A long trend of sexually transmitted diseases before and after the COVID-19 pandemic in China (2010–21)
Aifang Xu A # , Zhongbao Zuo A # , Chunli Yang B # , Fei Ye C , Miaochan Wang A , Jing Wu A , Chengjing Tao D , Yunhao Xun E , Zhaoyi Li F , Shourong Liu E and Jinsong Huang
A Department of Clinical Laboratory, Hangzhou Xixi Hospital, Hangzhou, Zhejiang 310023, China.
B Department of Clinical Laboratory, The 903rd Hospital of PLA, Hangzhou, Zhejiang 310013, China.
C Health Examination Center, Hangzhou Xixi Hospital, Hangzhou, Zhejiang 310023, China.
D Department of Obstetrics and Gynecology, Hangzhou Xixi Hospital, Hangzhou, Zhejiang 310023, China.
E Department of Hepatology, Hangzhou Xixi Hospital, Hangzhou, Zhejiang 310023, China.
F Science and Education Department, Hangzhou Xixi Hospital, Hangzhou, Zhejiang 310023, China.
Sexual Health - https://doi.org/10.1071/SH22172
Submitted: 12 October 2022 Accepted: 4 August 2023 Published online: 29 August 2023
© 2023 The Author(s) (or their employer(s)). Published by CSIRO Publishing. This is an open access article distributed under the Creative Commons Attribution-NonCommercial-NoDerivatives 4.0 International License (CC BY-NC-ND)
Abstract
Background: The longer ongoing benefits of coronavirus disease 2019 (COVID-19) non-pharmaceutical interventions (NPIs) for sexually transmitted diseases (STDs) in China are still unclear. We aimed to explore the changes in five STDs (AIDS, hepatitis B, hepatitis C, gonorrhoea, and syphilis) before, during, and after the COVID-19 pandemic in mainland China, from 2010 to 2021.
Methods: The number of the monthly reported cases of the five STDs were extracted from the website to construct the Joinpoint regression and autoregressive integrated moving average (ARIMA) models. Eight indicators reflecting NPIs were chosen from the COVID-19 Government Response Tracker system. The STDs and eight indicators were used to establish the Multivariable generalised linear model (GLM) to calculate the incidence rate ratios (IRRs).
Results: With the exception of hepatitis B, the other four STDs (AIDS, hepatitis C, gonorrhoea, and syphilis) had a positive average annual percent change over the past 12 years. All the ARIMA models had passed the Ljung–Box test, and the predicted data fit well with the data from 2010 to 2019. All five STDs were significantly reduced in 2020 compared with 2019, with significant estimated IRRs ranging from 0.88 to 0.92. In the GLM, using data for the years 2020 (February–December) and 2021, the IRRs were not significant after adjusting for the eight indicators in multivariate analysis.
Conclusion: Our study demonstrated that the incidence of the five STDs decreased rapidly during the COVID-19 pandemic in 2020. A recovery of STDs in 2021 was found to occur compared with that in 2020, but the rising trend disappeared after adjusting for the NPIs. Our study demonstrated that NPIs have an effect on STDs, but the relaxation of NPI usage might lead to a resurgence.
Keywords: AIDS, COVID-19, epidemiology, gonorrhoea, hepatitis B, hepatitis C, non-pharmaceutical interventions, sexually transmitted diseases, syphilis.
Introduction
The first confirmed Chinese coronavirus disease 2019 (COVID-19) patient was reported in December 2019,1,2 and the disease caused a pandemic in China within a few months. COVID-19 quickly spread around the world,3–6 and the number of COVID-19 cases has exceeded 579 million worldwide, involving more than 200 countries.7 China implemented some public interventions to prevent the pandemic, including pharmaceutical measures (vaccines, antibodies, ventilators, etc.) and non-pharmaceutical measures (wearing masks, travel restrictions, school closing, cancel public events, stay-at-home requirements, etc.). Although pharmaceutical measures can target specific pathogens, non-pharmaceutical interventions (NPIs) affect a wide range of infectious diseases. Recent studies8–10 have shown that NPIs (lockdown, restrictions on gatherings, etc.) during the COVID-19 pandemic might reduce the prevalence of sexually transmitted diseases (STDs). Similar outcomes were also seen for tuberculosis,11 seasonal influenza,12 and some other diseases.11,13,14 Forty notifiable diseases need to be monitored in the China Information System for Disease Control and Prevention, especially in the context of COVID-19.
Some studies8–10 have explored the impact of NPIs on STDs during the COVID-19 pandemic; however, these studies only focused on the year 2020 when COVID-19 first began to spread widely, and when strict NPIs were being used. The NPIs persisted for the years 2020 and 2021, but changed with the control levels when the pandemic subsided. The Chinese government adopted relaxed control measures to maintain social activities and the economy in 2021, but the incidence of the STDs was still unknown in the context of COVID-19. At the same time, we assumed that the numbers of STDs would rise with the relaxation of NPIs, but the rising numbers were due to the diseases themselves or the fact that the effect of relaxation of NPIs was still unknown. In this study, we aimed to explore the changes in five STDs (AIDS, hepatitis B, hepatitis C, gonorrhoea, and syphilis) before, during, and after the COVID-19 pandemic in mainland China from 2010 to 2021.
