Cumming SG (2005) Effective fire suppression in boreal forests. Canadian Journal of Forest Research 35, 772–786.
| Effective fire suppression in boreal forests.Crossref | GoogleScholarGoogle Scholar |
FireSmart Canada (2022) FireSmart Canada. Available at https://firesmartcanada.ca/ [Verified 5 March 2022]
Goeman JJ, Solari A (2014) Multiple hypothesis testing in genomics. Statistics in Medicine 33, 1946–1978.
| Multiple hypothesis testing in genomics.Crossref | GoogleScholarGoogle Scholar |
Government of Ontario (1994) Crown Forest Sustainability Act, Revised Statutes of Canada. c. 25. Available at https://www.ontario.ca/laws/statute/94c25 [Verified 5 March 2022]
Granville K, Woolford DG, Dean CB, McFayden CB (2022) A case-crossover study of the impact of the Modifying Industrial Operations Protocol on the frequency of industrial forestry-caused wildland fires in Ontario, Canada. Journal of Agricultural, Biological and Environmental Statistics
| A case-crossover study of the impact of the Modifying Industrial Operations Protocol on the frequency of industrial forestry-caused wildland fires in Ontario, Canada.Crossref | GoogleScholarGoogle Scholar |
Grunstra MR, Martell DL (2014) A history of railway fires in Ontario’s forests. The Forestry Chronicle 90, 314–320.
| A history of railway fires in Ontario’s forests.Crossref | GoogleScholarGoogle Scholar |
Hantson S, Pueyo S, Chuvieco E (2015) Global fire size distribution is driven by human impact and climate. Global Ecology and Biogeography 24, 77–86.
| Global fire size distribution is driven by human impact and climate.Crossref | GoogleScholarGoogle Scholar |
Hesseln H (2018) Wildland fire prevention: a review. Current Forestry Reports 4, 178–190.
| Wildland fire prevention: a review.Crossref | GoogleScholarGoogle Scholar |
Hirsch KG, Podur JJ, Janser RF, McAlpine RS, Martell DL (2004) Productivity of Ontario initial-attack fire crews: results of an expert-judgement elicitation study. Canadian Journal of Forest Research 34, 705–715.
| Productivity of Ontario initial-attack fire crews: results of an expert-judgement elicitation study.Crossref | GoogleScholarGoogle Scholar |
Invest Ontario (2021) Forestry. Available at https://www.investontario.ca/forestry [Verified 5 March 2022]
Johnston LM, Wang X, Erni S, Taylor SW, McFayden CB, Oliver JA, Stockdale C, Christianson A, Boulanger Y, Gauthier S, Arseneault D, Wotton BM, Parisien MA, Flannigan MD (2020) Wildland fire risk research in Canada. Environmental Reviews 28, 164–186.
| Wildland fire risk research in Canada.Crossref | GoogleScholarGoogle Scholar |
Kruskal WH, Wallis WA (1952) Use of ranks in one-criterion variance analysis. Journal of the American Statistical Association 47, 583–621.
| Use of ranks in one-criterion variance analysis.Crossref | GoogleScholarGoogle Scholar |
Labossière LMM, McGee TK (2017) Innovative wildfire mitigation by municipal governments: two case studies in Western Canada. International Journal of Disaster Risk Reduction 22, 204–210.
| Innovative wildfire mitigation by municipal governments: two case studies in Western Canada.Crossref | GoogleScholarGoogle Scholar |
Maffei C, Menenti M (2019) Predicting forest fires burned area and rate of spread from pre-fire multispectral satellite measurements. ISPRS Journal of Photogrammetry and Remote Sensing 158, 263–278.
| Predicting forest fires burned area and rate of spread from pre-fire multispectral satellite measurements.Crossref | GoogleScholarGoogle Scholar |
Malamud BD, Morein G, Turcotte DL (1998) Forest fires: an example of self-organized critical behavior. Science 281, 1840–1842.
| Forest fires: an example of self-organized critical behavior.Crossref | GoogleScholarGoogle Scholar |
Mann HB, Whitney DR (1947) On a test of whether one of two random variables is stochastically larger than the other. The Annals of Mathematical Statistics 18, 50–60.
| On a test of whether one of two random variables is stochastically larger than the other.Crossref | GoogleScholarGoogle Scholar |
Marchal J, Cumming SG, McIntire EJB (2017) Land cover, more than monthly fire weather, drives fire-size distribution in Southern Québec forests: implications for fire risk management. PLoS One 12, e0179294
| Land cover, more than monthly fire weather, drives fire-size distribution in Southern Québec forests: implications for fire risk management.Crossref | GoogleScholarGoogle Scholar |
Marino E, Hernando C, Planelles R, Madrigal J, Guijarro M, Sebastián A (2014) Forest fuel management for wildfire prevention in Spain: a quantitative SWOT analysis. International Journal of Wildland Fire 23, 373–384.
| Forest fuel management for wildfire prevention in Spain: a quantitative SWOT analysis.Crossref | GoogleScholarGoogle Scholar |
Martell DL (2015) A review of recent forest and wildland fire management decision support systems research. Current Forestry Reports 1, 128–137.
| A review of recent forest and wildland fire management decision support systems research.Crossref | GoogleScholarGoogle Scholar |
Martell DL, Sun H (2008) The impact of fire suppression, vegetation, and weather on the area burned by lightning-caused forest fires in Ontario. Canadian Journal of Forest Research 38, 1547–1563.