Methods
Data collection
The monthly reported number of cases of the five STDs (AIDS, hepatitis B, hepatitis C, gonorrhoea, and syphilis) were extracted from the National Health Commission website (http://www.nhc.gov.cn/jkj/s2907/new_list.shtml). All data published by the Commission were originally from the China Information System for Disease Control and Prevention (CISDCP),15 which was a real-time disease-reporting system covering 40 notifiable infectious diseases (COVID-19 was included in from January 2020). The surveillance system was first established in 2004, covering 397 cities in 31 provinces in mainland China. All notifiable infectious diseases were reported in a timely manner to the local centres for disease control and prevention after diagnosis according to their standard criteria.16 Five STDs without detailed information from the government’s website were included in our research. Demographic statistics data came from the website of the statistical yearbook of the National Bureau of Statistics (http://www.stats.gov.cn/tjsj/ndsj/, available in China).
The study was approved by the institutional ethics review committee at Hangzhou Xixi Hospital (2022 Science Ethic No. 36). Written informed consent was not required because of the retrospective nature of the study.
The confirmed Chinese COVID-19 case datafor 2020 and 2021 came from the 2019 Novel Coronavirus COVID-19 (2019-nCoV) Data Repository provided by Johns Hopkins University.17 The indicators of government control measures were extracted from the COVID-19 Government Response Tracker (GRT).18 Eight indicators were chosen from the GRT system, which were workplace closing, school closing, cancel public events, stay-at-home requirements, restrictions on gathering size, closed public transport, restrictions on internal movement, and international travel controls. The strictness of these NPIs increased with the score of indicators.
Statistical analysis
From 2010 to 2021, the incidence trend of the five STDs was analysed by using Joinpoint software (version 4.8.0.1; National Cancer Institute, Rockville, MD, US). The grid search method was used to find significant trends, and multiple permutation tests can detect the Joinpoint points for each trend.19 The overall time trend was calculated by using the average annual percent change (AAPC).
Autoregressive integrated moving average (ARIMA) models were used to determine the trend of STDs, and we used the forecast::auto.Arima() function in the R software (version 3.6.0) to find a fitted model. Root mean square error (RMSE), mean absolute percentage error (MAPE), Akaike’s information criterion (AIC), and Bayesian information criterion (BIC) were used to evaluate the goodness-of-fit of constructed models. Ljung–Box test was used to check whether the residual of the model was white noise (P > 0.05 for white noise).
The daily national indicators of the eight COVID-19 control measures from the GRT system were newly calculated by using the average score from the 31 provinces, and the monthly national indicators were the average of the daily data. Pearson correlation analysis was conducted to measure the relationship between the eight indicators and the COVID-19 cases, and five STDs. A two-sided P < 0.05 was considered statistically significant.
To adjust for potential confounding factors of NPIs (e.g. long-term disease trends, and indicators of government control measures), some multivariable generalised linear models (GLM) were constructed to explore the impact of NPIs on each STD. The COVID-19 pandemic phases were defined as Phase 1 (2010–2018), Phase 2 (2019), Phase 3 (2020), and Phase 4 (2021), and the reference period is Phase 2 (2019). The X-13ARIMA-SEATS (signal extraction) method was used to obtain seasonality-removed monthly case numbers for each disease.13 SEATS (Seasonal Extraction in ARIMA Time Series) breaks down the time series into seasonal, trend, transitory, and irregular components, assigning deterministic effects to each component. A fundamental assumption made by SEATS is that the linearised time series, yt (log of monthly case numbers in our analysis), follows the ARIMA model.
yt is the time series, is the regression part with covariates xt, αt is the white noise with mean 0 and variance σ, B and Bs are the non-seasonal and seasonal operators, B (yt) = yt − 1, Bs (yt) = yt – 12; reflects a non-seasonal autoregressive (AR) operator of order ρ; reflects the seasonal AR operator of order ρ; is the non-seasonal and seasonal operators of orders d and D; reflects non-seasonal moving average (MA) order of q; and reflects the seasonal MA order of Q.
The holiday effect of Chinese New Year was adjusted for by using the ‘genhol’ function in the R package ‘seasonal’ (ver. 1.8.3). SEATS automatically detects the shifts in the mean level of the time series, which means it can partially account for the impact of NPIs during the COVID-19 pandemic when estimating seasonality. Two outputs were obtained from SEATS; the seasonality-removed monthly case numbers and the seasonal trend itself. The seasonality-removed monthly case numbers were used to construct a GLM with two stages. For stage I, we fitted a GLM with the quasi-Poisson method using the following factors: phase 1–4 indicators for the year 2010–2021, long-term trend, and number of person-days (the number of days × population size) as an offset. For stage II, we extracted the residuals from the stage I model, with 1 month lag as an independent variable to account for autocorrelation. The incidence rate ratios (IRR) estimated by the model of stage II reflect the effects of COVID-19 NPIs on the incidence of five STDs in 2020 and 2021.20 In addition, we selected the pandemic year of 2020 and 2021 to construct a GLM. The IRR of model 1 was not adjusted; the IRR of model 2 was adjusted by using the indicators of school closing, workplace closing, cancel public events, restrictions on gatherings, close public transport, stay at home, restrictions on internal movement, and international travel controls. In model 2, the IRRs of Phase 2 (April 2020–August 2020, the reopening of the school) and Phase 3 (September 2020–December 2021, the later period of the NPIs) compared with Phase 1 (February 2020 and March 2020, the strictest NPIs period) would reflect the effects of COVID-19 NPIs. A sensitivity analysis using the harmonic functions to adjust for seasonality (detailed in the Supplementary material, Table S1) was conducted in this research. All statistical analyses were conducted in Joinpoint software (ver. 4.9.1.0; the National Cancer Institute) and R software (ver. 4.0.5; R Development Core Team 2020). A two-sided P < 0.05 was considered statistically significant.
Results
Time trends of the five STDs
We included 24 547 912 incident cases in mainland China from 2010 to 2021 in our research, with a yearly average of 2 045 659 STDs cases. With the exception of hepatitis B, the other four STDs had a positive AAPC over the past 12 years (Fig. 1). Hepatitis B, gonorrhoea, and syphilis had one slope determined by the Joinpoint regression, with an AAPC of −0.42%, 2.09%, and 2.87% (P < 0.05) (Table 1), respectively. The annual percent change (APC) of AIDS in 2010–2019 was 6.22% (P < 0.05), but it decreased in 2019–2021 with an APC of −6.70% (Table 1). Hepatitis C increased with an APC of 15.60% in 2010–2012, but the APC from 2012 to 2021 was 0.95% (Table 1).
The trend of incidence rates for five STDs from 2010 to 2021 is shown by the Joinpoint software. (a) AIDS, (b) hepatitis B, (c) hepatitis C, (d) gonorrhoea, (e) syphilis.
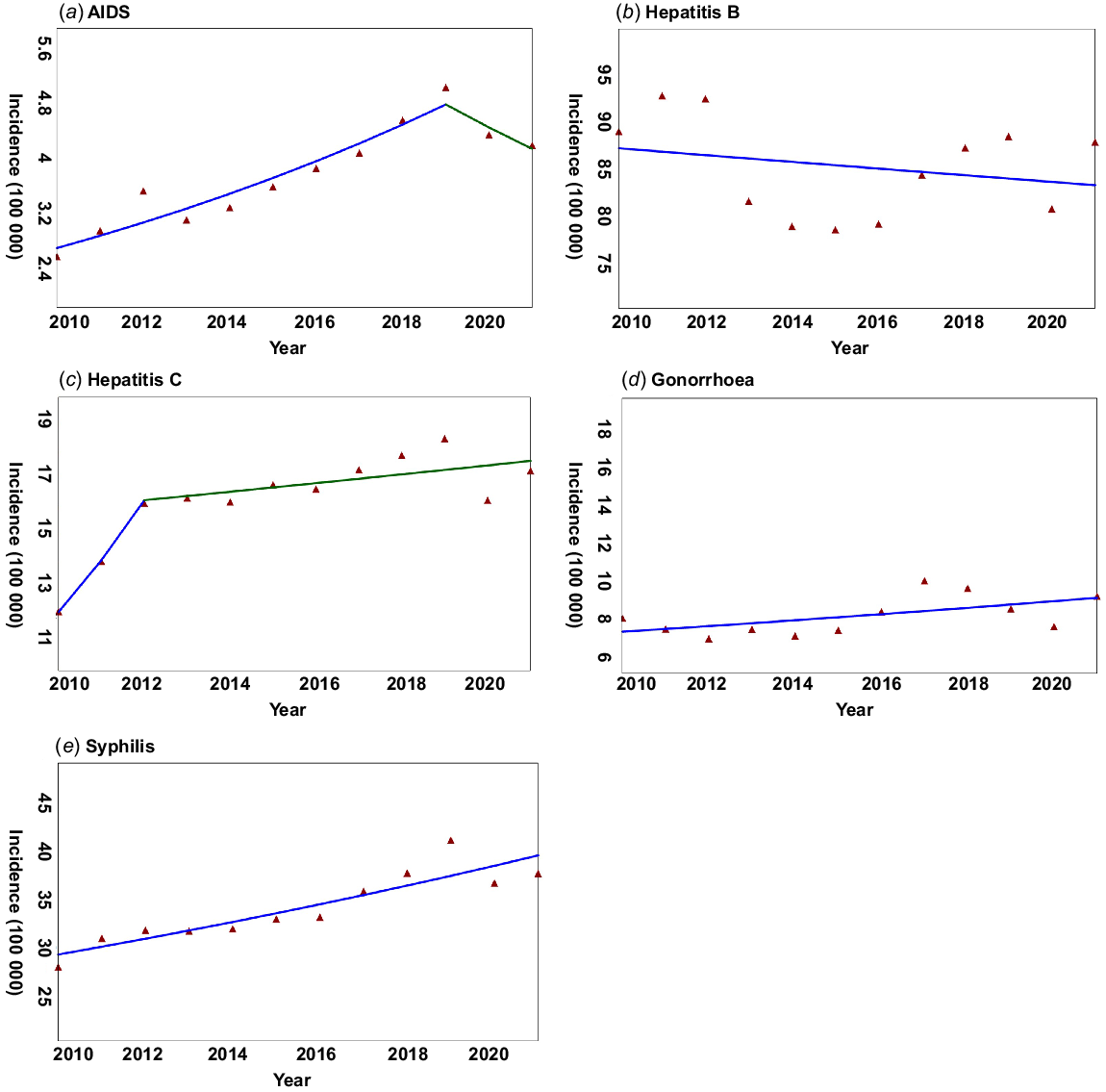
Disease | APC (%) | AAPC (%) |
---|---|---|
AIDS | 2010–2019: 6.22* | 3.7* |
2019–2021: −6.70 | ||
Hepatitis B | 2010–2021: −0.42 | −0.42 |
Hepatitis C | 2010–2012: 15.60 | 3.5* |
2012–2021: 0.95 | ||
Gonorrhoea | 2010–2021: 2.09 | 2.09 |
Syphilis | 2010–2021: 2.87* | 2.87* |
APC, annual percent change; AAPC, average annual percent change; *P < 0.05.