| The impact of fire suppression, vegetation, and weather on the area burned by lightning-caused forest fires in Ontario.Crossref | GoogleScholarGoogle Scholar |
McFayden CB, Woolford DG, Stacey A, Boychuk D, Johnston JM, Wheatley MJ, Martell DL (2019) Risk assessment for wildland fire aerial detection patrol route planning in Ontario, Canada. International Journal of Wildland Fire 29, 28–41.
| Risk assessment for wildland fire aerial detection patrol route planning in Ontario, Canada.Crossref | GoogleScholarGoogle Scholar |
OMNR (2011) ‘Modifying Industrial Operations Protocol’. 24 pp. (Ontario Ministry of Natural Resources)
Prestemon JP, Butry DT, Abt KL, Sutphen R (2010) Net benefits of wildfire prevention education efforts. Forest Science 56, 181–192.
| Net benefits of wildfire prevention education efforts.Crossref | GoogleScholarGoogle Scholar |
Price OF, Bradstock RA (2012) The efficacy of fuel treatment in mitigating property loss during wildfires: insights from analysis of the severity of the catastrophic fires in 2009 in Victoria, Australia. Journal of Environmental Management 113, 146–157.
| The efficacy of fuel treatment in mitigating property loss during wildfires: insights from analysis of the severity of the catastrophic fires in 2009 in Victoria, Australia.Crossref | GoogleScholarGoogle Scholar |
R Core Team (2021) ‘R: A Language and Environment for Statistical Computing.’ (R Foundation for Statistical Computing: Vienna, Austria) Available at https://www.R-project.org/ [Verified 7 August 2021]
Reed WJ, McKelvey KS (2002) Power-law behaviour and parametric models for the size-distribution of forest fires. Ecological Modelling 150, 239–254.
| Power-law behaviour and parametric models for the size-distribution of forest fires.Crossref | GoogleScholarGoogle Scholar |
Ruiz-Mirazo J (2012) Environmental benefits of extensive livestock farming: wildfire prevention and beyond. Options Méditerranéennes 100, 75–82.
Sankey S (2018) ‘Blueprint for Wildland Fire Science in Canada (2019–2029).’ (Natural Resources Canada, Canadian Forest Service, Northern Forestry Centre: Edmonton, AB, Canada)
Schoenberg FP, Peng R, Woods J (2003) On the distribution of wildfire sizes. Environmetrics 14, 583–592.
| On the distribution of wildfire sizes.Crossref | GoogleScholarGoogle Scholar |
Scott JH (2006) An analytical framework for quantifying wildland fire risk and fuel treatment benefit. In ‘Fuel Management – How to Measure Success: Conference Proceedings’, 28–30 March 2006, Portland, OR. (Eds PL Andrews, BW Butler) Proceedings RMRS-P-41, pp. 169–184. (US Department of Agriculture, Forest Service, Rocky Mountain Research Station: Fort Collins, CO, USA)
Thomas D, Butry D, Prestemon J (2013) The effects of wildfire prevention activities. In ‘Proceedings of the Natural Resources Symposium’, Baton Rouge, LA. (Eds TF Shupe, MS Bowen) pp. 101–115. (LSU AgCenter: Baton Rouge, LA, USA)
Varela E, Giergiczny M, Riera P, Mahieu PA, Soliño M (2014) Social preferences for fuel break management programs in Spain: a choice modelling application to prevention of forest fires. International Journal of Wildland Fire 23, 281–289.
| Social preferences for fuel break management programs in Spain: a choice modelling application to prevention of forest fires.Crossref | GoogleScholarGoogle Scholar |
Wang X, Studens K, Parisien MA, Taylor SW, Candau JN, Boulanger Y, Flannigan MD (2020) Projected changes in fire size from daily spread potential in Canada over the 21st century. Environmental Research Letters 15, 104048
| Projected changes in fire size from daily spread potential in Canada over the 21st century.Crossref | GoogleScholarGoogle Scholar |
Woolford DG, Martell DL, McFayden CB, Evens J, Stacey A, Wotton BM, Boychuk D (2021) The development and implementation of a human-caused wildland fire occurrence prediction system for the province of Ontario, Canada. Canadian Journal of Forest Research 51, 303–325.
| The development and implementation of a human-caused wildland fire occurrence prediction system for the province of Ontario, Canada.Crossref | GoogleScholarGoogle Scholar |
Wotton BM, Martell DL (2005) A lightning fire occurrence model for Ontario. Canadian Journal of Forest Research 35, 1389–1401.
| A lightning fire occurrence model for Ontario.Crossref | GoogleScholarGoogle Scholar |
Appendix A: The Kruskal–Wallis test
The Kruskal–Wallis test (Kruskal and Wallis 1952) of whether two or more samples are from the same distribution may be conducted as follows. To begin, observed values across all samples are arranged in an increasing order and assigned a rank based on this order (e.g. the smallest value gets a rank of one, the second smallest a rank of two, etc.). If there exists one or more groups of tied values, each value within a tied group is assigned the average rank of all observations in that group. Letting C denote the number of samples and G denote the number of unique numerical values observed amongst all samples, we define the following:
ni: the number of observations in the ith sample, i = 1, 2, …, C.
: the total number of observations across all samples.
: the average rank of the ni observations in the ith sample.
tj: the number of observations across all samples that are tied at the jth unique observed value, j = 1, 2, …, G.
Now, letting Tj = (tj − 1)tj(tj + 1), j = 1, 2, …, G, the KW test statistic equals

Note that if there are no ties in the data, each Tj = 0, which simplifies the above form. Under the assumption that the null hypothesis of identical distributions is true, so long as the sample sizes ni are not small, the test statistic H approximately follows a χ2(C − 1) distribution, which may be used with the observed value of H to calculate the KW test’s P-value.