ARIMA model construction and forecast
Using the forecast::auto.arima() function in the R software to find a fitted model, the optimal models for the five STDs are listed in Table 2. All the models had passed the Ljung–Box test, and MAPE showed a good fit for the model. The predicted data fitted well with the actual data from 2010 to 2019 (Fig. 2). The predictions of higher case numbers for hepatitis B, hepatitis C, and syphilis compared with the real data, but AIDS and gonorrhoea showed a different pattern in that the predicted cases were lower than the real numbers.
Disease | Optimal model | Goodness-of-fit | Ljung–Box test | ||||
---|---|---|---|---|---|---|---|
RMSE | MAPE (%) | AIC | BIC | χ2 value | P-value | ||
AIDS | (1,0,1) × (0,1,1)12 | 489.07 | 9.04 | 1668.14 | 1681.55 | 0.01 | 0.92 |
Hepatitis B | (3,1,0) × (2,1,0)12 | 5447.44 | 3.62 | 2179.39 | 2195.43 | <0.001 | 0.99 |
Hepatitis C | (2,1,1) × (2,1,1)12 | 1053.56 | 3.91 | 1842.86 | 1861.57 | 0.08 | 0.78 |
Gonorrhoea | (0,1,3) × (0,1,1)12 | 507.16 | 3.91 | 1673.12 | 1686.48 | <0.001 | 0.98 |
Syphilis | (2,1,2) × (0,1,1)12 | 1794.85 | 3.39 | 1950.4 | 1966.44 | 0.002 | 0.96 |
RMSE, root mean square error; MAPE, mean absolute percentage error; AIC, Akaike’s information criterion; BIC, Bayesian information criterion.
Correlation analysis for COVID-19 cases, five STDs, and eight indicators
All the five STDs were significantly negative with the monthly cases of COVID-19 (Table 3). With the exception of restrictions on internal movement, the other seven NPIs could affect the incidence of STD occurrence. School closing, workplace closing, cancel public events, restrictions on gatherings, close public transport, and stay at home were negatively associated with hepatitis B, hepatitis C, gonorrhoea, and syphilis. The measure of international travel controls had significantly positive correlations with AIDS (r = 0.63, P < 0.01), hepatitis B (r = 0.54, P < 0.05), hepatitis C (r = 0.57, P < 0.01), and syphilis (r = 0.59, P < 0.01) (Table 3).
Variable | AIDS | Hepatitis B | Hepatitis C | Gonorrhoea | Syphilis |
---|---|---|---|---|---|
School closing | −0.16 | −0.60** | −0.58** | −0.78** | −0.51* |
Workplace closing | −0.04 | −0.53* | −0.53* | −0.66** | −0.49* |
Cancel public events | −0.08 | −0.50* | −0.54* | −0.57** | −0.51* |
Restrictions on gatherings | −0.26 | −0.61** | −0.68** | −0.62** | −0.68** |
Close public transport | −0.04 | −0.64** | −0.56** | −0.66** | −0.49* |
Stay at home | −0.17 | −0.75** | −0.71** | −0.74** | −0.67** |
Restrictions on internal movement | 0.11 | −0.19 | −0.26 | −0.04 | −0.34 |
International travel controls | 0.63** | 0.54* | 0.57** | 0.38 | 0.59** |
COVID-19 cases | −0.50* | −0.80** | −0.76** | −0.58** | −0.77** |
*P < 0.05.
**P < 0.01.
Generalised linear model-estimated association of NPIs with disease trend
All five STDs were significantly reduced in phase 3 (2020) compared with phase 2 (2019), with significant estimated IRRs ranging from 0.88 to 0.92 (Table 4). The incidences of AIDS, gonorrhoea, and syphilis were significantly reduced in phase 4 (2021), with IRRs <1 compared with phase 2, but there were no statistically significant IRRs for hepatitis B and hepatitis C. In phase 1 (2010–2018), the significant IRRs for AIDS, hepatitis C, gonorrhoea, and syphilis were 0.72 (0.67–0.77), 0.87 (0.83–0.92), 0.94 (0.9–0.99) and 0.79 (0.76–0.83), respectively. The sensitivity analysis using harmonic functions showed qualitatively similar results to the primary analysis (Supplementary Table S1).
Disease | Phase 2 (Control group) (2019) reported cases | Phase 1 (2010–2018) | Phase 3 (2020) | Phase 4 (2021) | |||
---|---|---|---|---|---|---|---|
IRR (95%CI) | P-value | IRR (95%CI) | P-value | IRR (95%CI) | P-value | ||
AIDS | 72 630 | 0.72 (0.67–0.77)* | <0.001 | 0.89 (0.81–0.97)* | 0.009 | 0.87 (0.79–0.95)* | 0.003 |
Hepatitis B | 1 247 092 | 0.96 (0.93–1) | 0.07 | 0.92 (0.87–0.97)* | 0.004 | 1 (0.95–1.05) | 0.98 |
Hepatitis C | 260 704 | 0.87 (0.83–0.92)* | <0.001 | 0.89 (0.83–0.96)* | 0.001 | 0.94 (0.88–1) | 0.07 |
Gonorrhoea | 120 146 | 0.94 (0.9–0.99)* | 0.01 | 0.88 (0.83–0.94)* | <0.001 | 1.07 (1–1.13)* | 0.05 |
Syphilis | 587 402 | 0.79 (0.76–0.83)* | <0.001 | 0.9 (0.84–0.95)* | <0.001 | 0.91 (0.86–0.97)* | 0.003 |
Generalised linear models (GLM) were used for estimating the IRRs of five STDs. The time series method removed the seasonality of the reported cases in this model. IRR < 1 with P < 0.05 indicates a significant decline in incidence rate in the year 2020 compared to the year 2019. All P-values are two-sided and not adjusted for multiple comparisons. The reference period is the year 2019. *P < 0.05. CI, confidence interval.
Eight indicators from the GRT system reflecting the NPIs were included to construct the models for the years 2020 and 2021, and the reference period is February 2020 and March 2020. In the primary univariate analysis of Model 1 (Table 5), the IRR for gonorrhoea was significantly above 1 for phase 2, but the IRRs of Model 2 in Phase 2 were not statistically significant after adjusting for the indicators. In Phase 3, hepatitis B and gonorrhoea were significantly different from Phase 1 (February 2020 and March 2020) with IRRs above 1, but the statistical significance disappeared after adjusting for the eight indicators.
Disease | IRR (95%CI) of Phase 2 | IRR (95%CI) of Phase 3 | ||
---|---|---|---|---|
Model 1 | Model 2 | Model 1 | Model 2 | |
AIDS | 1.25 (0.72–2.18) | 1 (0.30–3.35) | 1.12 (0.67–1.88) | 1.37 (0.45–4.15) |
Hepatitis B | 1.16 (0.97–1.39) | 0.97 (0.61–1.55) | 1.18 (1–1.39)* | 1.03 (0.67–1.59) |
Hepatitis C | 1.23 (0.97–1.56) | 1.01 (0.59–1.72) | 1.23 (0.99–1.52) | 1.06 (0.64–1.74) |
Gonorrhoea | 1.91 (1.37–2.67)* | 0.72 (0.42–1.22) | 2.32 (1.68–3.21)* | 0.84 (0.51–1.4) |
Syphilis | 1.1 (0.88–1.38) | 0.84 (0.53–1.33) | 1.06 (0.86–1.31) | 0.89 (0.58–1.36) |
Generalised linear models (GLM) were used for estimating the IRRs for five STDs. The IRR of Model 1 was not adjusted; The IRR of Model 2 was adjusted by the eight indicators: school closing, workplace closing, cancel public events, restrictions on gatherings, close public transport, stay at home, restrictions on internal movement, and international travel controls. All P-values are two-sided and not adjusted for multiple comparisons. Phase 2: April 2020–August 2020; Phase 3: September 2020–December 2021. The reference period is February 2020 and March 2020; January 2020 was not included in our analysis because of incomplete information on eight indicators. *P < 0.05.
Discussion
The changing incidences of the five STDs varied from 2010 to 2021, especially in the implementation and subsequent relaxation of NPIs aimed at alleviating the COVID-19 pandemic. In incidence rates for all the five STDs dramatically decreased in 2020 compared with 2019. But later in 2021, the numbers for the five STDs were on track to match and surpass the 2019 totals when the NPIs were relaxed.
The sharply decreasing observed case numbers in 2020 were not surprising, especially in the strictest months of NPIs (February–March 2020). As China urgently requisitioned many infectious disease hospitals to help combat the number of COVID-19 infections, one possible explanation for the decreasing STD cases in 2020 is the decline in STD screening and diagnosis. During the COVID-19 pandemic, many hospitals either closed completely to help fight COVID-19 or restricted personal visits to symptomatic cases.21–24 Some STDs, such as HIV infection, gonococcal infections, and urogenital chlamydial infections among women, were often asymptomatic, and screening is required to determine infection.25 In addition, the susceptible population could avoid or delay routine health visits due to adherence to the NPIs, reducing the exposure to COVID-19.26 At present, there are no nationally representative data on STD screening coverage in China, so it is impossible to quantify changes in national-level screening. Researchers in the US found that the hepatitis C virus antibody testing volume decreased 59% during April 2020 compared with results from 2018 and 2019,27 which is similar to the situation in China during this COVID-19 pandemic. Given the combined impact of these factors, the devastating impact of the COVID-19 pandemic on the capacity of medical clinics and patients’ access to screening and testing might significantly reduce the number of reported positive cases of STDs.
Although the decreasing STD screening and testing might result in a reduction of reported positive cases, the true decrease in STD cases might be attributed to the NPIs intended to mitigate the COVID-19 pandemic. With the strict NPIs, an individual would find it difficult to use public transport to get to medical clinics, and then obtain medical services. Meanwhile, people who went to hospital were at risk of being infected with COVID-19, and seeking medical service would also be a concern for their families who know about their STD status. The reduction of STD clinic visits was common during the pandemic. The study by Geng et al.13 found a significant decrease in health-seeking behaviour during the pandemic. The research by Tao et al.28 showed an 84% decline in total absolute clinic visits, a 100% reduction in screening visits, and a 77% reduction in treatment visits in the COVID-19 plateau phase compared to pre-COVID-19. In addition, the NPIs could also affect the seeking of sexual partners and high-risk sexual behaviours. A study conducted in China29 found a 44% reduction in the number of sexual partners during the COVID-19 pandemic among the general population, and research from Amsterdam30 showed a 73% decrease in the reported number of casual sex partners for the men who have sex with men (MSM).
Previous studies8–10,24,31 found that the incidence of STDs decreased dramatically in 2020, which was also found in our research. The IRRs of the five STDs were significantly below one in 2020 compared with 2019, but there was no statistical significance for the IRRs of hepatitis B, hepatitis C, and gonorrhoea in 2021 (Table 4). Many studies11,13,24 have reported the recovery of the incidence rates for infectious diseases when NPIs were relaxed, and our research also observed three STD incidence levels (hepatitis B, hepatitis C, and gonorrhoea) return to pre-COVID-19 levels. The recovery of the gonorrhoea incidence level was relatively quick compared to the other four STDs, which could be explained by the shorter incubation period (1–14 days) and obvious clinical symptoms when present.32 Furthermore, studies from Denmark33 and Taiwan34 found an increasing number of cases of gonorrhoea during the COVID-19 lockdown, which might be explained by their unsuccessful lockdown measures and the subsequent increasing risk of unsafe sexual behaviour. A quick recovery of the gonorrhoea incidence rate after the lockdown was also observed.
To quantify the effect of NPIs on the transmission of STDs, eight indicators from the GRT system were extracted. Only international travel control was positively correlated with the five STDs, which means stopping travel into the country by foreigners can help China control the STD incidence rates to a certain extent. The other seven measures, such as school closing, workplace closing, etc., were intended to limit the gathering of people, also had an impact on STD incidence. We then constructed a GLM with eight indicators for the years 2020 and 2021 corresponding to the COVID-19 pandemic and found IRRs were not significant after adjusting for the eight control measures (Table 5). Previous research10,13 showed the recovery of STDs when the NPIs were relaxed, and this was determined by the lockdown period, but our research found the rising trend disappeared in the post-lockdown period after adjusting for the eight indicators. Hence, our research demonstrated the recovery of the STDs was caused by the relaxation of NPIs.
Two differences can be found between previous research and our study. First is the way that NPIs were evaluated. Many studies10,13 evaluated NPIs by classifying them based on some major social events, but we evaluated NPIs through quantitative indicators, which can be more objective to reflect the impact of NPIs. Second, many studies8–10,13 evaluated infectious diseases in the pandemic year of 2020 and before, whereas our study extended the collection of disease data to 2021. Our study demonstrates that the implementation of NPIs can effectively block the spread of STDs, but the highly destructive NPIs cannot be used as long-term solutions because they can have a huge effect on social activities and the economy. Many countries in the world have used different measures to deal with the COVID-19 pandemic, and they have had to decide whether to maintain normal operations within a society or introduce and maintain NPIs to prevent disease spread. However, combining the less destructive NPIs with effective vaccines and treatment schemes, such as social distancing and wearing masks when using public transport, might be a better solution to stop the spread of infectious diseases.
Our study has some limitations. First, the monthly data we collected from the government website waere originally from the China Information System for Disease Control and Prevention, which inevitably had a bias. Patients with a sexually transmitted disease cannot be diagnosed by medical services, which cause missing reports and data, and thus the actual number of STD cases could be underestimated. Second, many variables could affect the prevalence of STDs, such as population vaccination levels, climate change, and virus variation, but we only included eight indicators of NPIs. More variables should be included to specify the impact of other NPIs in the future. Third, the monthly data were taken from national data, and thus were not specific to provinces or cities, which might neglect the spatial heterogeneity of disease patterns.
In conclusion, our study demonstrated that the incidence of five STDs decreased rapidly during the COVID-19 pandemic in 2020. Therecovery of the incidence rates of these STDs in 2021 showed that, compared with the rates in 2020, the rising trend disappeared after adjusting for the NPIs. Our study showed that NPIs have an effect on STD incidence, but the relaxation of NPIs might lead to a resurgence.
Data availability
All data and code are available on the following online site (https://osf.io/dc92e/).
Declaration of funding
This research was funded by a Zhejiang Medical and Health Science and Technology Plan Project (2022KY1028), Hangzhou Science and Technology Bureau (2021WJCY185), and a Hangzhou Science and Technology Development plan (202004A20). The funders had no role in the study design, data collection, and analysis, decision to publish, or preparation of the manuscript.
Acknowledgements
The authors thank all the clinical personnel for investigating and reporting the information about the infectious cases.
References
1 Huang C, Wang Y, Li X, Ren L, Zhao J, Hu Y, et al. Clinical features of patients infected with 2019 novel coronavirus in Wuhan, China. Lancet 2020; 395(10223): 497-506.
| Crossref | Google Scholar | PubMed |
2 Li J, Gong X, Wang Z, Chen R, Li T, Zeng D, et al. Clinical features of familial clustering in patients infected with 2019 novel coronavirus in Wuhan, China. Virus Res 2020; 286: 198043.
| Crossref | Google Scholar | PubMed |
3 Spina S, Marrazzo F, Migliari M, Stucchi R, Sforza A, Fumagalli R. The response of Milan’s Emergency Medical System to the COVID-19 outbreak in Italy. Lancet 2020; 395(10227): e49-e50.
| Crossref | Google Scholar | PubMed |
4 Tsang HF, Chan LWC, Cho WCS, Yu ACS, Yim AKY, Chan AKC, et al. An update on COVID-19 pandemic: the epidemiology, pathogenesis, prevention and treatment strategies. Expert Rev Anti Infect Ther 2021; 19(7): 877-88.
| Crossref | Google Scholar | PubMed |
5 Saalim K, Sakyi KS, Fatema-Tuz-Zohra , Morrison E, Owusu P, Dalglish SL, et al. Reported health and social consequences of the COVID-19 pandemic on vulnerable populations and implemented solutions in six West African countries: a media content analysis. PLoS ONE 2021; 16(6): e0252890.
| Crossref | Google Scholar | PubMed |
6 Bonnet E, Bodson O, Le Marcis F, Faye A, Sambieni NE, Fournet F, et al. The COVID-19 pandemic in francophone West Africa: from the first cases to responses in seven countries. BMC Public Health 2021; 21(1): 1490.
| Crossref | Google Scholar | PubMed |
7 World Health Organization. WHO Coronavirus (COVID-19) Dashboard 2022. 2023. Available at https://covid19.who.int/
8 Latini A, Magri F, Donà MG, Giuliani M, Cristaudo A, Zaccarelli M. Is COVID-19 affecting the epidemiology of STIs? The experience of syphilis in Rome. Sex Transm Infect 2021; 97(1): 78.
| Crossref | Google Scholar | PubMed |
9 de Miguel Buckley R, Trigo E, de la Calle-Prieto F, Arsuaga M, Díaz-Menéndez M. Social distancing to combat COVID-19 led to a marked decrease in food-borne infections and sexually transmitted diseases in Spain. J Travel Med 2020; 27(8): taaa134.
| Crossref | Google Scholar |
10 Yan X, Wang X, Zhang X, Wang L, Zhang B, Jia Z. The epidemic of sexually transmitted diseases under the influence of COVID-19 in China. Front Public Health 2021; 9: 737817.
| Crossref | Google Scholar | PubMed |
11 Chen B, Wang M, Huang X, Xie M, Pan L, Liu H, et al. Changes in incidence of notifiable infectious diseases in china under the prevention and control measures of COVID-19. Front Public Health 2021; 9: 728768.
| Crossref | Google Scholar | PubMed |
12 Feng L, Zhang T, Wang Q, Xie Y, Peng Z, Zheng J, et al. Impact of COVID-19 outbreaks and interventions on influenza in China and the United States. Nat Commun 2021; 12(1): 3249.
| Crossref | Google Scholar | PubMed |
13 Geng MJ, Zhang HY, Yu LJ, Lv CL, Wang T, Che TL, et al. Changes in notifiable infectious disease incidence in China during the COVID-19 pandemic. Nat Commun 2021; 12(1): 6923.
| Crossref | Google Scholar | PubMed |
14 Dadras O, Alinaghi SAS, Karimi A, MohsseniPour M, Barzegary A, Vahedi F, et al. Effects of COVID-19 prevention procedures on other common infections: a systematic review. Eur J Med Res 2021; 26(1): 67 PMID:.
| Crossref | Google Scholar | PubMed |
15 Wang L, Wang Y, Jin S, Wu Z, Chin DP, Koplan JP, et al. Emergence and control of infectious diseases in China. Lancet 2008; 372(9649): 1598-605.
| Crossref | Google Scholar | PubMed |
16 Dong Y, Wang L, Burgner DP, Miller JE, Song Y, Ren X, et al. Infectious diseases in children and adolescents in China: analysis of national surveillance data from 2008 to 2017. BMJ 2020; 369: m1043.
| Crossref | Google Scholar |
17 Dong E, Du H, Gardner L. An interactive web-based dashboard to track COVID-19 in real time. Lancet Infect Dis 2020; 20(5): 533-4.
| Crossref | Google Scholar | PubMed |
18 Hale T, Angrist N, Goldszmidt R, Kira B, Petherick A, Phillips T, et al. A global panel database of pandemic policies (Oxford COVID-19 Government Response Tracker). Nat Hum Behav 2021; 5(4): 529-38.
| Crossref | Google Scholar | PubMed |
19 Zuo Z, Wang M, Cui H, Wang Y, Wu J, Qi J, et al. Spatiotemporal characteristics and the epidemiology of tuberculosis in China from 2004 to 2017 by the nationwide surveillance system. BMC Public Health 2020; 20(1): 1284.
| Crossref | Google Scholar |
20 Imai C, Armstrong B, Chalabi Z, Mangtani P, Hashizume M. Time series regression model for infectious disease and weather. Environ Res 2015; 142: 319-27.
| Crossref | Google Scholar | PubMed |
21 Xiong S, Liu L, Lin F, Shi J, Han L, Liu H, et al. Clinical characteristics of 116 hospitalized patients with COVID-19 in Wuhan, China: a single-centered, retrospective, observational study. BMC Infect Dis 2020; 20(1): 787.
| Crossref | Google Scholar | PubMed |
22 Xu X-W, Wu X-X, Jiang X-G, Xu K-J, Ying L-J, Ma C-L, et al. Clinical findings in a group of patients infected with the 2019 novel coronavirus (SARS-Cov-2) outside of Wuhan, China: retrospective case series. BMJ 2020; 368: m606.
| Crossref | Google Scholar |
23 Wang D, Hu B, Hu C, Zhu F, Liu X, Zhang J, et al. Clinical characteristics of 138 hospitalized patients with 2019 novel coronavirus-infected pneumonia in Wuhan, China. JAMA 2020; 323(11): 1061-9.
| Crossref | Google Scholar | PubMed |
24 Yan J, Li Y, Zhou P. Impact of COVID-19 pandemic on the epidemiology of STDs in China: based on the GM (1,1) model. BMC Infect Dis 2022; 22(1): 519.
| Crossref | Google Scholar | PubMed |
25 Jones J, Le Guillou A, Gift TL, Chesson H, Bernstein KT, Delaney KP, et al. Effect of screening and treatment for gonorrhea and chlamydia on HIV Incidence among men who have sex with men in the United States: a modeling analysis. Sex Transm Dis 2022; 49: 669-76.
| Crossref | Google Scholar |
26 Ogunbodede OT, Zablotska-Manos I, Lewis DA. Potential and demonstrated impacts of the COVID-19 pandemic on sexually transmissible infections. Curr Opin Infect Dis 2021; 34(1): 56-61.
| Crossref | Google Scholar | PubMed |
27 Kaufman HW, Bull-Otterson L, Meyer WA, III, Huang X, Doshani M, Thompson WW, et al. Decreases in Hepatitis C testing and treatment during the COVID-19 pandemic. Am J Prev Med 2021; 61(3): 369-76.
| Crossref | Google Scholar | PubMed |
28 Tao J, Napoleon SC, Maynard MA, Almonte A, Silva E, Toma E, et al. Impact of the COVID-19 pandemic on sexually transmitted infection clinic visits. Sex Transm Dis 2021; 48(1): e5-e7.
| Crossref | Google Scholar | PubMed |
29 Li W, Li G, Xin C, Wang Y, Yang S. Challenges in the practice of sexual medicine in the time of COVID-19 in China. J Sex Med 2020; 17(7): 1225-8.
| Crossref | Google Scholar | PubMed |
30 van Bilsen WPH, Zimmermann HML, Boyd A, Coyer L, van der Hoek L, Kootstra NA, et al. Sexual behavior and its determinants during COVID-19 restrictions among men who have sex with men in Amsterdam. J Acquir Immune Defic Syndr 2021; 86(3): 288-96.
| Crossref | Google Scholar |
31 Whitlock GG, McOwan A, Nugent D. Gonorrhoea during COVID-19 in London, UK. Sex Transm Infect 2021; 97(8): 622-3.
| Crossref | Google Scholar | PubMed |
32 Harrison WO, Hooper RR, Wiesner PJ, Campbell AF, Karney WW, Reynolds GH, et al. A trial of minocycline given after exposure to prevent gonorrhea. N Engl J Med 1979; 300(19): 1074-8.
| Crossref | Google Scholar | PubMed |
33 Heerfordt IM. STIs during the first and second wave of COVID-19 in Denmark. Sex Transm Infect 2022; 98(2): 150-1.
| Crossref | Google Scholar | PubMed |
34 Lee K-K, Lai C-C, Chao C-M, Tang H-J. Increase in sexually transmitted infection during the COVID-19 pandemic in Taiwan. J Eur Acad Dermatol Venereol 2021; 35(3): e171-e2.
| Crossref | Google Scholar | PubMed |